- 1MARE—Marine and Environmental Sciences Centre, University of Coimbra, Coimbra, Portugal
- 2Department of Civil Engineering, Faculty of Sciences and Technology, University of Coimbra, Coimbra, Portugal
Rice cultivation is one of the largest users of the world’s freshwater resources. The contribution of remote sensing observations for identifying the conditions under which rice is cultivated, particularly throughout the growing season, can be instrumental for water, and crop management. Data from different remote sensing platforms are being used in agriculture, namely to detecting anomalies in crops. This is attempted by calculating vegetation indices (VI) that are based on different vegetation reflectance bands, especially those that rely on the Red, Green, and near-infrared bands, such as the Normalised Difference Vegetation Index (NDVI) or the Green Normalised Difference Vegetation Index (GNDVI). However, particular features of different crops and growing conditions justify that some indices are more adequate than others on a case-to-case basis, according to the different vegetation’s spectral signatures. In recent years, a vegetation index related to the Red Edge reflectance band, the Normalised Difference Red Edge (NDRE) has shown potential to be used as a tool to support agricultural management practices; this edge band, by taking a transition position, is very sensitive to changes in vegetation properties. This work, focusing on the rice crop and the application of different irrigation practices, explores the capability of several VIs calculated from different reflectance bands to detect variability, at the plot scale, in rice cultivation in the Lower Mondego region (Portugal). The remote sensing data were obtained from satellite Sentinel-2A imagery and using a multispectral camera mounted on an Unmanned Aerial System (UAS). By comparing several vegetation indices, we found that NDRE is particularly useful for identifying non-homogeneities in irrigation and crop growth in rice fields. Since few satellite sensors are sensible in the Red Edge band and none has the spatial resolution offered by UAS, this study explores the potential of UAS to be used as a useful support information tool in rice farming and precision agriculture, regarding irrigation, and agronomic management.
Introduction
Rice is an important cereal crop that plays a critical role in global food security and sustainable development, feeding more people in the world than any other crop (e.g., Cantrell and Reeves, 2002; Yu et al., 2002; Nguyen and Ferrero, 2006; Normile, 2008). However, since food security problems persist in many areas of the world, robust, and reliable tools that can be used to improve water and crop management and also for mapping and the early forecasting of rice yields are very important.
The contribution of remote sensing (RS) observations in identifying the conditions under which rice is cultivated, particularly throughout the growing season, can be instrumental in water, and crop management. Images obtained from satellites and Unmanned Aerial Systems (UAS) are offering attractive routes to acquire field data of important temporal and spatial resolutions in a fast and easy way (e.g., Manfreda et al., 2018; Tmušić et al., 2020) that could routinely assist to support better informed agronomic management decisions. Moreover, reliable and timely estimates of rice crop production areas and yields are essential for providing information for planners and decision makers to formulate policies in the case of shortfall or surplus (e.g., Mosleh et al., 2015). However, whereas RS has the potential to contribute overall to these goals in a variety of forms (we recall all advances in this research area and the applications in agriculture that have boosted in recent years), at present, the understanding of the potential of such contributions to rice cropping lags behind other crops.
Nevertheless, in recent years several studies already started to show the potential of RS in rice cultivation, namely using UAS (e.g., Duan et al., 2019; Wu et al., 2019; Hama et al., 2020; Zha et al., 2020). This opportunity can be associated to the higher resolution of the data provided by UAS platforms in relation to satellites, which allows for more detailed surveys at the rice field plot scale; this scale is particularly relevant for rice cultivation monitoring and management. In addition, it is yet not clear which RS based indicators could better depict the spectral signature of the rice cultivation conditions, namely throughout the rice growing season and for different irrigation, and agronomic management practices. The need to guarantee worldwide the sustainability of rice production makes these issues important research topics of practical environmental and socio-economic interest.
The main objective of this study is thus to explore the application of available RS tools to assess specific rice farming conditions (aiming at improving crop and water management through the better understanding of the rice cropping system and assessment of the crop status) and, ultimately, the calculation of rice production risk. In particular, we search for the most adequate Vegetation Indices (VIs) to be applied in the rice growing region of the Lower Mondego Valley (Portugal), based on available data from satellite imagery and UAS field surveys.
Remote Sensing Products and Precision Agriculture
Precision agriculture is designed to target crop inputs according to within-field requirements to increase profitability while protecting the environment. Whereas precision rice crop management is crucial to meet the double challenge of sustainable development and food security (Zhao et al., 2013), it requires data of adequate temporal and spatial resolutions and coverage.
For already some time, the attributes of RS data and the suite of tools offered by this technology have triggered the search for attractive non-destructive approaches to obtain important data in agriculture. This led to using innovative RS technologies and products (e.g., Xiao et al., 2005; Zhang et al., 2016; Kawamura et al., 2020), but further research is needed to understand the usefulness and applicability of some of those products to rice production. In fact, the majority of available studies using satellite images to monitor rice growth (e.g., Kuroso et al., 1997; Le Toan et al., 1997; Panigrahy and Sharma, 1997; Shao et al., 1997; Oette et al., 2001; Shao et al., 2001; Dawe et al., 2004; Motohka et al., 2009; Mosleh et al., 2015) had global concerns and used moderate image resolution. Satellite RS provides both cost-effective and multispectral and multi-temporal data, but data resolutions are still too coarse to address plot (small) scale conditions. Thus, although satellite data have witnessed increases in spatial resolution, a process that has been developing fast, data resolution does not yet satisfy copiously the needs of precision agriculture.
On the other hand, UAS constitute a useful solution to obtain higher resolution RS data. These aerial systems have the potential to fill the scale gap between space borne observations and detailed field observations, and are contributing to increase our knowledge about e.g., surface processes and land cover dynamics (e.g., González-Jorge et al., 2017; Tatum and Liu, 2017; Manfreda et al., 2018; de Jong, 2020; Tmušić et al., 2020).
In recent decades, advances in UAS have made this technology a promising tool that involves relatively smaller investments (Manfreda et al., 2018; Yang et al., 2021). Also, continued improvements of aerial vehicles and of the imaging and sensing equipment mounted on these platforms classify UAS as alternative environmental monitoring platforms. This technology provides an opportunity to capture spectral signals at spatial and temporal scales of interest across a range of applications. Whereas satellite RS is mostly useful for large geographical scales monitoring, UAS offer advanced crop image data analytics at high spatial and temporal resolution and crop monitoring in near real-time, which are important elements in agriculture and water management (Nhamo et al., 2020). We stress, however, that since studies reported by different authors report results that, in general, were obtained using different sensors at different levels, ground truth measurements have a complementary and essential role for validation and comparability purposes.
Different vegetation reflectance bands’ data obtained from different RS platforms are used to calculate VIs, especially those that rely on the Red, Green, and near-infrared (NIR) wavelength bands. The calculation of most VIs is based on two of the most stable sections of the plants’ spectral reflectance curve, thus involving different spectral ranges of RS data. The effectiveness of VIs in assessing different attributes of the plants at the image’s pixel scale is determined by the characteristics of reflectance. Vegetation reflectance is low in both the blue and red regions of the visible spectrum, it peaks locally in the green region, and it is highest in the NIR range. Thus, by algebraically combining these bands, VIs allow that different spectral signatures are enhanced for different vegetation properties.
Overall, VIs are related to several physical attributes of vegetation, e.g., plant height, leaf area, leaf greenness, canopy greenness, aboveground biomass, percent cover, photosynthetic activity, evapotranspiration. The advantage of using VIs, in comparison to using single spectral bands, is reducing the spectral data to a single number that is related to those physical characteristics of vegetation while minimising the effect of internal (e.g., canopy geometry, and leaf and soil properties) and external (e.g., sun-target-sensor angles, and atmospheric conditions at the time of image acquisition) factors on the spectral data. In this way, VIs assist to predict crop yields and monitor crop disease development and water stress (Tilly et al., 2014; Stroppiana et al., 2015; Hoffmann et al., 2016; Cen et al., 2019; Duan et al., 2019; Maes and Steppe, 2019; Poley and McDermid, 2020; Zhou et al., 2021), having therefore the potential to be incorporated in risk assessment and the definition of management strategies and policies, and income compensation (Kasampalis et al., 2018; Huang et al., 2019; Shiu and Chuang, 2019).
During drought events, vegetation canopies can be affected by water stress. This can have major impact on the plant development in general and can cause crop failure or lower crop production in agricultural areas. Early recognition of plant water stress can be critical to prevent such consequences. By providing RS near-real time information on the plant water stress to stakeholders, water and agricultural management can be much improved, notably by specifically irrigating areas where plant water needs are not completely fulfilled anymore (e.g., Norasma et al., 2018; Wan et al., 2020; Yang et al., 2020; Cao et al., 2021).
However, using any vegetation index requires careful attention in identifying its strengths and weaknesses in relation to a specific analysis goal, thus, its adequacy for different applications (e.g., Akbarian et al., 2020). Critical issues that are not yet completely solved include the selection of the most appropriate temporal, spatial, and spectral resolutions of the data. Spectral resolution describes the ability of a sensor to define fine wavelength intervals: the finer the spectral resolution, the narrower the wavelength range for a particular channel or band.
