the Creative Commons Attribution 4.0 License.
the Creative Commons Attribution 4.0 License.
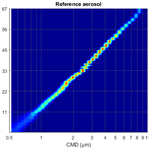
Laboratory evaluation of particle-size selectivity of optical low-cost particulate matter sensors
Timo Mäkelä
Minna Aurela
Kimmo Teinilä
Samu Varjonen
Óscar González
Hilkka Timonen
Low-cost particulate matter (PM) sensors have been under investigation as it has been hypothesized that the use of low-cost and easy-to-use sensors could allow cost-efficient extension of the currently sparse measurement coverage. While the majority of the existing literature highlights that low-cost sensors can indeed be a valuable addition to the list of commonly used measurement tools, it often reiterates that the risk of sensor misuse is still high and that the data obtained from the sensors are only representative of the specific site and its ambient conditions. This implies that there are underlying reasons for inaccuracies in sensor measurements that have yet to be characterized. The objective of this study is to investigate the particle-size selectivity of low-cost sensors. Evaluated sensors were Plantower PMS5003, Nova SDS011, Sensirion SPS30, Sharp GP2Y1010AU0F, Shinyei PPD42NS, and Omron B5W-LD0101. The investigation of size selectivity was carried out in the laboratory using a novel reference aerosol generation system capable of steadily producing monodisperse particles of different sizes (from ∼0.55 to 8.4 µm) on-line. The results of the study show that none of the low-cost sensors adhered to the detection ranges declared by the manufacturers; moreover, cursory comparison to a mid-cost aerosol size spectrometer (Grimm 1.108, 2020) indicates that the sensors can only achieve independent responses for one or two size bins, whereas the spectrometer can sufficiently characterize particles with 15 different size bins. These observations provide insight into and evidence of the notion that particle-size selectivity has an essential role in the analysis of the sources of errors in sensors.
- Article
(1143 KB) - Full-text XML
-
Supplement
(885 KB) - BibTeX
- EndNote
The recent emergence of low-cost sensors has enabled new possibilities in traditional air quality monitoring (Kumar et al., 2015; Morawska et al., 2018; Snyder et al., 2013). As a result of low unit costs and compact size, sensors can be deployed to the field in much higher quantities than before, thus enabling higher-resolution spatiotemporal data. Few studies have demonstrated applications of sensor networks (Caubel et al., 2019; Feinberg et al., 2019; Gao et al., 2015; Jiao et al., 2016; Popoola et al., 2018; Yuval et al., 2019). Distributed sensing of air quality can be seen as an important progression towards a more comprehensive understanding of city-scale air quality dynamics as air pollution, and particulate matter (PM) in particular, may have highly localized concentration “hot spots” in urban areas. Practical limitations, such as expensiveness and bulkiness, constrain the use of conventional instrumentation in monitoring networks; therefore, low-cost sensors could have an essential role in the spatial extension of measurement coverage.
Numerous field studies have been conducted previously; the majority have underlined the potential usefulness of optical particulate matter sensors (Karagulian et al., 2019; Rai et al., 2017). However, the literature has also emphasized that the risk of sensor misuse is still high and that some external factors, such as relative humidity, may produce significant measurement artifacts in the data (Jayaratne et al., 2018; Kuula et al., 2018; Liu et al., 2019). In comparison to gas sensing, PM measurements are notably more challenging when ambient particle sizes and their respective distributions vary significantly from source to source and from location to location. Along with size, particle physical properties such as shape and refractive index also affect the sensor output. Several studies have pointed out that along with dynamic adjustment for meteorological parameters, on-site calibrations are required in order to achieve higher levels of accuracy and precision (Zheng et al., 2018). However, when considering advanced calibration techniques, Schneider et al. (2019) have raised a valid point noting that it may be unclear whether the sensor data resulting from complex correction and conversion processes (e.g., machine learning) are still a legitimate and independent product of the sensor measurement and not a combination of secondary data and statistical model prediction. This is an important remark when evaluating the usability of sensors as it highlights the need to identify the reasons behind inaccuracies in low-cost sensor measurements.
While field evaluations are a natural step towards understanding and developing sensors, they provide limited information about the detailed sensor response characteristics. In particular, less attention has been paid to the investigation of particle-size discrimination of sensors. Although a few studies have noted that the detectable particle-size ranges of sensors may be significantly different from the ones declared in their respective technical specification sheets (Budde et al., 2018; Levy Zamora et al., 2019), this factor is not commonly considered when assessing sensor accuracy. Thus, more research is needed. The objective of this study was to investigate and characterize the size selectiveness of some of the optical low-cost sensors commonly appearing in the literature. The evaluated sensors were Plantower PMS5003, Nova SDS011, Sensirion SPS30, Sharp GP2Y1010AU0F, Shinyei PPD42NS, and Omron B5W-LD0101. Along with these low-cost sensors, a mid-cost optical aerosol size spectrometer (Grimm model 1.108, Grimm Aerosol Technik GmbH, Germany) was evaluated cursorily to highlight the differences between the responses of low-cost and mid-cost devices. The investigation of size selectivity was carried out in the laboratory using a novel reference aerosol generation system capable of steadily producing monodisperse particles of different sizes. Sensor responses were compared to a reference instrument (APS, aerodynamic particle sizer 3321, TSI Inc., USA), and detectable particle-size ranges of the sensors were obtained.
2.1 Evaluated sensors
The sensors evaluated in this study, and their main detection properties, are listed in Table 1. The optical detection configurations of these sensors were arranged in either a 90 or 120∘ scattering angle, and either a red laser or an infrared (IR) light-emitting diode (LED) was used as a light source. Sensors utilizing an LED were equipped with additional light focusing lenses. The optical chamber itself was composed of an injection-molded plastic body which was placed onto an electronic circuit board. The PMS5003, SDS011, and SPS30 use fans to generate sample flow, whereas the PPD42 and B5W utilized natural convection resulting from a heating resistor. The sampling of the GP2Y1010AU0F was based on diffusion. The optical configurations and plastic body layouts are shown in Fig. S1 in the Supplement. Three units for each sensor model were evaluated in order to assess their inter-unit variation.
