- Wee Kee Wee School of Communication and Information, Nanyang Technological University, Singapore, Singapore
Conventionally, human-controlled and machine-controlled virtual characters are studied separately under different theoretical frameworks based on the ontological nature of the particular virtual character. However, in recent years, the technological advancement has made the boundaries between human and machine agency increasingly blurred. This manuscript proposes a theoretical framework that can explain how various virtual characters, regardless of their ontological agency, can be treated as unique social actors with a focus on perceived authenticity. Specifically, drawing on the authenticity model in computer-mediated communication proposed by Lee (2020) and a typology of virtual characters, a multi-layered perceived authenticity model is proposed to demonstrate how virtual characters do not have to be perceived as humans and yet can be perceived as authentic to their human interactants.
1 Introduction
With the development in communication and Artificial Intelligence (AI) technologies, people nowadays are interacting with various human-controlled and machine-controlled virtual characters more on a daily basis, which is re-shaping our social experience and challenging the ontological concept of authenticity (Lee and Shin, 2004; Gulz, 2005; Turkle, 2007; Van Oijen and Dignum, 2013; Enli, 2014; Xueming; Luo, 2019). Although research under the CASA (Computers As Social Actors) paradigm indicates that some social rules in human-to-human interactions can be applied to people’s interaction with non-human machines, resulting in human responses as if non-human machines were actual social actors (Reeves and Nass, 1996), studies also suggest that in many cases, people still treat technology and humans as different kinds of communicators (Shechtman and Horowitz, 2003; de Borst and de Gelder, 2015; Mou and Xu, 2017). Scholars from the emerging field of Human-Machine Communication (HMC) argue that CASA research, focusing on people’s social responses to social cues in technology should not be misinterpreted as people think of and behave towards human and non-human communicators in the same manner (Guzman and Lewis, 2020). In other words, people’s responses to non-human machines vary, and it is still unclear in terms of what triggers so-called mindless responses from human communicators when they interact with virtual characters, regardless of such virtual characters’ ontological agency.
Previous research on virtual characters often studies human-controlled virtual characters (e.g., user-controlled avatars in computer games) and machine-controlled virtual characters (e.g., artificial agents) as two separate fields under different theoretical frameworks. The former is primarily placed in computer-mediated communication, and the latter is mainly examined in the area of human-computer interaction. As such, despite the emerging growth in research from both fields, the discussion and theoretical explanations tend to be separate, based on the ontological agency of virtual characters. However, as the performance of AI agents improves, the boundary between human agency and machine agency is increasingly blurred from users’ perspectives (Jaderberg et al., 2019; Ye et al., 2020). In addition, some emerging virtual characters, such as Vocaloid virtual idols can comprise elements of both human agency and machine agency (Lam, 2016; Marsh, 2016; Sousa, 2016; Jenkins, 2018; Zhang, 2019). Therefore, it is necessary to have one unified theoretical framework that can explain how people interact with various virtual characters naturally, regardless of their ontological agency. One approach for developing a new theoretical framework is to revisit the concept of authenticity.
In understanding people’s experience with virtual characters, authenticity has been studied as a core concept. In conventional HCI research, “realness” is often regarded as equal to “human-likeness,” which mainly concerns whether a machine looks and feels like an actual human to users (Turing, 1950; Weizenbaum, 1966; de Borst and de Gelder, 2015; Shum et al., 2018; Chaves and Gerosa, 2019). However, as machine agents are becoming more ubiquitous in human life (Rahwan et al., 2019), they are increasingly conceived as distinctive communicators rather than mere copies of human communicators (Guzman and Lewis, 2020). In this process, people’s expectations and perceptions of machine-controlled virtual characters and their relationships with them can also evolve (Turkle, 2007; Turkle, 2011; Sundar, 2020): People know that such virtual characters are machines, but they do not care about virtual characters’ agency.
Meanwhile, people’s perception of “what is real” can also be reshaped by their virtual experience (Turkle, 2011; Enli, 2014). Particularly for the young generation who grew up in a culture where “human contact is routinely replaced by virtual life, computer games, and relational artifacts” (Turkle, 2007, p. 514), sometimes ontologically authentic objects (i.e., actual objects) may not be perceived as authentic as virtual objects to them. In other words, machine agents may not necessarily need to be perceived as humans to be perceived as authentic—as long as they satisfy individuals’ heuristics about machines.
Drawing on the authenticity model in communication proposed by Lee (2020), the current article suggests that people’s experience with virtual characters be examined based on the perceived authenticity of source—whether the virtual characters’ claimed identities are perceived as authentic. The most distinctive characteristic of perceived source authenticity in people’s experience with virtual characters is that it is multi-layered: the identity of a particular virtual character consists of two layers. The first layer pertains to its claimed agency, whether the perceived agency of a virtual character matches with its claimed agency. The second layer pertains to perceived authenticity of the virtual character’s represented identities, whether the designed social or individual identities of the virtual character’s representation are perceived as authentic. In this newly proposed model of perceived authenticity of source, an AI agent that looks and talks like a machine can be perceived as authentic as long as the AI agent satisfies users’ needs and heuristics.
2 The rise of virtual characters
According to Lee (2004), the ontological nature of objects experienced by human beings can be classified into three categories: actual objects, virtual objects, and imaginary objects. Actual objects are objects that can be directly experienced via the human sensory systems without using technology; virtual objects are objects that cannot be experienced by human beings without using technology; imaginary objects are objects that can only be experienced by human beings in their hallucination in a non-sensory way. Typically, virtual objects are either mediated by technology (i.e., para-authentic objects) or created/simulated with technology (i.e., artificial objects). With the advancement in media, information, and communication technologies, virtual objects, including various media representations and artificial-intelligence-enabled machines, have become increasingly pervasive in contemporary life (Deuze, 2011; Rahwan et al., 2019). As a result, virtual experience (i.e., human beings’ experience of virtual objects) has become more prominent in our everyday experience (Lee and Jung, 2005).
Among various virtual objects, virtual objects that look or act like humans or other living organisms play a large part in people’s virtual social experience. Some of these virtual objects—such as the video game character Mario—have human-like appearances. Some do not manifest human appearances, such as the Disney character Mickey Mouse, but behave in human-like ways. Some others—such as the Tamagotchi the digital pet, and Aibo the robotic dog—look or act like other living creatures instead of human beings, but still have been popular virtual characters (Turkle, 2007).
Initially, the concept of characters refers to fictional human- or non-human- beings in narratives, typically in literature (Everett, 2007; Maslej et al., 2017; James, 2019). As technology develops, both the locus and the representations of characters have been enriched: no longer do characters exist in the “word-masses” in literature works (Keen, 2011), but they can also be seen, heard, and even felt in media as well as in the physical world via technology (Hoorn and Koinijn, 2003; Gulz, 2005; Reynolds, 2006; Holz et al., 2009; de Borst and de Gelder, 2015; Peters et al., 2019; Kellems et al., 2020). As such, virtual characters are defined as any virtual objects that manifest characteristics of or similar to living beings in the current paper.
People nowadays increasingly interact with various virtual characters. These interactions may range from playing online games with other people’s avatars (i.e., individuals’ digital representations) to ordering products via chatbots on messaging apps, from talking to smart speakers to playing with pet robots, and from watching streaming events of virtual YouTubers who are actual people in the form of animated avatars, to attending offline concerts where they can witness, for example, the performance of the 3-D projection of Hatsune Miku, a popular virtual singer created by a Japanese Vocaloid software. As AI and media technologies evolve, the scope of virtual characters continues to expand rapidly (Rahwan et al., 2019). For example, animated virtual characters have been widely used in enriching messages in CMC (Kiskola 2018; Cha, 2018); the use of chatbots has been increasing exponentially and is still trending (Trivedi and Thakkar, 2019); people’s use of voice assistants such as Apple Siri and Amazon Echo are on the rise (Pew Research Centre 2017, 2019); markets for virtual idols and virtual streamers are fast-growing (Sone, 2017; Zhou, 2020); social robots may substitute humans in child education (Park et al., 2017) and elderly care in the future (Bemelmans et al., 2012).
Despite the potential and benefits that virtual characters may bring to human life (Dautenhahn, 2003; Rahwan et al., 2019), there are also growing concerns over issues that may accompany the prevalence of virtual characters. For example, there are concerns about the individual psychological consequences of people’s increasing reliance on digitalized friends and social robots: simulation provides relationships simpler than what real life can offer, and thus may diminish people’s ability to handle the necessary complexities to achieve interpersonal intimacies (Turkle, 2011). To address these concerns, a better understanding of people’s experience with virtual characters would be an essential process.
3 Virtual characters as unique social actors
A primary reason that makes virtual characters important in people’s virtual experience lies in their social nature. Most virtual characters are designed to interact with people, and extensive research under the Computers Are Social Actors (CASA) paradigm has demonstrated that they do serve as social actors when experienced by people (Nass et al., 1994; Reeves and Nass, 1996; Lee and Nass, 2003). As a pivotal paradigm in Human-Computer Interaction (HCI) related research, CASA suggests that people respond to computers as if they are social actors (Nass et al., 1994; Nass et al., 1996) and interact with them socially in a mindless way (Nass and Moon 2000), as long as they display social cues (e.g., “being polite,” in Reeves and Nass, 1996). The effect is not limited to people’s reaction to computers but to other media technology such as television and new media, supported by a series of research in which social rules in human-human interaction were found to also work in human-media interaction (Reeves and Nass, 1996). The phenomenon of people reaction to mediated or simulated objects as social actors were thus termed “media equation”—meaning “media equals real life” (Reeves and Nass, 1996).
