the Creative Commons Attribution 4.0 License.
the Creative Commons Attribution 4.0 License.
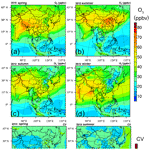
Model evaluation and intercomparison of surface-level ozone and relevant species in East Asia in the context of MICS-Asia Phase III – Part 1: Overview
Jie Li
Tatsuya Nagashima
Baozhu Ge
Kazuyo Yamaji
Joshua S. Fu
Xuemei Wang
Qi Fan
Syuichi Itahashi
Hyo-Jung Lee
Cheol-Hee Kim
Chuan-Yao Lin
Meigen Zhang
Zhining Tao
Mizuo Kajino
Hong Liao
Jung-Hun Woo
Jun-ichi Kurokawa
Qizhong Wu
Hajime Akimoto
Gregory R. Carmichael
Zifa Wang
Spatiotemporal variations of ozone (O3) and nitrogen oxide (NOx) mixing ratios from 14 state-of-the-art chemical transport models (CTMs) are intercompared and evaluated with O3 observations in East Asia, within the framework of the Model Inter-Comparison Study for Asia Phase III (MICS-Asia III). This study was designed to evaluate the capabilities and uncertainties of current CTMs simulations for Asia and to provide multi-model estimates of pollutant distributions. These models were run by 14 independent groups working in China, Japan, South Korea, the United States and other countries/regions. Compared with the previous phase of MICS-Asia (MICS-Asia II), the evaluation with observations was extended from 4 months to 1 full year across China and the western Pacific Rim. In general, model performance levels for O3 varied widely by region and season. Most models captured the key patterns of monthly and diurnal variation of surface O3 and its precursors in the North China Plain and western Pacific Rim but failed to do so for the Pearl River Delta. A significant overestimation of surface O3 was evident from May to September/October and from January to May over the North China Plain, the western Pacific Rim and the Pearl River Delta. Comparisons drawn from observations show that the considerable diversity in O3 photochemical production partly contributed to this overestimation and to high levels of inter-model variability in O3 for North China. In terms of O3 soundings, the ensemble average of models reproduced the vertical structure for the western Pacific, but overestimated O3 levels to below 800 hPa in the summer. In the industrialized Pearl River Delta, the ensemble average presented an overestimation for the lower troposphere and an underestimation for the middle troposphere. The ensemble average of 13 models for O3 did not always exhibit superior performance compared with certain individual models in contrast with its superior value for Europe. This finding suggests that the spread of ensemble-model values does not represent all of the uncertainties of O3 or that most MICS-Asia III models missed key processes. This study improved the performance of modeling O3 in March at Japanese sites compared with MICS-Asia II. However, it overpredicted surface O3 concentrations for western Japan in July, which was not found by MICS-Asia II. Major challenges still remain with regard to identifying the sources of bias in surface O3 over East Asia in CTMs.
- Article
(8196 KB) - Full-text XML
-
Supplement
(2605 KB) - BibTeX
- EndNote
Tropospheric ozone (O3) is a significant secondary air pollutant produced via thousands of photochemical reactions and, as a strong oxidant, it is detrimental to human health, ecosystems and climate change (WHO, 2005; The Royal Society, 2008). Due to rapid industrialization and urbanization over the last 2 decades, the O3 concentration is rising at a higher rate in East Asia than in other regions, and on 30 % of days in megacities (e.g., Beijing, Shanghai and Guangzhou in China) values exceed the air quality standard of the World Health Organization (100 µg m−3) for the 8 h average surface O3 concentration (Wang et al., 2017). Thus, high O3 concentrations have received more attention from the public and policy makers in East Asia. The Ministry of Environment of Japan has imposed stringent measures to reduce traffic emissions since the 1990s, and non-methane volatile organic compounds (NMVOCs) and NOx mixing ratios have decreased by 40 %–50 % and 51 %–54 %, respectively (Akimoto et al., 2015). In 2012, China released a new ambient air quality standard under which a limit on the 8 h O3 maximum was set for the first time. However, these measures have not prevented the persistent increase of ground-level O3 in East Asia. The averaged mixing ratio of O3 has increased by 20 %–30 % in Japan over the last 20 years (Akimoto et al., 2015). In Chinese megacities, 8 h O3 concentrations have increased by 10 %–30 % since 2013 (Wang et al., 2017).
The main method used for the detailed evaluation of the effects of air quality policies at the scale of East Asia is that of numerical air quality modeling. Several global and regional scale CTMs (e.g., GEOS-Chem, CHASER, CMAQ, CAMx, WRF-Chem and NAQPMS) have been developed over the past few decades and have been widely used to simulate the O3 formation process and to evaluate strategies for its control (Streets et al., 2007; Li et al., 2007, 2008; Yamaji et al., 2006; Zhang et al., 2008; Liu et al., 2010; Wang et al., 2013; He et al., 2017; Nagashima et al., 2010). Such simulations have identified the key precursors of O3 formation in East Asia (Zhang et al., 2008; Liu et al., 2010; Tang et al., 2010; He et al., 2017), have assessed the contributions of international and regional transport (Streets et al., 2008; Li et al., 2008) and have predicted O3 mixing ratios under different future emission scenarios (Wang et al., 2013). However, discrepancies remain between models and observations, indicating that model simulations of O3 in East Asia still need to be improved (Han et al., 2008). Modeling uncertainties related to emissions, chemistry, wet and dry deposition, and transport can hardly be addressed using a single model. Thus, model intercomparison has been recognized as an effective way to address problems and has been successfully applied in Europe and North America in phase 2 of the Air Quality Model Evaluation International Initiative (AQMEII; Rao et al., 2011). Limited model intercomparison related to air quality in East Asia has been conducted. Phases I and II of the Model Inter-Comparison Study for Asia (MICS-Asia) were initiated in 1998 and 2003, respectively, to explore the potential sources of model uncertainties regarding sulfur, O3, nitrogen compounds and aerosols (Carmichael et al., 2002, 2008). The study has shown that the predicted temporal variations of surface O3 in eight regional CTMs generally tended to be lower than those observed in 2001 with poor correlations in the western Pacific in March and December (Han et al., 2008). Model performance levels for O3 were found to vary greatly in southern China. Inconsistencies in the horizontal grids, emissions and meteorological inputs used among models have rendered explaining inter-model variability in MICS-Asia II results more difficult. More importantly, model evaluations for industrialized China have not been conducted due to a lack of observations, which has been detrimental to efforts made to improve O3 model performance levels.
Recently, regional CTMs have been greatly improved by coupling more mechanisms (e.g., heterogeneous chemistry and online calculation of photolysis rates) and accurate chemical reaction rates. For example, gas-phase chemistry mechanisms of Models-3 Community Multiscale Air Quality (CMAQ) have been evolved into CBM05 and SAPRC07 from CB04 and SAPRC99. It is critical to evaluate the updated models' abilities to simulate current air quality levels over East Asia. In 2010, MICS-Asia was expanded to Phase III; in this phase, 13 regional CTMs and 1 global CTM were run over 1 full year by 14 independent groups from East Asia and North America, using a common reference model input dataset (namely, the emission inventory, meteorological fields and horizontal grids). In addition to observations made in Japan by the Acid Deposition Monitoring Network in East Asia (EANET) that were used for MICS-Asia II, new observational data from China were made available for MICS-Asia III and were obtained from the Chinese Ecosystem Research Network (CERN) and the Pearl River Delta Regional Air Quality Monitoring Network (PRD RAQMN). An intercomparison of CTMs in China, Japan and the western Pacific for 1 full year had never before been performed, creating a broader database to use for comparisons. Therefore, the completeness of MICS-Asia III is unique.
In this paper, we mainly evaluate the capacities of models participating in MICS-Asia III to simulate concentrations of O3 and its related species within the MICS-Asia III framework. The following questions are addressed: (1) How well do various air quality models perform in simulating O3 levels in East Asia? (2) How consistent or discrepant are the models? (3) How do multi-model ensembles improve O3 simulation accuracy?
This paper is expected to provide valuable insights into the capacities and limitations of CTMs when applied to East Asia.
2.1 Experimental setup
In this study, all participating models were run for the year 2010 and provided gridded monthly mean diurnal mixing ratios of O3 and its precursors in the lowest model layer. For O3, monthly three-dimensional data were also submitted.
Table 1Basic structures, schemes and relevant parameters of the 14 participating models.
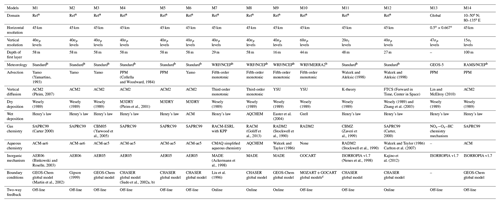
a Ref represents the domain referenced by the MICS-Asia III project.
b Standard represents the reference meteorological field provided by the
MICS-Asia III project; WRF/NCEP and WRF/MERRA represent the meteorological
field of the participating model itself, which was run by WRF and driven by the
NCEP
and Modern Era Retrospective-analysis for Research and Applications
(MERRA) reanalysis dataset.
c Boundary conditions of M10 are from MOZART and GOCART (Chin et al.,
2002; Horowitz et al., 2003), which provided results for gaseous pollutants
and aerosols, respectively.
2.2 Participating models and input data
Table 1 summarizes the specifications of participating CTMs. These models include two versions of CMAQ (v4.7.1 and 5.0.2; Byun and Schere, 2006), the Weather Research and Forecasting model coupled with Chemistry (WRF-Chem; http://www.acd.ucar.edu/wrf-chem, last access: 8 October 2019), the Nested Air Quality Prediction Modeling System (NAQPMS; Li et al., 2007), the Japan Meteorological Agency's (JMA) non-hydrostatic meteorology–chemistry model (NHM-Chem; Kajino et al., 2012), the NASA-Unified Weather Research and Forecasting model (NU-WRF; Tao et al., 2013) and GEOS-Chem (http://acmg.seas.harvard.edu/geos/, last access: 8 October 2019). They have been documented in the scientific literature and have been widely applied in modeling studies of East Asia. Table 1 does not list model names to maintain each model's anonymity. Similar behavior was observed to MICS-Asia II and other model intercomparison projects (e.g., AQME II).