Table 1Basic features of the evaluated sensors declared by the manufacturers.
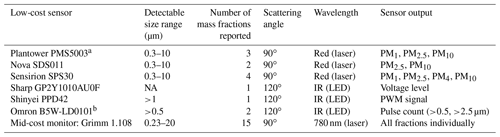
a Standard particle (CF =1) output was used. b Manually adjusted threshold voltage was set to 0.5 V as recommended by the manufacturer.
The mid-cost Grimm 1.108 spectrometer, which was used here for demonstration purposes, is an optical aerosol size spectrometer with 15 size bins (from 0.23 to 20 µm). Previous evaluations of the Grimm 1.108 spectrometer have shown its response to be similar to that of the APS (Peters et al., 2006); furthermore, its accuracy (mass of C-factor-adjusted total suspended particles) is comparable to that of mass measurement methods such as the filter weighing method (Burkart et al., 2010).
2.2 Reference aerosol
2.2.1 Vibrating orifice aerosol generator and gradient elution pump
The aerosol sampled by the low-cost sensors was generated using a vibrating orifice aerosol generator 3450 (VOAG, TSI Inc., USA). The operating principle of the VOAG is based on the instability and breakup of a cylindrical liquid jet. Mechanical disturbances of a resonance frequency vibration disintegrate the cylindrical jet into uniform droplets, which are dispersed into an aerosol flow system with appropriate dilution air. Dispersed droplets evaporate before significant coagulation occurs and form particles from the non-volatile solute dissolved in the volatile liquid. If the droplet liquid is non-volatile, the particle diameter and droplet diameter are equal. Otherwise, the produced particle size is calculable from the volumetric fraction of the non-volatile solute, as shown in Eqs. (1)–(2):
where Dd is the generated droplet diameter, Q is the solution feed rate, and f is the disturbance frequency.
where Dp is the diameter of the formed particle, C is the volumetric concentration of the non-volatile solute in the volatile liquid (typically 2-propanol or purified water), and I is the volumetric fraction of impurity in the volatile liquid.
According to Berglund and Liu (1973), the output aerosol number concentration of the VOAG has a relative standard deviation of less than 3 %, and the formed particle-size distribution is monodisperse having a geometric standard deviation (GSD) less than 1.014. These, and particularly the capability to produce highly monodisperse size distribution, are important features regarding sensor size selectivity evaluation; while polydisperse aerosol can be used, for instance, to estimate response stability and linearity to varying concentration levels (Hapidin et al., 2019; Papapostolou et al., 2017; Sayahi et al., 2019a), the presence of multiple different-sized particles prevents the distinction between sensor response and specific particle size. The most significant deficiency of the VOAG (and the main limitation of this study) is that its smallest producible particle size is in practice limited by the impurity within the carrier liquid to approximately 0.55 µm.
The novelty of the aerosol generation method used in this research is based on the observation that the particle size of the monodisperse and constant number concentration reference aerosol can be controlled by feeding solutions with different non-volatile concentrations to the VOAG, one after the other. Such an aerosol generation technique was first utilized by Kuula et al. (2017), who accomplished the solution blending with a supplementary syringe pump and a manually operated three-way valve. In this study, however, the solution feeding was done with a gradient elution pump typically used in ion chromatography (GP50, Dionex Inc., USA). The GP50 gradient pump has four different eluent channels and is capable of dispensing liquids with high pressure (max. 5000 psi) and accurate volume flow rate (0.04–10.0 mL min−1 in increments of 0.01 mL min−1). The four eluent channels can be mixed with a resolution of 0.1 % (combined output of the four channels is always 100 %); furthermore, the GP50 has a user interface that enables the operator to generate parameterized eluent-dispensing programs. In essence, the utilization of the GP50 allows the user to freely choose and produce monodisperse aerosols of desired particle sizes without the tuning of VOAG running parameters or manual alternation of the liquid concentrations. Additionally, the preconfigured dispensing programs are fully automated, making the comparison of consecutive test runs more reliable.
2.2.2 Sampling configuration
A schematic figure of the used test setup is shown in Fig. 1. Reference aerosol was generated using the VOAG–GP50 system as described in the previous section. Dioctyl sebacate (DOS, density of 0.914 g cm−3) was used as a non-volatile solute in a 2-propanol solvent (>99.999 %, Sigma-Aldrich), and the formed particles were transparent oil droplets. Although the reference instrument APS is known for having decreased counting efficiency for liquid droplets over ∼5 µm in size (Volckens and Peters, 2005), no additional corrections were used. Running parameters of the VOAG and GP50 are shown in Table S1. The three different DOS concentrations (A–C) refer to the four different eluent channels of the GP50 (the use of three channels was sufficient for this study).
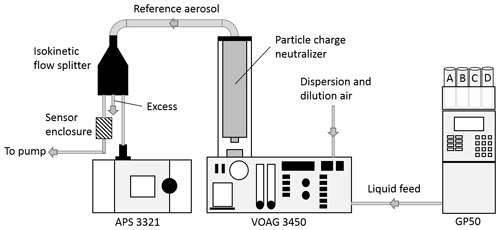
Figure 1Schematic of the sensor evaluation setup. The Grimm 1.108 spectrometer drew its sample from where the sensor enclosure is now shown.
The GP50 used an automated program for dispensing the liquids. A program involves a number of consecutive time steps in which the blending ratios of eluent channels, step durations, and volumetric flow rate of the liquid can be defined separately. Executing the program means that the GP50 dispenses the liquids according to the settings determined in each step. The program used in this evaluation consisted of 10 steps in which the produced particle sizes were logarithmically spaced from 0.45 to 9.78 µm. The calculated blending ratios and the respective particle sizes are shown in Supplemental Table S2. Step duration of 5 min was used; a single test run thus lasted approximately 60 min. Dead volumes in the GP50 and VOAG slightly extend the theoretical run time duration. A complete test run can be performed as quickly as in 15 min, which results in fewer measurement points and weaker statistical power though. An example of the produced reference aerosol number size distribution measured with the APS is shown in Fig. 2. It is worth underlining that the number of steps used in the GP50 dispensing program does not dictate the number of different particle sizes produced. The number of steps and the parameters assigned to them simply define the minimum (blending ratio of the first step) and maximum (blending ratio of the last step) particle size and the rate (step duration) at which the particle-size gradient evolves from the minimum size to maximum size. The word “gradient” is used to note that a step from 2 to 3 µm, for instance, does not lead to a discontinuous and sudden step from one particle size to another.