Although CASA research indicates that some social rules in human-to-human interactions can be applied to people’s interaction with computers, including virtual characters (Reeves and Nass, 1996), studies also suggest that people treat technology and humans as different kinds of communicators (Shechtman and Horowitz, 2003; de Borst and de Gelder, 2015; Mou and Xu, 2017). Nevertheless, it is unclear whether people’s social responses to computers in the CASA paradigm are subject to certain conditions; it is also unclear whether the kind and level of people’s social responses in human-machine communication are different from those in human-human communication (Fischer et al., 2011; Mou and Xu, 2017; Guzman and Lewis, 2020). Some studies have suggested the “media inequality” when people respond differently to machine and human communicators: for example, participants talk more and use four-times-more relationship statements when interacting with humans than with artificial agents (Shechtman and Horowitz, 2003); participants responded faster to humans’ greetings than to robots greetings (Kanda et al., 2005); participants tend to use different strategies when interacting with artificial agents from when they are interacting with humans—they displayed more socially desirable personality traits during the initial conversations with humans than with artificial agents (Mou and Xu, 2017).
To explain this phenomenon, scholars from the emerging field of Human-Machine Communication (HMC) argue that machines should be treated as communicative subjects rather than just channels of communication, especially when AI is involved (Guzman, 2018). They emphasize that CASA research should not be “misinterpreted as evidence that people think of a particular technology as human or behave exactly the same toward a human and device in all aspects of communication” (Guzman and Lewis, 2020, p.76). In other words, despite the fact that both virtual characters and humans may be perceived as social actors in people’s social experience, it is necessary to differentiate the types of social actors between human beings and virtual characters, as well as among various virtual characters.
As a complement to conventional communication paradigms that focus on human-human communication, the HMC frameworks treat technology as “communicative objects, instead of mere interactive objects” (Guzman and Lewis, 2020, p.71) and is particularly suitable for studying people’s experience with artificial agents (Mou and Xu, 2017; Rahwan et al., 2019). Given the increasingly prominent role of virtual characters in our daily lives, research that treats virtual characters as unique social actors, different from traditional social actors, can contribute to the field of HMC and foster a more sophisticated understanding of people’s virtual social experience.
4 Virtual characters as a multi-dimensional concept
Previous research on people’s experience with virtual characters tends to study virtual characters of different ontological nature under different theoretical frameworks, often in separate fields. For example, human-controlled virtual characters, such as avatars—defined as digital representations of human users “that facilitates interactions with other users, entities, or the environment” (Nowak and Fox, 2018, p.34)—are primarily studied in computer-mediated communication (Kang and Yang, 2006; Nowak and Rauh, 2006; Yee and Bailenson, 2007; Vasalou and Joinson, 2009; Nowak and Fox, 2018). On the contrary, machine-controlled virtual characters, such as artificial agents—defined as computer-algorithm-driven autonomous entities capable of pro-active and reactive behaviour in the environments they situate in (Franklin and Graesser, 1996; Holz et al., 2009)—are typically studied in the fields of human-computer interaction, human-robot interaction, and human-machine communication (Franklin and Graesser, 1996; Dehn and Van Mulken, 2000; Lee et al., 2006; Dautenhahn, 2007; Holz et al., 2009; Chaves and Gerosa, 2019). However, it is timely and meaningful to comprehend virtual characters as a multi-dimensional unifying concept, particularly in understanding people’s experience with increasingly complex virtual characters.
4.1 The complexity of virtual characters
With the advances in simulation technology and artificial intelligence (AI), both the types and complexity of virtual characters are increasing (Rahwan et al., 2019), blurring the presumed boundaries between avatars and artificial agents.
Firstly, due to the exponentially growing AI capabilities, the ontological differences between avatars and artificial agents can sometimes be inconspicuous from the perspective of individual users. Although computer-controlled agents and human-controlled avatars are qualitatively different, in digital environments, sometimes people do not have first-hand knowledge about the agency of the digital representation they encounter. As a result, people sometimes cannot tell whether a virtual representation is controlled by a computer agent or a human agent, especially with the advances in AI technology (Nowak and Biocca, 2003). For example, the emerging AI agents can cooperate with both AI and human players with human-level performance in massively multiplayer video games (Jaderberg et al., 2019; Ye et al., 2020), which can make users hard to tell whether they are playing with an artificial agent or someone’s avatar if not informed. As a result, perceived agency became an essential assessment that contributes to people’s reactions to claimed “avatars” (Nowak and Fox, 2018). In other words, although the agency of a virtual character perceived as avatars is expected to be humans, it can actually be operated by computer algorithms—the boundaries between artificial agents and human avatars are increasingly blurred from the perspective of users.
Secondly, unlike typical avatars and artificial agents that are studied within separate fields, some emerging virtual characters are more complicated and have both characteristics of avatars and AI agents, which demands research across fields. The increasingly popular Vocaloid characters is an example of such complex virtual characters.
Vocaloid characters are virtual characters, mostly virtual performers, created using the software, Vocaloid (Conner, 2016). The representations of Vocaloid characters are mostly animated, possibly because this design strategy can help them stay away from the “uncanny valley1” (Conner, 2016). Using Vocaloid, users can design singing and dancing actions to produce music video clips, performed by the animated characters. Although Vocaloid was initially released in 2004, the surging popularity of Vocaloid idols did not emerge until 2011 when the Vocaloid virtual idol, Hatsune Miku was introduced to the public. So far, Vocaloid virtual idols have attracted millions of fans globally, especially in East Asia (Conner, 2016). For example, in China, the Vocaloid virtual idol, Luo Tianyi has become the spokeswoman for a number of brands, including KFC, Nestlé, and L’Occitane (Yau, 2020).
Compared to earlier animated virtual performers such as the Muppets, the Archies, and Gorillaz—which were voice-acted by real human artists (Conner, 2013, 2016), Vocaloid characters have several distinct features. Vocaloid character’s voice production is a combination of original biological elements and artificial manipulation. Although the singings of Vocaloid characters are produced using computer programs, yet the original material of the voicebank is extracted from natural human voices, instead of being purely synthesized by computers. For example, the voicebank of Hatsune Miku was sampled from the Japanese voice actress, Saki Fujita (Sousa, 2016). This strategy makes the voice of Vocaloid characters much less machinery, and yet still allows the software users to manipulate the content of the singings. In addition, 3D hologram imagery technology has brought Vocaloid characters from television and computer screens into physical spaces (Conner, 2016). For example, both Hatsune Miku and Luo Tianyi have held live concerts as holographic projections in physical theaters in front of thousands of live attendees. They sometimes even perform together with real-human artists on the same stage (as shown in Figure 1), which has blurred the perceived boundary between reality and virtuality for the audience (Lam, 2016; Marsh, 2016; Zhang, 2019). Lastly, while the animated representations of Vocaloid characters were initially created by commercial companies, they have evolved through the co-creation by their fans. To be specific, any Vocaloid users may input lyrics and melodies to the software to produce new performances, acted by these Vocaloid idols. As a result, Vocaloid virtual idols are peer-produced media characters rather than conventional media-produced characters, which contributes to their success significantly (Leavitt et al., 2016).
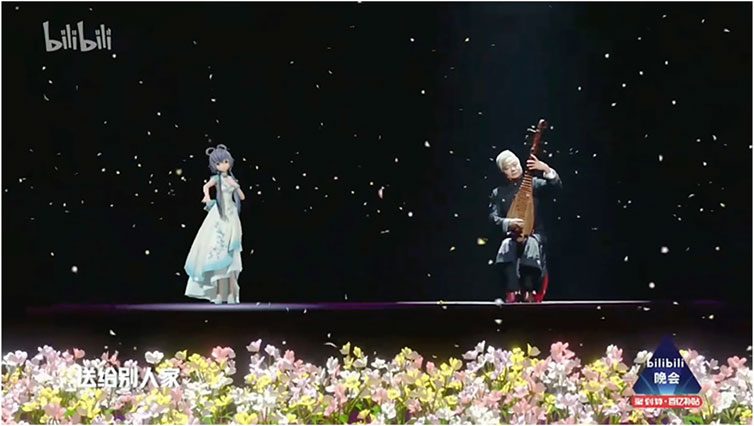
FIGURE 1. The hologram version of Chinese Vocaloid character Luo Tianyi performs on the same physical stage with the Pipa master Fang Jinlong during a New Year’s Eve Concert hosted by Bilibili on December 31, 2019. The flowers and snowflakes were also generated by CGI technology and projected via hologram technology.
Besides being media characters, Vocaloid characters also have mixed characteristics of avatars and artificial agents, although they cannot be strictly categorized as either. Their movements are generated from computer programs, and when they perform on stage, it seems like they are acting on their own. In this sense, they are computer-algorithm-driven autonomous entities just like artificial agents. However, an important distinctive feature of artificial agents is that they are capable of being pro-active and reactive in the environments they situate in (Franklin and Graesser, 1996; Holz et al., 2009), which Vocaloid characters are not capable of yet. Although they interact with the audience in live concerts and during online live-streaming (Jenkins, 2018; Shen, 2020; Xue, 2020), they cannot really improvise. Their “improvised” speeches mostly rely on either pre-recorded vocals or simultaneous human voice-acting (Jenkins, 2018).
To summarize, the increased prevalence of complex virtual characters such as Vocaloid characters necessitates a more comprehensive understanding of people’s experience with virtual characters. Such a comprehensive understanding requires us to examine virtual characters as a multi-dimensional concept, instead of only considering emerging types of virtual characters separately on a case-by-case basis. Not only can a multi-dimensional approach to virtual characters facilitate a better understanding of recently emerged complex virtual characters, but it can also contribute to the fundamental understanding of virtual characters that may help understand future virtual characters yet to appear. By categorizing virtual characters into common dimensions, different aspects of technology can be studied comprehensively, which is meaningful to both academic research and industrial practice.