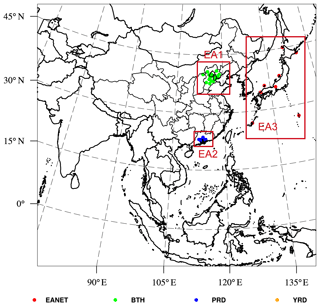
Figure 1Model domain of models (except M13 and M14) showing the locations of three subregions marked in this study. Also shown are the locations of surface monitoring stations used in this study. The meteorological model used to provide meteorological fields for most models also uses this domain. Note that the domains of M13 and M14 are shown in Fig. 10.
MICS-Asia III participants were provided with a reference meteorological field for the year 2010, generated with the Weather Research and Forecasting model (WRF; version 3.4.1). The domain of the meteorological fields is shown in Fig. 1. WRF v3.4.1 is driven by the final analysis dataset (ds083.2) from the National Centers for Environmental Prediction (NCEP), with a resolution and a temporal resolution of 6 h. A four-dimensional data assimilation nudging toward the NCEP dataset was performed to increase the accuracy of the WRF. The horizontal model domain of 182×172 grids on a Lambert conformal map projection with a 45 km horizontal resolution is shown in Fig. 1. Vertically, the WRF grid structure consists of 40 layers from the surface to the top of model (10 hPa). Standard meteorological fields were applied by the majority of groups. Several other models were employed to perform simulations using their own meteorological models (e.g., RAMS-CMAQ and GEOS-Chem). WRF-Chem utilized the same model (WRF) as the standard meteorological simulation but considered the feedback of pollutants to the meteorological fields. Consequently, the meteorological fields from this model may be slightly different from the standard. GEOS-Chem is driven by the GEOS-5 assimilated meteorological fields taken from the Goddard Earth Observing System of the NASA Global Modeling Assimilation Office. The meteorological data and CTMs couples varied for each group, likely resulting in a diversified set of model outputs.
MICS-Asia III provided a set of monthly anthropogenic emission inventories for the year 2010 called MIX (Li et al., 2016). MIX is a mosaic of up-to-date regional and national emission inventories that includes Regional Emission inventory in ASia (REAS) version 2.1 for the whole Asian region (Kurokawa et al., 2013), the Multi-resolution Emission Inventory for China (MEIC) developed by Tsinghua University, a high-resolution NH3 emission inventory by Peking University (Huang et al., 2012), an Indian emission inventory developed by Argonne National Laboratory (ANL India, Lu et al., 2011; Lu and Streets, 2012) and the official Korean emission inventory from the Clean Air Policy Support System (CAPSS; Lee et al., 2011). Biogenic emissions were taken from the Model of Emissions of Gases and Aerosols from Nature (MEGAN); hourly biogenic emissions were obtained for the entire year of 2010 using version 2.04 (Guenther et al., 2006). Biomass burning emissions were processed by regridding the Global Fire Emissions Database version 3 (GFEDv3; 0.5∘ by 0.5∘). Volcano SO2 emissions were provided with a daily temporal resolution by the Asia Center for Air Pollution Research (ACAP). The MICS-Asia III emission group directly prepared a gridded inventory according to the configuration of each CTM. NMVOC emissions were spectated into model-ready inputs for three chemical mechanisms: CBMZ, CB05 and SAPRC-99. Weekly and diurnal profiles were also provided. The standard emission inventory was applied by all models. The majority of models employed the official suggested vertical and time profiles of pollutants from each sector by emission group. M13 and M14 made the projections themselves. More information can be found in Li et al. (2017) and Gao et al. (2018).
MICS-Asia III also provided two sets of chemical concentrations for the top and lateral boundaries of the model domain, which were derived from 3-hourly global model outputs for the year 2010. The global models were run by the University of Tennessee (http://acmg.seas.harvard.edu/geos/, last access: 8 October 2019) and Nagoya University (Sudo et al., 2002). GEOS-Chem was run with a horizontal resolution and 47 vertical layers by the University of Tennessee, and the Chemical AGCM for Study of Atmospheric Environment and Radiative Forcing (CHASER) was run with a horizontal resolution with 32 vertical layers by Nagoya University. Some models applied boundary conditions depending on their own past experiences.
2.3 Observational data for O3
In this study, East Asia was divided into three subregions as shown in Fig. 1. The selection of subregions was based on emission, climate and observation data coverage. The North China Plain (EA1) and the Pearl River Delta (EA2) represent highly industrialized regions of the midlatitudes. EA1 is characterized by a temperate and tropical continental monsoon climate with marked seasonality. EA2 is located in southern China and is less affected by continental air masses. EA3 covers the northwest Pacific and the Sea of Japan and represents the downwind regions of the Asian continent with a marine climate.
Hourly O3 and NOx observations for the year 2010 in East Asia were obtained from the CERN, PRD-RAQMN and EANET. The CERN was built by the Institute of Atmospheric Physics, Chinese Academy of Sciences and includes 19 surface stations covering an area of 500km× 500km across the North China Plain (EA1 subregion; Ji et al., 2012). The stations are set up according to United States Environmental Protection Agency method designations. Half of them are remote, rural, suburban and clear urban sites. Nine sites are located within meteorological stations or on campuses of universities in urban regions, with little influence from local sources and sinks. The PRD RAQMN was jointly established by the governments of Guangdong Province and the Hong Kong Special Administrative Region and consists of 16 automatic air quality monitoring stations located across the EA2 subregion (Zhong et al., 2013). Thirteen of these stations are operated by the Environmental Monitoring Centers in Guangdong Province and the other three are located in Hong Kong and are managed by the Hong Kong Environmental Pollution Department. The PRD RAQMN was designed to probe regional air quality, in order to assess the effectiveness of emission reduction measures and to enhance the roles of monitoring networks in characterizing regional air quality and in supporting air quality management. Thus, the sites are rarely influenced by local sources and sinks. The EANET was launched in 1998 to address acid deposition problems in East Asia, following the model of the Cooperative Program for Monitoring and Evaluation of the Long-Range Transmission of Air Pollutants in Europe. In this study, eight remote stations in the northwestern Pacific and Japan (EA3 subregion) were selected to evaluate model performance levels for the downwind regions of the Asian continent (Ban et al., 2016). More information on the EANET can be found at http://www.eanet.asia/ (last access: 8 October 2019). Note that only stations with at least 75 % data validity were chosen. Table S1 in the Supplement provides a detailed site description. Our comparisons of NOx and VOC emission rates conducted on grids for these stations using 45 and 3 km resolution emission inventories suggest that our selected stations rarely received local emissions.
O3 was measured by a Thermo Scientific 49i ozone analyzer with UV photometric technology in the CERN network and by a Thermo Scientific 49C ozone analyzer in the PRD-RAQMN and EANET networks. NOx was measured by a Thermo Scientific 42C analyzer with chemiluminescence technology at 40 sites in all three networks (CERN, PRD-RAQMN and EANET). NOx measurements sometimes exhibited biases (especially for stations located far from sources) when using molybdenum converter devices as all nitrogen oxides were measured. This bias was found to be dependent on the chemical conditions. A 1-month continuous measurement with a chemiluminescence analyzer and Aerodyne cavity-attenuated phase shift spectrometer (CAPS) undertaken in August at an urban site in Beijing shows that this bias from the chemiluminescence analyzer is minor when NO2 concentrations exceed 10–15 ppbv (parts per billion by volume), ranging from 10 % to 30 % under low-NO2 conditions (<10 ppbv) (Ge et al., 2013). Measurements collected from a rural site in South Korea revealed a similar pattern across all seasons (Jung et al., 2017). These comparisons suggest that observations made using molybdenum converters may overestimate NO2 by 10 %–20 % for EA1 and EA2 and 30 % for EA3, introducing uncertainties into the NO2 model evaluation in this study.
Table 2Statistical analysis for surface O3 in three subregions over East Asia. R denotes the correlation coefficient, NMB is the normalized mean bias and RMSE is the root-mean-square error (units are ppbv).
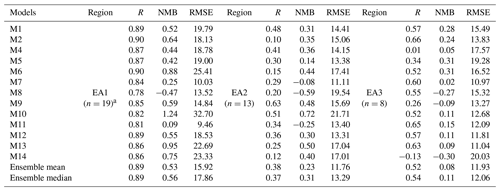
a n represents the number of observation stations.
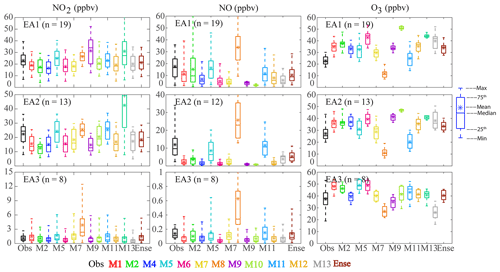
Figure 2Box plots of the observed and simulated annual NO2 (a, d, g), NO (b, e, h) and O3 (c, f, i) frequency distributions determined from 13 models and averaged for stations in EA1, EA2 and EA3 for 2010. n denotes the number of stations. The rectangle represents the interquartile range (25th to 75th percentiles). The asterisk identifies the mean, the continuous horizontal line within the rectangle identifies the median, and whiskers extend between the minimum and maximum values.
3.1 Annual concentrations of surface O3, nitric oxide (NO) and nitrogen dioxide (NO2)
Figure 2 provides a concise comparison of model performances for annual O3, NO and NO2 for three sub regions of East Asia. A box-and-whisker representation was used to show the frequency distribution of monthly concentrations measured from stations in each subregion. The O3 normalized mean bias (NMB) and root-mean-square error (RMSE) of the ensemble mean were found to be significantly less than the ensemble median in most cases (Table 2). Therefore, we only present multi-model mean ensemble results (Ense). In general, the majority of the models significantly overestimated annual surface O3 relative to observations in EA1, EA2 and EA3. Ense overestimated surface O3 by 10–30 ppbv for these subregions. Ense NO2 levels closely reflected observations to within ±20 % across all subregions. In EA1 and EA2, Ense NO levels were found to be 5–10 ppbv lower than observations while exhibiting reasonable levels for EA3.
Of the models, M11 (for subregions EA1 and EA2) and M7 (for EA2 and EA3) more closely reflected O3 observations. M11 simulated O3 with RMSE values of 9.5 and 13.3 ppbv for EA1 and EA2, respectively (Table 2). The models' performance with respect to simulating O3 was found to be closely related to their performance for NO2 and NO. In highly polluted regions (EA1 and EA2), a persistent underestimation of NO was evident across most models. As an interesting phenomenon, we found the models' performance regarding O3 measurements to vary greatly for EA3, although M8 exhibited consistent performance with respect to NO and NO2. This finding suggests that O3 was significantly affected by other factors in addition to local chemistry in EA3. M8 underestimated O3 and overestimated NO in all subregions by 40 %–50 %. The highest O3 titration level observed in M8 may have generated lower O3 levels than those indicated by other models and observations.