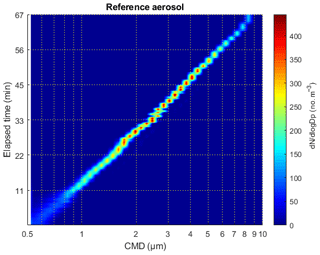
Figure 2An example of the produced reference aerosol. Decreasing number concentrations below 1 and above 5 µm result from approaching the lower detection limit (0.5 µm) of the APS and increasing inertial deposition losses in the sampling lines, respectively (concentration range 30–90 cm−3 of particle number concentration). This had, however, no effect on the evaluation results as the sensor response was normalized against the concentration measured by the APS. Along with the lower detection limit of the APS, another limiting factor of the study was the smallest producible particle size, which was approximately 0.55 µm. The GSD of the size distribution remained below 1.2.
Formed particles were neutralized in the dispersion outlet of the VOAG and further fed into a flow splitting section where the reference aerosol was symmetrically directed to both the reference instrument (aerodynamic particle sizer 3321, TSI Inc., USA) and sensor. The sensors were encapsulated in 3D-printed airtight enclosures with an external pump connected to them in order to ensure appropriate sample flow through the sensor. The sample flow rate was set to be 1 L min−1 – the aerosol flow rate of the APS (sheath flow of the APS taken from the laboratory air). Although there is no clear theoretical basis as to why a different flow rate would affect the way the sensor discriminates different particle sizes (apart from different particle-size-specific sampling losses), additional tests were conducted with flow rates of 0.5 and 2 L min−1 to ensure that this was indeed the case (see Fig. S2). For the PMS5003 and SPS30 sensors, an exhaust deflector was used to prevent unwanted sample mixing resulting from the fan outlet, which for these sensors, was situated right next to the sensor inlet. An illustration of the PMS5003 sampling arrangement is shown in Fig. 3. A schematic figure of all the sampling arrangements is shown in Fig. S3.
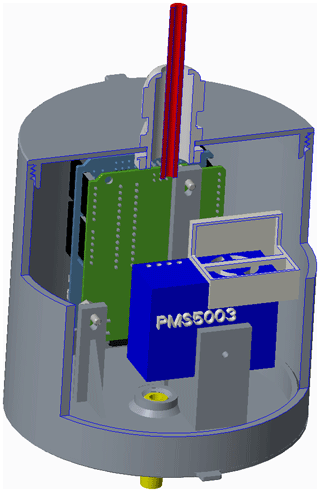
Figure 3A cross-section view of the sampling arrangement of the PMS5003 sensor. The flow deflector is shown in light grey, the inlet pipe in red, and the exhaust connector of the housing in yellow.
All sensor units were in the original condition except for the PPD42 and B5W sensors which had their air heating resistors removed. The evaluation platform used in this study did not require independent means of sample flow. Furthermore, holes were drilled into the plastic body of the PPD42 to ensure that the sample aerosol could reach to the optical detection volume. The inlet of the PPD42 was originally designed to be on top of the plastic body (facing towards the electronic circuit board); therefore, when the electronic circuit board of the sensor was oriented in parallel with the sample stream, the majority of the particles would have bypassed the sensor. In general, along with the PPD42, the plastic body layouts of the PMS5003 and SPS30 are susceptible to inertial deposition losses due to their 90∘ elbows in particle stream pathways. However, the more stable sample flow system (i.e., fan instead of convection) might help compensate for the sub-optimal layouts of these sensors.
2.3 Data processing
The output signal of the evaluated sensor and APS was measured synchronously using a 10 s time resolution and moving average. Any raw measurement point which had GSD (calculated from the APS data) exceeding 1.2 was disregarded (∼2.1 % of the data), but typically the GSD values ranged between 1.04 and 1.08. The sensor bias was set to zero by sampling clean air for 10 min (60 data points) and then subtracting the clean air response from the test aerosol response. The bias correction was only relevant for the GP2Y1010AU0F and B5W sensors. In order to prevent arbitrary unit comparisons, the sensor response was normalized using Eq. (3):
where i is the ith measurement point, “sensor” is the sensor signal, and APS is the APS total mass concentration. The maximum sensor ∕ APS ratio refers to the maximum ratio measured during a single test run.
The normalized 10 s resolution data were divided into 30 logarithmically spaced size bins (from 0.45 to 9.73 µm) according to the count median diameters (CMDs, aerodynamic) measured by the APS. An average sensor response as a function of average CMD was then calculated for each size bin. The decision to divide the data into 30 bins was based on the clarity of the produced figure and statistically sufficient number of measurement points belonging to each bin. This process was completed for three different sensor units, and a combined (average and standard deviation) sensor response was calculated. Valid detection ranges, which were defined as the upper half of the detection efficiency curve, of the sensors were linearly interpolated from the average response functions. A detailed example of how the data were processed and how the valid detection ranges were calculated is shown in the Supplement. The cursory evaluation of the Grimm instrument was conducted using the same data processing method. The size bins of PMS5003, SPS30, SDS011, and B5W were discretized so that no overlapping signals were obtained. For example, the outputs of the SDS011 were used as PM2.5 and PM10−2.5 (PM10−2.5 calculated as PM10–PM2.5) instead of PM2.5 and PM10.
The PMS5003, SDS011, and SPS30 sensors have digital outputs whereas the others are analog-based. Along with the PM mass fractions listed in Table 1, the PMS5003 and SPS30 sensors also output particle number concentrations, but these signals were not used because the response comparison to the reference instrument was carried out using only mass concentration values. This decision was based on the observation that low-cost sensors have been predominantly used to measure mass concentration and not number concentration.
