- 1EPI Beagle, INRIA Rhône-Alpes, Villeurbanne, France
- 2LIRIS, UMR 5205 CNRS-INSA, Université de Lyon, Villeurbanne, France
- 3School of Physics and Astronomy, Tel Aviv University, Ramat Aviv, Israel
- 4Center for Theoretical Biological Physics, Rice University, Houston, TX, USA
Traditionally, astrocytes have been considered to couple via gap-junctions into a syncytium with only rudimentary spatial organization. However, this view is challenged by growing experimental evidence that astrocytes organize as a proper gap-junction mediated network with more complex region-dependent properties. On the other hand, the propagation range of intercellular calcium waves (ICW) within astrocyte populations is as well highly variable, depending on the brain region considered. This suggests that the variability of the topology of gap-junction couplings could play a role in the variability of the ICW propagation range. Since this hypothesis is very difficult to investigate with current experimental approaches, we explore it here using a biophysically realistic model of three-dimensional astrocyte networks in which we varied the topology of the astrocyte network, while keeping intracellular properties and spatial cell distribution and density constant. Computer simulations of the model suggest that changing the topology of the network is indeed sufficient to reproduce the distinct ranges of ICW propagation reported experimentally. Unexpectedly, our simulations also predict that sparse connectivity and restriction of gap-junction couplings to short distances should favor propagation while long–distance or dense connectivity should impair it. Altogether, our results provide support to recent experimental findings that point toward a significant functional role of the organization of gap-junction couplings into proper astroglial networks. Dynamic control of this topology by neurons and signaling molecules could thus constitute a new type of regulation of neuron-glia and glia-glia interactions.
1. Introduction
Astrocytes, one type of glial cells of the brain, respond to neighboring neuronal activity by increases of their cytoplasmic Ca2+ concentration (Zonta and Carmignoto, 2002; Agulhon et al., 2008). Such transient intracellular Ca2+ signals are generally accepted to trigger the release from the astrocytes of signaling molecules (i.e., “gliotransmitters” like glutamate or ATP) that may regulate neuronal activity (Haydon and Carmignoto, 2006; Perea et al., 2009). Although the molecular pathways supporting these mechanisms remain partly under debate (Agulhon et al., 2010; Sun et al., 2013), their existence suggests a possible signaling role for astrocytes in brain communication, implying that brain information could travel not just in the neuronal circuitry but in an expanded neuron–astrocyte network (Haydon, 2001; Nedergaard et al., 2003; Volterra and Meldolesi, 2005; Giaume et al., 2010).
Calcium elevations can propagate within astrocyte populations as intercellular Ca2+ waves (ICWs) (Haydon, 2001; Scemes and Giaume, 2006; Zorec et al., 2012) and these ICWs have extensively been observed in astrocyte cultures. Recent experiments on brain slices as well as in vivo (Zorec et al., 2012), confirmed their existence in physiological conditions (Kuga et al., 2011). Much effort has been devoted to understand the biochemical mechanisms responsible for initiation and propagation of ICWs (Charles, 1998; Scemes et al., 2000; Charles and Giaume, 2002). Within the single astrocyte, intracellular Ca2+ dynamics is mainly due to Ca2+-induced Ca2+ release (CICR) from the endoplasmic reticulum (ER) stores—a self-amplifying release mechanism triggered and regulated by inositol 1,4,5-triphosphate (IP3) (Nimmerjahn, 2009). On the other hand, although experimental protocols monitor it as variations of intracellular Ca2+, the signal that is transmitted from one astrocyte to another in an ICW is generally not Ca2+, but ATP or IP3 (Scemes and Giaume, 2006). In the first case, the release of ATP from one astrocyte into the extracellular space activates purinergic receptors on neighboring astrocytes, which leads to Ca2+ elevations therein (Guthrie et al., 1999; Arcuino et al., 2002). In the second scenario instead, Ca2+-increase in the source cell favors IP3 production by phospholipase Cδ (PLCδ). Direct IP3 transport from the cytoplasm of this cell to the cytoplasm of a coupled astrocyte through gap junction channels (GJCs) then triggers CICR and Ca2+ increase in the coupled astrocyte (Venance et al., 1997; Giaume and Venance, 1998; Goldberg et al., 2010). Although the ATP and IP3 signaling pathways for ICWs are not mutually exclusive, several lines of evidence suggest that direct IP3 diffusion through GJCs is likely the predominant route for propagation in many astrocyte types and brain areas (Carmignoto, 2000; Kettenmann and Ransom, 2004).