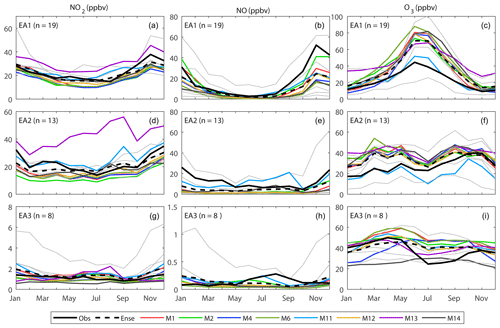
Figure 3Time series of monthly NO2, NO and O3 levels simulated by all models and their ensembles (Ense) in parts per billion by volume (ppbv), averaged over all observed stations across three subregions of East Asia: EA1 (a–c), EA2 (d–f) and EA3 (g–i). Observations are denoted by the solid black line. n represents the number of stations. The gray lines represent NO2, NO and O3 levels simulated by models except M1, M2, M4, M6, M11, M12, M13 and M14.
3.2 Monthly variations of surface O3, NO and NO2
Figure 3 presents monthly mean concentrations of O3, NO and NO2 for the three subregions across East Asia. When two or more observation sites are located in the same model grid, their mean values are used to evaluate model performance. All models captured the observed seasonal cycles of O3, NO and NO2 for EA1. From May to September, Ense O3 was 10–30 ppbv higher than observed values (30 %–70 % of observed values), while Ense NO and NO2 levels appeared to be consistent with observations with mean biases of <3 ppbv. This finding suggests that an intercomparison of O3 production efficiency levels per NOx with observations is needed. For EA2, Ense O3 values agreed well with observed high autumn O3 levels but were overestimated by 5–15 ppbv (15 %–60 % of observations) from January to September. This overestimation reached a maximum in March–April (15 ppbv) and led to a spring peak in simulated O3 values not found in the observations. This overestimation is partly related to the underestimation of NO in the same months, which decreased the titration effect. For NO2, Ense values agreed well with observed values for June–December, and slightly underestimated observations for January–May. For EA3, the ensemble NO2 was generally close to observed values (within ±0.5 ppbv). Significant overestimations of O3 and underestimations of NO were observed from June to October. Similar results were found in MICS-Asia II and in another model intercomparison project of the Task Force on Hemispheric Transport of Air Pollution (TF-HTAP), suggesting that such results may stem from differences in the representation of the dispersion of southwesterly clean marine air masses observed across different metrological fields used in CTMs (Han et al., 2008; Fiore et al., 2009).
For individual models, M11 achieved the highest degree of model reproductivity for monthly mean O3 levels in EA1. Most of the other models overestimated O3 by 100 %–200 % for May–October. The largest levels of model bias and inter-model variability for NO and NO2 appeared in the winter, and likely came from the NOx vertical diffusion and heterogeneous chemistry (Akimoto et al., 2019). In EA2, M7 seems to have achieved the highest levels of O3 reproducibility. Most of the models (except for M11 and M12) showed high O3 concentrations for March–May and September–November. Observed O3 values show that the highest concentrations appeared from October to November. M11 captured the observed January–May O3 value due to relatively high NO concentrations. However, NO was overestimated by M11 from May to September, leading to an underestimation of O3 levels. In EA3, spatially averaged O3 concentrations often differed by more than 20 ppbv in individual models. The highest levels of inter-model variability in O3 values appeared from May to October, overestimating O3 levels relative to observations by 10–40 ppbv. Interestingly, although M8, M9 and M14 exhibited similar magnitudes with observations for June–September, they significantly underestimated observations in other months by 200 %–300 %. A detailed investigation is required in future studies.
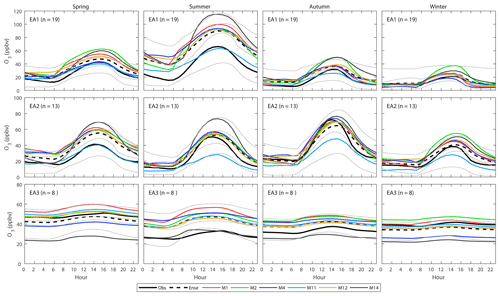
Figure 4Seasonal mean diurnal cycle of surface O3, in parts per billion by volume (ppbv), as a function of hours, for all models and their ensembles, averaged across all observed stations in three subregions of East Asia: EA1 (top row), EA2 (middle row) and EA3 (bottom row). Observations are denoted by the thick black line. n represents the number of stations. The gray lines represent O3 levels simulated by models except M1, M2, M4, M11, M12 and M14. Spring, summer, autumn and winter were defined as March–April–May, June–July–August, September–October–November and December–January–February, respectively.
3.3 Diurnal concentrations of surface O3
Subregional O3 diurnal variations are shown in Fig. 4. In general, model results for three subregions exhibited a larger spread with a magnitude of 10–50 ppbv across the diurnal cycle than those observed in Europe and North America (Solazzo et al., 2012). Summer Ense O3 levels exhibited a systematic pattern of overestimation (20 ppbv) throughout the diurnal cycle in EA1. This indicates that the models had difficulty in estimating summer O3 levels for the North China Plain. Compared with summer conditions, only a slightly systematic overestimation of Ense O3 levels was observed for the other seasons (3–5 ppbv). In EA2, Ense O3 levels generally agreed with summer, autumn and winter observations. In particular, the O3 maximum occurring at around noon was reasonably reproduced. Only a 3–5 ppbv overestimation was observed from 16:00 to 23:00 LT (local time) and in the early morning (06:00 to 10:00 LT). In the spring, a systematic overestimation of Ense O3 values was observed across the whole diurnal cycle (5–10 ppbv). In EA3, Ense captured the minor diurnal variations in O3 across all four seasons, but significantly overestimated observations for the summer and autumn (5–20 ppbv). In the spring and winter, differences between Ense and observations fell within 5 ppbv.
Of all of the models, M11 exhibited the best model performance level with respect to measuring peak daily O3 concentrations of 60 ppbv from 14:00 to 16:00 LT in EA1, but it still overestimated nighttime O3 levels by 10 ppbv. Compared with their performance in simulating summer patterns, the models performed significantly better in simulating winter conditions due to the weak intensity of photochemical reactions except in the case of M2, M10 and M8. Differences between observations and most simulations for both the nighttime and daytime fell within 5 ppbv. These differences in the models' performances between the summer and winter imply that the variety of chemistry parameterizations applied to different models partly explain the inter-model variability of simulated O3 levels in EA1 (North China Plain). For EA2, the majority of models agreed well with diurnal variations occurring in the summer and autumn. However, most models exhibited a tendency to overestimate the O3 concentrations for both the daytime and nighttime in the spring. The overestimated magnitudes exceeded 10 ppbv and 25 ppbv (of observed values of 20–35 ppbv) for the nighttime and daytime, respectively. M11 reproduced observed O3 levels for the spring but underestimated O3 levels for the summer and autumn. For EA3, significant levels of inter-model variability persisted throughout the year. Amplitudes of inter-model variability except for those of M8 and M14 reached approximately 20 ppbv and 10 ppbv in the spring–summer and autumn–winter, respectively. M8 and M14 generated the lowest O3 values of the models for the whole year.
3.4 Error statistics on surface concentrations
In this section, we present statistics on the models' performance based on monthly values. Values are calculated using the equations shown in Appendix A. On a yearly basis, all models showed the highest (0.8–0.9) and lowest (0.1–0.6) correlation coefficients for O3 for EA1 and EA2, respectively (Table 2). High correlations were found in EA1 mainly because the summer-maximum and winter-minimum seasonal cycles are typical of polluted regions represented in all of the participating models. In general, Ense performed better than the individual models in representing NO2 for East Asia, reproducing the observed seasonal cycles and magnitudes. However, Ense did not always exhibit superior performance in simulating O3 levels over individual models for East Asia, which stands in contrast with its performance for Europe (Table 2). M7 and M11 agreed well with observations for EA1 and EA2, whereas Ense tended to overestimate O3 concentrations for May–September in EA1 and for January–September in EA2. Loon et al. (2007) indicated that Ense exhibits superior performance only when the spread of ensemble-model values is representative of O3 uncertainty. This indicates that most models do not reflect this uncertainty or miss key processes of MICS-Asia III.
Considerable overestimations made by most of the models for May–September led to high NMB (0.25–1.25) and RMSE (10–33 ppbv) values for EA1. M11 generated the lowest NMB (0.09) and RMSE (9.46 ppbv) values of the examined models. For EA2, M9 and M10 generated stronger correlations than the other models. However, their corresponding NMB and RMSE values were also the highest. These findings imply that systematic model biases are present in these two models. M7 exhibited lower NMB and RMSE values than the other models, but its correlation was measured as only 0.29. For EA3, correlations exhibited the largest degree of inter-model variability across all subregions, ranging from −0.13 to 0.65. M7 generated the lowest NMB and RMSE, likely due to the canceling effect of its overestimation for the summer and underestimation for other seasons (Fig. 3).
Table 3Statistical analysis for surface NO in three subregions over East Asia. R denotes the correlation coefficient, NMB is the normalized mean bias and RMSE is the root-mean-square error (units are ppbv).
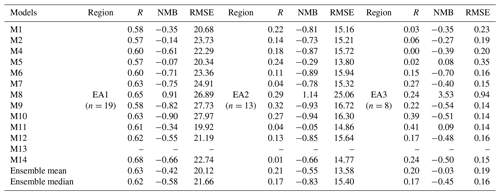
a n represents the number of observation stations.
Table 4Statistical analysis for surface NO2 in three subregions over East Asia. R denotes the correlation coefficient, NMB is the normalized mean bias and RMSE is the root-mean-square error (units are ppbv).
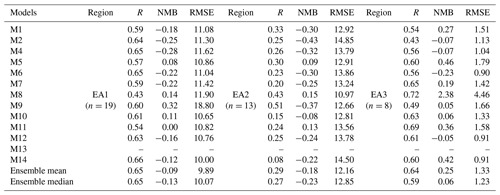
a n represents the number of observation stations.