3.1 Grimm model 1.108
The normalized detection efficiencies of the 15-bin Grimm 1.108 spectrometer are shown in Fig. 3. The normalized detection efficiency of 70 %–90 % results from the average efficiency from multiple data points and, in this case, does not imply that the Grimm spectrometer would systematically underestimate particle mass concentrations. The same applies to the respective sensor response figures (next section).
The response characteristics of the Grimm spectrometer are in line with its technical specifications showing that each size bin only corresponds to its specific detection range. A flat response curve would indicate that the strength of the output signal remains unchanged regardless of the particle size, which would show that the size bin is unable to make a distinction between different particle sizes. Some mismatch between the particle sizing of the APS and the Grimm spectrometer can be observed as a result of different particle sizing techniques (time of flight and optical), but this is trivial, considering the objective of this study. The purpose of this figure is to highlight how an aerosol measurement device with several particle sizing bins should respond to the evaluation method used in this study.
3.2 Low-cost sensors
Response functions of the evaluated sensors are shown in Fig. 4a–f.
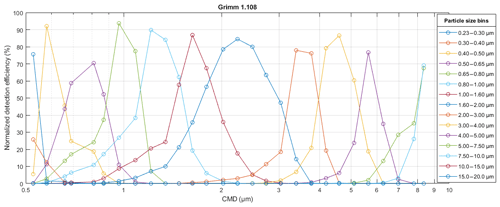
Figure 4Normalized detection efficiency of the 15 particle-size bins as a function of the count median diameter of the reference aerosol. Consecutively increasing and decreasing response curves indicate that the particle sizing of the instrument is functioning correctly. For the sake of clarity, degrees of measurement variation have been excluded from the figure. Bins 14 and 15, which correspond to 10–15 and 15–20 µm, respectively, are not shown as they did not produce any response (as expected).
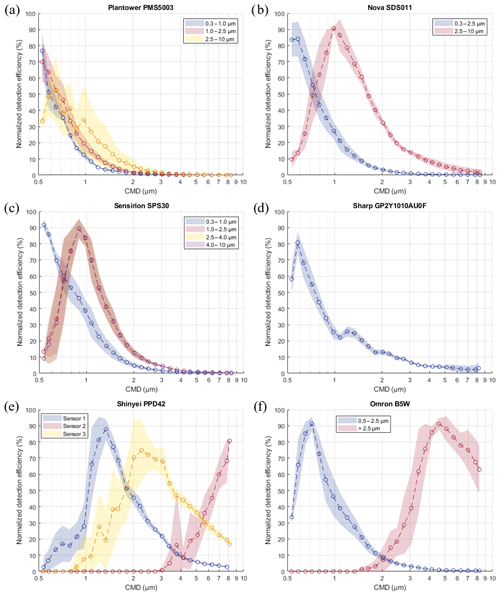
Figure 5Normalized detection efficiency of discretized PM mass fractions reported by the low-cost sensors as a function of the count median diameter of the reference aerosol. The colored circles represent the calculated average responses of the three sensor units, and the shaded background areas represent the respective standard deviations. Standard deviations of the average CMDs were negligible due to the reliable and reproducible test method. Figure legends correspond to the bin size ranges stated by the corresponding manufacturer.
Plantower PMS5003
According to Fig. 4a, it is apparent that the PMS5003 does not accurately distinguish between PM1, PM2.5, and PM10 size fractions. The first and the second bin (supposedly corresponding to 0.3–1.0 and 1.0–2.5 µm) are similar, with valid detection ranges of approximately <0.7 and <0.8 µm, respectively (valid detection ranges were defined as the upper half of the detection range; see the section “Data processing”). The lower cut points of these bins may reach close to 0.3 µm, as stated by the manufacturer; however, this could not be confirmed using the VOAG–GP50 system. As the larger standard deviations indicate, the third bin is noisier and significantly off of its stated detection range (2.5–10 µm).
Based on the test, the PMS5003 cannot be used to measure coarse-mode particles (2.5–10 µm); furthermore, its ability to measure PM2.5 depends on the stability of the ambient air size distribution: for example, if the proportions of mass in <0.8 and >0.8 µm fractions change significantly, the PMS5003 is susceptible to inaccuracies because its valid detection range cannot account for changes occurring in parts of the size distribution that it can hardly observe. However, if the ambient size distribution is stable, the PMS5003 can be adjusted to measure PM2.5 with reasonable accuracy (Bulot et al., 2019; Feenstra et al., 2019; Magi et al., 2019; Malings et al., 2019). Similarly, the validity of PM10 measurements can only be ensured when the proportion of mass in >0.7 or >0.8 µm size fractions is either constant or negligible with respect to the total PM10 mass. In reality, this is rarely the case, which poses a high risk of sensor misuse. This observation is in line with the findings from previous studies (Laquai, 2017b; Li et al., 2019; Sayahi et al., 2019b) which show, for instance, that the PMS5003 could not detect a substantial dust storm episode while deployed in the field. The most accurate and reliable results are most likely achieved for the PM1 size fraction by using either bin 1 or bin 2 signals.
Nova SDS011
The response function of the SDS011 is shown in Fig. 4b. Contrary to the PMS5003, the SDS011 exhibits two clearly different detection ranges: the first bin (0.3–2.5 µm) corresponds approximately to <0.8 µm, and the second bin (2.5–10 µm) corresponds approximately to 0.7–1.7 µm. Similarly to the PMS5003, the SDS011 is not suitable for the measurement of coarse-mode particles, and the measurements of PM10 can be grossly inaccurate, as also noted by Budde et al. (2018) and Laquai (2017a). However, due to the clearer difference between bin 1 and bin 2 detection ranges, the SDS011 has the potential to measure PM2.5 more accurately than the PMS5003. For example, by calculating the ratio of bins 1 and 2, it is possible to approximate the distribution of mass in the 0.3–2.5 µm size range, thus using an additional correction factor to obtain more accurate results. Previous studies have shown that the SDS011 can be reasonably accurate in the measurements of PM2.5 (Badura et al., 2018; Liu et al., 2019).