The spatial arrangement of astrocytes in vivo remains largely unclear. Early reports pointed that astrocytes form non-overlapping domains that “tile” the brain space (Bushong et al., 2002). This suggests a regular spatial arrangement of the cells (Barthélemy, 2010) and leads to a proximity-based coupling rule whereby each astrocyte would be GJC-coupled only to its nearest neighbors, at the boundary of their respective non-overlapping domains. However, more recent data suggested more complex coupling rules (Schipke et al., 2008; Giaume et al., 2010; Roux et al., 2011). Local variability of the coupling organization was reported in the olfactory glomeruli (Roux et al., 2011) or the somatosensory cortex (Houades et al., 2008). More generally, a significant number of the astrocytes found within a given coupling domain are not GJC-coupled to the main astrocyte coupling network. (Houades et al., 2006, 2008; Rela et al., 2010). This indicates that the rule deciding whether two astrocytes are GJC coupled is not purely based on their distance but may be more finely organized into precise anatomical and functional compartments (Pannasch and Rouach, 2013).
One possible effect of the heterogeneity of GJC couplings organization could be a variability in the propagation range of ICW. Indeed, experimental reports of the number of astrocytes activated by a single ICW yield highly variable numbers, from a few cells (Sul et al., 2004; Sasaki et al., 2011) up to 30 (Tian et al., 2006) or even hundreds of cells (Kuga et al., 2011). These discrepancies persist even when the variability due to the type of stimulation employed is factored out (Scemes and Giaume, 2006). This leads to the hypothesis that the variability of the ICW propagation range could be explained by variations of the organization of GJC couplings. The experimental investigation of this hypothesis is however severely limited by the difficulty to distinguish experimentally between variations in the intracellular signaling parameters (enzyme activities, receptor densities…) and variations of the spatial organization of GJC couplings. In such a situation, computer simulations are highly useful since one can easily vary intracellular signaling parameters while guarantying constant GJC-couplings and vice-versa.
Accordingly, computer simulations have been employed in previous studies to investigate how the ICW propagation range depends on the astrocyte-to-astrocyte variability of intracellular signaling parameters, including local IP3 regeneration, receptor subtypes, affinity of IP3 receptor-channels on Ca2+ stores or kinetics of IP3 transport through gap-junctions (Höfer et al., 2002; Iacobas et al., 2006; Goldberg et al., 2010). However, in these studies, the astrocytes are generally positioned in a one or two-dimensional space. The organization of GJC couplings in these simulation studies is usually unique and consists in nearest-neighbor coupling (or a variant thereof), in agreement with the hypothesis of non-overlapping domains. In light of the growing evidence that GJC coupling in astrocyte networks may be more complex, the hypothesis that the variability of the ICW propagation range may be due to variations of the organization of GJC couplings, needs to be tested in three-dimensional astrocyte networks with a variety of complex GJC-coupling organizations.
Here, we used computer simulations of ICW propagation in three-dimensional networks of GJC-coupled astrocytes using a realistic biophysical model for astrocytic Ca2+ dynamics (De Pittà et al., 2009; Goldberg et al., 2010). Our simulations suggest that changing the organization of GJC couplings is enough to reproduce the large variability of the range of ICW propagation observed in experiments. Therefore, our simulations predict that zones with distinct GJC-coupling should be expected to support ICW with distinct propagation ranges. Moreover, they hint that ICW propagation is favored in astrocyte networks that are sparsely GJC-coupled and that display large mean shortest paths, as found when the GJC couplings are restricted to short Euclidean distances. These results suggest that the principles whereby signals travel in astrocyte networks are different from those at play in neuronal networks.