For NO, model correlations for EA1 ranged from 0.57 to 0.68, showing that all of the models effectively reproduced the spatial variability in NO for this subregion (Table 3). NMBs indicated that underestimations by the models except in the case of M8, mostly occurred for the winter. This underestimation can be partly attributed to the coarse model horizontal resolution (45 km) used in MICS-Asia III, which hardly reproduced concentrations of short-lived species (e.g., NO). In contrast with most of the other models, M8 overestimated NO concentrations for all three subregions. It is noted that NO observations for EA3 were too low (<0.3 ppbv) to be discussed in this study.
Table 4 shows statistics regarding the models' performance in measuring NO2 levels. In general, most of the models performed better with respect to representing NO2 than O3 and NO for EA1. NMBs ranged from −0.28 to 0.32, falling far below those measured for O3 (0.48–1.25). Correlations of 0.54–0.66 were recorded, implying the models' reliable performance in reproducing spatial and monthly variability of NO2 for EA1. Similar to those for O3 and NO, correlation coefficients for NO2 in EA2 remained low. Thus, a dedicated investigation of O3, NO and NO2 levels in EA2 is urgently needed, but falls beyond the scope of this study. In EA3, correlation coefficients ranged from 0.43- to 0.72. NMBs and RMSEs, except for those of M8, ranged from −0.23 to 0.46 and 0.90 to 1.79 ppbv, respectively.
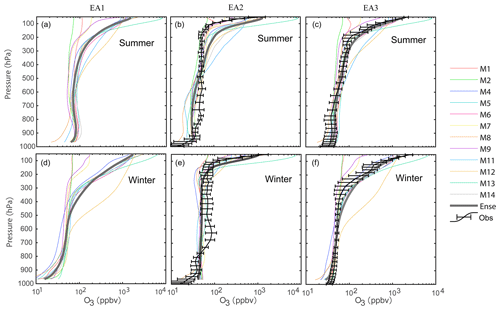
Figure 5Simulated O3 profiles for the summer (June–July–August) and winter (December–January–February) of 2010, averaged over all observed stations across three subregions of East Asia: EA1 (a, d), EA2 (b, e) and EA3: (c, f). Ozonesonde data for 2010 were taken from the World Ozone and Ultraviolet Radiation Data Centre (WOUDC) database.
3.5 Vertical profiles of O3
Figure 5 shows the vertical profiles of the observed and simulated O3 levels for East Asia for the summer and winter. Ensemble means (Ense) showed underestimations and overestimations of EA2 O3 levels for the middle (500–800 hPa) and lower (below 900 hPa) troposphere, respectively. In the winter, underestimations extended to 200 hPa. Magnitudes of underestimations and overestimations reached 10–40 and 10–20 ppbv, respectively. For EA3, Ense reproduced the vertical structure of O3 for both the summer and winter. An overestimation of the region below 800 hPa, with a magnitude of 10–20 ppbv, was observed for the summer.
High levels of inter-model variability in O3 exceeding 300 hPa was evident across all subregions, which was attributable to the varied top boundary conditions applied by the models. However, this considerable variability was not transmitted to the middle troposphere (400–600 hPa), in which O3 concentrations were consistent across the models. In the lower troposphere, a minor level of inter-model variability below 900 hPa appeared in the winter in three subregions, and slowly decreased with height. Mean standard deviations (SD) of models below 900 hPa were recorded as 7.6 6.9 and 6.0 ppbv for EA1, EA2 and EA3, respectively, covering 18.3 %, 15.0 % and 15.4 % of mean O3 concentrations. In the 700–900 hPa region, SD levels decreased to 5.4, 4.4 and 4.8 ppbv for EA1, EA2 and EA3, representing 12.2 %, 9.4 % and 10.8 % of mean O3 concentrations, respectively.
In the lower troposphere, inter-model variability in the summer was generally higher than that in the winter. In polluted regions (EA1), SD levels reached 16.3 ppbv (20.8 % of mean concentrations) in the summer, greatly exceeding those in winter (6.2 ppbv, 15.2 %). Various vertical structures of O3 were found below 700 hPa in summer. O3 concentrations slowly increased with height in M8 and M11, but they mixed well in the planetary boundary layer (PBL) and decreased from 800 to 700 hPa in the other models. Akimoto et al. (2019) found that the parameterization on downward O3 transport from the upper boundary layer contributed considerably to discrepancies between M1, M6 and M11. In EA2, vertical structures of O3 among models were found to be consistent, but concentrations differed more than those in EA1. SD values covered 22 % of mean concentrations.
4.1 Spatial distributions of single-model and multi-model ensemble O3
Figure 6 shows the spatial distributions of MICS-Asia III ensemble mean surface O3 values (Ense) and the coefficient of variation (CV). The CV is defined as the standard deviation of the modeled O3 divided by the average. The larger the CV value, the lower the degree of consistency among the models. For the summer, Ense predicted an elevated O3 concentration belt in the midlatitudes (30–45∘ N). A region of O3 in excess of 60 ppbv stretched across the North China Plain and East China Sea, far exceeding MICS-Asia II (45–50 ppbv) values for 2001 (Han et al., 2008). In other seasons, the O3 distribution showed higher O3 over the ocean than in eastern China, reflecting O3 titration from high NOx emissions. Due to the stratospheric injection, surface O3 over the Tibetan Plateau remained at high levels throughout the year, ranging from 50 to 65 ppbv. The seasonal cycle of surface O3 levels determined from Ense via MICS-Asia III agreed with that observed from MICS-Asia II, but O3 levels in polluted regions were higher (Han et al., 2008).
The CV ranged from 0.1 to 0.6 in East Asia. The high values were found in EA1 in the winter. These high values in the low-latitude western Pacific (10∘ S–15∘ N) and Indian oceans were likely caused by the treatment of lateral boundaries in the models. For MICS-Asia III, M7, M8 and M9 employed the default model configurations, and the others employed outputs of the GEOS-Chem/CHASER/MOZART-GOCART global model. Compared with those of MICS-Asia II, CVs for the Asian continent except for the winter remained at similar levels in this study (0.1–0.3) (Carmichael et al., 2008).
Although all of the models similarly predicted the emergence of an elevated summer O3 concentration belt in the midlatitudes (30–45∘ N), the magnitudes of enhanced O3 levels varied between the models (Fig. 7). M5 predicted the highest O3 concentrations of 60–90 ppbv for the North China Plain (EA1) and for its outflow pathways including the Bohai Sea, East China Sea, Korea, Japan and the Sea of Japan (locations are shown in Fig. S1 in the Supplement), whereas M8 predicted the lowest levels of 35–50 ppbv. Overhangs of 30 ppbv contour lines extending into the northwestern Pacific along the Asian continent outflow plume differed considerably between the models. A plume of 30 ppbv, or higher O3 levels, was simulated in M1–M6, M13 and M14, reaching further south and east of Japan (135∘ E, 20∘ N), than those of M8, M10 and M11 (120∘ E, 30∘ N). From MICS-Asia II and HTAP, differences in the frequency of marine air masses from the western Pacific Ocean were thought to be a possible cause of O3 discrepancies observed over oceans between the models due to different meteorological drivers (Han et al., 2008). For MICS-Asia III, wind fields employed by the models were similar due to the use of the same or similar meteorological fields (Fig. S2). These inconsistencies between the models resulted from the combined effects of a series of factors, including the diversity of the condensed gas chemical mechanism and heterogeneous chemistry. Li et al. (2016) found chemical production to be the dominant controlling factor of O3 along outflow pathways near the North China Plain in the summer rather than lateral and top boundary conditions. Impacts of aerosols on O3 in these regions have frequently been reported (e.g., Olson et al., 1997 and Li et al., 2018) to alter photolysis rates and heterogeneous chemistry patterns. Detailed comparisons of parameterizations of these processes in models are needed in future inter-model comparison projects focused on Asia.
In the winter, distribution patterns of O3 were quite similar between the models with high concentrations observed over parts of western China, northeastern India and the western Pacific from the East China Sea to southern Japan (Fig. S3). In the spring and autumn (Figs. S4 and S5), O3 concentrations were generally higher than they were in the winter across the whole model domain due to the enhancement of solar radiation or stratosphere–troposphere exchange fluxes of O3. All of the models exhibited an enhancement of O3 levels over southern Tibet, northeastern India and the western Pacific, generally echoing patterns observed in the winter. Increases of O3 further north in Japan were comparable with winter.
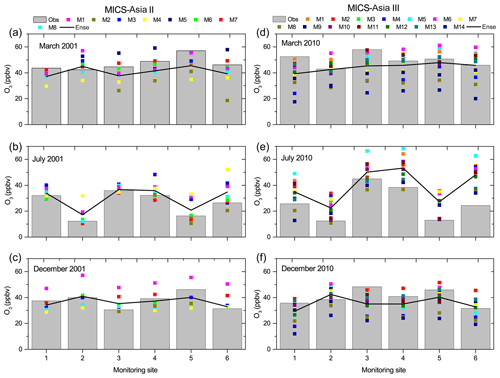
Figure 8Modeled and observed monthly mean concentrations of O3 for EANET sites in phases II (a–c) and III (d–f) of the MICS-Asia project. The solid line represents the ensemble mean. Note that MICS-Asia II and III data refer to March, July and December of 2001 and 2010, respectively. IDs of the monitoring sites denote the following: 1 – Rishiri (45.12∘ N, 141.23∘ E), 2 – Ogasawara (27.83∘ N, 142.22∘ E), 3 – Sado-seki (38.23∘ N, 138.4∘ E), 4 – Oki (36.28∘ N, 133.18∘ E), 5 – Hedo (26.85∘ N, 128.25∘ E) and 6 – Banryu (34.67∘ N, 131.80∘ E)
4.2 Comparison with MICS-Asia II
From MICS-Asia II, model evaluation of O3 was conducted on sites in the western Pacific. Figure 8 presents the simulated and observed surface O3 levels at these monitoring sites derived from phases II and III of the MICS-Asia project. Note that different models were employed in the two phases. In general, most of the models captured distributions of O3 mixing ratios at most sites in both MICS-Asia II and III. Ense results were consistent for March and December of 2001 and 2010. Underestimations of O3 levels in March at the Japanese sites (Site 4 – Oki, Site 5 – Hedo and Site 6 – Banryu) in Phase II were largely remedied in Phase III. However, surface O3 observed in western Japan (Site 4 – Oki, Site 5 – Hedo and Site 6 – Banryu) were severely overestimated in July 2010 by 10–30 ppbv. This overestimation was not found in Phase II, for which differences from observations were valued at approximately 5 ppbv. Rural sites in western Japan are located in the upwind regions of Japanese domestic emissions, and are subjected to the impacts of Asian continent outflows. Overestimated O3 values for the North China Plain (EA1) in Phase III contributed considerably to enhanced concentrations simulated for western Japanese sites in July 2010. This indicates that transboundary transport from the Asian continent according to MICS-Asia III was likely overestimated relative to that measured from MICS-Asia II.