Among the many VIs calculated from multispectral RS data that are often used in different applications (for a review see e.g., Xue and Su, 2017), we highlight the Normalized Difference Vegetation Index (NDVI), the Green Normalized Difference Vegetation Index (GNDVI), the Normalized Difference Red Edge index (NDRE), and the Normalized Difference Water Index (NDWI). These normalized indices, which will be used in this study, take values between −1.0 and +1.0. They are defined in Table 1 and revisited in Revisiting the Remote Sensing Based Indicators. For rice cultivation conditions, the usefulness of these and other RS based indices needs better insight.
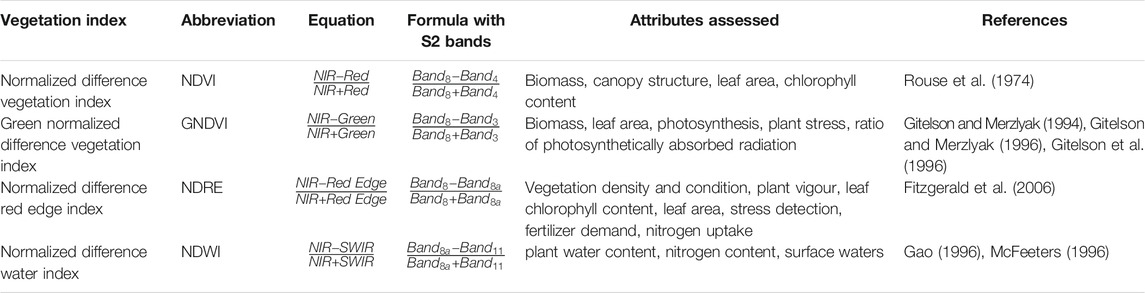
TABLE 1. Spectral indices calculated in this study. For Sentinel-2 (S2), multispectral wavelength band centres are: Band 3 (560 nm), Band 4 (665 nm), Band 8 (842 nm), Band 8a (865 nm), and Band 11 (1,610 nm).
Materials and Methods
Description of the Study Area
Similar to other main European rice producer countries, such as Italy and Spain, Portugal is concerned with rice cultivation (global) sustainability. Here, attention is devoted to rice farming in the Lower Mondego Valley (Portugal), which is found mostly in the lowlands of this valley where soils have alluvial origin and high agricultural value. Rice cultivation has long been a tradition in this area and generates significant local rural employment and direct and indirect economic activity (e.g., rice industry, machinery, agrochemicals). There is also a cultural value associated with the tradition of rice production in this region, which influences other activities of a social and gastronomic nature.
It is anticipated that the current situation, that faces a number of problems, is aggravated by the consequences of the increasing pressure on water resources, and the expected changes in the climate, in particular increased climate variability. Research in progress explores alternative irrigation and agronomic practices adapted to different local conditions that might lead to less irrigation water applications, keeping crop productivity high and environmental impacts low; for this purpose, it is important to increase the knowledge about the water dynamics of rice growing.
Traditional rice irrigation is by continuous flooding. This practice is repeatedly identified as contributing largely to low agricultural water use efficiency, an issue that is often studied (e.g., Howell, 2001; Gonçalves and de Lima, 2018) and discussed in water users and managers’ forums. In the Lower Mondego area, local farmers have empirically introduced alternative irrigation practices, alternating between wet/flooded and dry conditions of the rice fields. However, this irrigation practice is adjusted to the particular conditions of the different plots; the length of the periods when the rice plot is flooded or dry is decided upon the farmers’ assessment of the actual conditions of the field crop. At present, the local irrigation practice often applies a type of Alternate Wet and Dry (AWD) irrigation, which aims at water saving, but it is not based on any specific irrigation scheme. This study aims at clarifying the usefulness of different RS tools for assessing field conditions during the rice cultivation season and assisting in water management practices decisions that could improve rice farming in the area. For this purpose, several RS based indices are explored and discussed.
The selected study area (Figure 1A), of approximately 335 ha, is an irrigation unit (Bloco 1—Quinta do Canal, coordinates: 40° 7′ 0.16″ N, 8° 47′ 47.34″ W) of the Lower Mondego Irrigation District (LMID) that is predominantly dedicated to rice cultivation (about 332 ha). Quinta do Canal is located in the left bank of the Mondego River, in the downstream part of the Lower Mondego Valley. The area is bounded to the north by the Mondego River, to the south by the Pranto River and to the west by the Mondego River estuary. Quinta do Canal is amongst the most downstream irrigated lands of the LMID and it integrates 71 rice field plots. Ten of these plots were selected to be studied using RS products from satellite and UAS; their area ranges between 1.6 and 5.7 ha (Figure 1A). The rice produced has long grain, of the variety Ariete, sub-species Oryza sativa L. ssp. japonica; commercially, it is known as “Carolino” rice.
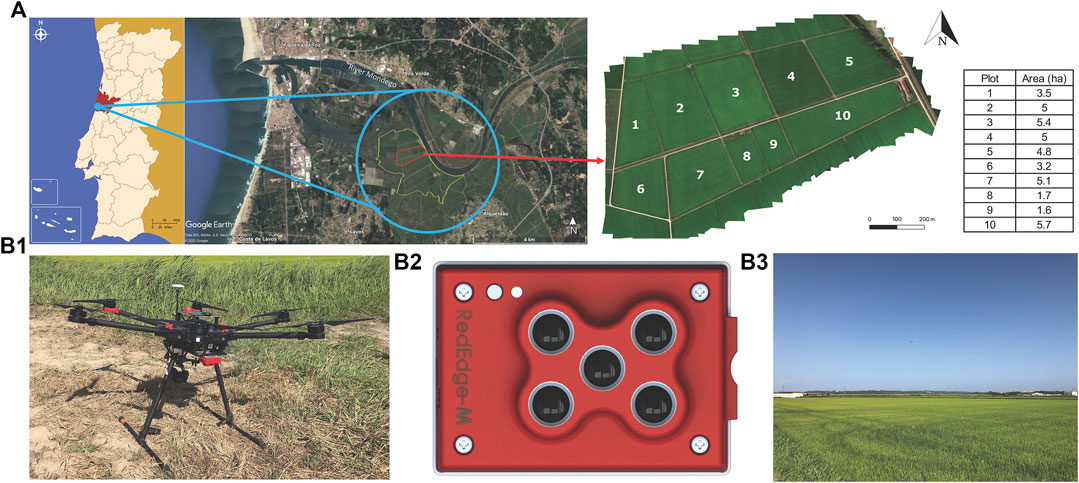
FIGURE 1. Study site and UAS survey. (A) Location of the rice production irrigation area “Quinta do Canal,” in Portugal (adapted from Google Maps, 2021) and limits of the ten selected study field plots and (B) Views of the UAS field survey in Quinta do Canal (July 9, 2020): (b1) DJI Matrice 600 drone, (b2) Micasense RED Edge—M multispectral sensor, and (b3) rice fields.
Remote Sensing Data Acquisition and Processing
Data from satellite Sentinel-2 and data obtained through dedicated UAS flights undertaken in 2020, during the rice crop cultivation season (from early May to October), were explored.
Available satellite Sentinel-2 images were downloaded from the Copernicus open-access website (https://scihub.copernicus.eu) for the period from January 1 to October 31, 2020. Level-2A images, already atmospheric corrected, were selected. Only images showing a cloud cover lower than 8% were retained. The satellite data that supported the estimation of VIs were available at 10 m full spatial resolution, although the data used to calculate NDWI were only available at 20 m spatial resolution. The corresponding multispectral wavelengths bands used to calculate the vegetation and water indices are given in Table 1 and are centred as follows: Band 3 (560 nm), Band 4 (665 nm), Band 8 (842 nm), Band 8a (865 nm), and Band 11 (1,610 nm).
The UAS data were collected during a flight conducted between 2:30 pm and 4:00 pm on July 9, 2020, under conditions of no wind and clear sky (Figure 1b3). A DJI Matrice 600 drone (Figure 1b1) equipped with a camera X5 for RGB and a Micasense RED Edge—M multispectral sensor was used (Figure 1b2), which collected images having a spatial resolution of 0.074 × 0.074 m2; relevant spectral wavelength bands (centre) are Blue (475 nm), Green (560 nm), Red (668 nm), Red Edge (717 nm), and NIR (840 nm). Ground sample distance was 7.21 cm/pixel. The radiometric correction processing option was Camera, Sun Irradiance and Sun Angle using DLS IMU.
The UAS was autonomous and was operated respecting a pre-set flight plan corresponding to a simple grid with a distance between flight lines of 25 m. There was 75% overlap and 70% sidelap; the flight height above the ground was 100 m. The speed of the DJI Matrice 600 drone was set to 7.5 m/s. A quick calibration was performed prior to the flight using a calibration target.