2. Methods
Astrocyte Network Model
Intracellular calcium dynamics in the cytoplasm of astrocytes can be described by the ChI model that we previously developed and studied. This model provides a realistic description of the dynamics in isolated astrocytes (De Pittà et al., 2009; Goldberg et al., 2010). In this model, possible spatial non-homogeneities of the intracellular distribution of chemical species are neglected. Similarly, the intricate and complex shape of the astrocytes is not taken into account. Astrocytes are thus simplified in the model as perfectly-stirred cells with spherical shapes. Albeit a crude approximation, this allows simulating ICW propagations in large astrocyte populations (>103 cells), an objective that is much harder to achieve with more detailed description of intracellular dynamics. The ChI model considers both Ca2+ regulation by IP3-dependent CICR as well as IP3 dynamics resulting from PLCδ–mediated production and degradation both by IP3 3-kinase (3K) and inositol polyphosphate 5-phosphatase (5P). Accordingly, the intracellular Ca2+ dynamics in the i-th astrocyte of the network is described by three coupled non–linear ordinary differential equations, for each astrocyte number i = {1, …, N}:
where the variables Ci,,hi, Ii respectively denote the cell-averaged cytosolic Ca2+ concentration, the fraction of activable IP3R channels on the ER membrane, and the cell-averaged cytosolic IP3 concentration. In this model, calcium dynamics (Equation 1) are the results of the interplay between three fluxes: a Ca2+ uptake from the cytosol to the ER (JP); a passive Ca2+ leak from the ER to the cytosol (JL); and an IP3-mediated Ca2+ efflux from the ER to the cytosol (JC). The fraction of activable IP3R channels (Equation 2) relaxes with a Ca2+ and IP3 dependent rate (Ωh) to an equilibrium value h∞. Finally, the IP3 concentration dynamics (Equation 3) are defined by the balance between IP3 production by PLCδ (Jδ) and degradation by 3K (J3K) and 5P (J5P). The additional term Jdiffi sums IP3 flows (Jij) from/to any cell j that is directly connected (GJC-coupled) to cell i, i.e., Jdiffi = ∑j∈i Jij with
i the set of astrocytes that are GJC-coupled to i (Goldberg et al., 2010). In terms of cell-averaged concentrations, the transport of IP3 between two cells may be more complex than simple linear diffusion through GJCs (Nagy and Rash, 2000). It could actually be regarded as a multiscale phenomenon that depends on many factors, including cell morphology and GJC location, permeability and physiology (De Pittà et al., 2012). To account for these factors, we assumed non–linear IP3 transport between GJC-coupled astrocytes, as detailed in the Supplementary Material S1, with
where ΔijI = Ii − Ij represents the IP3 gradient between cells i and j, Iθ represents the threshold IP3 gradient for effective intercellular diffusion, i.e., the minimal IP3 gradient allowing effective IP3 transfer. ωI sets the slope of the increase of the flux with ΔijI. Finally, the parameter F corresponds to the maximal diffusion flux between cells i and j, and may thus be regarded as the strength of coupling between two cells. Note however that our results do not crucially depend on this non–linear coupling, since linear (diffusive) couplings yields qualitatively similar results (see Supplementary Figure S7). A detailed presentation of the model can be found in the Supplementary Material S1.
Topologies of the GJC-Coupled Astrocyte Networks
Just like neurons, astrocyte-astrocyte signaling interactions via GJC coupling can be modeled as a network, where each node represents an astrocyte (whole cell) and the links locate GJC–mediated connections (couplings) between two astrocytes (Giaume et al., 2010). To address ICW propagation in realistic conditions, we constructed three-dimensional spatial networks of astrocytes by a two-step procedure. First N astrocytes were properly positioned in the physical (Euclidean) space to match experimental data on astrocyte spatial arrangement. Then, a wiring strategy was deployed to establish coupling among the astrocytes to obtain networks of desired coupling organizations.
To achieve proper positioning of astrocytes in space, N = 113 model astrocytes were initially positioned on a 11 × 11 × 11 cubic lattice with internode distance a (Figure 1A, circled panel). The position of each astrocyte was then jittered by Gaussian noise with zero mean and variance σ2 to reproduce the experimental measurements of the distribution of astrocyte-to-astrocyte distances in the mouse hippocampus: the distance between nearest–neighbor cell centers was reported to be 50 μm on average with a minimal value of 20 μm and a coefficient of variation ~0.25 (Sasaki et al., 2011). Using minimization of square errors, we could reproduce these experimental data in our model using a cubic lattice internode distance a ≈ 70 μm followed by a Gaussian jitter with σ2 = 552 μm2. Therefore, the simulation comprised N = 1331 astrocytes in a domain of size ≈ 0.50 mm3.