In reference to MICS-Asia II, Han et al. (2008) hypothesized that variations in meteorological fields, dry deposition, PBL, model treatments of chemistry and other physical processes contributed to model biases in relation to observations and inter-model variability. Quantifying the contributions of these processes can help explain model biases through sensitivity simulations. However, this task comes with tremendous computational costs when applied to 14 models. The qualitative analysis of potential causes of these processes based on comparisons of models and observations is essential to selecting sensitivity simulating scenarios for the next phase of MICS-Asia. In MICS-Asia III, common input data (emission and meteorology) were effectively used in this qualitative analysis based on model parameterizations. We evaluated the models on dry deposition, PBL and chemistry by collecting their observations (dry deposition velocity and PBL height). This work was not conducted under MICS-Asia II and is intended to help model developers improve model performance for East Asia.
5.1 Dry deposition
Previous studies show that dry deposition processes serve as the key net sink of O3, accounting for roughly 25 % of the total O3 removed from the troposphere (Lelieveld and Dentener, 2000). The uncertainty of dry deposition in CTMs is still high because many processes are heavily parameterized in models (Hardacre et al., 2015). In this study, the simulated dry deposition velocities of O3 were compared. Simulated deposition velocities were calculated from Eq. (1):
where F and C represent the simulated dry deposition flux and surface O3 concentrations, respectively. We determined spatial mean dry deposition velocities from stations in each subregion.
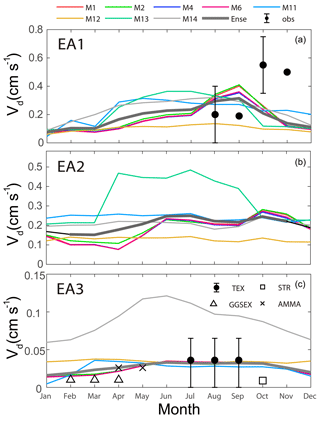
Figure 9Simulated and observed monthly O3 dry deposition velocities (Vd) for M1, M2, M4, M6, M11, M12, M13 and M14 for three subregions of East Asia: EA1 (a), EA3 (b) and EA3 (c). TEX, STR, GGSEX and AMMA denote observations for TexAQS06 (7 July–12 September 2006; northwestern Gulf of Mexico), STRATUS06 (9–27 October 2006; the persistent stratus cloud region off the coast of Chile in the eastern Pacific Ocean), GasEx08 (29 February–11 April 2008; the Southern Ocean), and AMMA08 (27 April–18 May 2008; the southern and northern Atlantic Ocean). Observational data were taken from Sorimachi et al. (2003), Pan et al. (2010) and Helmig et al. (2012).
Figure 9 presents the simulated and observed monthly spatial mean dry deposition velocities of O3. For EA1, ensemble mean values overestimated observed dry deposition velocities of O3 (vd) for August–September, but they still fell within the range of the observed standard deviation. This shows that factors other than dry deposition could play important roles in overestimations of August–September O3 values in EA1. In October–November, simulated vd apparently underestimated observations by 30 %–50 %. Among the models, the lower dry deposition velocities in May–July for M1, M2, M4 and M6 than that of M11 partly explained the higher May–July surface O3 from those simulations than the value from in M11. However, M13 and M14 still produced high O3 concentrations in May–September, although their dry deposition velocities were similar to that of M11 (Fig. 3). Notably, our observations were made on grassland, which covers ∼20 % of the land area in EA1. There are few vd observations on agriculture crops (50 % of the land area) in North China. Hardacre et al. (2015) reported O3 dry deposition measurements on crops in Europe and simulated O3 dry deposition in 15 global models. Both observations and simulations showed that O3 dry deposition velocities on the agriculture crop class were quite similar to those of grassland, displaying that uncertainties related to the representativeness of measurement sites used in this study did not affect our conclusions.
For EA2, similar features to those of EA1 were found. M1, M2, M4 and M6 were quite consistent with each other, with a seasonal cycle and a spring minimum. M11, M12 and M14 show no obvious signs of seasonal variability with a magnitude of 0.1–0.2 cm s−1. Seasonal patterns in M13 are considerably different from those of the other models, exhibiting a maximum in April–September with higher dry deposition velocities (0.5 cm s−1). The performance of the models for dry deposition velocities was not always consistent with O3 concentrations. For example, O3 concentrations in M13 remained high under higher dry deposition velocities.
In EA3, most stations were located at remote oceanic sites, and few dry deposition observations were made. Thus, we collected observations from other oceanic sites to evaluate model performance (Helmig et al., 2012). Ense values for vd agreed reasonably well with observations (Fig. 9). Both observations and simulated vd values showed a July–September maximum with a magnitude of 0.02–0.03 cm s−1. Park et al. (2014) found surface O3 levels in EA3 to be more sensitive to dry deposition parameterization schemes in CTMs. O3 measured from oceans differed by 5–15 ppbv in East Asia due to the use of various dry deposition parameterization schemes. Thus, more observations are needed over oceans in EA3 to mitigate O3 simulation uncertainties.
5.2 Relationships between surface NOx and O3
In general, surface O3 mainly comes from photochemistry processes involving NOx and VOCs in polluted regions. Examining O3−NOx relationships is effectual to investigating sources of inter-model variability and model errors concerning O3 chemistry in East Asia. Fig. 10 presents O3 concentrations as a function of NOx in May–September based on the monthly daytime (08:00–20:00 LT) mean observed and simulated results for the stations shown in Fig. 1.
For EA1 (North China Plain), observations clearly show that O3 concentrations decreased with an increase in the NOx concentrations. O3 concentrations mostly remained at high levels (40–60 ppbv) when NOx was less than 20 ppbv. The slope and intercept of the regression line between observed O3 and NOx were measured as −0.77 ppbv ppbv−1 and 59.5 ppbv, respectively. Among the models, M11 results were in relative agreement with observations. The slope and intercept (−1.01 ppbv ppbv−1, 63.23 ppbv) reflected the observations. The other models showed a higher degree of model bias and inter-model variability in relationships between O3 and NOx. Their slopes mostly ranged from −1.25 to −2.13 ppbv ppbv−1, amounting to 1.3–2.8 times the observed slope. Their intercepts were 74.9–121.2 ppbv, far exceeding observations (59.5 ppbv). Akimoto et al. (2019) calculated the net photochemical production of M1, M6 and M11 and found that weak net chemical production in M11 was mostly responsible for low O3 compared with those in M1 and M6. This finding is consistent with the low slope in M11. To reduce the impact of O3 buildup and transport due to NOx consumption, the relationship between Ox (NO2+O3) and NOx was compared (Fig. S7). Observed Ox increases with an increase in NOx levels, with a coefficient of determination (R2) of 0.61. Most of the models (except for M8, M11 and M13) failed to reproduced observed positive correlations between Ox and NOx, and their R2 only ranged from 0.01 to 0.08. The slope, intercept and R2 of M8 and M11 were in relative agreement with observations.
For EA2, all models reproduced observed key patterns in which Ox positively correlated with NOx. For O3−NOx relationships, M1, M2, M4 and M6 reproduced observed O3 levels under low-NOx conditions (<30 ppbv) but failed to capture low O3 under high-NOx conditions (30–40 ppbv), accounting for overestimations of these models for O3 in May–September. By contrast, M8 and M11 produced excessively high NOx values, resulting in their underestimations of O3 values. For M13 and M14, O3 concentrations were nearly constant at all levels of NOx. O3 was positively correlated with NOx in M9 and M10, which stands in contrast with observations. This finding suggests that more attention is required when using M9, M10, M13 and M14.
Stations in EA3 are mostly located over clean oceans or islands. NOx concentrations were less than 3 ppbv, showing that local chemistry was not a key factor shaping O3 formation. Thus, we did not examine the simulated O3–NOx relationship further.
5.3 Other factors
Previous studies show that O3 precursors are mostly constrained within the boundary layer (Quan et al., 2013). The planetary boundary layer height (PBLH) model evaluation is essential for the interpretation of model biases with observations. Unfortunately, this evaluation was not applied in MICS-Asia II. In 2016, Guo et al. (2016) calculated the PBLH using the bulk Richardson number (Ri) method from the radiosonde network of the L-band sounding system of the China Meteorological Administration (Vogelezang and Holtslag, 1996). The system provides fine-resolution profiles of temperature, pressure, relative humidity, wind speed and direction. In MICS-Asia III, all selected models exhibited the spring-maximum and winter-minimum seasonal cycle for EA1 (Fig. S6), capturing the main climatological pattern of PBLH observations (Guo et al., 2016). The Ense on the PBLH only overestimated radiosonde measurements by 100–200 m (∼10–15 %), likely due to sampling bias between the models and measurements. The simulation was recorded as the mean value of 12 h (08:00–20:00 LT), while the average of the measurements was calculated based on a 3 h period (08:00, 14:00 and 20:00 LT). For EA2, the observed PBLH did not vary as much as that for EA1, and differences between seasons ranged within 100 m. This pattern was captured by the models. As was observed from EA1, the simulated PBLH for EA2 exceeded the measurements by 100–200 m. Few measurements of remote oceanic sites in East Asia were collected. Thus, we compared simulations with European Centre for Medium-Range Weather Forecasts Reanalysis data (von Engeln et al., 2013). Both showed a winter-maximum pattern for PBLH.
Under the MICS-Asia III framework, the evaluation and intercomparison of 13 CTMs were conducted using a wide variety of observations covering two Chinese industrialized regions and the western Pacific, using continuous simulations for 2010 with a focus on O3, NO and NO2. In particular, surface O3 levels in China, which were neglected in previous model intercomparison projects, were evaluated. Considerable levels of inter-model variability in O3 were observed across all subregions of East Asia, with model concentrations varying by factors of 2 to 3 between different models.