4.2 Two basic dimensions of virtual characters
The multi-dimensional conceptualization of virtual characters can be understood in two basic dimensions: type of agency and way of representation. Specifically, the type of agency pertains to whether a virtual character is controlled by humans or machines, while the way of representation pertains to whether a virtual character is physically embodied, digitally embodied, or disembodied.
4.2.1 Agency
The first dimension of a virtual character pertains to its agency, defined as one’s ability to perform actions and engage in the environment (Dehn and Van Mulken, 2000; Hartmann, 2008; Thue et al., 2011). In HMC literature, agency has been typically classified into two categories—human agency and machine agency (Fox et al., 2015; Sundar, 2020). According to Bandura (2001), “to be an agent is to intentionally make things happen by one’s actions (p.2).” For a long time, agency had been equivalent to human agency (Davies, 1991). In recent years, machines are increasingly capable of acting autonomously and adaptively to achieve specific goals with minimum human interference (Sundar, 2020). According to its type of agency, a virtual character can be categorized as a human agent or a machine agent. Specifically, human-agent virtual characters are fundamentally driven by humans (e.g., avatars), whereas machine-agent virtual characters are fundamentally driven by machines (e.g., artificial agents).
It should be noted that since agency is essentially a “temporally embedded process of social engagement” which must be placed “within the contingencies of the moment” (Emirbayer and Mische, 1998, p.1), the agency of a certain virtual character must be determined in accordance with its status at a given moment. For example, since the dance movements and singings of the Chinese Vocaloid character, Luo Tianyi are pre-programmed using computer software, it is a machine agent when it performs on stage; but when it “talks” to the audience in live concerts or live streaming, it is actually live voice-acted by humans and therefore should be considered as a human agent. However, it does not mean that Luo Tianyi is a mixture of both human agency and machine agency because it cannot have both types of agencies at the same time at a given moment. Taken together, to specify the agency of a particular virtual character, we must specify the time of its actions that we are interested in.
4.2.2 Representation
The other dimension of virtual characters pertains to how they are represented. Compared to the agency of virtual characters, which users may not have first-hand knowledge about, the representation of virtual characters can be directly experienced by users. In other words, from the users’ perspective, the agency of a virtual character must be perceived by detecting and interpreting cues in their experience with the virtual characters, including cues in the physical representation of virtual characters. Therefore, it is the representation of a virtual character that influences users’ “perceived agency” judgement of the virtual characters, which has a greater impact on users than its actual agency (Fox et al., 2015). Although the representation of virtual characters can encompass numerous specific sub-dimensions, the technical aspect of the representation of virtual characters is important as it addresses whether the virtual characters are physically embodied, virtually embodied, or disembodied. Physically embodied virtual characters are represented with “a physical instantiation, a body” (Pfeifer and Scheier, 2001, p.649) that can be experienced by the human tactile sensory systems (e.g., robots). Digitally embodied virtual characters are represented with digital appearances, which can be sensed by humans visually but not tactilely (e.g., chatbots with digital bodies). Disembodied virtual characters, on the contrary, do not have any kind of visual representations of faces or bodies (e.g., Apple’s Siri).
A typology of virtual characters with the two fundamental dimensions is shown in Table 1.
5 Perceived authenticity as a core concept
To come up with a unified theoretical framework to understand people’s experience with virtual characters, perceived authenticity is an essential concept. First, the “virtual” nature of virtual characters often invites concerns about perceived authenticity. Although the term “virtual” is often associated with “unauthentic” from an ontological perspective (Lee, 2004), people can perceive authenticity in virtuality psychologically. Therefore, perceived authenticity is important to comprehend people’s experience with virtuality. Besides, as virtual experience becomes more and more prevalent in our daily lives (Lee, 2004; Rahwan et al., 2019), our used-to-be paralleled lives in the virtual and actual world are getting integrated seamlessly (Lee, 2004; Deuze, 2011; Yao and Ling, 2020). In this process, our perception of “what is real” can easily change, which in turn may influence the ways we perceive the world and our relationships with others (Turkle, 2011; Enli, 2014). This is particularly true for children who grew up in a culture where “human contact is routinely replaced by virtual life, computer games, and relational artifacts (Turkle, 2007, p. 514).” They may not necessarily see a living organism as more “alive” than its digital representation or simulation. For example, some children thought animated turtles were more authentic than real ones in museum exhibitions (Turkle, 2007; Turkle, 2011). In these cases, “the idea of the ‘original’ is in crisis” (Turkle, 2007, p.514). Sometimes, ontologically authentic objects (i.e., actual objects) may not be perceived as authentic as virtual objects. Therefore, understanding the mechanism of perceived authenticity in individuals’ experience with virtual characters is necessary.
5.1 Revisiting the concept of perceived authenticity
Originated in philosophy, authenticity has been a complex concept with multiple meanings (Golomb, 1995; Guignon, 2008; Newman and Smith, 2016; Varga and Guignon, 2020). Some earlier philosophers, such as David Hume, used the notion in the sense of genuineness—things being what they claim to be in origin or authorship (Golomb, 1995). Existentialist philosophers, such as Kierkegaard, Nietzche, Heidegger, and Sartre, used authenticity mainly in the sense of being truthful to oneself with a focus on questioning social norms and public roles while creating one’s selfhood (Golomb, 1995; Wang, 1999; Newman and Smith, 2016; Varga and Guignon, 2020). Authenticity has also been conceptualized as a virtue on the sincere expression of values, beliefs, and ideas (Dutton, 2003; Guignon, 2008; Newman and Smith, 2016). Despite the differences among different uses of authenticity, at the most common-sensical level, authenticity is a term that “captures dimensions of truth and verification” (Newman and Smith, 2016, p. 610).
In communication literature, the concept of authenticity has its particular focus. Although the meaning of authenticity may be ontological in the field of philosophy, in the context of media, the meaning of authenticity is essentially psychological—authenticity only exists in the “perceived” form. Enli (2014) uses the term “mediated authenticity” to capture the uniqueness of authenticity in the context of media by stating that it “traffics in representations of reality” (p. 1). It exists in the communicative process of the “symbolic negotiations between the participants in the communication” (Enli, 2005). In addition, unlike the concept of authenticity in philosophy, (perceived) authenticity in communication literature is rarely used to evaluate the self. In other words, authenticity in the context of media mostly concerns perceived authenticity of various objects (e.g., things, people, information, and actions) other than self, which also applies to people’s experience with virtual characters.
5.1.1 Components of perceived authenticity
In Enli’s conceptualization, mediated authenticity comprises three aspects: trustworthiness, originality, and spontaneity. Trustworthiness pertains to whether the communicated information is factually correct and accurate. Originality refers to something being genuine and original, as opposed to manipulations and copies. Spontaneity concerns whether the actions in the communication process are natural and unscripted. Enli (2014) also suggested that every time a new media technology is introduced to people’s daily communication, the three aspects of mediated authenticity will be redefined in this process.
Lee (2020) further developed Enli’s conceptualization by summarizing that (perceived) authenticity of communication comprises three components: perceived authenticity of source, perceived authenticity of message, and perceived authenticity of interaction. Perceived authenticity of source pertains to the veracity of the source’s identity—whether the source’s actual identity is consistent with its claimed identity. Perceived authenticity of message concerns whether people perceive the content of communication to be true—specifically, whether “a message truthfully represents its objects, be it an event, a person (including the source him/herself), or an issue” (Lee, 2020, p. 62). Perceived authenticity of interaction pertains to whether people “feel they are a part of actual interaction, which transforms a detached audience as in conventional mass communication into a participant in the communication process” (Lee, 2020, p. 63).
In Lee’s conceptualization, perceived authenticity “may boil down to ‘perceived typicality’ of the object” (p. 64), which is determined by whether a certain object (i.e., the source, the message, or the interaction in the communication process) is perceived as congruent with individuals’ expectancies on the object. And these expectancies are based on a number of comprehensive factors such as individuals’ cognitive schemas, stereotypes, past experience, prior knowledge, and the reciprocity and spontaneity of the interaction. Meeting the expectancies increases individuals’ degree of perceived authenticity. When the expectancies are violated, if the individuals have low accuracy motivation, such as low issue involvement or low need for cognition, they may perceive lower authenticity; however, if they have high accuracy motivation, they may look for particular authenticity markers such as source expertise and cues for consensus to determine the degree of perceived authenticity.
Based on the three components of perceived authenticity, Lee (2020) proposed a comprehensive authenticity model of (mass-oriented) computer mediated communication, suggesting that individuals’ perceived authenticity of source, message, and interaction are positively affected by the satisfaction of their expectancies on source, message, and interaction respectively, and subsequently influence individuals’ baseline cognitive, affective, or behavioral reactions. Specifically, perceived authenticity amplifies baseline affective outcomes and intended behavioral change; perceived source authenticity and perceived message authenticity intensify baseline cognitive outcomes, while perceived interaction authenticity interferes with baseline cognitive outcomes.
5.1.2 Similar concepts to perceived authenticity
In communication literature, perceived authenticity seems to share similar conceptual concerns with several notions such as credibility, perceived realism, presence, and para-social interaction. However, these concepts are either distinct from perceived authenticity theoretically or represent only partials of perceived authenticity in particular contexts.