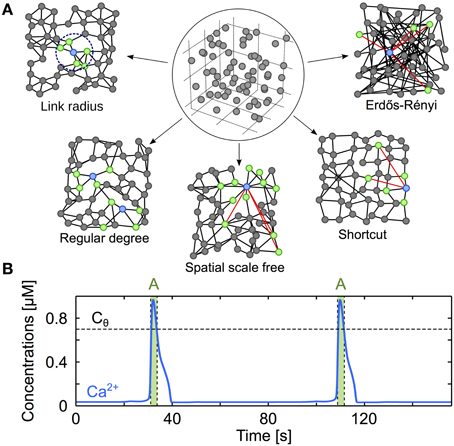
Figure 1. Modeling three-dimensional astrocyte networks. (A) The astrocytes (circles) are first positioned on a cubic lattice. The positions are then jittered by random values so as to reproduce experimentally-derived cell distance statistics (circled panel). The astrocytes are then GJC-coupled (black lines) according to different coupling rules (described in “Methods”), yielding distinct types of coupling organizations (other panels). For clarity, the networks illustrated here are two-dimensional, but the networks used in the study are systematically three-dimensional. Red links denote shortcuts, green cells locate the cells that are GJC-coupled to the blue one. (B) Typical trace from simulation of the ChI model that gives the intracellular Ca2+ dynamics in each cell of the network. Astrocytes are considered activated (A, green-shaded regions) when their cytosolic Ca2+ concentration (blue traces) exceeds the threshold Cθ = 0.7 μM (black dashed lines). Astrocyte parameters as in Table 1.
With astrocytes properly positioned, we implemented different organizations of the network of GJC couplings. Because the organization of GJC couplings in vivo remains uncertain, several plausible coupling networks were considered (see main text and Figure 1A):
1. Regular degree networks were constructed by coupling each astrocyte to its k nearest neighbors in space, where k is a parameter that we systematically varied. Each astrocyte in these networks is thus GJC-coupled to exactly the same number of cells (k).
2. Link radius networks are a subtle variant: they are constructed by coupling each astrocyte to all the cells that are found within a distance d, where d is a parameter that we varied here. Therefore, the number of distinct cells coupled to each astrocyte in link radius networks is a random variable, whose average value (〈k〉) increases with d.
3. Shortcut networks are cubic lattices in which a low amount of long distance GJC coupling is allowed. We started from a cubic lattice that we built with an internode distance a. We then linked each node to his nearest neighbors at distances that are multiples of a and lower or equal to a × mlatt. As we work in a d′ = 3 three-dimensional space, the mean degree of a node can only take discrete 2mlattd′ values. Detailed description of these lattices can be found in Watts (1999). Node position were then jittered as explained before and each GJC coupling was rewired with probability ps (a parameter that was varied): that is, with probability ps, one of the edge ends of each GJC coupling was replaced by a (uniformly) randomly chosen cell. These networks bear so–called “small–world” features depending on the value of ps (Albert and Barabási, 2002).
4. Spatial scale free networks were introduced to test the influence of “hub” astrocytes, i.e., astrocytes coupled to a very large number of other cells (over 20 neighbors, that is twice as much as the estimated mean degree in 3D astrocyte networks in situ Xu et al., 2010). These networks were incrementally built by a classical preferential attachment rule, where one introduces astrocytes one after the other in the network and preferentially connects them to the astrocytes with larger degree yet considering spatial distances, according to the procedure described in Barthélemy (2010). More precisely, each newcomer astrocyte is coupled to msf astrocytes of the network formed by the previously coupled astrocytes. These msf astrocytes are randomly chosen but with the constraint that the probability pi→j to choose astrocyte j increases with j′s degree and decreases with its distance: pi→j ∝ kj exp(−dij/rc) where i is the index of the newcomer and dij the distance between i and j. The parameter rc controls the trade-off between scale–free structure and the restriction of the couplings to short distances (Barthélemy, 2010). For large values of rc (larger than 20 μm in our case), some of the astrocytes (“hubs”) are coupled to a very large number of cells but the GJC couplings extend over large distances. On the contrary, small rc values (less than 5 μm in our case) lead to networks that are devoid of hubs but that feature short distance GJC couplings.