The UAS flight images’ data, including orthorectification, atmospheric correction and elaboration of VIs maps (section 3.3.), were processed using the Pix4D software (Pix4D S.A., Prilly, Switzerland).
Computation of Vegetation and Water Indices
Rice plants have specific land cover properties. In addition to the fact that rice land coverage changes during the rice crop life cycle, in irrigated rice fields the land coverage dominated by water also changes, which might depend on the crop growth stage and the irrigation practice.
During the rice crop season, rice vegetation coverage grows and reaches a maximum (at rice age of approximately 2 months) and then gradually decreases until harvest time. The traditional irrigation is by continuous flooding of the rice fields, although AWD irrigation is applied at many locations, mostly aiming at water saving. The fields are flooded at the time of sowing; the flooding is interrupted 2–3 weeks before harvest.
In this study, the vegetation and water indices computed for the rice crop were NDVI, GNDVI, NDRE, and NDWI. Their descriptions can be found in Revisiting the Remote Sensing Based Indicators. Depending on data availability, the indices were calculated from satellite Sentinel-2A, and UAS data. Basic descriptive statistics were obtained for the collected data, extracted using the zonal statistics plugin in Quantum GIS (QGIS) software.
Revisiting the Remote Sensing Based Indicators
References and the calculation equations for NDVI, GNDVI, NDRE, and NDWI, which are the indices used in this exploratory work, are given in Table 1. Main features of these indices are revisited below.
The NDVI is one of the most widely used vegetation indices in agriculture, in particular to detect live green plant canopies, thus, displaying greenness. This index takes advantage of characteristics of two spectral bands: the chlorophyll pigment absorptions in the Red spectral band (0.62–0.69 μm) and the high reflectance of plant materials in the NIR band (0.75–1.3 μm). It is calculated as the normalized ratio between the Red and the NIR wavelength bands. Since high photosynthetic activity leads to lower values of reflectance coefficients in the red region of the spectrum and large values in the NIR region of the spectrum, the ratio between these indicators allows to clearly separate the vegetation from other natural objects. Values between −1 and 0 indicate dead plants, or inorganic objects such as stones, roads, and houses. For live plants, NDVI values range between 0 and 1, with 1 being the healthiest and 0 being the least healthy.
Thus, NDVI is directly related to the plants’ photosynthetic capacity and hence energy absorption of plant canopies. As a result of the spectral analysis, this index has the potential to assess canopy growth or vigour, the density of vegetation, and to identify areas that need reseeding, application of plant protection products or fertilizers (e.g., Karnieli et al., 2010; Xue and Su, 2017); it can allow farmers to assess crop germination and growth, the presence of weeds or diseases, and to predict the productivity of the agricultural fields. One limitation in this index is that it shows low sensitivity to detect minor differences in high chlorophyll content and biomass, which is known as the “saturation effect” (e.g., Gao, 1996; Schaefer and Lamb, 2016); saturation occurs when NDVI is applied to images over areas having dense vegetation, i.e., when the level of the Leaf Area Index becomes high (i.e., LAI ≥ 3, see Gao, 1996). Consequently, NDVI is not always the most accurate index to detect anomalies in crops, particularly at small scales (Jorge et al., 2019). The index is moderately sensitive to changes in soil and atmospheric background, except in cases of poor vegetation.
The GNDVI is similar to NDVI except that instead of the red spectrum it assesses the green spectrum in the range 0.54–0.57 μm; it is derived from the Green and NIR spectral bands. GNDVI allows also to estimate photosynthetic activity of the vegetation cover; in fact, this index has been found to be more sensitive to chlorophyll content than NDVI (Gitelson and Merzlyak, 1998). GNDVI is also used to determine moisture content and nitrogen concentration in plant leaves and canopy according to multispectral data that do not have an extreme red channel, and for assessing depressed and aged vegetation.
A somewhat less commonly used index is the NDRE, which is based on differences in reflectance between the Red Edge (RE) and NIR wavelengths. RE wavelength extends across the band 0.68–0.75 μm (Horler et al., 1983), therefore between the visible Red and NIR electromagnetic spectra (Cui and Rekes, 2018). This region of the spectra depicts the limit between absorption by chlorophyll in the Red band, and scattering due to leaf internal structure in the NIR band (e.g., Jorge et al., 2019). Thus, the Red and RE wavelengths are strongly absorbed by the chlorophyll pigments, while the NIR reflectance is highly influenced by the leaf structure (e.g., Kanke et al., 2016).
Thus, the NDRE is offering a new alternative for estimating photosynthetic activity, with the RE band being very sensitive to changes in foliar chlorophyll content, which is strongly related to plant nitrogen concentration; it allows thus to quantify vegetation density and conditions (e.g., Broge and Leblanc, 2001). It is especially useful for estimating crop health in the mid to late stages of growth, when the chlorophyll concentration is relatively higher. In recent years, NDRE has shown potential to be used as a tool to support agricultural management practices since this edge band, by taking a transition position, is very sensitive to changes in vegetative properties: e.g., 1) it can be used to map the within-field variability of nitrogen foliage to understand the fertilizer requirements of crops and 2) it has been found more advantageous than the NDVI to optimising harvest times based on transitions of photosynthesis activity (Maccioni et al., 2001).
In the last few years, many new sensors have become available that are capable of detecting radiation in the red-NIR transition zone, or RE band; note that the limits of the RE band might depend on the sensor. Unfortunately, only a limited number of remote-sensing satellites are equipped with this type of sensors and in some cases their spatial resolution is modest (e.g., Sentinel-2 satellite, with a spatial resolution of 20 m). However, most commercial multispectral cameras that are installed in UAS detect RE radiation, providing detailed data that are relevant for small spatial scale studies.
Finally, the NDWI is a satellite-derived index that is used to delineate open water features and enhance their presence in remotely-sensed digital imagery: NDWI varies almost linearly with liquid water thickness (Gao, 1996). Moreover, NDWI is known to be also strongly related to plant water content. NDWI is computed using the NIR and the short wave infrared (SWIR) reflectance using MODIS data or using Green and NIR reflectance using LandSat-8 or Sentinel 2 data (Table 1), which makes it sensitive to changes in liquid water content and the spongy mesophyll of vegetation canopies (Gao, 1996; Ceccato et al., 2001). It is therefore a very good proxy for plant water stress and changes in surface water, and it is useful for drought monitoring and early warning (e.g., Ceccato et al., 2002; Gu et al., 2007). High/low values of NDWI correspond to high/low vegetation water content and to high/low vegetation fraction cover. In periods of water stress, the NDWI decreases It is possible to infer Equivalent Water Thickness (EWT) from NDWI over areas fully covered by green vegetation (Gao, 1996); however, it is difficult to do so from NDWI over partially vegetated areas, because soil contributions to NDWI are mostly negative, whereas green vegetation contributions are positive. Thus, NDWI is complementary to, not a substitute for other VIs.
Results and Discussion
Usually, the rice cropping season in Quinta do Canal (Portugal) runs from May to October, when harvest takes place, with the growing peak occurring between July and August. For the 2020 rice growing season, the temporal variation of the NDVI average values was estimated from Sentinel-2A data (Figure 2) for the 10 selected rice fields in Figure 1. Results reveal the limited number of images that are usually available during the approximate 120-days rice growth period but, overall, the satellite data are enough to perceive well the crop life cycle.
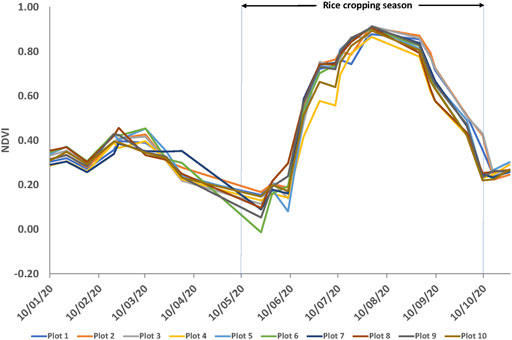
FIGURE 2. NDVI average values calculated for the selected irrigated rice field plots (Quinta do Canal, Figure 1) using satellite Sentinel-2A imagery available for 2020, at 10 m spatial resolution. The period plotted include the rice cropping season, which lasted 151 days in 2020: sowing date was May 10 and harvest date was October 10.
As expected, the 10 NDVI profiles reflect the different conditions, over time, of the field plots, and the different rice cropping phases: initial growth, crop development, reproduction and maturity phases. NDVI values closer to +1 indicate vegetation with high photosynthetic activity, under the premises that the photosynthetic process is carried out mainly by plant leaves and that solar radiation in the visible region (0.40–0.72 μm) is mostly absorbed by photosynthetic pigments; thus, NDVI values in this range indicate denser vegetation cover. In contrast, lower values of NDVI values indicate the conditions of lower vegetation cover.