Among the concepts similar to perceived authenticity, credibility is the most notable one (Lee, 2020). As a concept that has been extensively studied in conventional mass communication research, credibility has been conceptualized as source credibility, message credibility, and medium credibility (Metzger et al., 2003; Sundar, 2008). Source credibility refers to an individual’s judgment on the believability of a communicator, which is often operationalized as perceived trustworthiness and expertise of the source of a message (Wilson and Sherrell, 1993); message credibility refers to judgments made by individuals concerning the veracity of the content of communication (Appelman and Sundar, 2016); medium credibility pertains to the believability of a given medium or channel of message delivery (Metzger et al., 2003). And these three aspects of credibility are often confounded with each other (Appelman and Sundar, 2016). Among the three aspects of credibility, message credibility does conceptually overlap with perceived message authenticity, both concerning the veracity of message in communication (Lee, 2020). However, perceived message authenticity goes beyond the factuality of the message and also comprises “perceived transparency of the source’s professed goals and intent” (Lee, 2020, p. 62), which is not entailed in message credibility. In addition, the other two aspects of credibility are distinct from other aspects of perceived authenticity theoretically. Distinct from source credibility, perceived authenticity of source focuses on the veracity of claimed identity of the source (i.e., whether the communicator is really who he/she claims to be) rather than qualities of the source such as trustworthiness, degree of authority, or expertise as in source credibility. The uniqueness of perceived source authenticity echoes with the increasing concerns over fake/mistaken identity issues due to the permeation of mediated communication and advances in technologies like deep-fake and AI (McMurry, 2018; Solon, 2018; Vaccari and Chadwick, 2020). Similarly, neither perceived authenticity of interaction, nor medium credibility has its conceptual counterpart in the other notion. To summarize, although credibility and perceived authenticity do share some commonalities in terms of evaluating messages in communication, they are two distinct concepts with different research focuses.
Perceived realism is also a notion that is similar to perceived authenticity. However, the concept of perceived authenticity is broader than perceived realism. In the field of communication, perceived realism is mostly studied in the context of narratives research, particularly in studies on the experience of transportation and identification (Green and Brock, 2000; Busselle and Bilandzic, 2008). According to Busselle and Bilandzic (2008), perceived realism comprises external realism and narrative realism. The former concerns the degree of similarity between the story world and the actual world, while the latter concerns the coherence within the story world. Both types of perceived realism are distinct from the fictionality judgment—a perceiver’s knowledge of whether the story is fictional does not affect his or her perceived realism judgment; instead, it is whether the story matches their expectancies that matters (Busselle and Bilandzic, 2008; Lee, 2020). Perceived realism is similar to perceived authenticity in that both pertain to one’s subjective judgment of what is real. However, perceived authenticity applies to broader research contexts beyond the research on narratives. In addition, the concept of perceived authenticity also entails one’s judgment on factuality or fictionality, which is excluded in perceived realism.
Presence is another concept that has some conceptual commonalities with perceived authenticity, particularly in the contexts of CMC and HCI. Presence is defined as “a psychological state in which virtual objects are experienced as actual objects in either sensory or nonsensory ways” (Lee, 2004, p. 27). Its emphasis on “actual-like” experience refers to perceived non-mediation or perceived non-artificiality of the experience (Biocca, 1997; Lombard and Ditton, 1997; Lombard et al., 2000; Lee, 2004). According to Lee (2004), there are three types of presences—physical presence, social presence, and self-presence, in which virtual physical objects, virtual social actors, and virtual selves are experienced as actual ones, respectively. In a sense, presence and perceived authenticity share the same core concerns—a feeling of “realness.” However, these two concepts are qualitatively different from each other by definition. Presence is a particular psychological state of individuals themselves in particular experience, while perceived authenticity is a complex judgment made by individuals on particular objects. To be specific, presence is an individual’s psychological state of experiencing virtual objects as if they were actual objects, which emphasizes perceived “non-mediation” or perceived “non-artificiality” of individuals’ experience (Biocca, 1997; Lombard and Ditton, 1997; Lee, 2004). By contrast, perceived authenticity is a complex judgmental perception of source, message, and interaction regarding veracity, sincerity, and realness (Lee, 2020). All three types of presence seem to correspond with the notion of perceived authenticity of interaction—individuals’ perception of being part of actual interactions (Lee, 2020). However, they do not reflect perceived source authenticity or perceived message authenticity. For example, when people are aware of the mediation or simulation, which leads to low degrees of presence, they might still feel the entire experience is authentic and meaningful. In addition, presence comprises self-presence, while perceived authenticity does not concern judgement on the self. Taken together, presence and perceived authenticity are also two distinctive notions, although elements of social presence conceptually overlap with perceived interaction authenticity.
In a word, although some other similar concepts, such as credibility, perceived realism, and presence, also capture certain aspects of perceived authenticity, they are not as suitable as perceived authenticity in examining people’s subjective experience of “realness” in a comprehensive way. For example, credibility cannot be used to study individuals’ perceived authenticity of interaction, perceived realism cannot be used outside the narrative context, and presence cannot be used to evaluate perceived authenticity of message. In summary, in communication literature, perceived authenticity is a unique unifying concept with a focus on people’s subjective experience of “realness.”
5.2 Perceived authenticity in people’s experience with virtual characters
Despite that prior research on various virtual characters has touched on certain aspects of perceived authenticity in particular ways, few have taken perceived authenticity as a unifying concept in understanding people’s experience with them in a comprehensive way (Lee, 2020).
In conventional HCI frameworks under which people’s experience with machine-controlled virtual characters has been mostly studied, “realness” often equals “human-likeness,” which pertains to whether a machine feels like a real human to users (Turing, 1950; Weizenbaum, 1966; de Borst and de Gelder, 2015; Shum et al., 2018; Chaves and Gerosa, 2019). However, as machine agents are becoming more ubiquitous in human life (Rahwan et al., 2019), they are increasingly conceived as distinctive communicators rather than mere channels of communication (Guzman and Lewis, 2020). In this process, people’s expectations and perceptions of machine-controlled virtual characters and their relationships with them can also evolve (Turkle, 2007; Turkle, 2011; Sundar, 2020), which invites more holistic research on perceived authenticity. Specifically, as people are becoming more acquainted with interacting with machine-controlled virtual characters, their heuristics about machine agency and their relationships with machines can evolve to be unique and yet meaningful ones (Turkle, 2007; Sundar, 2020). For example, people may find machines more secure and credible than humans in some cases (Sundar and Kim, 2019). In other words, sometimes machine agents may not necessarily need to act like humans to be perceived as authentic as long as they satisfy individuals’ heuristics about machines. In these cases, perceived authenticity can be a particularly useful concept because it captures the complexity and multiple dimensions of “realness” in people’s experience with various virtual characters, including both machine-controlled and human-controlled virtual characters.
Although Lee’s (2020) conceptualization of perceived authenticity was proposed in the context of mass-oriented computer-mediated communication, it can also provide a preliminary framework to understand people’s experience with virtual characters. Perceived authenticity in people’s experience with virtual characters can also be examined from three aspects: whether the identity of the virtual character that they interact with feels authentic to people (i.e., perceived source authenticity), whether what the virtual character says feels true to them (i.e., perceived message authenticity), and whether the interaction with the virtual character feels like an actual interaction to them (i.e., perceived interaction authenticity). Nevertheless, scrutinizing people’s experience with virtual characters reveals some unique characteristics of perceived authenticity in this context, particularly with perceived source authenticity, which will be discussed in the following section.
5.2.1 Multi-layered perceived source authenticity
The most distinctive characteristic of perceived authenticity in people’s experience with virtual characters in comparison with the general version of perceived authenticity is that perceived authenticity of source in this context is always multi-layered.
According to Lee (2020), perceived authenticity of source in communication refers to the veracity of the claimed identity of the source (Lee, 2020). However, the model seemed to have simplified the complexity of sources in many communication contexts. For example, information transmitted through computer-mediated communication often has multiple layers of sources, resulting in “a confusing multiplicity of sources of varying levels of perceived credibility” (Sundar, 2008, p.73). In other words, when determining perceived source authenticity, individuals much distinguish which layer of source they are referring to.
This is particularly true for people’s experience with virtual characters because a virtual character as a multi-dimensional concept always has multiple layers of identities. Since agency and representation are two dimensions of virtual characters, the identity of a particular virtual character naturally also has at least two layers. The first layer of its identity concerns agency, whether the virtual character is controlled by a human or a machine. The second layer of its identity contains multiple sub-layers concerning various specific identities related to its representation, such as the social-group identities represented by the virtual character (e.g., gender, race, and profession of an animated game character) and the identifiable individual identity related to the representation of the virtual character (e.g., an animated Barack Obama).
As a result, perceived source authenticity of a virtual character also comprises at least two basic layers: perceived authenticity of the virtual character’s claimed agency, and perceived authenticity of the identities of the virtual character’s representation. Perceived authenticity of agency pertains to whether individuals perceive a virtual character’s agency matches its claimed agency. For example, when a virtual character is labeled as or claims itself to be a human agent, people may feel it is indeed a human agent (high perceived source authenticity in terms of agency) or is faked by a machine agent (low perceived source authenticity in terms of agency). Perceived authenticity of represented identity pertains to whether individuals perceive the specific social or individual identity of a virtual character’s representation matches with their judgement of its “actual” social or individual identity. For example, if a virtual influencer identifies herself as a liberal, people can read his or her posts and judge whether he or she really is liberal.
5.3 A multi-layered model of perceived source authenticity for people’s experience with virtual characters
Because of the unique characteristics of perceived source authenticity in people’s experience with virtual characters, a multi-layered model of perceived authenticity of source is proposed based on Lee’s authenticity model (Lee, 2020). Specifically, the multi-layered identity of virtual characters and subsequent multi-layered perceived source authenticity should be reflected in the modified authenticity model for people’s experience with virtual characters. The antecedents of different layers of perceived source authenticity, cues that may have a major impact on people’s authenticity judgment should be examined to advance theoretical and practical research. As such a multi-layered model of perceived authenticity of source for people’s experience with virtual characters is proposed, as shown in Figure 2.