5. Erdős-Rényi networks were constructed by coupling each pair of astrocytes with probability p, independently of their distance. Hence, in these networks the distance between astrocytes is not a constraint for their GJC coupling. They have mostly been used here as a control to test our theories about what quantifiers of the network organization conditions ICW propagation.
While both regular degree and link radius networks may be regarded as spatially–constrained networks inasmuch as the coupling between the astrocytes are limited by the distance between cells, the presence of long-distance GJC couplings in shortcut and Erdős-Rényi networks, makes these networks essentially spatially–unconstrained.
Numerical Methods
Simulations of Ca2+ propagation in astrocyte networks were performed by numerical integration of the network model by a fourth order Runge–Kutta scheme with a time step of 0.01 s. The parameters of the ChI model Equations (1–3), (reported in Table 1) were chosen according to previous studies (De Pittà et al., 2009; Goldberg et al., 2010) so as to reproduce the experimentally-observed pulse-like shape (Figure 1B) of propagating ICW waveforms (namely Ca2+ pulses of width much smaller than their wavelength) and whose frequency increases with the frequency or the intensity of stimulation (Pasti et al., 1997; Tian et al., 2006; Kuga et al., 2011). The organization parameters (e.g., k, d, rc, ps, p) were systematically varied so as to obtain networks with a mean degree 〈k〉 ranging from 3 to 17 (reported in Table 2). The minimal value of 〈k〉 was set by the constraint that the fraction of node pairs with infinite topological distance should be below 2%. The coupling organizations used here are essentially random networks so that each organization parameter defines a distribution of coupling networks. For statistical significance, quantification of ICW propagation in these coupling networks must therefore be averaged over several samples of the random networks defined by a given coupling organization type and a given parameter. Here, simulation results were averaged over 20 different network samples for each value of the organization parameter and each organization class. Ca2+ wave propagation was triggered by selectively stimulating an astrocyte of the network for all the duration T = 200 s of the simulation. This allowed Ca2+ waves to fully propagate to their maximum extent (see Supplementary Material S1). During simulations, an astrocyte was considered activated if its cytosolic Ca2+ concentration exceeded a threshold value of Cθ = 0.7 μM (Figure 1B). As calcium oscillations are very stereotypical with the parameters that we chose, significant calcium oscillations always crossed this threshold. The extent of Ca2+ ICW propagation was quantified by the total number of cells (Nact) that were activated at least once during a simulation. To minimize boundary effects due to the spatial confinement of the modeled network, stimulation was delivered to an astrocyte located in the center of the network.
Theoretical Analysis
In order to identify the major properties conditioning the ICW propagation range, we have carried out mathematical analysis of the computational model, focusing on the effect of network structure. This analysis is detailed in Supplementary Material S2.
3. Results
The Spatial Organization of GJC Couplings Drives the Extent of ICW Propagation
To investigate the effect of network organization on ICW propagation, we developed a computer model that simulates ICWs propagation in three-dimensional astrocyte networks. In the model, each astrocyte is a point-like object described by its location in space and three internal variables (see Methods): its cytoplasmic Ca2+ concentration C, the fraction h of activable IP3R channels in the membranes of Ca2+ stores and its cytoplasmic IP3 concentration I. Therefore we neglect complications due to the intricate shape of astrocytes. The model expresses the evolution in time of each of these quantities taking into account IP3-mediated exchanges between internal Ca2+ stores and Ca2+-dependent IP3 synthesis and degradation. To this aim, we used the ChI model that provides a realistic description of Ca2+-IP3 dynamics in isolated astrocytes (De Pittà et al., 2009; Goldberg et al., 2010) (see Methods and Supplementary Material S1 for a detailed description).