Sensirion SPS30
The response function of the SPS30 is shown in Fig. 4c. The valid detection range of the first bin (0.3–1.0 µm) is approximately <0.9 µm. The second, third, and fourth bins (supposedly corresponding to 1.0–2.5, 2.5–4.0, and 4.0–10 µm) are nearly identical, with valid detection ranges of approximately 0.7–1.3 µm. The identical detection ranges indicate that these bins may have been factory calibrated using the same test aerosol. The SPS30 is a relatively new sensor (introduced to the markets in late 2018), and neither Web of Science nor Scopus showed any existing studies as of September 2019. However, the South Coast Air Quality Management District (SCAQMD, USA) has conducted a preliminary field test where three SPS30 units were compared to three different federal equivalent method (FEM) monitors (SCAQMD, 2019). The results of this test showed that the SPS30 sensors had very low cross-unit variability (∼1 %, 1.3 %, and 2.4 % for PM1, PM2.5, and PM10, respectively), and, more importantly, the coefficient of determinations for the measurement of PM1, PM2.5, and PM10 decreased from R2∼0.91 to 0.83 and further down to 0.12, respectively. These observations strongly align with the results of this study; furthermore, they illustrate how a sensor with limited operational range may exhibit a near-regulatory-grade performance if the measured size fraction is in alignment with the valid detection range of the sensor (<0.9 µm and PM1). On the other hand, the severity of data misinterpretation is apparent when the sensor measurement is extended to cover particle sizes that it cannot observe.
Sharp GP2Y1010AU0F
The response function of the GP2Y1010AU0F is shown in Fig. 4d, and its valid detection range appears to be approximately <0.8 µm. Like the previously discussed sensors, the GP2Y1010AU0F can be used to measure small particles (e.g., PM1) but not coarse-mode particles. Several laboratory evaluations have been previously conducted for the GP2Y1010AU0F, but none of these have assessed its detection range using monodisperse test aerosols (Li and Biswas, 2017; Manikonda et al., 2016; Sousan et al., 2016). Wang et al. (2015) used atomized polystyrene latex (PSL) particles to evaluate the effect of particle size on the GP2Y1010AU0F response, but no concluding remarks can be obtained from these results. The study method utilized only three different sized PSLs; moreover, it was not designed to investigate the complete detection range of the GP2Y1010AU0F. However, according to the authors, the results implied that the sensor was more sensitive to 300 nm particles than to 600 and 900 nm particles, which is in slight disagreement with the results of this study whereby the normalized detection efficiency curve shows the highest sensitivity peak for 0.6 µm sized particles as well as a decreasing trend for particles smaller than this. There is no obvious explanation for this discrepancy, but it is worth re-emphasizing the differences in the used evaluation approaches.
Shinyei PPD42
Response functions of the three PPD42 sensor units are shown in Fig. 4e. Contrary to the other sensors, a combined response function was not calculated as the three units exhibited significantly different response characteristics. The circles and shaded background areas represent average responses and respective standard deviations of the individual sensor units (calculated from the ∼300 raw data points). The valid detection range of the first unit is 1.0–2.1 µm, and it is likely to be best suited for PM2.5 measurements. However, the low detection efficiency of <1.0 µm sized particles may considerably hinder its accuracy. Valid detection ranges of the second and third units are >5.9 and 1.5–4.9 µm, indicating preferable applicability to coarse-mode particle measurements. Previous laboratory evaluations have noted that the PPD42 output is a function of particle size but could not provide a more detailed analysis of the complete detection range (Austin et al., 2015; Wang et al., 2015). A study of Kuula et al. (2017) reported a valid detection range of approximately 2.5–4.0 µm, which is in the same range as the third unit of this study.
Due to the apparent inter-unit inconsistency in valid detection ranges, it is evident that the response characteristics of the PPD42 have to be quantified case by case before reliable measurements can be achieved. Accordingly, the inconsistent response characteristics may also contribute to the fact that previous field evaluation studies have achieved varying results regarding the performance of PPD42; Bai et al. (2019) and Holstius et al. (2014) reported R2 values of 0.75 and 0.55–0.60, respectively, for the measurement of PM2.5, whereas N. E. Johnson et al. (2018) and K. K. Johnson et al. (2018) reported more modest values of 0.36–0.51 and 0–0.28, respectively (Bai et al., 2019; Holstius et al., 2014; N. E. Johnson et al., 2018, K. K. Johnson et al., 2018). On the other hand, Kuula et al. (2017, 2018) showed that higher levels of accuracy can be achieved if the measured size fraction is targeted to correspond to the characteristic response function of the PPD42 (R2=0.96 and R2=0.87, respectively).
Omron B5W
The response function of the B5W is shown in Fig. 4f. The two size bins exhibit two different detection ranges (0.6–1.0 and >3.2 µm) that are reasonably close to the ones declared by the manufacturer (0.5–2.5 and >2.5 µm). In fact, out of all sensors, the B5W appears to be the most promising sensor for the ambient monitoring of PM2.5 and PM10–2.5 size fractions. In comparison to SDS011 and SPS30, for instance, the usability of the B5W may be hindered by its temperature-gradient-based sampling method, which is not as reliable as the respective fan-based method. Nonetheless, it is the only sensor capable of measuring both fine- and coarse-fraction particles. Neither Web of Science nor Scopus showed existing studies for the Omron B5W.
According to the results obtained in this study, low-cost optical sensors exhibit widely varying response characteristics regarding their size selectivity (from <0.7 to >5.9 µm, Table 2). However, none of the sensors have precisely the same response characteristics stated by their manufacturers, which provides evidence of the fact that particle-size selectivity may play an essential role in the analysis of the sources of errors in sensors and underlines that scientists, as well as manufacturers, need to acknowledge the limitations related to this: attempts to artificially extend the operational range of sensors beyond their practical capabilities using complex statistical models may be unreasonable and lead to misleading conclusions. Empirical corrections for known artifacts, such as humidity, can be justifiable; however, sensor data and advanced modeling techniques should be merged cautiously in order to retain both the validity and representativeness of the data.
Table 2Valid detection ranges of the evaluated sensors. Symbols of “greater than” or “smaller than” refer to cases where the other end of the size cut point was outside of the particle-size range producible by the VOAG–GP50 system (0.45–9.73 µm). Units are in micrometers.