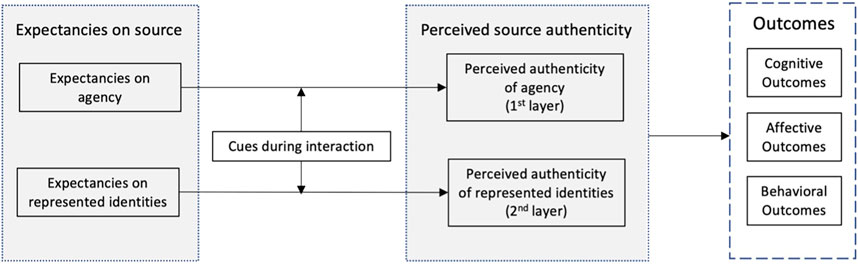
FIGURE 2. A multi-layered model of perceived source authenticity in people’s experience with virtual characters.
The proposed model argues that perceived authenticity of source in people’s experience with virtual characters should be examined in two layers: the first layer pertains to its agency; and the second layer pertains to the social identities of the representation of the virtual character. In the original authenticity model, Lee proposed that the confirmation of people’s expectancies regarding the identity of the source can enhance perceived source authenticity and subsequent outcomes (Lee, 2020). This argument is actually in line with a core argument in Expectancy Confirmation Theory (ECT) that expectations act on satisfaction via later perceptions such as perceived performance and disconfirmation of beliefs (Oliver, 1976; Oliver, 1980; Hossain and Quaddus, 2012). In line with the logic of ECT, Lee’s authenticity model suggested that expectancies act on people’s perceived authenticity via the confirmation of expectancies through authenticity markers such as consensus cues (Lee, 2020). In other words, it is the consistency between people’s expectancies of source and the information and cues they perceived in the communication process that determines perceived source authenticity. Following the same logic, in our proposed model, the consistency between individuals’ expectancies about a virtual character’s claimed agency and cues they perceive during the interaction with virtual characters would enhance perceived authenticity of the virtual characters’ claimed agency; and the consistency between individuals’ expectancies about a virtual character’s represented identities and cues they perceive during the interaction with virtual characters will enhance perceived authenticity of the virtual characters’ represented identity. And the two layers of perceived authenticity of source together may determine people’s overall perceived source authenticity with the virtual characters, and subsequently influence the cognitive, affective, and behavioral outcomes.
In other words, for a virtual character to be perceived as authentic, there are two aspects to consider. On the first layer, if a virtual character is claimed/labeled as machine- or human-controlled, showing characteristics or cues that match people’s expectancies of what a machine or a human should look or act like shall enhance its perceived source authenticity in terms of agency. On contrary, violating these expectancies will reduce perceived source authenticity. For example, introducing a chatbot as an avatar shall induce people’s expectancies about human-human communication, and raise people’s expectations of interactivity because people have higher expectancies for human-human communication than for human-machine communication (Go and Sundar, 2019). As a result, if the chatbot subsequently shows low interactivity, perceived source authenticity will be derogated. However, it should be noted that people’s heuristic about machines, defined as “the mental shortcut wherein we attribute machine characteristic or machine-like operation when making judgements about the outcome of an interaction” (Sundar and Kim, 2019, p. 539), have been and will continue to be evolving, particularly with the increasing capability of AI (Sundar, 2020; Yang and Sundar, 2020). Although the word “machine-likeness” has conveyed some pejorative meanings associated with low performance in many contexts, the application of AI in various fields of human life has been re-shaping people’s perception of machines (Burmester et al., 2019; Muresan and Pohl, 2019; Rahwan et al., 2019). In some contexts, machines are perceived as possessing “intelligence that is not just human-like, but surpasses human abilities” (Sundar, 2020, p. 79). In these contexts, being “machine-like” may not necessarily contain negative connotation. Instead, it may activate individuals’ positive machine heuristics and may affect the interaction outcomes in a positive way. In such situations, being perceived as an “authentic” machine may particularly enhance the positive effect of machine heuristics on cognitive, affective, and behavioral outcomes.
On the second layer, to enhance perceived source authenticity, the cues from a virtual character should also be consistent with the expectancies associated with the virtual character’s represented social or individual identities. Specifically, when a virtual character is represented as a certain social role or character, its subsequent behavioral cues need to match its representation. It should be noted that such representation includes not only visual representations, more associated with physically embodied virtual characters, but also auditory representations and nominal representations such as a simple name, which is more associated with disembodied virtual characters. For example, Daodao (as shown in Figure 3), a Chinese bookkeeping and social networking application uses chatbots as bookkeeping companions for its users, in which users may search and select a celebrity character like a pop idol, a movie star, a game or anime character, as their daily bookkeeping companion, and set up a social role, such as a boyfriend, girlfriend, brother, or sister. The corpus of each celebrity bot has combined both 1) input from both the content team who produce individualized chats for each celebrity figure according to their public personae, and 2) “crowdsourced” input from fans who really know how their idols would act (Tsang, 2019). From the lens of the proposed multi-layered model of perceived source authenticity, the level of authenticity perceived by the users related to a particular celebrity bot happens mainly on the second layer of perceived source authenticity. Users have certain expectancies on particular celebrity characters and their assigned social roles, and auto-replies in congruent with those celebrity characters and their social roles can satisfy those expectancies and thus enhance perceived source authenticity in terms of the virtual character’s represented identities.
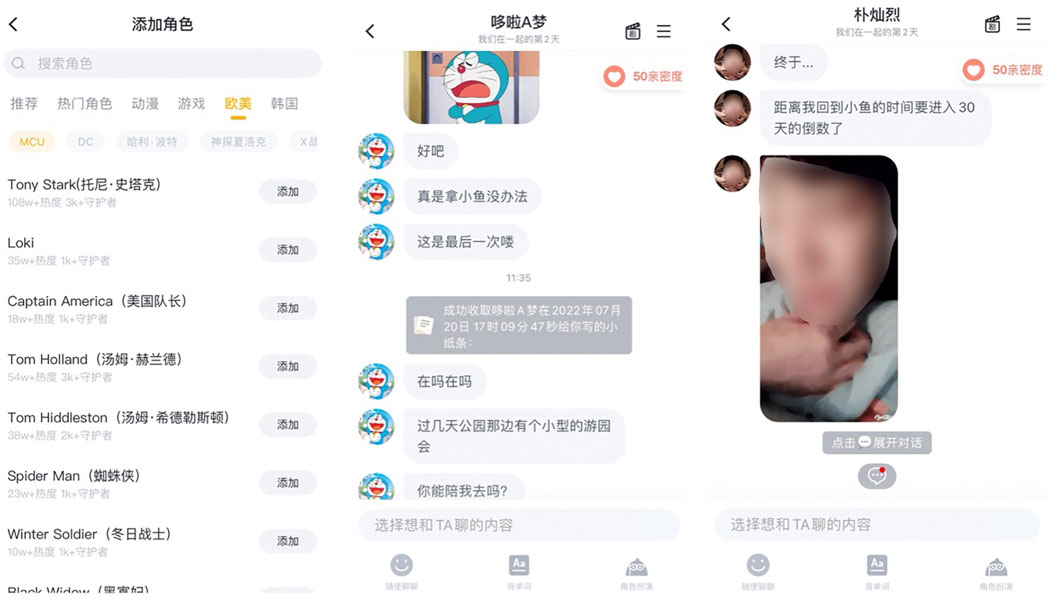
FIGURE 3. Screenshots from the Chinese application Daodao. From left to right: the “adding character” page where users may select a variety of virtual characters such as movie characters, stars, and virtual celebrities; a screenshot from chats with the anime character Doraemon; a screenshot from chats with a Korean Pop idol character.
Although these two layers together shaped individuals’ overall perceived authenticity of source and the subsequent cognitive, affective, and behavioral outcomes, the magnitude of the influence of the two layers may differ according to specific contexts. Specifically, in contexts where machine agency or human agency has an overt advantage—for example, machines are generally considered to be more reliable in terms of handling large qualitative data than human beings (Yang and Sundar, 2020), perceived source authenticity in terms of claimed/labeled agency (i.e., the first layer) shall have a greater impact on the overall perceived authenticity of source. On the other hand, in contexts where people are more drawn to the virtual character’s represented identities, perceived source authenticity in terms of the virtual character’s represented social/individual identities (i.e., the second layer) shall have a greater impact on the virtual character’s overall perceived authenticity of source. In other words, the two layers of perceived authenticity of source might have different degrees of impact on the overall perceived source authenticity, depending on which layer of source identity plays a more important role in a particular interaction with a specific virtual character.
To summarize, this manuscript proposes that machine agents may not necessarily need to be perceived as humans to be perceived as authentic as long as they satisfy individuals’ heuristics about machines and/or the virtual character’s represented identities. According to the proposed model, a machine-like machine is more likely to be perceived as authentic than a machine that pretends to be a human as perceived authenticity of virtual characters is determined by the two layers of source authenticity, regardless of the ontological nature of such virtual characters.
6 Concluding remarks and future research
Since their invention, virtual characters have been studied extensively under myriad theoretical frameworks in various fields. Despite the accumulated knowledge about virtual characters, understandings of people’s experiences with them are dispersed in separate fields, mostly based on the virtual characters’ ontological nature. As technology advances, both the quantity and complexity of virtual characters are rising drastically, which demands more comprehensive research frameworks. The current manuscript intends to initiate such efforts by proposing a two-dimensional typology of virtual characters and a multi-layered model with a focus on perceived source authenticity.