In the model, we position N = 1331 astrocytes in a three-dimensional domain of size ≈ 800 × 800 × 800 μm3 (roughly 0.5 mm3) so as to emulate the distribution of cell-cell distances reported in mouse hippocampus (Sasaki et al., 2011) (see Methods). Each astrocyte can be coupled via gap-junction channels (GJCs) to other astrocytes. Such a coupling allows bidirectional transport of IP3 directly from cytoplasm to cytoplasm of the two coupled cells. How these gap junction couplings are organized, i.e., what is the rule deciding whether two astrocytes are GJC-coupled in the network (Figure 1A) is referred to as the “topology” of the network and is the main focus of our study. Since the exact organization of couplings in astrocyte networks in vivo is still unclear, we have implemented several possible coupling topologies (see Methods). The topologies included (i) spatially–constrained link radius or regular degree networks, where astrocyte are GJC-coupled only when they are close in space, and (ii) spatially–unconstrained networks, such as Erdős-Rényi networks, where astrocytes are GJC-coupled independently of their distance. In between these two network classes, spatial scale free and shortcut networks feature a parameter (rc and ps, respectively) that allows to continuously vary them from spatially–constrained to spatially–unconstrained.
To quantify these coupling topologies, we introduce two classical quantifiers of such complex networks (Albert and Barabási, 2002; Barthélemy, 2010): the average number of distinct cells coupled to each astrocyte, 〈k〉 (or mean degree), that quantifies the well-connectedness of the network, and the mean shortest path L. The shortest path (or topological distance) between two astrocytes is the minimal number of GJC couplings one must cross to connect the two astrocytes. L is the average of the shortest paths between all astrocyte pairs and quantifies the mean distance between astrocytes in terms of numbers of couplings. Each type of coupling organization listed above comes with one or two construction parameters (k, d, ps…). We varied these parameters so as to change the values of the mean degree 〈k〉 and the mean shortest path L for each type of coupling organization. Note that network topologies can be characterized using other quantifiers of complex networks [like the clustering coefficient, the distribution of the degrees P(k) or the hierarchical clustering coefficient (Boccaletti et al., 2006; Costa and da Rocha, 2006; Feldt et al., 2010)] but a preliminary investigation by these quantifiers did not account for the propagation extent as well as 〈k〉 and L did (see Supplementary Material S2).
All the above networks differed only by the topology of the GJC couplings since all other parameters were kept constant, including the kinetics of GJC coupling, the number (N = 1331), spatial distribution and density of the astrocytes, the parameters of the dynamics inside each astrocyte as well as the stimulation that triggers the ICW (see Methods). Therefore, differences in ICW propagations across different networks can unambiguously be related to differences in coupling organization. In the simulations, the propagation of the ICW to a given astrocyte is characterized by a strong and transient elevation of cytosolic Ca2+ in this astrocyte (Figure 1B). We considered that the ICW reaches an astrocyte, or that, equivalently, an astrocyte is activated by the ICW, if the cytosolic Ca2+ concentration in the astrocyte exceeds a threshold value of Cθ = 0.7 μM. To quantify the propagation range of an ICW, we counted the number of astrocytes that got activated at least once (Nact) during the propagation of the ICW.
Figure 2 illustrates the type of behaviors observed during typical simulation examples. It shows the cells (green circles) that got activated by an ICW triggered by a prolonged stimulation (t = 200 s) of the astrocyte shown in red. In a spatial scale free network (Figure 2A) with mean degree as large as 〈k〉 = 6 and relatively small mean shortest path L = 5, ICW propagation was restricted only to Nact ≈ 20 astrocytes around the stimulated cell. The extent of propagation was considerably larger (i.e., Nact ≈ 80) in the regular degree network shown in Figure 2B that shares the same mean degree but has larger mean shortest path (i.e., L = 9) than the previous one. Remarkably however, in another regular degree network with even larger mean shortest path (L = 15) but small mean degree 〈k〉 = 3 (Figure 2C), ICW propagated to roughly all the cells in the network, that is Nact ≈ N. Keeping a constant mean degree 〈k〉 = 6, increases in mean-shortest path are linked to increases in ICW extent. Indeed, from a purely random Erdős-Rényi network with very low mean-shortest path (Figure 2D) to shortcut networks with 5% rewiring probability (Figure 2F), lowering the amount of shortcuts in a network increases ICW extents. Figures 2A–C are reminiscent of the different types of ICWs observed in experiments: namely, localized Ca2+ waves, for which Nact ≈ 10−50 (Charles, 1998; Sul et al., 2004; Sasaki et al., 2011), as well as long-range propagating ICWs involving a large number of cells (Nact > 100) (Hirase et al., 2004; Peters et al., 2005; Kuga et al., 2011).