In Figure 2, the lowest NDVI values, around zero (−0.1 to 0.1), are observed in the initial phase of rice crop cultivation; they are related to the flooding of the fields close to the crop (wet) sowing time. The highest NDVI values, of approximately 0.90, are reached in August, and reflect the peak greenness of rice attained during the heading stage. Towards the end of the cultivation period, the NDVI values decrease until the plant reaches full maturation and is harvested, in the beginning of October. In irrigated rice fields, especially in early periods, water environment is expected to play an important role in the rice spectral signal.
The differences between the field plots (Figure 2) are explained by differences in field conditions and crop responses to the applied water and agronomic practices. A somewhat larger discrepancy in NDVI was found for plots 4 and 10, around the date when the UAS flight took place (i.e., July 9, 2020). This led us to further investigate the conditions in these plots, at that time.
In view of the issues mentioned above, and since the irrigation scheme and timing differ among the selected rice field plots, the NDWI derived from satellite Sentinel-2A data was used complementary to NDVI to assist in assessing the water conditions/status prevailing in the rice fields at the time of the UAS survey (Figure 1b3). Satellite data were available for July 8, 2020, the day before the UAS survey took place.
The NDVI and NDWI maps are shown in Figure 3, at 20 m spatial resolution. The NDVI scale applies a red-yellow-green palette to NDVI-processed imagery, which is generally seen as more intuitive: green signals healthy vegetation and red highlighting hotspots of concern, or areas lacking vegetation. In the NDWI map, the NDWI values over areas with dead grass are negative, while those over green vegetation areas are positive; high NDWI values (in blue) correspond to high vegetation water content and to high vegetation fraction cover, whereas low NDWI values (in red) correspond to low vegetation water content and low vegetation fraction cover. For both indices, NDVI, and NDWI, the field plots’ average indices and coefficients of variation are shown in Figure 4.
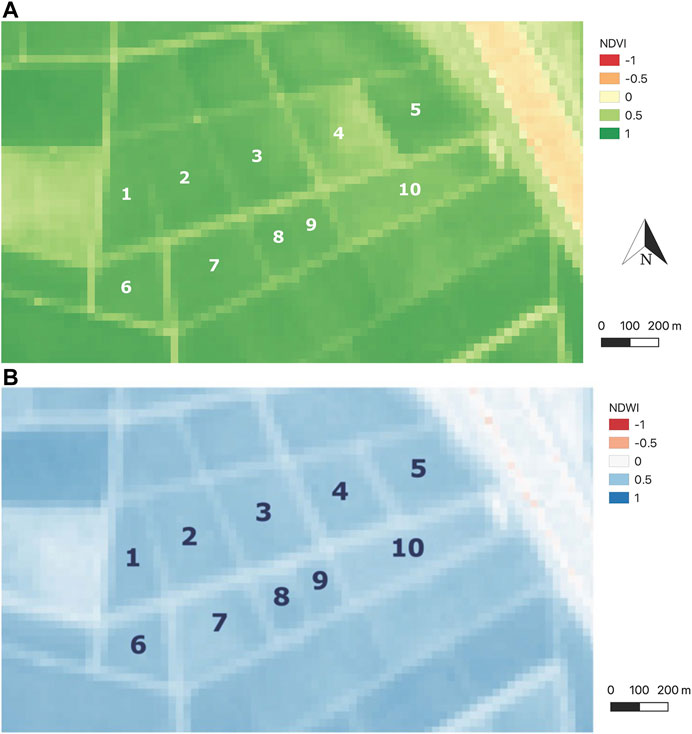
FIGURE 3. Maps of the (A) NDVI and (B) NDWI for the selected rice fields in Quinta do Canal, on July 8, 2020. Data are from Sentinel-2A, at 20 m spatial resolution.
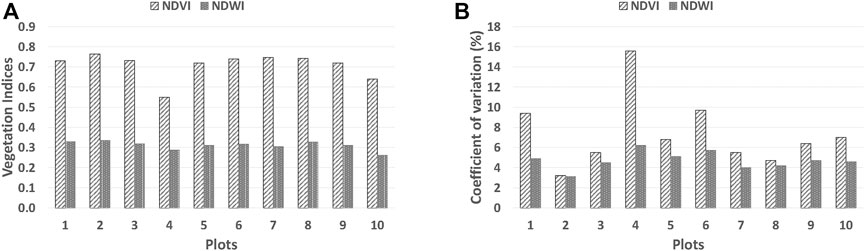
FIGURE 4. Vegetation indices NDVI and NDWI for the selected rice field plots, in Quinta do Canal, on July 8, 2020: (A) average values, and (B) coefficient of variation. Data are from Sentinel-2A, at 20 m resolution.
Field plots 4 and 10 exhibit the smallest NDVI and NDWI (Figures 3, 4), which is in agreement with the fact that these plots were only recently flooded (i.e., the day before) when the UAS data were collected; therefore, it is expected that the thickness of the water layer over the soil is smaller for these two plots and that this reflects in the index value. The situation for NDVI has been already identified above. Complementary, NDWI reveals spatial non-uniformity in water conditions between the 10 selected plots and at the plot scale (i.e., within each plot), which confirms that NDWI is a good indicator for vegetation liquid water content.
Results confirm that contrary to NDWI, NDVI has limited capability for retrieving vegetation water content information. NDVI provides information on vegetation greenness (chlorophyll), which is not directly and uniformly related to the quantity of water in the vegetation (Ceccato et al., 2002). On the other hand, NDWI varies almost linearly with liquid water thickness and it also strongly relates to plant water content, which could be important to assess the water conditions in rice fields. Gu et al. (2007) found that NDWI values exhibited a quicker response to drought conditions than NDVI, a study that led to the testing of NDWI as a drought indicator (Gu et al., 2008). According to Gao (1996) this index is less sensitive to atmospheric scattering effects than NDVI.
However, in general, drought and water stress are not the only factors that can cause a decrease of NDWI values/anomalies. Changes in land cover or pests and diseases can also be responsible for such variation in the signal. Therefore, this indicator must be used jointly with other indicators that give information on the deficit of rainfall/soil moisture in order to determine if the variation in the vegetation response (signal) is associated with a drought event or not.
For the selected study plots, maps of NDVI (Figure 5A), GNDVI (Figure 5B), and NDRE (Figure 5C) have been created from the data collected using UAS during the last period of the rice vegetative phase, on July 9, 2020. For all indices, the red, green, and yellow colours in the images indicate, respectively, the lowest, the highest, and intermediate biomass production. Since the spectral classes used in the maps are the same, comparison of the maps permits to appreciate that there are indeed differences in the way the spectral signature of the rice fields is captured by each of the indices. In particular, differences in the visual assessment of the spatial variability within each field are notorious, in addition to the differences observed between neighbouring rice fields.
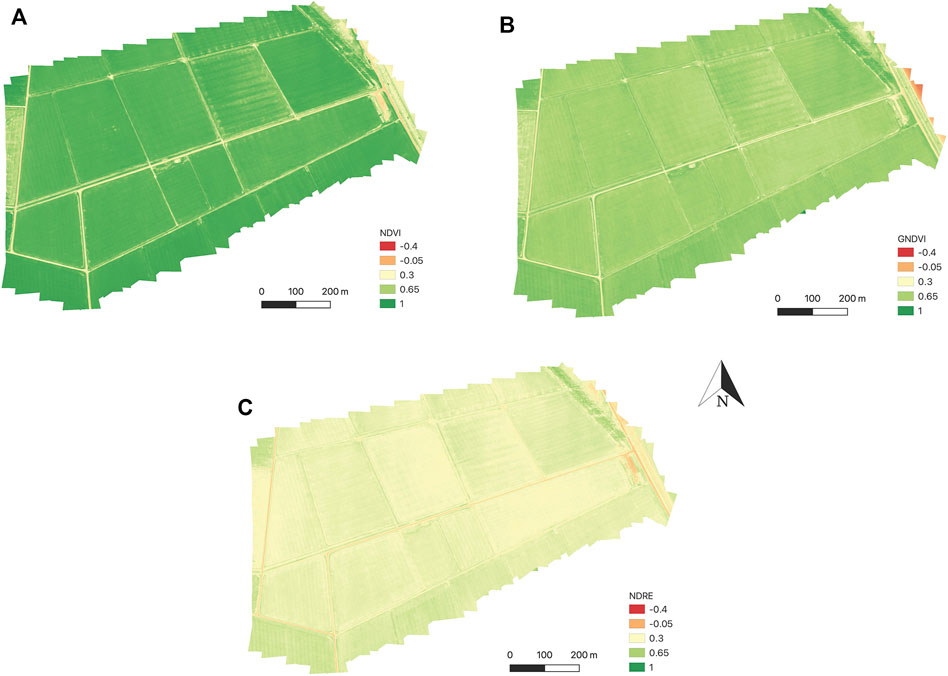
FIGURE 5. Maps of (A) NDVI, (B) GNDVI, and (C) NDRE, for the selected rice plots in Quinta do Canal (July 9, 2020). The UAS field images have a spatial resolution of 0.074 × 0.074 m2.