The proposed typology and the model can be used in several ways. The typology can be used as a guide to identify the category of virtual characters in a given context, which helps hypothesize the expectancies and associated cues. Then, using the multi-layered model of perceived source authenticity, one may examine which layer of perceived source authenticity matters more in that specific context, which can help guide or modify the design of a particular virtual character. For example, if it is found that the second layer of perceived source authenticity of a virtual streamer (e.g., perceived source authenticity in terms of its represented identities such as a certain profession) has a greater impact on the overall perceived source authenticity and subsequent outcomes such as recall of information delivered by the virtual streamer (cognitive outcome), likeness of the virtual streamer (affective outcome), and audience engagement (behavioral outcome), it implies that people may not care whether this virtual streamer is ontologically a human or a machine. Then, resources probably should not be wasted in making this virtual streamer look as human-like as possible. Instead, it should prioritize identifying typical cues or pivotal markers that make the virtual streamer’s represented social and individual identities in the virtual environment feel authentic to its audience (e.g., typical cues related to the virtual streamer’s represented profession). And hypotheses in which 1) such potential cues are independent variables, 2) expected outcomes are dependent variables, and 3) two layers of perceived source authenticity are mediators, can be proposed and tested empirically for this scenario.
As a simplified model to initiate more comprehensive research on complicated virtual characters, the multi-layer model of perceived source authenticity has some limitations. One is that it does not comprise the other two aspects of perceived authenticity, perceived authenticity of message and perceived authenticity of interaction. Since Lee’s authenticity model is a preliminary model, the relationships among perceived authenticity of source, message, and interaction still need further investigation (Lee, 2020). Like other communication processes, people’s experience with virtual characters is often a continuous process of making judgments on the source, the messages, and the interaction. In this process, their judgment can also be changed and adjusted as communication deepens. Specifically, as first impression plays an important role in initiating and maintaining relationships, the first impression of virtual characters may influence users’ initial perception of virtual characters and subsequent assessment of their interaction with the virtual characters. In this sense, perceived source authenticity is essential in determining the first impression of virtual characters, which may subsequently influence the other types of perceived authenticity. Nevertheless, future research may look for ways to add the other two aspects of perceived authenticity to an extended version of the currently proposed model.
As a final remark, perceived authenticity of virtual characters attracts more interests from both the academia and industry as virtual characters play an increasingly essential role in our daily lives. Perceived authenticity is a concept that may bridge the traditionally separated research on virtual characters across various disciplines, based on their ontological nature. The proposed typology of virtual characters and the multi-layered model of perceived authenticity sheds some lights on how to take a holistic approach to understand emerging virtual characters and human experience with them as a meaningful first step.
Data availability statement
The original contributions presented in the study are included in the article/Supplementary Material, further inquiries can be directed to the corresponding authors.
Author contributions
JH and YJ have collaborated on this manuscript. The order of the authors reflects the amount of work put into the manuscript. Specifically, JH: original idea of the concept, most of the writing; YJ: advising and editing.
Acknowledgments
We would like to thank Lee Kwan Min, Benjy Lee, and Xu Hong for their insightful feedback on an earlier version of this manuscript.
Conflict of interest
The authors declare that the research was conducted in the absence of any commercial or financial relationships that could be construed as a potential conflict of interest.
Publisher’s note
All claims expressed in this article are solely those of the authors and do not necessarily represent those of their affiliated organizations, or those of the publisher, the editors and the reviewers. Any product that may be evaluated in this article, or claim that may be made by its manufacturer, is not guaranteed or endorsed by the publisher.
Supplementary material
The Supplementary Material for this article can be found online at: https://www.frontiersin.org/articles/10.3389/frvir.2022.1033709/full#supplementary-material
Footnotes
1“Uncanny Valley” (Mori et al., 2012) refers to the phenomenon that people’s positive response to human-like robots would abruptly shift negatively as the robots approach but fail to achieve a perfect life-like level
2Screenshot of video retrieved on 24 March 2021 from https://www.bilibili.com/bangumi/play/ss29067/?from=search&seid=12930183317510447104
References
Appelman, A., and Sundar, S. S. (2016). Measuring message credibility: Construction and validation of an exclusive scale. J. Mass Commun. Q. 93 (1), 59–79. Available at:. doi:10.1177/1077699015606057
Bandura, A. (2001). Social cognitive theory: An agentic perspective. Asian J. Soc. Psychol. 52, 21–41. doi:10.1111/1467-839x.00024
Bemelmans, R., Gelderblom, G. J., Jonker, P., and de Witte, L. (2012). Socially assistive robots in elderly care: A systematic review into effects and effectiveness. J. Am. Med. Dir. Assoc. 13 (2), 114–120.e1. doi:10.1016/j.jamda.2010.10.002
Biocca, F. (1997). The cyborg’s dilemma: Progressive embodiment in virtual environments. J. Comput. Mediat. Commun. 3 (2), 0–29. Available at:. doi:10.1111/j.1083-6101.1997.tb00070.x
Burmester, M., Zeiner, K., Schippert, K., and Platz, A. (2019). Creating positive experiences with digital companions. Conf. Hum. Factors Comput. Syst. - Proc., 1–6. doi:10.1145/3290607.3312821
Busselle, R., and Bilandzic, H. (2008). Fictionality and perceived realism in experiencing stories: A model of narrative comprehension and engagement. Commun. Theory 18 (2), 255–280. doi:10.1111/j.1468-2885.2008.00322.x
Cha, Y. (2018) “Complex and ambiguous: Understanding sticker misinterpretations in instant messaging,” in Proceedings of the ACM on Human-Computer Interaction, 2(CSCW). Available at:. doi:10.1145/3274299
Chaves, A. P., and Gerosa, M. A. (2019). How should my chatbot interact? A survey on human-chatbot interaction design. Available at: http://arxiv.org/abs/1904.02743. (Accessed March 15, 2021).
Conner, T. (2016). “Hatsune Miku, 2.0Pac, and beyond: Rewinding and fast-forwarding the virtual pop star,” in The oxford handbook of music and virtuality. Editors S. W. S. Rambarran (Oxford, UK: Oxford University Press). Available at:. doi:10.1093/oxfordhb/9780199321285.013.8
Conner, T. (2013). Rei toei lives!: Hatsune Miku and the design of the virtual pop star. Master’s Thesis. University of Illinois at Chicago.
Dautenhahn, K. (2003). Roles and functions of robots in human society: Implications from research in autism therapy. Robotica 21 (4), 443–452. Available at:. doi:10.1017/S0263574703004922
Dautenhahn, K. (2007). Socially intelligent robots: Dimensions of human-robot interaction. Phil. Trans. R. Soc. B 362 (1480), 679–704. Available at:. doi:10.1098/rstb.2006.2004
Davies, B. (1991). The concept of agency : A feminist poststructuralist analysis. Int. J. Anthropol. (30), 42–53.
de Borst, A. W., and de Gelder, B. (2015). Is it the real deal? Perception of virtual characters versus humans: An affective cognitive neuroscience perspective. Front. Psychol. 6, 576. Available at:. doi:10.3389/fpsyg.2015.00576
Dehn, D. M., and Van Mulken, S. (2000). The impact of animated interface agents: A review of empirical research. Int. J. Hum. Comput. Stud. 52 (1), 1–22. Available at:. doi:10.1006/ijhc.1999.0325
Deuze, M. (2011). Media life. Media, Cult. Soc. 33 (1), 137–148. Available at:. doi:10.1177/0163443710386518
Dutton, D. (2003). “Authenticity in art,” in The oxford handbook of aesthetics. Editor J. Levinson (Oxford, UK: Oxford University Press), 258–274. Available at:. doi:10.1093/oxfordhb/9780199279456.003.0014
Emirbayer, M., and Mische, A. (1998). What is agency? Am. J. Sociol. 103 (4), 962–1023. Available at:. doi:10.1086/231294
Enli, G. (2014). Mediated authenticity: How the media constructs reality. New York: Peter Lang Incorporated.
Everett, A. (2007). Pretense , existence, and fictional objects. Philos. Phenomenol. Res. 74 (1), 56–80. doi:10.1111/j.1933-1592.2007.00003.x
Fischer, K., Foth, K., Rohlfing, K. J., and Wrede, B. (2011). Mindful tutors: Linguistic choice and action demonstration in speech to infants and a simulated robot. Interact. Stud. 12 (1), 134–161. Available at:. doi:10.1075/is.12.1.06fis
Fox, J., Ahn, S. J. G., Janssen, J. H., Yeykelis, L., Segovia, K. Y., and Bailenson, J. N. (2015). Avatars versus agents: A meta-analysis quantifying the effect of agency on social influence. Human–Computer. Interact. 30 (5), 401–432. Available at:. doi:10.1080/07370024.2014.921494
Franklin, S., and Graesser, A. (1996). “Is it an agent, or just a program?: A taxomony of autonomous agents’,” in International workshop on agent theories, architectures, and languages, 215.