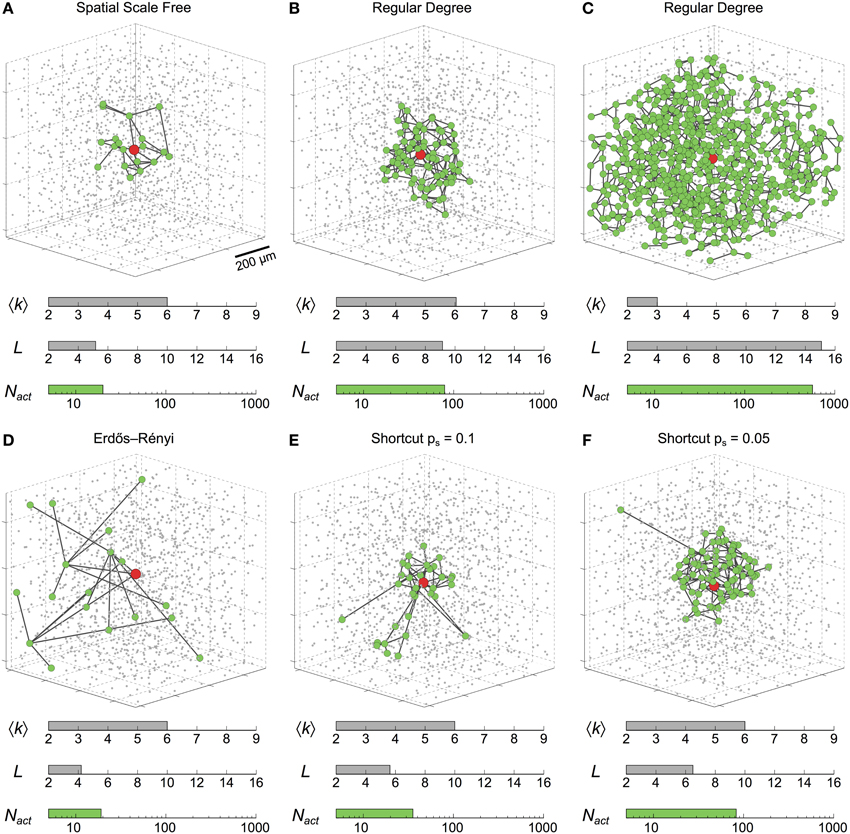
Figure 2. Intercellular Ca2+ waves in 3D astrocyte networks of different coupling organizations. Changing the organization of the couplings between astrocytes dramatically affects the extent of propagation of ICWs, as quantified by the number of activated astrocytes (Nact). The six networks in this figure feature the same number of cells (gray dots). Green circles denote astrocytes that were activated by the ICW triggered via stimulation of the red astrocyte in the center of the network. (A) rc = 25 μm, msf = 3; (B) 〈k〉 = 6; (C) 〈k〉 = 3; (D) p = ; (E) ps = 0.1, mlatt = 1; (F) ps = 0.05, mlatt = 1. Astrocyte parameters as in Table 1.
Taken together, the simulations in Figure 2 suggest that the organization of coupling in an astrocyte network can dramatically control the extent of propagation of ICWs. Accordingly, the different ranges of ICW propagation observed in experiments can be partly explained by differences in the organization of GJC coupling between cells in the network. In particular, as in the case of the regular degree networks of Figures 2B,C, the propagation extent seems to critically depend on network characteristics such as the mean degree 〈k〉 and the mean shortest path L. We investigate this issue in the next section.