For better insight, Figure 6 shows the NDVI, GNDVI, and NDRE average values and coefficients of variation obtained from the data collected during the UAS campaign. Overall, although apparently there is no much difference in the average value of the indices for the different plots, there are consistent relative differences exhibited by the different VIs at the plot scale, for all 10 rice plots. The NDVI average values ranged from 0.83 to 0.89: the minimum average value was reached in plot 4 and the maximum value in plot 5. The GNDVI average values ranged from 0.61 to 0.70: the minimum average value is for plot 10 and the maximum value for plot 5. The NDRE average value ranged from 0.32 to 0.41, also with the minimum average value reached in plot 10 and the maximum average value reached in plot 5; the second lowest NDRE value is for plot 3. The NDVI is higher than the remaining VIs at the time of the UAS data collection; this is explained by the fact that the rice crop is richer in chlorophyll content during its latest growing stages, which is the plant attribute more strongly captured by this VI.
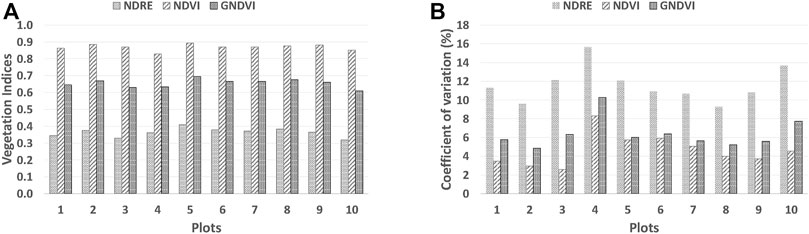
FIGURE 6. Vegetation indices NDVI, GNDVI, and NDRE, for the selected rice fields (Quinta do Canal): (A) average values, and (B) coefficient of variation. The data are for July 9, 2020, from UAS images, at 0.074 m resolution.
On the other hand, the coefficients of variation (Figure 6B) were higher for the NDRE index than for the NDVI and GNDVI indices. This suggests that the NDRE index may be more responsive to variations in crop vigour than the other indices and could present greater sensitivity in detecting differences in chlorophyll content, a pigment that is closely linked to plant vigour (de la Casa et al., 2018). This is expected to result from the conditions of the rice fields, since chlorophyll content might have reached the point at which NDVI “saturates” (Revisiting the Remote Sensing Based Indicators). Under this condition, which is usually found at the stage of the rice growing season, and captured by the data in this particular case, variability in the rice crop at the plot scale is hard to detect with NDVI and GNDVI; in particular, this can be appreciated in Figure 5A. Nevertheless, results confirm that GNDVI presents greater sensitivity than NDVI (as suggested by the relatively higher coefficient of variation, Figure 6B), which can be explained by variations in the underlying vegetative surface, that is, the soil and the elements that constitute it, such as organic matter, texture, mineralogical composition, moisture, roughness (e.g., Ben-Dor et al., 1999). Thus, among the three indices, NDRE is likely the one that is able to identify better existing differences among and within crop fields. In contrast, the lower the value of the coefficient of variation, the more precise the estimate of the vegetation index is at the field scale.
Overall, results suggest that NDRE should be taken as a valid and alternative vegetation index (e.g., in relation to NDVI), and that it may constitute a useful (and more adequate) tool to inform farmers and support agronomic management decisions. This has already been discussed in previous studies, but results of this study show that it applies also to the rice growing conditions in the Lower Mondego Valley and, expectedly, also elsewhere, at least for similar rice growing conditions. Yuhao et al. (2020) studied multispectral images in the early season of rice growth, which were obtained using UAS technology, and correlated vegetation indices, including NDRE, to soil plant analysis development (SPAD) data chlorophyll meter readings to assess the potential of those indices for examining the amount of chlorophyll concentration present in rice.
The positive relationships between the different VIs obtained at the plot scale and for the conditions surveyed are shown in Figure 7. The lowest value (R = 0.56) of the Pearson correlation coefficient, R, was found between NDRE and NDVI (Figure 7B) and the highest (R = 0.95) was exhibited between NDRE and GNDVI (Figure 7C); the positive relationship that is studied more often, between the GNDVI and NDVI, exhibited a correlation coefficient of R = 0.76 (Figure 7A). The low NDVI value calculated for plot 4 might somehow influence these corresponding results. A crude appraisal of the strength of the correlations would classify them as strong for the first case (NDRE and NDVI) and very strong for the other cases (NDRE and GNDVI, and GNDVI and NDVI).
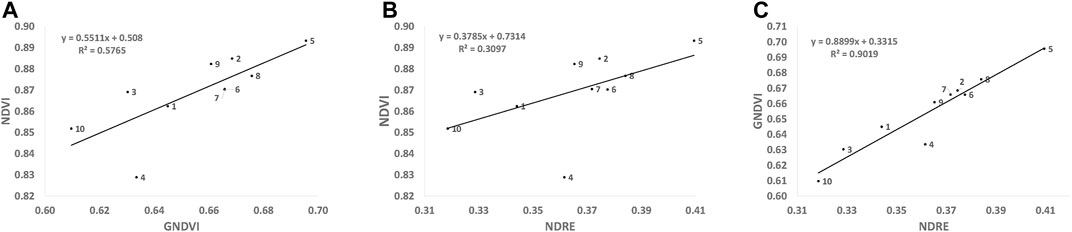
FIGURE 7. Relationship between the VIs for the study plots in Quinta do Canal: (A) GNDVI and NDVI, (B) NDRE and NDVI, and (C) NDRE and GNDVI. Data are for July 9, 2020, from UAS images. The relations obtained by linear regression and the corresponding coefficients of determination are given.
Note that rice plots 4 and 10 exhibit the lowest values of NDWI, with the lowest average values of all studied indices found for plot 10. It is thus clear that there is variability between the plots. It is expected that this variability is partially explained by the different stages of crop development in the plots and perhaps by different watering practices adapted to specific field conditions. These are nevertheless neighbouring plots and overall managed according to the same general judgment. It is also expected that within each plot, the spatial variability in cultivation conditions influences the output VI value for that plot. In this respect, it is remarkable that the NDRE, followed by GNDVI, performed better than NVDI for identifying that variability, as highlighted by the higher coefficient of variation of NDRE and GNDVI found for plots 4 and 10 (Figure 6B). These results catch the eye, whereas the NDVI results give no indication that these plots might present any particular striking difference, within the group of plots.
Thus, more data are needed to better understand the relations between the vegetation indices, and their signatures concerning rice cropping. Nevertheless, results suggest that NDRE index can indeed play an important role for identifying non-homogeneities in rice fields, and that analysis based on NDVI alone might not fulfil all requirements for assessing the status of this particular crop.
Finally, a word goes to the comparison of the NDVI values obtained from satellite Sentinel-2A (July 8) and UAS data (July 9) during the rice reproductive phase, for the study plots and the spatial resolution of, respectively, 10 m and 0.074 m. Results show that the NDVI average values estimated from UAS data are on average higher than the values estimated based on Sentinel-2A data. For the specific case reported in this work, the ratio between the satellite and the UAS based indices is on average 1.22: for the 10 plots, average NDVI is 0.87 ± 0.02 (UAS) and 0.71 ± 0.06 (Sentinel-2A). For illustration, the corresponding NDVI maps are shown for rice field plot 6 (Figure 8): average NDVI estimates are 0.87 ± 0.05 (6.26 × 106 pixels) from UAS data and 0.74 ± 0.07 (321 pixels) from Sentinel-2A data, for this plot. For the data analysed, it was also found that the satellite data identified a larger deviation in the conditions in plots 4 and 10 than the UAS data, which could nevertheless be biased by border effects.
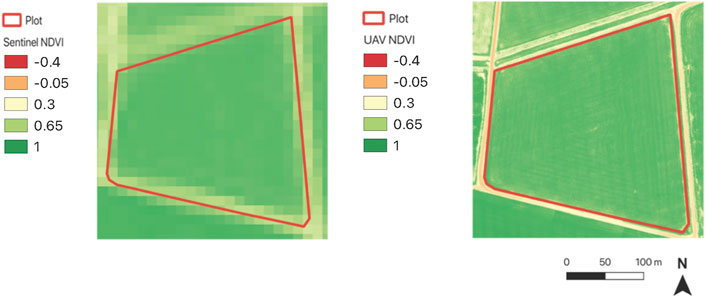
FIGURE 8. NDVI maps calculated for rice plot 6 (Quinta do Canal) using data from Sentinel-2A (left) and UAS (right) imagery. The data were obtained during the rice reproductive phase, respectively, on the 8th and 9th of July, 2020.
Between the two RS platforms (Sentinel-2A and UAS), differences in the NDVI estimates could be explained by using reflectance measurements that were not recorded exactly simultaneously, the fact that different instruments acquire different spectral channels through different cameras or focal planes (since each sensor has its own characteristics and performance, e.g., spectral resolution), and also by the different spatial resolution of the data. It is also to be expected that the NDVI estimates obtained at other times from data of different origins and other conditions (e.g., weather, rice growing stages, water stress) could be diverse between the platforms.