Go, E., and Sundar, S. S. (20192018). Humanizing chatbots: The effects of visual, identity and conversational cues on humanness perceptions. Comput. Hum. Behav. 97, 304–316. doi:10.1016/j.chb.2019.01.020
Golomb, J. (1995). In search of authenticity: From Kierkegaard to Camus. London: Routledge. doi:10.1017/CBO9781107415324.004
Green, M. C., and Brock, T. C. (2000). The role of transportation in the persuasiveness of public narratives. J. Personality Soc. Psychol. 79 (5), 701–721. doi:10.1037/0022-3514.79.5.701
Guignon, C. (2008). Authenticity. Philos. Compass 2 (3), 277–290. doi:10.1111/j.1747-9991.2008.00131.x
Gulz, A. (2005). Social enrichment by virtual characters - differential benefits. J. Comput. Assist. Learn. 21 (6), 405–418. doi:10.1111/j.1365-2729.2005.00147.x
Guzman, A. L., and Lewis, S. C. (2020). Artificial intelligence and communication: A human–machine communication research agenda. New Media Soc. 22 (1), 70–86. Available at:. doi:10.1177/1461444819858691
Guzman, A. L. (Editor) (2018). “What is human-machine communication, anyway?” Human-machine communication: Rethinking communication, technology, and ourselves (New York: Peter Lang), 1.
Hartmann, T. (2008). “Parasocial interactions and paracommunication with new media characters,” in Mediated interpersonal communication. Editor E. A. Konjin (New York: Routledge), 177–199. doi:10.4324/9780203926864
Holz, T., Dragone, M., and O’Hare, G. M. P. (2009). Where robots and virtual agents meet. Int. J. Soc. Robot. 1 (1), 83–93. doi:10.1007/s12369-008-0002-2
Hoorn, J. F., and Koinijn, E. A. (2003). Perceiving and experiencing fictional characters: An integrative account. Jpn. Psychol. Res. 45 (4), 250–268. doi:10.1111/1468-5884.00225
Hossain, M. A., and Quaddus, M. (2012). “Expectation–confirmation theory in information system research: A review and analysis,” in Information systems theory: Explaining and predicting our digital society. Editors Y. K. Dwivedi, M. R. Wade, and S. L. Schneberger (New York: Springer), 441–469. doi:10.1007/978-1-4419-6108-2
Jaderberg, M., Czarnecki, W. M., Dunning, I., Marris, L., Lever, G., Castaneda, A. G., et al. (2019). Human-level performance in 3D multiplayer games with population-based reinforcement learning. Science 364 (6443), 859–865. doi:10.1126/science.aau6249
James, E. (2019). Nonhuman fictional characters and the empathy-altruism hypothesis. Poet. Today 40 (3), 579–596. doi:10.1215/03335372-7558164
Jenkins, M. (2018). This singer is part hologram, part avatar, and might be the pop star of the future - the Washington Post, the Washington Post. Available at: https://www.washingtonpost.com/entertainment/music/this-singer-is-part-hologram-part-avatar-and-might-be-the-pop-star-of-the-future/2018/07/05/e2557cdc-7ed3-11e8-b660-4d0f9f0351f1_story.html. (Accessed March 15, 2021).
Kanda, T., Miyashita, T., Osada, T., Haikawa, Y., Ishiguro, H., et al. (2008). Analysis of humanoid appearances in human-robot interaction. IEEE Transactions on Robotics, 24 (3), 725–735.
Kang, H. S., and Yang, H. D. (2006). The visual characteristics of avatars in computer-mediated communication: Comparison of internet relay chat and instant messenger as of 2003. Int. J. Hum. Comput. Stud. 64 (12), 1173–1183. doi:10.1016/j.ijhcs.2006.07.003
Keen, S. (2011). Readers’ temperaments and fictional character. New Lit. Hist. 42 (2), 295–314. doi:10.1353/nlh.2011.0013
Kellems, R. O., Charlton, C., Kversoy, K. S., and Gyori, M. (2020). Exploring the use of virtual characters (Avatars), live animation, and augmented reality to teach social skills to individuals with autism. Multimodal Technol. Interact. 4 (3), 48–11. doi:10.3390/mti4030048
Kiskola, J. (2018). Virtual animal characters in future communication: Exploratory study on character choice and agency. Master’s Thesis. University of Tampere.
Lam, K. Y. (2016). The Hatsune Miku phenomenon: More than a virtual J-pop diva. J. Pop. Cult. 49 (5), 1107–1124. doi:10.1111/jpcu.12455
Leavitt, A., Knight, T., and Yoshiba, A. (2016). “Producing Hatsune Miku: Concerts, commercialization, and the politics of peer production,” in Media convergence in Japan. Editors P. W. Galbraith, and J. G. Karlin (Kinema Club), 200.
Lee, E.-J. (2020). Authenticity model of (Mass-Oriented) computer-mediated communication: Conceptual explorations and testable propositions. J. Comput. Mediat. Commun. 25 (1), 60–73. doi:10.1093/jcmc/zmz025
Lee, K. M., and Jung, Y. (2005). Evolutionary nature of virtual experience. J. Cult. Evol. Psychol. 3 (2), 159–176. Available at:. doi:10.1556/jcep.3.2005.2.4
Lee, K. M., Jung, Y., Kim, J., and Kim, S. R. (2006). Are physically embodied social agents better than disembodied social agents?: The effects of physical embodiment, tactile interaction, and people’s loneliness in human-robot interaction. Int. J. Hum. Comput. Stud. 64 (10), 962–973. doi:10.1016/j.ijhcs.2006.05.002
Lee, K. M., and Nass, C. (2003). Designing social presence of social actors in human computer interaction. Conf. Hum. Factors Comput. Syst. - Proc. (5), 289–296. doi:10.1145/642611.642662
Lee, K. M. (2004). Presence , explicated. Commun. Theory 14 (1), 27–50. doi:10.1111/j.1468-2885.2004.tb00302.x
Lee, O., and Shin, M. (2004). Addictive consumption of avatars in cyberspace. Cyberpsychol. Behav. 7 (4), 417–420. doi:10.1089/cpb.2004.7.417
Lombard, M., Ditton, T., Crane, D., Davis, B., Gil-Egui, G., and Horvath, K. (1997). At the heart of it all: The concept of presence. J. Comput. Mediat. Commun. 3 (2), 0. Available at:. doi:10.1111/j.1083-6101.1997.tb00072.x
Lombard, M., Ditton, T., Crane, D., Davis, B., Gil-Egui, G., Horvath, K., Rossman, J., et al. (2000). “Measuring presence: A literature-based approach to the development of a standardized paper-and-pencil instrument,” in Presence 2000: The third international workshop on presence, 2–4.
Luo, X., Tong, S., Fang, Z., Qu, Z., et al. (2019). Machines versus humans: The impact of AI chatbot disclosure on customer purchases xueming Luo, siliang tong, zheng fang, and zhe qu june 2019 forthcoming. Markting Science, 38 (6), 937–947.
Marsh, C. (2016). We attended the Hatsune Miku expo to find out if a hologram pop star could Be human, vice. Available at: https://www.vice.com/en/article/ae87yb/hatsune-miku-expo-feature (Accessed March 15, 2021).
Maslej, M. M., Oatley, K., and Mar, R. A. (2017). Creating fictional characters: The role of experience, personality, and social processes. Psychol. Aesthet. Creativity, Arts 11 (4), 487–499. Available at:. doi:10.1037/aca0000094
McMurry, E. (2018). A fake Warren Buffett Twitter account is giving life advice, and people are listening. ABC News. August, 28. Available at: https://abcnews.go.com/US/fake-warren-buffett-twitter-account-giving-life-advice/story?id=57424237 (Accessed May 20, 2021).
Metzger, M. J., Flanagin, A. J., Eyal, K., Lemus, D. R., and Mccann, R. M. (2003). Chapter 10: Credibility for the 21st century: Integrating perspectives on source, message, and media credibility in the contemporary media environment. Commun. Yearb. 27 (1), 293–335. doi:10.1207/s15567419cy2701_10
Mori, M., MacDorman, K. F., and Kageki, N. (2012). The uncanny valley [from the field]. IEEE Robot. Autom. Mag. 19 (2), 98–100. Available at:. doi:10.1109/MRA.2012.2192811
Mou, Y., and Xu, K. (2017). The media inequality: Comparing the initial human-human and human-AI social interactions. Comput. Hum. Behav. 72, 432–440. doi:10.1016/j.chb.2017.02.067
Muresan, A., and Pohl, H. (2019). “Chats with bots: Balancing imitation and engagement,” in Conference on Human Factors in Computing Systems - Proceedings, Glasgow, United Kingdom, May 4–7, 2019, 1–6. doi:10.1145/3290607.3313084
Nass, C., Fogg, B. J., and Moon, Y. (1996). Can computer be teammates?. International Journal of Human-Computer Studies 45 (6), 669–678.
Nass, C., and Moon, Y. (2000). Machines and mindlessness: Social responses to computers. Journal of social issues 56 (1), 81–103.
Nass, C., Steuer, J., and Tauber, E. R. (1994). “Computer are social actors,” in Conference on Human Factors in Computing Systems - Proceedings, Boston, United States, April 24–28, 1994, 72–78.
Newman, G. E., and Smith, R. K. (2016). Kinds of authenticity. Philos. Compass 11 (10), 609–618. doi:10.1111/phc3.12343
Nowak, K. L., and Biocca, F. (2003). The effect of the agency and anthropomorphism on users’ sense of telepresence, copresence, and social presence in virtual environments. Presence. (Camb). 12 (5), 481–494. doi:10.1162/105474603322761289
Nowak, K. L., and Fox, J. (2018). Avatars and computer-mediated communication: A review of the definitions, uses, and effects of digital representations on communication. Rev. Commun. Res. 6, 30–53. doi:10.12840/issn.2255-4165.2018.06.01.015
Nowak, K. L., and Rauh, C. (2006). The influence of the avatar on online perceptions of anthropomorphism, androgyny, credibility, homophily, and attraction. J. Comput. Mediat. Commun. 11, 153–178. doi:10.1111/j.1083-6101.2006.tb00308.x
Oliver, R. L. (1980). A cognitive model of the antecedents and consequences of satisfaction decisions. J. Mark. Res. 17 (4), 460. Available at:. doi:10.2307/3150499
Oliver, R. L. (1976). Effect of expectation and disconfirmation on postexposure product evaluations: An alternative interpretation. J. Appl. Psychol. 62 (4), 480–486. doi:10.1037/0021-9010.62.4.480
Park, H. W., Rosenberg-Kima, R., Rosenberg, M., Gordon, G., and Breazeal, C. (2017). “Growing growth mindset with a social robot peer,” in Proceedings of the 2017 ACM IEEE International Conference on Human-Robot Interaction, 137–145.