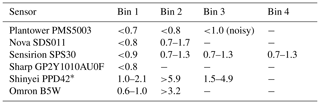
* Valid detection ranges of the individual sensors, not bins.
A cursory comparison to a mid-cost aerosol size spectrometer (Grimm 1.108) shows that low-cost sensor development is still considerably behind its more expensive alternative: while the Grimm 1.108 spectrometer could sufficiently characterize particle sizes with up to 15 different size bins, the low-cost sensors could only achieve independent responses for one or two bins, which is a significant weakness, considering that the ability to measure particle size correctly is at the foundation of accurate mass measurement (mass α dp3). The development of low-cost sensors should focus on increasing the number of size bins, and more importantly, making sure that each size bin is calibrated correctly. Improperly configured bin sizing poses a significant risk of data misinterpretation and will inevitably lead to inaccurate measurements. A low number of size bins limits the valid operational range of sensors; however, it is unclear how the number of advanced measurement features and low unit cost should be reconciled.
The VOAG–GP50 aerosol generation system described in this study introduced a novel approach to the quick and efficient evaluation of aerosol measurement devices. The use of a GP50 gradient pump eliminates much of the manual labor that previously was an inseparable part of the VOAG operation, thus making the generation of reference aerosols more consistent and reliable. Its automated dispensing programs allow for highly repeatable testing; furthermore, the four different eluent channels enable the operator to pick and choose the desired particle size to be produced freely. Along with saving manual labor and time, this is also a cost-saving feature as traditionally used polystyrene latex (PSL) particles are not needed. Considering these matters, the VOAG–GP50 system can potentially be scaled to an industrial-level operation, which is an intriguing feature when considering the mass deployment of sensors and their respective quality assurance and control.
The data can be openly accessed upon request.
The supplement related to this article is available online at: https://doi.org/10.5194/amt-13-2413-2020-supplement.
JK and TM designed the experimental setup, and JK carried out the tests. KT had an important role in refurbishing the gradient elution pump. SM and OG provided some of the sensors. JK was responsible for the data analysis, although all co-authors provided valuable feedback, particularly TM. JK wrote the manuscript with the help of all co-authors.
The authors declare that they have no conflict of interest.
This research has been supported by the European Regional Development Fund (Urban innovative actions initiative project HOPE; UIA03-240 grant) and Horizon 2020 (iSCAPE grant no. (689954)).
This paper was edited by Murray Hamilton and reviewed by two anonymous referees.
Austin, E., Novosselov, I., Seto, E., and Yost, M. G.: Laboratory evaluation of the Shinyei PPD42NS low-cost particulate matter sensor, PLoS One, 10, 1–17, https://doi.org/10.1371/journal.pone.0137789, 2015.
Badura, M., Batog, P., Drzeniecka-Osiadacz, A., and Modzel, P.: Evaluation of low-cost sensors for ambient PM2.5 monitoring, J. Sensors, 2018, 5096540, https://doi.org/10.1155/2018/5096540, 2018.
Bai, L., Huang, L., Wang, Z., Ying, Q., Zheng, J., Shi, X., and Hu, J.: Long-term field Evaluation of Low-cost Particulate Matter Sensors in Nanjing, Aerosol Air Qual. Res., 20, 1–12, https://doi.org/10.4209/aaqr.2018.11.0424, 2019.
Berglund, R. N. and Liu, B. Y. H.: Generation of Monodisperse Aerosol Standards, Environ. Sci. Technol., 7, 147–153, https://doi.org/10.1021/es60074a001, 1973.
Budde, M., D. Schwarz, A., Müller, T., Laquai, B., Streibl, N., Schindler, G., Köpke, M., Riedel, T., Dittler, A., and Beigl, M.: Potential and Limitations of the Low-Cost SDS011 Particle Sensor for Monitoring Urban Air Quality, 3rd International Conference on Atmospheric Dust (DUST2018), ProScience, 5, 6–12, https://doi.org/10.14644/dust.2018.002, 2018.
Bulot, F. M. J. J., Johnston, S. J., Basford, P. J., Easton, N. H. C. C., Apetroaie-Cristea, M., Foster, G. L., Morris, A. K. R. R., Cox, S. J., and Loxham, M.: Long-term field comparison of multiple low-cost particulate matter sensors in an outdoor urban environment, Sci. Rep., 9, 7497, https://doi.org/10.1038/s41598-019-43716-3, 2019.
Burkart, J., Steiner, G., Reischl, G., Moshammer, H., Neuberger, M., and Hitzenberger, R.: Characterizing the performance of two optical particle counters (Grimm OPC1.108 and OPC1.109) under urban aerosol conditions, J. Aerosol Sci., 41, 953–962, https://doi.org/10.1016/j.jaerosci.2010.07.007, 2010.
Caubel, J. J., Cados, T. E., Preble, C. V., and Kirchstetter, T. W.: A Distributed Network of 100 Black Carbon Sensors for 100 Days of Air Quality Monitoring in West Oakland, California, Environ. Sci. Technol., 53, 7564–7573, https://doi.org/10.1021/acs.est.9b00282, 2019.
Feenstra, B., Papapostolou, V., Hasheminassab, S., Zhang, H., Boghossian, B. Der, Cocker, D., and Polidori, A.: Performance evaluation of twelve low-cost PM2.5 sensors at an ambient air monitoring site, Atmos. Environ., 216, 116946, https://doi.org/10.1016/j.atmosenv.2019.116946, 2019.
Feinberg, S. N., Williams, R., Hagler, G., Low, J., Smith, L., Brown, R., Garver, D., Davis, M., Morton, M., Schaefer, J., and Campbell, J.: Examining spatiotemporal variability of urban particulate matter and application of high-time resolution data from a network of low-cost air pollution sensors, Atmos. Environ., 213, 579–584, https://doi.org/10.1016/j.atmosenv.2019.06.026, 2019.
Gao, M., Cao, J., and Seto, E.: A distributed network of low-cost continuous reading sensors to measure spatiotemporal variations of PM2.5 in Xi'an, China, Environ. Pollut., 199, 56–65, https://doi.org/10.1016/j.envpol.2015.01.013, 2015.