In particular, scale issues in RS (e.g., Wu, 2009; Lausch et al., 2013) require the collection of larger data sets and further investigation before such issues come to a closure. Whereas scale is a basic concept for describing the hierarchical structure of landscapes, structures, and processes, RS techniques involve different spatial and spectral resolutions. It is thus not surprising that results obtained using many common methods are doubtful or not adequately understood, as the applicability of such methods across different scales is not always obvious and sometimes questionable. On the one hand, each method has its specific problems, limitations, and applicability conditions and, on the other hand, the effects of different scales, methods of scaling, and scale transitions cannot be overlooked.
In short, using different VIs for quantitative assessments as opposed to qualitative surveys demand that a number of issues are properly addressed for interpretation and comparability of results to be meaningful. Such discussion is outside the scope of this text. However, in particular, we stress the importance of assessing ground truth measurements (spectral and chemical) in future similar studies. Without ground truth measurements and spectral similitude analyses that compare field against aerial (and S2) observations it is not possible to fully clarify the relationship between spectral signals from different RS platforms, which is as yet a big gap in the already existing knowledge on the complementarity role of RS products.
Alongside, developments are expected in image processing and in transferring analysis outputs to automation and robotics; in real time, this would constitute a useful information tool that would greatly support decisions in rice farming and quick responses, leading to improvements in rice irrigation and agronomic management. In particular, it would support the adoption, and assessment of water saving irrigation management practices in rice cultivation.
Concluding Remarks
This study explores the role of RS as a tool to better characterize, e.g., rice crop responses and water requirements, and to support water management and agronomic decisions, namely in precision agriculture. Whereas literature report many RS applications in agriculture, those dedicated to rice farming are scarce. Moreover, to the best of our knowledge, no such study has yet been reported for the specific rice farming field conditions in the Lower Mondego Valley (Portugal); this rice production region contributes significantly to the rice production in Portugal.
Thus, this study contributes to the existing knowledge in this field. It investigates the potential usefulness of different RS technologies and products to assess rice cultivation conditions by calculating and comparing vegetation and water indices obtained from satellite and UAS multispectral and multi-temporal data. Main outcomes are:
1) The different data (from satellite and UAS) play a complementary role: while, overall, not offering yet high enough resolutions to allow for detailed surveys (which can be secured by the high resolution UAS technology) at the rice field plot scale, satellites’ images are nevertheless providing attractive routes to acquire field data of important temporal and spatial resolutions in a fast and easy way. Thus, overall, RS can play a crucial role in the process of rice cultivation, providing trustful tools for timely monitoring crop growth, and field conditions.
2) Particular features of the rice crop physiology and production systems demand that meaningful RS based indices are identified to adequately depict the spectral signature of specific local/regional rice growing conditions, because some vegetation indices could be more adequate than others on a case-to-case basis.
3) Land-use classifications based on multispectral data may generally benefit from using the NDRE index, which demonstrated to be particularly useful for identifying non-homogeneities in rice crop vigour during the crop’s vegetative phase, for the studied conditions. Since few satellite sensors are sensible in the Red Edge band and none has the high spatial resolution offered by UAS, this study confirms the potential of UAS to collect pertinent high-resolution images for spatial data on rice growing.
Whilst, more studies and tests dedicated to the whole rice crop season and diverse environmental conditions are still needed and being pursued, aiming at the increased understanding of different issues in RS satellite and UAS data. For example:
1) How much different from the description of the rice cultivation ground truth (as observed by ground RS measurements) is the signal provided by the sensors used in different RS platforms (e.g., satellite, UAS), so that comparability studies are meaningful? It is well known that one of the key requirements of RS applications is the need of ground truth measurements due to the several unknown factors that affect the quality of aerial spectral readings.
2) One specific problem of applying RS to study vegetation is the fact that different spectral signatures can be obtained at different hours along the day; thus, future studies with UAS should clarify this daily variation by examining the rice cultivation system at different times of the day and, moreover, confront results with companion ground truth measurements.
3) How to deal with the different spatial and spectral resolutions involved in RS data, acquired by different sensors at different levels, which involves non-trivial scale issues, and multiscale analysis methods?
All these issues highlight the importance of ground truth measurements, which are sometimes missing as a reference signal in many studies. Overall, there is still the need to deepen the knowledge in the field, with particular emphasis on the potential practical applications foreseen in precision agriculture, in particular those that pursue rice production sustainability.
Data Availability Statement
The original contributions presented in the study are included in the article/Supplementary Material, further inquiries can be directed to the corresponding author.
Author Contributions
IL and RJ conceived and designed the study and wrote the manuscript. RJ analysed the data. All authors contributed to article revision, read, and approved the submitted version of the manuscript.
Funding
This research was funded by Fundação para a Ciência e a Tecnologia (FCT), grant PRIMA/0006/2018 (project MEDWATERICE: Towards a sustainable water use in Mediterranean rice-based agro-ecosystems), with the support of PRIMA Programme (PRIMA-Section-2018; Topic: 1.1.3: Irrigation technologies and practices).
Conflict of Interest
The authors declare that the research was conducted in the absence of any commercial or financial relationships that could be construed as a potential conflict of interest.
Publisher’s Note
All claims expressed in this article are solely those of the authors and do not necessarily represent those of their affiliated organizations, or those of the publisher, the editors and the reviewers. Any product that may be evaluated in this article, or claim that may be made by its manufacturer, is not guaranteed or endorsed by the publisher.
Acknowledgments
The authors acknowledge the collaboration of Eng. A. Russo and Eng. J. Paixão, from the Associação de Beneficiários da Obra de Fomento Hidroagrícola do Baixo Mondego (Portugal), for their assistance regarding the identification of field conditions.
References
Akbarian, S., Xu, C.-Y., and Lim, S. (2020). Analysis on the Effect of Spatial and Spectral Resolution of Different Remote Sensing Data in Sugarcane Crop Yield Study. ISPRS Ann. Photogramm. Remote Sens. Spat. Inf. Sci. V-3-2020, 655–661. doi:10.5194/isprs-annals-V-3-2020-655-2020
Ben-Dor, E., Irons, J., and Epema, G. F. (1999). “Soil Reflectance,” in Remote Sensing for the Earth Sciences. Editor A. N. Rencz (New York: J. Wiley & Sons), 111–188.
Broge, N. H., and Leblanc, E. (2001). Comparing Prediction Power and Stability of Broadband and Hyperspectral Vegetation Indices for Estimation of green Leaf Area index and Canopy Chlorophyll Density. Remote Sensing Environ. 76, 156–172. doi:10.1016/S0034-4257(00)00197-8
Cantrell, R. P., and Reeves, T. G. (2002). The Rice Genome: The Cereal of the World's Poor Takes Center Stage. Science 296 (5565), 53. doi:10.1126/science.1070721
Cao, J., Cai, X., Tan, J., Cui, Y., Xie, H., Liu, F., et al. (2021). Mapping Paddy rice Using Landsat Time Series Data in the Ganfu Plain Irrigation System, Southern China, from 1988−2017. Int. J. Remote Sensing 42 (4), 1556–1576. doi:10.1080/01431161.2020.1841321
Ceccato, P., Flasse, S., and Grégoire, J.-M. (2002). Designing a Spectral index to Estimate Vegetation Water Content from Remote Sensing Data. Remote Sensing Environ. 82, 198–207. doi:10.1016/s0034-4257(02)00036-6
Ceccato, P., Flasse, S., Tarantola, S., Jacquemoud, S., and Grégoire, J.-M. (2001). Detecting Vegetation Leaf Water Content Using Reflectance in the Optical Domain. Remote Sensing Environ. 77 (1), 22–33. doi:10.1016/S0034-4257(01)00191-2
Cen, H., Wan, L., Zhu, J., Li, Y., Li, X., Zhu, Y., et al. (2019). Dynamic Monitoring of Biomass of rice under Different Nitrogen Treatments Using a Lightweight UAV with Dual Image-Frame Snapshot Cameras. Plant Methods 15, 32. doi:10.1186/s13007-019-0418-8