Peters, C. E., Peters, C., and Skantze, G. (2019). Towards the use of mixed reality for HRI design via virtual robots towards the use of mixed reality for HRI design via virtual robots, 1
Pew Research Center (2017). Nearly half of Americans use digital voice assistants, mostly on their smartphones. Pew Research Center, Washington DC, 12 December. Available at: https://www.pewresearch.org/fact-tank/2017/12/12/nearly-half-of-americans-use-digital-voice-assistants-mostly-on-their-smartphones/ (Accessed December 30, 2020).
Pew Research Center (2019). 5 things to know about Americans and their smart speakers. Pew Research Center, Washington DC, 21 November. Available at: https://www.pewresearch.org/fact-tank/2019/11/21/5-things-to-know-about-americans-and-their-smart-speakers/ (Accessed December 30, 2020).
Pfeifer, R., and Scheier, C. (2001). “Glossary,” in Understanding intelligence (MIT Press), 645–665. doi:10.1007/978-3-642-41714-6_140047
Rahwan, I., Cebrian, M., Obradovich, N., Bongard, J., Bonnefon, J. F., Breazeal, C., et al. (2019). Machine behaviour. Nature 568 (7753), 477–486. doi:10.1038/s41586-019-1138-y
Reeves, B., and Nass, C. I. (1996). The media equation: How people treat computers, television, and new media like real people and places. Cambridge University Press.
Reynolds, C. W. (2006). Steering behaviors for autonomous characters steering behaviors for autonomous characters, 1–14. Available at: http://www.red3d.com/cwr/steer/gdc99/.
Shechtman, N., and Horowitz, L. M. (2003). Media inequality in conversation, 281. Available at:. doi:10.1145/642659.6426615
Shen, X. (2020). Virtual anime idols join China’s live streaming ecommerce craze | South China Morning Post, South China Morning Post. Available at: https://www.scmp.com/abacus/news-bites/article/3082730/virtual-anime-idols-join-chinas-live-streaming-ecommerce-craze.
Shum, H. Y., He, X., and Li, D. (2018). From Eliza to XiaoIce: Challenges and opportunities with social chatbots. arXiv [Preprint].
Solon, O. (2018). Google’s robot assistant now makes eerily lifelike phone calls for you, the Guardian. Available at: https://www.theguardian.com/technology/2018/may/08/google-duplex-assistant-phone-calls-robot-human (Accessed: May 20, 2021).
Sousa, A. M. (2016). “Beauty is in the eye of the “produser”: Virtual idol Hatsune Miku from software, to network, to stage” in Post Screen: Intermittence + Interference. Editors A. Montinho, A. Vicente, H. Ferreira, J. Pinto, and J. Primo. (Lisbon: Edições Universitárias Lusófonas), 117–128.
Sundar, S. S., and Kim, J. (2019). “Machine heuristic: When we trust computers more than humans with our personal information,” in Proceedings of the 2019 CHI Conference on human factors in computing systems, 1–9. doi:10.1145/3290605.3300768
Sundar, S. S. (2020). Rise of machine agency: A framework for studying the psychology of human–AI interaction (haii). J. Comput. Mediat. Commun. 25 (1), 74–88. doi:10.1093/jcmc/zmz026
Sundar, S. S. (2008). “The main model: A heuristic approach to understanding technology effects on credibility” in Digital Media, Youth, and Credibility. Editors M.J. Metzger, and A.J. Flanagin (Cambridge: The MIT Press), 73–100. doi:10.1162/dmal.9780262562324.073
Thue, D., Bulitko, V., Spetch, M., and Romanuik, T. (2011). A computational model of perceived agency in video games. Proc. 7th AAAI Conf. Artif. Intell. Interact. Digital Entertain. AIIDE 2011, 91–96. doi:10.1609/aiide.v7i1.12437
Trivedi, A., and Thakkar, Z. (2019). Chatbot generation and integration: A review. Int. J. Adv. Res. 5 (2), 1308–1311.
Tsang, M. (2019). Talk” to your celebrity Crush about your daily Spending with this app, product coalition. Available at: https://productcoalition.com/tell-me-where-you-spent-your-money-and-how-you-felt-today-c1469b316c1e (Accessed August 28, 2022).
Turing, A. M. (1950). I.—computing machinery and intelligence. Mind 49, 433–460. doi:10.1093/mind/lix.236.433
Turkle, S. (2011). Alone together: Why we expect more from technology and less from each other. New York: Basic Books.
Turkle, S. (2007). Authenticity in the age of digital companions. Interact. Stud. 8 (3), 501–517. doi:10.1075/is.8.3.11tur
Vaccari, C., and Chadwick, A. (2020). Deepfakes and disinformation: Exploring the impact of synthetic political video on deception, uncertainty, and trust in news. Soc. Media Soc. 6 (1), 205630512090340. doi:10.1177/2056305120903408
Van Oijen, J., and Dignum, F. (2013). Agent communication for believable human-like interactions between virtual characters. Lect. Notes Comput. Sci. Incl. Subser. Lect. Notes Artif. Intell. Lect. Notes Bioinforma., 7764, 37–54. doi:10.1007/978-3-642-36444-0_3
Varga, S., and Guignon, C. (2020). Authenticity, Stanford encyclopedia of philosophy. doi:10.5860/choice.41sup-0181
Vasalou, A., and Joinson, A. N. (2009). Me, myself and I: The role of interactional context on self-presentation through avatars. Comput. Hum. Behav. 25 (2), 510–520. doi:10.1016/j.chb.2008.11.007
Weizenbaum, J. (1966). ELIZA—a computer program for the study of natural language communication between man and machine. Commun. ACM 9 (1), 23–28. doi:10.1145/357980.357991
Wilson, E. J., and Sherrell, D. L. (1993). Source effects in communication and persuasion research: A meta-analysis of effect size. J. Acad. Mark. Sci. 21 (2), 101–112. doi:10.1007/BF02894421
Xue, Y. (2020). Japanese virtual idol Hatsune Miku joins Taobao as a live streaming ambassador for upcoming 618 festival, South China Morning Post. Available at: https://www.scmp.com/tech/e-commerce/article/3088189/japanese-virtual-idol-hatsune-miku-joins-taobao-live-streaming (Accessed March 15, 2021).
Yang, H., and Sundar, S. (2020). “Machine heuristic: A concept explication and development of a scale,” in Paper presented at the 71st International Communication Association Annual Conference (Virtual Conference).
Yao, M. Z., and Ling, R. (2020). What is computer-mediated communication?”—an introduction to the special issue. J. Comput. Mediat. Commun. 25 (1), 4–8. doi:10.1093/jcmc/zmz027
Yau, Elaine (2020). China’s virtual idols with digital avatars and human voices: Meet the live-stream kings and queens of bilibili | South China morning post, South China morning post. Available at: https://www.scmp.com/lifestyle/entertainment/article/3111890/chinas-virtual-idols-digital-avatars-and-human-voices-meet (Accessed March 15, 2021).
Ye, D., Chen, G., Zhao, P., Qiu, F., Yuan, B., Zhang, W., et al. (2020). “‘Supervised learning achieves human-level performance in moba games: A case study of honor of kings’,” in IEEE transactions on neural networks and learning systems [preprint].
Yee, N., and Bailenson, J. (2007). The Proteus effect: The effect of transformed self-representation on behavior. Hum. Commun. Res. 33 (3), 271–290. doi:10.1111/j.1468-2958.2007.00299.x
Zhang, J. (2019). China’s virtual idols meet their fans at the intersection of entertainment and technology. South China Morning Post, South China Morning Post. Available at: https://www.scmp.com/tech/article/2188238/chinas-virtual-idols-meet-their-fans-intersection-entertainment-and-technology (Accessed: March 15, 2021).
Zhou, X. (2020). Virtual youtuber kizuna AI : Co-Creating human-non-human interaction and celebrity-audience relationship. Lund University. Available at: https://lup.lub.lu.se/luur/download?func=downloadFile&recordOId=9009369&fileOId=9009370.
Keywords: artificial agents, virtual characters, perceived authenticity, human-machine communication, human-likeness, perceived realness, agency
Citation: Huang J and Jung Y (2022) Perceived authenticity of virtual characters makes the difference. Front. Virtual Real. 3:1033709. doi: 10.3389/frvir.2022.1033709
Received: 31 August 2022; Accepted: 11 November 2022;
Published: 25 November 2022.
Edited by:
Mincheol Shin, Tilburg University, NetherlandsReviewed by:
Stephen Song, University of South Florida, United StatesPeng Hu, Anhui Agricultural University, China
Copyright © 2022 Huang and Jung. This is an open-access article distributed under the terms of the Creative Commons Attribution License (CC BY). The use, distribution or reproduction in other forums is permitted, provided the original author(s) and the copyright owner(s) are credited and that the original publication in this journal is cited, in accordance with accepted academic practice. No use, distribution or reproduction is permitted which does not comply with these terms.
*Correspondence: Junru Huang, anVucnUwMDFAZS5udHUuZWR1LnNn; Younbo Jung, anVuZ1lCQG50dS5lZHUuc2c=