Grimm 1.108: Aerosol spectrometer user manual, available at: https://wmo-gaw-wcc-aerosol-physics.org/files/opc-grimm-model--1.108-and-1.109.pdf (last access: 11 February 2020), GRIMM Aerosol Technik GmbH, Germany, 2020.
Hapidin, D. A., Saputra, C., Maulana, D. S., Munir, M. M., and Khairurrijal, K.: Aerosol chamber characterization for commercial particulate matter (PM) sensor evaluation, Aerosol Air Qual. Res., 19, 181–194, https://doi.org/10.4209/aaqr.2017.12.0611, 2019.
Holstius, D. M., Pillarisetti, A., Smith, K. R., and Seto, E.: Field calibrations of a low-cost aerosol sensor at a regulatory monitoring site in California, Atmos. Meas. Tech., 7, 1121–1131, https://doi.org/10.5194/amt-7-1121-2014, 2014.
Jayaratne, R., Liu, X., Thai, P., Dunbabin, M., and Morawska, L.: The influence of humidity on the performance of a low-cost air particle mass sensor and the effect of atmospheric fog, Atmos. Meas. Tech., 11, 4883–4890, https://doi.org/10.5194/amt-11-4883-2018, 2018.
Jiao, W., Hagler, G., Williams, R., Sharpe, R., Brown, R., Garver, D., Judge, R., Caudill, M., Rickard, J., Davis, M., Weinstock, L., Zimmer-Dauphinee, S., and Buckley, K.: Community Air Sensor Network (CAIRSENSE) project: evaluation of low-cost sensor performance in a suburban environment in the southeastern United States, Atmos. Meas. Tech., 9, 5281–5292, https://doi.org/10.5194/amt-9-5281-2016, 2016.
Johnson, K. K., Bergin, M. H., Russell, A. G., and Hagler, G. S. W.: Field test of several low-cost particulate matter sensors in high and low concentration urban environments, Aerosol Air Qual. Res., 18, 565–578, https://doi.org/10.4209/aaqr.2017.10.0418, 2018.
Johnson, N. E., Bonczak, B., and Kontokosta, C. E.: Using a gradient boosting model to improve the performance of low-cost aerosol monitors in a dense, heterogeneous urban environment, Atmos. Environ., 184, 9–16, https://doi.org/10.1016/j.atmosenv.2018.04.019, 2018.
Karagulian, F., Barbiere, M., Kotsev, A., Spinelle, L., Gerboles, M., Lagler, F., Redon, N., Crunaire, S., and Borowiak, A.: Review of the Performance of Low-Cost Sensors for Air Quality Monitoring, Atmosphere, 10, 506, https://doi.org/10.3390/atmos10090506, 2019.
Kumar, P., Morawska, L., Martani, C., Biskos, G., Neophytou, M., Di Sabatino, S., Bell, M., Norford, L., and Britter, R.: The rise of low-cost sensing for managing air pollution in cities, Environ. Int., 75, 199–205, https://doi.org/10.1016/j.envint.2014.11.019, 2015.
Kuula, J., Mäkelä, T., Hillamo, R., and Timonen, H.: Response characterization of an inexpensive aerosol sensor, Sensors, 17, 2915, https://doi.org/10.3390/s17122915, 2017.
Kuula, J., Kuuluvainen, H., Rönkkö, T., Niemi, J. V., Saukko, E., Portin, H., Aurela, M., Saarikoski, S., Rostedt, A., Hillamo, R., and Timonen, H.: Applicability of Optical and Diffusion Charging-Based Particulate Matter Sensors to Urban Air Quality Measurements, Aerosol Air Qual. Res., 122, 1–16, https://doi.org/10.4209/aaqr.2018.04.0143, 2018.
Laquai, B.: Impact of Particle Mass Distribution on the Measurement Accuracy of Low-Cost PM-Sensors, October, available at: https://www.researchgate.net/publication/320537200_Impact_of_Particle_Mass_Distribution_on_the_Measurement_Accuracy_of_Low-Cost_PM-Sensors (last access: 1 April 2020), 2017a.
Laquai, B.: Particle Distribution Dependent Inaccuracy of the Plantower PMS5003 low-cost PM-sensor Particle Distribution Dependent Inaccuracy of the Plantower PMS5003 low-cost PM-sensor, October, available at: https://www.researchgate.net/publication/320555036_Particle Distribution_Dependent_Inaccuracy_of_the_Plantower_PMS5003_low-cost_PM-sensor (last access: 10 September 2019), 2017b.
Levy Zamora, M., Xiong, F., Gentner, D., Kerkez, B., Kohrman-Glaser, J., and Koehler, K.: Field and Laboratory Evaluations of the Low-Cost Plantower Particulate Matter Sensor, Environ. Sci. Technol., 53, 838–849, https://doi.org/10.1021/acs.est.8b05174, 2019.
Li, J. and Biswas, P.: Optical characterization studies of a low-cost particle sensor, Aerosol Air Qual. Res., 17, 1691–1704, https://doi.org/10.4209/aaqr.2017.02.0085, 2017.
Li, J., Mattewal, S. K., Patel, S., and Biswas, P.: Evaluation of Nine Low-cost-sensor-based Particulate Matter Monitors, Aerosol Air Qual. Res., 20, 1–17, https://doi.org/10.4209/aaqr.2018.12.0485, 2019.
Liu, H. Y., Schneider, P., Haugen, R., and Vogt, M.: Performance assessment of a low-cost PM2.5 sensor for a near four-month period in Oslo, Norway, Atmosphere, 10, 41, https://doi.org/10.3390/atmos10020041, 2019.
Magi, B. I., Cupini, C., Francis, J., Green, M., and Hauser, C.: Evaluation of PM2.5 measured in an urban setting using a low-cost optical particle counter and a Federal Equivalent Method Beta Attenuation Monitor, Aerosol Sci. Technol., 0, 1–13, https://doi.org/10.1080/02786826.2019.1619915, 2019.