Cui, Z., and Rekes, K. P. (2018). Potential of Red Edge Spectral Bands in Future Landsat Satellites on Agroecosystem Canopy green Leaf Area index Retrieval. Remote Sens 10 (9), 1–14. doi:10.3390/rs10091458
Dawe, D., Frolking, S., and Li, C. (2004). Trends in Rice-Wheat Area in China. Field Crops Res. 87 (1), 89–95. doi:10.1016/j.fcr.2003.08.008
de Jong, S. M. (2020). Grand Challenges in Earth Observation Using Unpiloted Airborne Systems. Front. Remote Sens. 1, 601737. doi:10.3389/frsen.2020.601737
de la Casa, A., Ovando, G., Bressanini, L., Martínez, J., Díaz, G., and Miranda, C. (2018). Soybean Crop Coverage Estimation from NDVI Images with Different Spatial Resolution to Evaluate Yield Variability in a Plot. ISPRS J. Photogrammetry Remote Sensing 146, 531–547. doi:10.1016/j.isprsjprs.2018.10.018
Duan, B., Liu, Y., Gong, Y., Peng, Y., Wu, X., Zhu, R., et al. (2019). Remote Estimation of rice LAI Based on Fourier Spectrum Texture from UAV Image. Plant Methods 15, 124. doi:10.1186/s13007-019-0507-8
Fitzgerald, G. J., Rodriguez, D., Christensen, L. K., Belford, R., Sadras, V. O., and Clarke, T. R. (2006). Spectral and thermal Sensing for Nitrogen and Water Status in Rainfed and Irrigated Wheat Environments. Precision Agric. 7, 233–248. doi:10.1007/s11119-006-9011-z
Gao, B.-c. (1996). NDWI-A Normalized Difference Water index for Remote Sensing of Vegetation Liquid Water from Space. Remote Sensing Environ. 58 (3), 257–266. doi:10.1016/s0034-4257(96)00067-3
Gitelson, A. A., Kaufman, Y. J., and Merzlyak, M. N. (1996). Use of a green Channel in Remote Sensing of Global Vegetation from EOS-MODIS. Remote Sensing Environ. 58, 289–298. doi:10.1016/s0034-4257(96)00072-7
Gitelson, A. A., and Merzlyak, M. N. (1998). Remote Sensing of Chlorophyll Concentration in Higher Plant Leaves. Adv. Space Res. 22, 689–692. doi:10.1016/s0273-1177(97)01133-2
Gitelson, A. A., and Merzlyak, M. N. (1996). Signature Analysis of Leaf Reflectance Spectra: Algorithm Development for Remote Sensing of Chlorophyll. J. Plant Physiol. 148 (3-4), 494–500. doi:10.1016/s0176-1617(96)80284-7
Gitelson, A., and Merzlyak, M. N. (1994). Spectral Reflectance Changes Associated with Autumn Senescence of Aesculus Hippocastanum L. And Acer Platanoides L. Leaves. Spectral Features and Relation to Chlorophyll Estimation. J. Plant Physiol. 143 (3), 286–292. doi:10.1016/s0176-1617(11)81633-0
González-Jorge, H., Martínez-Sánchez, J., Bueno, M., Arias, A. P., and Arias, P. (2017). Unmanned Aerial Systems for Civil Applications: a Review. Drones 1 (1), 2. doi:10.3390/drones1010002
Gonçalves, J. M., and de Lima, I. P. (2018). “Improving Water Productivity in Irrigated Agriculture: Challenges from Climate Change and New Water Resources Paradigms,” in Theory and Practice of Climate Adaptation. Editors F. Alves, W. Leal Filho, and U. Azeiteiro (Cham: Springer), 347–361. doi:10.1007/978-3-319-72874-2_20
G. Poley, L., and J. McDermid, G. (2020). A Systematic Review of the Factors Influencing the Estimation of Vegetation Aboveground Biomass Using Unmanned Aerial Systems. Remote Sensing 12, 1052. doi:10.3390/rs12071052
Gu, Y., Brown, J. F., Verdin, J. P., and Wardlow, B. (2007). A Five-Year Analysis of MODIS NDVI and NDWI for Grassland Drought Assessment over the central Great Plains of the United States. Geophys. Res. Lett. 34, L06407. doi:10.1029/2006GL029127
Gu, Y., Hunt, E., Wardlow, B., Basara, J. B., Brown, J. F., and Verdin, J. P. (2008). Evaluation of MODIS NDVI and NDWI for Vegetation Drought Monitoring Using Oklahoma Mesonet Soil Moisture Data. Geophys. Res. Lett. 35, L22401. doi:10.1029/2008GL035772
Hama, A., Tanaka, K., Mochizuki, A., Tsuruoka, Y., and Kondoh, A. (2020). Estimating the Protein Concentration in Rice Grain Using UAV Imagery Together with Agroclimatic Data. Agronomy 10 (3), 431. doi:10.3390/agronomy100304310.3390/agronomy10030431
Hoffmann, H., Nieto, H., Jensen, R., Guzinski, R., Zarco-Tejada, P., and Friborg, T. (2016). Estimating Evaporation with thermal UAV Data and Two-Source Energy Balance Models. Hydrol. Earth Syst. Sci. 20, 697–713. doi:10.5194/hess-20-697-2016
Horler, D. N. H., Dockray, M., and Barber, J. (1983). The Red Edge of Plant Leaf Reflectance. Int. J. Remote Sensing 4 (2), 273–288. doi:10.1080/01431168308948546
Howell, T. A. (2001). Enhancing Water Use Efficiency in Irrigated Agriculture. Agron.j. 93, 281–289. doi:10.2134/agronj2001.932281x
Huang, J., Gómez-Dans, J. L., Huang, H., Ma, H., Wu, Q., Lewis, P. E., et al. (2019). Assimilation of Remote Sensing into Crop Growth Models: Current Status and Perspectives. Agric. For. Meteorology 276-277, 107609. doi:10.1016/j.agrformet.2019.06.008
Jorge, J., Vallbé, M., and Soler, J. A. (2019). Detection of Irrigation Inhomogeneities in an Olive grove Using the NDRE Vegetation index Obtained from UAV Images. Eur. J. Remote Sensing 52 (1), 169–177. doi:10.1080/22797254.2019.1572459
Kanke, Y., Tubaña, B., Dalen, M., and Harrell, D. (2016). Evaluation of Red and Red-Edge Reflectance-Based Vegetation Indices for rice Biomass and Grain Yield Prediction Models in Paddy fields. Precision Agric. 17 (5), 507–530. doi:10.1007/s11119-016-9433-1
Karnieli, A., Agam, N., Pinker, R. T., Anderson, M., Imhoff, M. L., Gutman, G. G., et al. (2010). Use of NDVI and Land Surface Temperature for Drought Assessment: Merits and Limitations. J. Clim. 23 (3), 618–633. doi:10.1175/2009jcli2900.1
Kasampalis, D., Alexandridis, T., Deva, C., Challinor, A., Moshou, D., and Zalidis, G. (2018). Contribution of Remote Sensing on Crop Models: A Review. J. Imaging, 4, 52. doi:10.3390/jimaging4040052
Kawamura, K., Asai, H., Yasuda, T., Soisouvanh, P., and Phongchanmixay, S. (2020). Discriminating Crops/weeds in an upland rice Field from UAV Images with the SLIC-RF Algorithm. Plant Prod. Sci. 24 (2), 198–215. doi:10.1080/1343943X.2020.1829490
Kuroso, T., Fujita, M., and Chiba, K. (1997). Monitoring of Rice Fields Using Multi-Temporal ERS-1 C-Band SAR Data. Int. J. Remote Sensing 14, 2953–2965.
Lausch, A., Pause, M., Merbach, I., Zacharias, S., Doktor, D., Volk, M., et al. (2013). A New Multiscale Approach for Monitoring Vegetation Using Remote Sensing-Based Indicators in Laboratory, Field, and Landscape. Environ. Monit. Assess. 185, 1215–1235. doi:10.1007/s10661-012-2627-8
Le Toan, T., Ribbes, F., Li-Fang Wang, L.-F., Floury, N., Kung-Hau Ding, K.-H., Jin Au Kong, J. A., et al. (1997). Rice Crop Mapping and Monitoring Using ERS-1 Data Based on experiment and Modeling Results. IEEE Trans. Geosci. Remote Sensing 35 (1), 41–56. doi:10.1109/36.551933
Maccioni, A., Agati, G., and Mazzinghi, P. (2001). New Vegetation Indices for Remote Measurement of Chlorophylls Based on Leaf Directional Reflectance Spectra. J. Photochem. Photobiol. B 61 (1–2), 52–61. doi:10.1016/S1011-1344(01)00145-2
Maes, W. H., and Steppe, K. (2019). Perspectives for Remote Sensing with Unmanned Aerial Vehicles in Precision Agriculture. Trends Plant Sci. 24 (2), 152–164. doi:10.1016/j.tplants.2018.11.007
Manfreda, S., McCabe, M., Miller, P., Lucas, R., Pajuelo Madrigal, V., Mallinis, G., et al. (2018). On the Use of Unmanned Aerial Systems for Environmental Monitoring. Remote Sensing 10 (4), 641. doi:10.3390/rs10040641
McFeeters, S. K. (1996). The Use of the Normalized Difference Water Index (NDWI) in the Delineation of Open Water Features. Int. J. Remote Sensing 17 (7), 1425–1432. doi:10.1080/01431169608948714
Mosleh, M., Hassan, Q., and Chowdhury, E. (2015). Application of Remote Sensors in Mapping rice Area and Forecasting its Production: A Review. Sensors 15 (1), 769–791. doi:10.3390/s150100769
Motohka, T., Nasahara, K. N., Miyata, A., Mano, M., and Tsuchida, S. (2009). Evaluation of Optical Satellite Remote Sensing for rice Paddy Phenology in Monsoon Asia Using a Continuous In Situ Dataset. Int. J. Remote Sensing 30 (17), 4343–4357. doi:10.1080/01431160802549369
Nhamo, L., Magidi, J., Nyamugama, A., Clulow, A. D., Sibanda, M., Chimonyo, V. G. P., et al. (2020). Prospects of Improving Agricultural and Water Productivity through Unmanned Aerial Vehicles. Agriculture 10 (7), 256. doi:10.3390/agriculture10070256
Norasma, C. Y. N., Abu Sari, M. Y., Fadzilah, M. A., Ismail, M. R., Omar, M. H., Zulkarami, B., et al. (2018). Rice Crop Monitoring Using Multirotor UAV and RGB Digital Camera at Early Stage of Growth. IOP Conf. Ser. Earth Environ. Sci. IOP Publishing 169 (1), 012095. doi:10.1088/1755-1315/169/1/012095
Normile, D. (2008). AGRICULTURAL RESEARCH: Reinventing Rice to Feed the World. Science 321, 330–333. doi:10.1126/science.321.5887.330
Oette, D. R., Warren, B. C., Mercedes, B., Maiersperger, T. K., and Kennedy, R. E. (2001). Land Cover Mapping in Agricultural Setting Using Multiseasonal Thematic Mapper Data. Remote Sens. Environ. 76, 139–155. doi:10.1016/S0034-4257(00)00202-9
Panigrahy, S., and Sharma, S. A. (1997). Mapping of Crop Rotation Using Multidate Indian Remote Sensing Satellite Digital Data. ISPRS J. Photogrammetry Remote Sensing 52, 85–91. doi:10.1016/s0924-2716(97)83003-1
Rouse, J. W., Haas, R. H., Schell, J. A., and Deering, D. W. (1974). “Monitoring Vegetation Systems in the Great Plains with ERTS,” in Third Earth Resources Technology Satellite–1 Symposium. Volume I: Technical Presentations, NASA SP-351. Editors S. C. Freden, E. P. Mercanti, and M. Becker (Washington, D.C.: NASA), 309–317.