A model ensemble was produced and evaluated. In general, the model ensemble captured key patterns of monthly and diurnal O3, NO and NO2 in the North China Plain and on the western Pacific Rim. It failed to capture the observed seasonal cycle of O3 for the Pearl River Delta. For the North China Plain and the western Pacific Rim, the model ensemble severely overestimated surface O3 levels for May–September by 10–30 ppbv. This overestimation systematically appeared at both daytime and nighttime. Similarly, the model ensemble tended to overestimate spring daytime and nighttime O3 concentrations for the Pearl River Delta. Compared with MICS-Asia II, MICS-Asia III was less prone to underestimating surface O3 in March for Japanese sites. However, it predicted excessively high surface O3 concentrations for western Japan in July, which was not the case for MICS-Asia II. In term of O3 soundings, the ensemble model used in this study reproduced the vertical structure in the western Pacific, but overestimated O3 below 800 hPa in the summer. For the industrialized Pearl River Delta, the ensemble average presented an overestimation of O3 levels for the lower troposphere and underestimations in the middle troposphere. We find that the ensemble average of 13 models for O3 did not always perform better than individual models for East Asia in contrast with their performance for Europe. This suggest that the spread of ensemble-model values does not represent all uncertainties in O3 levels or that most MICS-Asia III models missed key processes. In contrast to performance levels for O3, Ense performed better than individual models for NO2 in East Asia.
MICS-Asia II outlines potential causes of variability among models. Quantifying the contributions of these processes to O3 concentrations serves as an effective way to explain model biases through sensitivity simulations. However, this would incur tremendous computational costs when applied to 14 models. In this study, we conducted a qualitative analysis of potential causes by comparing models and observations for these processes to identify sensitivity simulating scenarios for the next phase of MICS-Asia. Our comparisons show that the ensemble model overestimated observed dry deposition velocities of O3 for August–September in the North China Plain, displaying that other factors rather than dry deposition may contribute to the overestimation of the simulated O3 concentrations in the summer. For the western Pacific, simulated vd values agreed reasonably well with observations. Photochemical treatment in models may contribute to O3 overestimations in the North China Plain. The models studied captured the major climatological pattern of PBLH observations for three subregions of East Asia. More evaluations of turbulent kinetic energy in the PBL are needed to assess vertical mixing in future studies.
Data in Figs. 2, 3, 4, 5 and 9 can be obtained in https://figshare.com/s/cf48231dcd9529fc3bc6 (Li, 2019, last access date: 8 October 2019). The outputs from the simulations in Figs. 6, 7, 8 and 10 are available at https://pan.baidu.com/s/1IaaCDhrAR-z2tO6yQNz2cg (Chen et al., 2019, last access date: 8 October 2019).
Defining yij and Obsij (modeled and observed, respectively) of the ith monthly concentrations of air pollutants at the jth station, with respective mean values of and . m and n represent the number of stations and months, respectively.
A1 Correlation coefficient (R)
A2 Root-mean-square error (RMSE)
A3 Normalized mean bias (NMB)
The supplement related to this article is available online at: https://doi.org/10.5194/acp-19-12993-2019-supplement.
JL, ZW and GC designed the study. JL, TN, BG, KY, JF, XW, QF, SI, HL, CK, CL, MZ, ZT, MK, HL and ZW contributed to modeling data. ML, JW, JK and QW provided the emission data. LK helped with data processing. HA, GC and ZW were involved in the scientific interpretation and discussion. JL prepared the paper with contributions from all co-authors.
The authors declare that they have no conflict of interest.
This article is part of the special issue “Regional assessment of air pollution and climate change over East and Southeast Asia: results from MICS-Asia Phase III”. It is not associated with a conference.
This work was supported by the Natural Science Foundation of China (grant nos. 41620104008, 41571130034, 91544227 and 91744203), and the National Key R&D Program of China (grant nos. 2017YFC0212402 and 2018YFC0213205). This work was partly supported by the Environment Research and Technology Development Fund (grant no. S-12) of the Environmental Restoration and Conservation Agency of Japan and the Ministry of Environment, Japan. We thank the Pearl River Delta Regional Air Quality Monitoring Network for observations in the Pearl River Delta. Kengo Sudo from Nagoya University and Rokjin J. Park provided us with CHASER and GEOS-Chem outputs for boundary conditions. This paper was edited by Wallace Academic Editing.
This research has been supported by the National Key R&D Program of China (grant no. 2017YFC0212402), the Natural Science Foundation of China (grant nos. 41571130034, 91544227, 91744203, 41225019 and 41425020), and the Environment Research and Technology Development Fund of the Environmental Restoration and Conservation Agency of Japan and the Ministry of Environment, Japan (grant no. S-12).
This paper was edited by Yugo Kanaya and reviewed by two anonymous referees.
Ackermann, I. J., Hass, H., Memmesheimer, M., Ebel, A., Binkowski, F. S., and Shankar, U.: Modal aerosol dynamics model for Europe: Development and first applications, Atmos. Environ., 32, 2981–2999, 1998.
Akimoto, H., Mori, Y., Sasaki, K., Nakanishi, H., Ohizumi, T., and Itano, Y.: Analysis of monitoring data of ground-level ozone in japan for long-term trend during 1990–2010: causes of temporal and spatial variation, Atmos. Environ., 102, 302–310, 2015.
Akimoto, H., Nagashima, T., Li, J., Fu, J. S., Ji, D., Tan, J., and Wang, Z.: Comparison of surface ozone simulation among selected regional models in MICS-Asia III – effects of chemistry and vertical transport for the causes of difference, Atmos. Chem. Phys., 19, 603–615, https://doi.org/10.5194/acp-19-603-2019, 2019.
Ban, S., Matsuda, K., Sato, K., and Ohizumi, T.: Long-term assessment of nitrogen deposition at remote EANET sites in Japan, Atmos. Environ., 146, 70–78, 2016.
Binkowski, F. S. and Roselle, S. J.: Models 3-Community Multiscale Air Quality (CMAQ) model aerosol component:1. Model description, J. Geophys. Res.-Atmos., 108, 4183, https://doi.org/10.1029/2001JD001409, 2003.
Byun, D. W. and Schere, K. L.: Rewiew of the governing equations, computational algorithms, and other components of the Models-3 Community Multiscale Air Quality (CMAQ) modeling system, Appl. Mech. Rev., 59, 51–77, 2006.
Carlton, A. G., Turpin, B. J., Altieri, K. E., Seitzinger, S., Reff, A., Lim, H.-J., and Ervens, B.: Atmospheric oxalic acid and SOA production from glyoxal: Results of aqueous photooxidation experiment, Atmos. Environ., 41, 7588–7602, 2007.
Carmichael, G. R., Calori, G., Hayami, H., Uno, I., Cho, S. Y., Engardt, M., Kim, S. B., Ichikawa, Y., Ikeda, Y., Woo, J. H., Ueda, H., and Amann, M.: The MICS-Asia study: model intercomparison of long-range transport and sulfur deposition in East Asia, Atmos. Environ., 36, 175–199, 2002.
Carmichael, G. R., Sakurai, T., Streets, D., Hozumi, Y., Ueda, H., Park, S. U., Fung, C., Han, Z., Kajino, M., Engardt, M., Bennet, C., Hayami, H., Sartelet, K., Holloway, T., Wang, Z., Kannari, A., Fu, J., Matsuda, K., Thongboonchoo, N., and Amann, M.: MICS-Asia II: The model intercomparison study for Asia Phase II methodology and overview of findings, Atmos. Environ., 42, 3468–3490, 2008.
Carter, W. P. L.: Implementation of the SAPRC-99 Chemical Mechanism into the Models-3 Framework, Report to the United States Environmental Protection Agency, available at: http://www. engr.ucr.edu/~carter/pubs/s99mod3.pdf (last access: 6 February 2015), 2000.
Chen, L., Gao, Y., Zhang, M., Fu, J. S., Zhu, J., Liao, H., Li, J., Huang, K., Ge, B., Wang, X., Lam, Y. F., Lin, C.-Y., Itahashi, S., Nagashima, T., Kajino, M., Yamaji, K., Wang, Z., and Kurokawa, J.: MICS-Asia III: multi-model comparison and evaluation of aerosol over East Asia, available at: https://pan.baidu.com/s/1IaaCDhrAR-z2tO6yQNz2cg, last access: 8 October 2019.
Chin, M., Ginoux, P., Kinne, S., Torres, O., Holben, B., Duncan, B.N., Martin, R.V., Logan, J., Higurashi, A., and Nakajima T.: Tropospheric aerosol optical thickness from the GOCART model and comparisons with satellite and sun photometer measurements, J. Atmos. Phys., 59, 461–483, 2012.
Colella, P. and Woodward, P. L.: The piecewise parabolic method (PPM) for gas-dynamical simulations, J. Comput. Phys., 54, 174–201, 1984
Easter, R. C., Ghan, S. J., Zhang, Y., Saylor, R. D., Chapman, E. G., Laulainen, N. S., Abdul-Razzak, H., Leung, L. R., Bian, X. D., and Zaveri, R. A.: MIRAGE: Model description and evaluation of aerosols and trace gases, J. Geophys. Res.-Atmos., 109, 46, https://doi.org/10.1029/2004jd004571, 2004.
Fiore, A. M., Dentener, F. J., Wild, O., Cuvelier, C., Schultz, M. G., Hess, P., Textor, C., Schulz, M., Doherty, R. M., Horowitz, L. W., MacKenzie, I. A., Sanderson, M. G., Shindell, D. T., Stevenson, D. S., Szopa, S., van Dingenen, R., Zeng, G., Atherton, C. S., Bergmann, D. J., Bey, I., Carmichael, G. R., Collins, W. J., Duncan, B. N., Faluvegi, G., Folberth, G. A., Gauss, M., Gong, S., Hauglustaine, D., Holloway, T., Isaksen, I. S. A., Jacob, D. J., Jonson, J. E., Kaminski, J. W., Keating, T. J., Lupu, A., Marmer, E., Montanaro, V., Park, R. J., Pitari, G., Pringle, K. J., Pyle, J. A., Schroeder, S., Vivanco, M. G., Wind, P., Wojcik, G., Wu, S., and Zuber, A.: Multimodel estimates of intercontinental source-receptor relationships for ozone pollution, J. Geophys. Res.-Atmos., 114, 83–84, 2009.