Malings, C., Tanzer, R., Hauryliuk, A., Saha, P. K., Robinson, A. L., Presto, A. A., and Subramanian, R.: Fine particle mass monitoring with low-cost sensors: Corrections and long-term performance evaluation, Aerosol Sci. Technol., 0, 1–15, https://doi.org/10.1080/02786826.2019.1623863, 2019.
Manikonda, A., Zikova, N., Hopke, P. K., and Ferro, A. R.: Laboratory assessment of low-cost PM monitors, J. Aerosol Sci., 102, 29–40, 2016.
Morawska, L., Thai, P. K., Liu, X., Asumadu-Sakyi, A., Ayoko, G., Bartonova, A., Bedini, A., Chai, F., Christensen, B., Dunbabin, M., Gao, J., Hagler, G., Jayaratne, R., Kumar, P., Lau, A. K. H., Louie, P. K. K., Mazaheri, M., Ning, Z., Motta, N., Mullins, B., Rahman, M. M., Ristovski, Z., Shafiei, M., Tjondronegoro, D., Westerdahl, D., and Williams, R.: Applications of low-cost sensing technologies for air quality monitoring and exposure assessment: How far have they gone?, Environ. Int., 116, 286–299, https://doi.org/10.1016/j.envint.2018.04.018, 2018.
Papapostolou, V., Zhang, H., Feenstra, B. J., and Polidori, A.: Development of an environmental chamber for evaluating the performance of low-cost air quality sensors under controlled conditions, Atmos. Environ., 171, 82–90, https://doi.org/10.1016/j.atmosenv.2017.10.003, 2017.
Peters, T. M., Ott, D., and O'Shaughnessy, P. T.: Comparison of the Grimm 1.108 and 1.109 portable aerosol spectrometer to the TSI 3321 aerodynamic particle sizer for dry particles, Ann. Occup. Hyg., 50, 843–850, https://doi.org/10.1093/annhyg/mel067, 2006.
Popoola, O. A. M., Carruthers, D., Lad, C., Bright, V. B., Mead, M. I., Stettler, M. E. J., Saffell, J. R., and Jones, R. L.: Use of networks of low cost air quality sensors to quantify air quality in urban settings, Atmos. Environ., 194, 58–70, https://doi.org/10.1016/j.atmosenv.2018.09.030, 2018.
Rai, A. C., Kumar, P., Pilla, F., Skouloudis, A. N., Di Sabatino, S., Ratti, C., Yasar, A., and Rickerby, D.: End-user perspective of low-cost sensors for outdoor air pollution monitoring, Sci. Total Environ., 607–608, 691–705, https://doi.org/10.1016/j.scitotenv.2017.06.266, 2017.
Sayahi, T., Kaufman, D., Becnel, T., Kaur, K., Butterfield, A., Collingwoodd, S., Zhang, Y., Gaillardon, P.-E., and Kelly, K. E.: Development of a calibration chamber to evaluate the performance of low-cost particulate matter sensors, Environ. Pollut., 255, 113131, https://doi.org/10.1016/j.envpol.2019.113131, 2019a.
Sayahi, T., Butterfield, A., and Kelly, K. E.: Long-term field evaluation of the Plantower PMS low-cost particulate matter sensors, Environ. Pollut., 245, 932–940, https://doi.org/10.1016/j.envpol.2018.11.065, 2019b.
Schneider, P., Bartonova, A., Castell, N., Dauge, F. R., Gerboles, M., Hagler, G. S. W., Hüglin, C., Jones, R. L., Khan, S., Lewis, A. C., Mijling, B., Müller, M., Penza, M., Spinelle, L., Stacey, B., Vogt, M., Wesseling, J., and Williams, R. W.: Toward a Unified Terminology of Processing Levels for Low-Cost Air-Quality Sensors, Environ. Sci. Technol., 53, 8485–8487, https://doi.org/10.1021/acs.est.9b03950, 2019.
Snyder, E. G., Watkins, T. H., Solomon, P. A., Thoma, E. D., Williams, R. W., Hagler, G., Shelow, D., Hindin, D. A., Kilaru, V. J., and Preuss, P. W.: The changing paradigm of air pollution monitoring, Environ. Sci. Technol., 47, 11369–11377, https://doi.org/10.1021/es4022602, 2013.
Sousan, S., Koehler, K., Thomas, G., Park, J. H., Hillman, M., Halterman, A., and Peters, T. M.: Inter-comparison of low-cost sensors for measuring the mass concentration of occupational aerosols, Aerosol Sci. Technol., 50, 462–473, https://doi.org/10.1080/02786826.2016.1162901, 2016.
South Coast Air Quality Management District (SCAQMD): Field Evaluation Sensirion SPS30 Evaluation Kit Background, available at: http://www.aqmd.gov/docs/default-source/aq-spec/field-evaluations/sensirion-sps30-evaluation-kit---field-evaluation.pdf, last access: 5 November 2019.
Volckens, J. and Peters, T. M.: Counting and particle transmission efficiency of the aerodynamic particle sizer, J. Aerosol Sci., 36, 1400–1408, https://doi.org/10.1016/j.jaerosci.2005.03.009, 2005.
Wang, Y., Li, J., Jing, H., Zhang, Q., Jiang, J., and Biswas, P.: Laboratory Evaluation and Calibration of Three Low-Cost Particle Sensors for Particulate Matter Measurement, Aerosol Sci. Technol., 49, 1063–1077, https://doi.org/10.1080/02786826.2015.1100710, 2015.
Yuval, Magen Molho, H., Zivan, O., Broday, D. M., and Raz, R.: Application of a sensor network of low cost optical particle counters for assessing the impact of quarry emissions on its vicinity, Atmos. Environ., 211, 29–37, https://doi.org/10.1016/j.atmosenv.2019.04.054, 2019.
Zheng, T., Bergin, M. H., Johnson, K. K., Tripathi, S. N., Shirodkar, S., Landis, M. S., Sutaria, R., and Carlson, D. E.: Field evaluation of low-cost particulate matter sensors in high- and low-concentration environments, Atmos. Meas. Tech., 11, 4823–4846, https://doi.org/10.5194/amt-11-4823-2018, 2018.