Schaefer, M., and Lamb, D. (2016). A Combination of Plant NDVI and LiDAR Measurements Improve the Estimation of Pasture Biomass in Tall Fescue (Festuca Arundinacea Var. Fletcher). Remote Sensing 8 (2), 109. doi:10.3390/rs8020109
Shao, Y., Fan, X., Liu, H., Xiao, J., Ross, S., Brisco, B., et al. (2001). Rice Monitoring and Production Estimation Using Multitemporal RADARSAT. Remote Sensing Environ. 76 (3), 310–325. doi:10.1016/s0034-4257(00)00212-1
Shao, Y., Wang, C., Fan, X., and Liu, H. (1997). Geomatics in Era of RADARSAT. Ottawa, Canada.Evaluation of SAR Image for Rice Monitoring and Land Cover Mapping
Shiu, Y.-S., and Chuang, Y.-C. (2019). Yield Estimation of Paddy Rice Based on Satellite Imagery: Comparison of Global and Local Regression Models. Remote Sensing 11, 111. doi:10.3390/rs11020111
Stroppiana, D., Migliazzi, M., Chiarabini, V., Crema, A., Musanti, M., Franchino, C., et al. (2015). “Rice Yield Estimation Using Multispectral Data from UAV: A Preliminary experiment in Northern Italy,” in Geoscience and Remote Sensing Symposium (IGARSS), 2015 IEEE International (IEEE), 4664–4667. doi:10.1109/igarss.2015.7326869
Tatum, M. C., and Liu, J. (2017). Unmanned Aircraft System Applications in Construction. Proced. Eng. 196, 167–175. doi:10.1016/j.proeng.2017.07.187
Tilly, N., Hoffmeister, D., Cao, Q., Huang, S., Lenz-Wiedemann, V., Miao, Y., et al. (2014). Multitemporal Crop Surface Models: Accurate Plant Height Measurement and Biomass Estimation with Terrestrial Laser Scanning in Paddy rice. J. Appl. Remote Sens 8, 083671. doi:10.1117/1.JRS.8.083671
Tmušić, G., Manfreda, S., Aasen, H., James, M. R., Gonçalves, G., Ben-Dor, E., et al. (2020). Current Practices in UAS-Based Environmental Monitoring. Remote Sensing 12 (6), 1001. doi:10.3390/rs12061001
Van Nguyen, N., and Ferrero, A. (2006). Meeting the Challenges of Global rice Production. Paddy Water Environ. 4, 1–9. doi:10.1007/s10333-005-0031-5
Wan, L., Cen, H., Zhu, J., Zhang, J., Du, X., and He, Y. (2020). Using Fusion of Texture Features and Vegetation Indices from Water Concentration in rice Crop to UAV Remote Sensing Monitor. Smart Agric. 2 (1), 58–67.
Wu, H., and Li, Z.-L. (2009). Scale Issues in Remote Sensing: a Review on Analysis, Processing and Modeling. Sensors 9, 1768–1793. doi:10.3390/s90301768
Wu, J., Yang, G., Yang, X., Xu, B., Han, L., and Zhu, Y. (2019). Automatic Counting of In Situ Rice Seedlings from UAV Images Based on a Deep Fully Convolutional Neural Network. Remote Sensing 11 (6), 691. doi:10.3390/rs11060691
Xiao, X., Boles, S., Liu, J., Zhuang, D., Frolking, S., Li, C., et al. (2005). Mapping Paddy rice Agriculture in Southern China Using Multi-Temporal MODIS Images. Remote Sensing Environ. 95 (4), 480–492. doi:10.1016/j.rse.2004.12.009
Xue, J., and Su, B. (2017). Significant Remote Sensing Vegetation Indices: A Review of Developments and Applications. J. Sensors 2017, 1–17. Article ID 1353691. doi:10.1155/2017/1353691
Yang, C.-Y., Yang, M.-D., Tseng, W.-C., Hsu, Y.-C., Li, G.-S., Lai, M.-H., et al. (2020). Assessment of Rice Developmental Stage Using Time Series UAV Imagery for Variable Irrigation Management. Sensors 20 (18), 5354. doi:10.3390/s20185354
Yang, M.-D., Tseng, H.-H., Hsu, Y.-C., Yang, C.-Y., Lai, M.-H., and Wu, D.-H. (2021). A Uav Open Dataset of Rice Paddies for Deep Learning Practice. Remote Sensing 13, 1358. doi:10.3390/rs13071358
Yu, J., Hu, S., Wang, J., Wong, G. K., Li, S., Liu, B., et al. (2002). A Draft Sequence of the rice Genome (Oryza Sativa L. Ssp. Indica). Science 296 (5565), 79–92. PMID: 11935017. doi:10.1126/science.1068037
Yuhao, A., Che’Ya, N. N., Roslin, N. A., and Ismail, M. R. (2020). Rice Chlorophyll Content Monitoring Using Vegetation Indices from Multispectral Aerial Imagery. Pertanika J. Sci. Technol. 28 (3), 779–795. http://www.pertanika.upm.edu.my/pjst/browse/regular-issue?article=JST-1950-2020.
Zha, H., Miao, Y., Wang, T., Li, Y., Zhang, J., Sun, W., et al. (2020). Improving Unmanned Aerial Vehicle Remote Sensing-Based Rice Nitrogen Nutrition Index Prediction with Machine Learning. Remote Sensing 12 (2), 215. doi:10.3390/rs12020215
Zhang, K., Kimball, J. S., and Running, S. W. (2016). A Review of Remote Sensing Based Actual Evapotranspiration Estimation. WIREs Water 3 (6), 834–853. doi:10.1002/wat2.1168
Zhao, G., Miao, Y., Wang, H., Su, M., Fan, M., Zhang, F., et al. (2013). A Preliminary Precision rice Management System for Increasing Both Grain Yield and Nitrogen Use Efficiency. Field Crops Res. 154, 23–30. doi:10.1016/j.fcr.2013.07.019
Keywords: UAS (unmanned aerial system), vegetation indices, Red edge band, precision agriculture, rice irrigation, satellite images, Sentinel-2
Citation: de Lima IP, Jorge RG and de Lima JLMP (2021) Remote Sensing Monitoring of Rice Fields: Towards Assessing Water Saving Irrigation Management Practices. Front. Remote Sens. 2:762093. doi: 10.3389/frsen.2021.762093
Received: 20 August 2021; Accepted: 08 October 2021;
Published: 25 October 2021.
Edited by:
Yijian Zeng, University of Twente, NetherlandsReviewed by:
Nicolas Francos, Tel Aviv University, IsraelRuodan Zhuang, University of Basilicata, Italy
Xiaodong Gao, Northwest A and F University, China
Copyright © 2021 de Lima, Jorge and de Lima. This is an open-access article distributed under the terms of the Creative Commons Attribution License (CC BY). The use, distribution or reproduction in other forums is permitted, provided the original author(s) and the copyright owner(s) are credited and that the original publication in this journal is cited, in accordance with accepted academic practice. No use, distribution or reproduction is permitted which does not comply with these terms.
*Correspondence: Isabel Pedroso de Lima, aXBsaW1hQHVjLnB0