Gao, M., Han, Z., Liu, Z., Li, M., Xin, J., Tao, Z., Li, J., Kang, J.-E., Huang, K., Dong, X., Zhuang, B., Li, S., Ge, B., Wu, Q., Cheng, Y., Wang, Y., Lee, H.-J., Kim, C.-H., Fu, J. S., Wang, T., Chin, M., Woo, J.-H., Zhang, Q., Wang, Z., and Carmichael, G. R.: Air quality and climate change, Topic 3 of the Model Inter-Comparison Study for Asia Phase III (MICS-Asia III) – Part 1: Overview and model evaluation, Atmos. Chem. Phys., 18, 4859-4884, https://doi.org/10.5194/acp-18-4859-2018, 2018.
Ge, B., Sun, Y., Liu, Y., Dong, H., Ji, D., Jiang, Q., Li, J., and Wang, Z.: Nitrogen dioxide measurement by cavity attenuated phase shift spectroscopy (CAPS) and implications in ozone production efficiency and nitrate formation in Beijing, China, J. Geophys. Res.-Atmos., 118, 9499–9509, https://doi.org/10.1002/jgrd.50757, 2013.
Gipson, G. L.: The Initial Concentration and Boundary Condition Processors. In Science algorithms of the EPA Models-3 Community Multiscale Air Quality (CMAQ) Modeling System, US Environmental Protection Agency Report, EPA-600/R-99/030, 12-1–12-91, 1999.
Goliff, W. S., Stockwell, W. R., and Lawson, C. V.: The regional atmospheric chemistry mechanism, version 2, Atmos. Environ., 68, 174–185, 2013.
Guo, J., Miao, Y., Zhang, Y., Liu, H., Li, Z., Zhang, W., He, J., Lou, M., Yan, Y., Bian, L., and Zhai, P.: The climatology of planetary boundary layer height in China derived from radiosonde and reanalysis data, Atmos. Chem. Phys., 16, 13309–13319, https://doi.org/10.5194/acp-16-13309-2016, 2016.
Guenther, A., Karl, T., Harley, P., Wiedinmyer, C., Palmer, P. I., and Geron, C.: Estimates of global terrestrial isoprene emissions using MEGAN (Model of Emissions of Gases and Aerosols from Nature), Atmos. Chem. Phys., 6, 3181–3210, https://doi.org/10.5194/acp-6-3181-2006, 2006.
Han, Z., Sakurai, T., Ueda, H., Carmichael, G. R., Streets, D., Hayami, H., Wang, Z., Holloway, T., Engardtg, M., Hozumib, Y., Parkh, S.U., Kajinoi, M., Sarteletj, K., Fungk, C., Bennetg, C., Thongboonchooc, N., Tangc, Y., Changk, A., Matsudal, K., and Amannm, M.: MICS-ASIA II: model intercomparison and evaluation of ozone and relevant species, Atmos. Environ., 42, 3491–3509, 2008.
Hardacre, C., Wild, O., and Emberson, L.: An evaluation of ozone dry deposition in global scale chemistry climate models, Atmos. Chem. Phys., 15, 6419–6436, https://doi.org/10.5194/acp-15-6419-2015, 2015.
He, J., Zhang, Y., Wang, K., Chen, Y., Leung, L. R., Fan, J., Li, M., Zheng, B., Zhang, Q., Duan, F., and He, K. B.: Multi-year application of WRF-CAM5 over East Asia-Part II: Interannual variability, trend analysis, and aerosol indirect effects, Atmos. Environ., 165, 122–142, 2017.
Helmig, D., Lang, E. K., Bariteau, L., Boylan, P., Fairall, C. W., Ganzeveld, L., Hare, J. E., Hueber, J., and Pallandt, M.: Atmosphere-ocean ozone fluxes during the TexAQS 2006, STRATUS 2006, GOMECC 2007, GasEx 2008, and AMMA 2008 cruises, J. Geophys. Res., 117, D04305, https://doi.org/10.1029/2011JD015955, 2012.
Horowitz, L. W., Walters, S. M., Mauzerall, D. L., Emmons, L. K., Rasch, P. J., Granier, C., Tie, X., Lamarque, J.-F., Schultz, M. G., and Brasseur, G. P: A global simulation of tropospheric ozone and related tracers: description and evaluation of MOZART, version 2, J. Geophys. Res.-Atmos., 108, 4784, https://doi.org/10.1029/2002JD002853, 2003.
Huang, X., Song, Y., Li, M., Li, J., Huo, Q., Cai, X., Zhu, T., Hu, M., and Zhang, H.: A high-resolution ammonia emission inventory in China, Global Biogeochem. Cy., 26, GB1030, https://doi.org/10.1029/2011GB004161, 2012.
Ji, D., Wang, Y., Wang, L., Chen, L., Hub, B., Tang, G., Xin, J., Song, T., Wen, T., Sun, Y., Pan, Y., and Liu, Z.: Analysis of heavy pollution episodes in selected cities of northern China, Atmos. Environ., 50, 338–348, 2012.
Jung, J., Lee, J. Y., Kim, B. M., and Oh, S. H.: Seasonal variations in the NO2 artifact from chemiluminescence measurements with a molybdenum converter at a suburban site in Korea (downwind of the Asian continental outflow) during 2015–2016, Atmos. Environ., 165, 290–300, 2017.
Kajino, M., Inomata, Y., Sato, K., Ueda, H., Han, Z., An, J., Katata, G., Deushi, M., Maki, T., Oshima, N., Kurokawa, J., Ohara, T., Takami, A., and Hatakeyama, S.: Development of the RAQM2 aerosol chemical transport model and predictions of the Northeast Asian aerosol mass, size, chemistry, and mixing type, Atmos. Chem. Phys., 12, 11833–11856, https://doi.org/10.5194/acp-12-11833-2012, 2012.
Kurokawa, J., Ohara, T., Morikawa, T., Hanayama, S., Janssens-Maenhout, G., Fukui, T., Kawashima, K., and Akimoto, H.: Emissions of air pollutants and greenhouse gases over Asian regions during 2000–2008: Regional Emission inventory in ASia (REAS) version 2, Atmos. Chem. Phys., 13, 11019–11058, https://doi.org/10.5194/acp-13-11019-2013, 2013.
Lee, D. G., Lee, Y. M., Jang, K. W., Yoo, C., Kang, K. H., Lee, J. H., Jung, S. W., Park, J. M., Lee, S. B., Han, J. S., Hong, J. H., and Lee, S. J.: Korean national emissions inventory system and 2007 air pollutant emissions, Asian J. Atmos. Environ., 5, 278–291, 2011.
Lelieveld, J. and Dentener, F. J.: What controls tropospheric ozone?, J. Geophys. Res., 105, 3531–3551, https://doi.org/10.1029/1999JD901011, 2000
Li, J., Wang, Z., Akimoto, H., Gao, C., Pochanart, P., and Wang, X.: Modeling study of ozone seasonal cycle in lower troposphere over East Asia, J. Geophys. Res.-Atmos., 112, D22S25, https://doi.org/10.1029/2006JD008209, 2007.
Li, J., Chen, X., Wang, Z., Du, H., Yang, W., Sun, Y., Hu, B., Li, J. J., Wang, W., Wang, T., Fu, P., and Huang, H.: Radiative and heterogeneous chemical effects of aerosols on ozone and inorganic aerosols over East Asia, Sci. Total Environ., 622, 1327–1342, 2018.
Li, J., Wang, Z., Akimoto, H., Yamaji, K., Takigawa, M., Pochanart, P., Liu, Y., Tanimoto, H., and Kanaya, Y.: Near-ground ozone source attributions and outflow in central eastern China during MTX2006, Atmos. Chem. Phys., 8, 7335–7351, https://doi.org/10.5194/acp-8-7335-2008, 2008.
Li, J., Yang, W., Wang, Z., Chen, H., Hu, B., Li, J., Sun, Y., Fu, P., and Zhang, Y.: Modeling study of surface ozone source-receptor relationships in east Asia, Atmos. Res., 167, 77–88, 2016.
Li, M., Zhang, Q., Kurokawa, J.-I., Woo, J.-H., He, K., Lu, Z., Ohara, T., Song, Y., Streets, D. G., Carmichael, G. R., Cheng, Y., Hong, C., Huo, H., Jiang, X., Kang, S., Liu, F., Su, H., and Zheng, B.: MIX: a mosaic Asian anthropogenic emission inventory under the international collaboration framework of the MICS-Asia and HTAP, Atmos. Chem. Phys., 17, 935–963, https://doi.org/10.5194/acp-17-935-2017, 2017.
Li, J.: Model outputs and observations: Model evaluation and intercomparison of surface-level ozone and relevant species in East Asia in the context of MICS-Asia Phase III, available at: https://figshare.com/s/cf48231dcd9529fc3bc6, last access: 8 October 2019.
Loon, M., Vautard, R., Schaap, M., Bergstr, M., R., Bessagnet, B., Brandt, J., Builtjes, P. J. H., Christensen, J. H., Curvelier, C., Graff, A., Jonson, J. E., Krol, M., Langner, J., Roberts, P., Rouil, L. M., Stern, R., Tarrason, L., Thunis, P., Vignati, E., White, L., and Wind, P.: Evaluation of long-term ozone simulations from seven regional air quality models and their ensemble, Atmos. Environ., 41, 2083–2097, 2007.
Lin, J. T. and Mcelroy, M. B.: Impacts of boundary layer mixing on pollutant vertical profiles in the lower troposphere: implications to satellite remote sensing, Atmos. Environ., 44, 1726–1739, 2010.
Liu, S. C., McKeen, S. A., Hsie, E-Y., Lin, X., Kelly, K. K., Bradshaw, J. D., Sandholm, S. T., Browell, E. V., Gregory, G. L., Sachse, G. W., Bandy, A. R., Thornton, D. C., Blake, D. R., Rowland, F. S., Newell, R., Heikes, B. G., Singh, H., and Talbot, R. W.: Model study of tropospheric trace species distributions during PEM-West A, J. Geophys. Res., 101, 2073–2085, 1996.
Liu, X. H., Zhang, Y., Xing, J., Zhang, Q., Wang, K., Streets, D. G., Jang, C., Wang, W. X., and Hao, J. M.: Understanding of regional air pollution over China using CMAQ, part II. Process analysis and sensitivity of ozone and particulate matter to precursor emissions, Atmos. Environ., 44, 3719–3727, 2010.
Lu, Z. and Streets, D. G.: Increase in NOx Emissions from Indian Thermal Power Plants during 1996-2010: Unit-Based Inventories and Multisatellite Observations, Environ. Sci. Technol., 46, 7463–7470, https://doi.org/10.1021/es300831w, 2012.
Lu, Z., Zhang, Q., and Streets, D. G.: Sulfur dioxide and primary carbonaceous aerosol emissions in China and India, 1996–2010, Atmos. Chem. Phys., 11, 9839–9864, https://doi.org/10.5194/acp-11-9839-2011, 2011.
Martin, R. V., Jacob, D. J., Logan, J. A., Bey, I., Yantosca, R. M., Staudt, A. C., Li, Q., Fiore, A. M., Duncan, B. N., and Liu, H.: Interpretation of TOMs observations of tropical tropospheric ozone with a global model and in situ observations, J. Geophys. Res.-Atmos, 107, ACH 4-1–ACH 4-27, 2002.
Nagashima, T., Ohara, T., Sudo, K., and Akimoto, H.: The relative importance of various source regions on East Asian surface ozone, Atmos. Chem. Phys., 10, 11305–11322, https://doi.org/10.5194/acp-10-11305-2010, 2010.
Nenes, A., Pandis, S. N., and Pilinis, C.: ISORROPIA: A new thermodynamic equilibrium model for multiphase multicomponent inorganic aerosols, Aquat. Geoch., 4, 123–152, 1998.
Olson, J., Prather, M., Berntsen, T., Carmichael, G., Chatfield, R., Connell, P., Derwent, R., Horowitz, L., Jin, S., Kanakidou, M., Kasibhatla, P., Kotamarthi, R., Kuhn, M., Law, K., Penner, J., Perliski, L., Sillman, S., Stordal, F., Thompson, A., and Wild, O.: Results from the intergovernmental panel on climatic change photochemical model intercomparison(PhotoComp), J. Geophys. Res.-Atmos., 102, 5979–5991, 1997.
Pan, X., Wang Z., Wang X., Dong, H., Xie, F., and Guo, Y.: An observation study of ozone dry deposition over grassland in the suburban area of Beijing, Chinese J. Atmos. Sci., 34, 120–130, 2010 (in Chinese).
Park, R. J., Hong, S. K., Kwon, H.-A., Kim, S., Guenther, A., Woo, J.-H., and Loughner, C. P.: An evaluation of ozone dry deposition simulations in East Asia, Atmos. Chem. Phys., 14, 7929–7940, https://doi.org/10.5194/acp-14-7929-2014, 2014.
Pleim, J. E., Xiu, A., Finkelstein, P. L., and Otte, T. L.: A Coupled Land-Surface and Dry Deposition Model and Comparison to Field Measurements of Surface Heat, Moisture, and Ozone Fluxes, Water Air Soil Poll., 1, 243–252, 2001.
Pleim, J. E.: A combined local and nonlocal closure model for the atmospheric boundary layer, Part I: Model description and testing, J. Appl. Meteor. Climatol., 46, 1383–1395, 2007.
Quan, J., Tie, X., Zhang, Q., Liu, Q., Li, X., Gao, Y., and Zhao, D.: Evolution of planetary boundary layer under different weather conditions, and its impact on aerosol concentrations, Particuology, 11, 34–40, 2013.
Rao, S. T., Galmarini, S., and Puckett, A. K.: Air quality model evaluation international initiative (AQMEII): advancing state-of-science in regional photochemical modeling and its applications, BAMS, 23–30, 2011.
Solazzo, E., Bianconi, R., Pirovano, G., Matthias, V., Vautard, R., Appel, K. W., Bessagnet, B., Brandt, J., Christensen, J. H., Chemel, C., Coll, I., Ferreira, J., Forkel, R., Francis, X. V., Grell, G., Grossi, P., Hansen, A., Miranda, A. I., Moran, M. D., Nopmongco, U., Parnk, M., Sartelet, K. N., Schaap, M., D. Silver, J., Sokhi, R. S., Vira, J., Werhahn, J., Wolke, R., Yarwood, G., Zhang, J., Rao, S. T., and Galmarin, S.: Model evaluation and ensemble modelling of surface-level ozone in Europe and north America in the context of AQMEII, Atmos. Environ., 53, 60–74, 2012.
Sorimachi, A, Sakamoto, K, Ishihara H, Fukuyama, T., Utiyama, M., Liu, H., Wang, W., Tang, D., Dong, X., and Quan, H.: Measurements of sulfur dioxide and ozone dry deposition over short vegetation in northern China-A preliminary study. Atmos. Environ., 37, 3157–3166, 2003.
Stockwell, W. R., Middleton, P., Chang, J. S., and Tang, X.: The second generation regional Acid Deposition Model chemical mechanism for regional air quality modeling, J. Geophys. Res., 95, 16343–16367, 1990.
Streets, D. G., Fu, J. S., Jang, C. J., Hao, J. M., He, K. B., Tang, X. Y., Zhang, Y. H., Wang, Z. F., Li, Z. P., Zhang, Q., Wang, L. T., Wang, B. Y., and Yu, C: Air quality during the 2008 Beijing Olympic games, Atmos. Environ., 41, 480–492, 2007.
Sudo, K., Takahashi, M., Kurokawa, J. I., and Akimoto, H.: Chaser: a global chemical model of the troposphere 1. model description. J. Geophys. Res.-Atmos., 107(D17), ACH 7-1–ACH 7-20., 2002a.
Sudo, K., Takahashi, M., and Akimoto, H.: CHASER: A global chemical model of the troposphere 2. Model results and evaluation, J. Geophys. Res., 107, 4586, https://doi.org/10.1029/2001/JD001114, 2002b.
Tang, X., Wang, Z., Zhu, J., Abaguidi, A., Wu, Q., Li, J., and Zhu, T.: Sensitivity of ozone to precursor emissions in urban Beijing with a Monte Carlo scheme, Atmos. Environ., 44, 3833–3842, 2010.
Tao, Z., Santanello, J. A., Chin, M., Zhou, S., Tan, Q., Kemp, E. M., and Peters-Lidard, C. D.: Effect of land cover on atmospheric processes and air quality over the continental United States – a NASA Unified WRF (NU-WRF) model study, Atmos. Chem. Phys., 13, 6207–6226, https://doi.org/10.5194/acp-13-6207-2013, 2013.
The Royal Society: Ground-level ozone in the 21st century: future trends, impacts and policy implications, Policy Document, 15/08, 2008.
Vogelezang, D. H. P. and Holtslag, A. A. M.: Evaluation and model impacts of alternative boundary-layer height for mulations, Bound.-Lay. Meteorol., 81, 245–269, https://doi.org/10.1007/BF02430331, 1996.
von Engeln, A. V. and Teixeira, J. A.: Planetary boundary layer height climatology derived from ECMWF reanalysis Data, J. Climate, 26, 6575–6590, 2013.
Walcek, C. J. and Aleksic, N. M.: A simple but accurate mass conservative peak-preserving, mixing ratio bounded advection algorithm with fortran code, Atmos. Environ., 32, 3863–3880, 1998
Walcek, C. J. and Teylor, G. R.: A theoretical method for computing vertical distributions of acidity and sulfate production within cumulus clouds, J. Atmos. Sci., 43, 339–355, 1986.
Wang, W. N., Cheng, T. H., Gu, X. F., Chen, H., Guo, H., Wang, Y., Bao, F. W., Shi, S. Y., Xu, B. R., Zuo, X., Meng, C., and Zhang, X. C.: Assessing spatial and temporal patterns of observed ground-level ozone in China, Sci. Rep., 7, 3651, https://doi.org/10.1038/s41598-017-03929-w, 2007.
Wang, Y. X., Shen, L. L., Wu, S., Mickley, L., He, J. W., and Hao, J.: Sensitivity of surface ozone over China to 2000–2050 global changes of climate and emissions, Atmos. Environ., 75, 374–382, 2013.
Wesely, M. L.: Parameterization of surface resistances to gaseous dry deposition in regional-scale numerical models, Atmos. Environ., 23, 1293–1304, 1989.
World Health Organization (WHO): WHO air quality guidelines global update, report on a working group meeting, Born, Germany, 18–20 October 2005, Rep., 25 pp., Geneva, 2005.
Yamaji, K., Ohara, T., Uno, I., Tanimoto, H., Kurokawa, J. I., and Akimoto, H.: Analysis of the seasonal variation of ozone in the boundary layer in east Asia using the community multi-scale air quality model: what controls surface ozone levels over Japan?, Atmos. Environ., 40, 1856–1868, 2006.
Yamartino, R. J.: Nonnegative, conserved scalar transport using grid-cell-centered, spectrally constrained Blackman cubics for applications on a variable-thickness mesh, Mon. Weather Rev., 121, 753–763, 1993.
Yarwood, G., Rao, S., Yocke, M., and Whitten, G.: Updates to the Carbon Bond Chemical Mechanism: CB05 Final Report to the US EPA, RT-0400675, 2005.
Zaveri, R. A. and Peters, L. K.: A new lumped structure photochemical mechanism for large-scale applications, J. Geophys. Res., 104, 30387–30415, 1999.
Zhang, J. and Trivikrama, Rao, S.: The role of vertical mixing in the temporal evolution of ground-level ozone concentrations, J. Appl. Meteorol., 38, 1674–1691, 1998.
Zhang, Y. H., Hu, M., Zhong, L. J., Wiedensohler, A., Liu, S. C., Andreae, M. O., Wang, W., and Fan, S. J.: Regional Integrated Experiments on Air Quality over Pearl River Delta 2004 (PRIDE-PRD2004): Overview, Atmos. Environ., 42, 6157–6173, 2008.
Zhong, L., Louie, P. K., Zheng, J., Wai, K. M., Ho, J. W. K., Yuan, Z., Lau A. K. H., Yue, D. L., and Zhou Y.: The pearl river delta regional air quality monitoring network – regional collaborative efforts on joint air quality management, Aero. Air Qual. Res., 13, 1582–1597, 2013.
- Abstract
- Introduction
- Models and data
- Model validation and general statistics
- Multi-model ensemble O3 and comparison with MICS-Asia II
- Discussions
- Summary
- Data availability
- Appendix A: Statistical measures
- Author contributions
- Competing interests
- Special issue statement
- Acknowledgements
- Financial support
- Review statement
- References
- Supplement
- Abstract
- Introduction
- Models and data
- Model validation and general statistics
- Multi-model ensemble O3 and comparison with MICS-Asia II
- Discussions
- Summary
- Data availability
- Appendix A: Statistical measures
- Author contributions
- Competing interests
- Special issue statement
- Acknowledgements
- Financial support
- Review statement
- References
- Supplement