Small Mean Degrees and Large Mean Shortest Paths Favor ICW Propagation
Figure 3A summarizes the number of cells activated by ICW propagation (Nact) as a function of the mean degree of the network (〈k〉) for different types of coupling organization. Although the precise behavior of Nact vs. 〈k〉 depends on the type of organization, the range of ICW propagation follows a generic rule: whatever the type of coupling organization, the propagation range decreases with increasing 〈k〉. Therefore, as a general rule, the larger the number of GJC couplings between cells, the worse the propagation. This is at odds with the conclusions from many studies related to dynamics on complex networks, where a large mean degree is usually associated with better signal propagation (Isham et al., 2011). Moreover, for a given value of the mean degree 〈k〉, propagation is generally better in networks with only short distance couplings (Link radius, Regular, Shortcut with ps = 0) than in networks featuring large-distance couplings (Erdős-Rényi, Shortcut with ps > 0). This can also be seen by comparing scale-free networks with rc = 2 μm (light blue, featuring almost no long-distance coupling) with scale-free networks with rc = 25 μm (dark blue, with many long-distance couplings). The latter essentially supports shorter propagation than the former. On the other hand, for a given 〈k〉 value (e.g., 〈k〉 = 6), the extent of ICW propagation can differ up to 5–10 folds between coupling organizations. This indicates that the mean degree is not the unique causal quantity, and that other features of the network are likely to regulate ICW propagation.
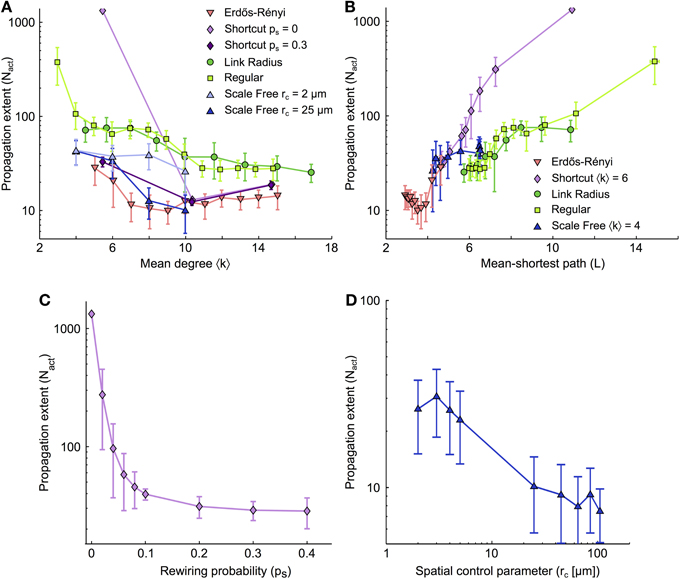
Figure 3. Dependence of the extent of ICW propagation on the main quantifiers of the coupling organization. (A) Extent of ICW propagation (quantified by the number of activated cells, Nact) as a function of the mean degree 〈k〉 and (B) of the mean-shortest path L. The number of activated cells segregates spatially–constrained (link radius, regular degree and shortcut with ps = 0) from spatially–unconstrained coupling organizations (shortcut with ps > 0, and Erdős-Rényi). (C) Extent of propagation in shortcut networks as a function of the rewiring probability ps. (D) Extent of propagation in spatial scale free networks as a function of the parameter rc that controls the trade-off between scale–free structure and the restriction of the couplings to short distances. (C,D) suggest that ICW propagation is favored by short-distance GJC couplings between astrocytes while long–distance couplings hinder propagation. Simulations as described in Figure 2. Data points ± errorbars correspond to mean values ± standard deviation over 20 sampled networks with the same statistical parameters (see Methods). The shortcut networks in (C) were all built with 〈k〉 = 6 (i.e., mlatt = 1). The spatial scale free networks in (D) were built with 〈k〉 = 10 (i.e., msf = 5). Astrocyte parameters as in Table 1.
In Figure 3B, we plot the propagation range Nact as a function of the mean shortest path L for all studied coupling organizations. Here again, a conserved trend is observed: whatever the type of organization, the propagation range Nact grows with the mean shortest path L. And here again, this result contrasts with the common intuition that small mean shortest paths represent large efficiency in signal transmission and should optimize signal propagation in a network (Zanette, 2002). Moreover, from the dependence of Nact on L, one clearly distinguishes the two distinct groups of coupling organizations: organizations that are strictly restricted to short-distance couplings (Link radius and Regular degree) and those that are not (shortcut, Erdős-Rényi). For each group, the curves of Nact vs L essentially collapse on roughly a single curve, so that the increase of Nact with L is described by only two curves for all coupling organizations.
For scale-free networks, we changed the value of the mean shortest path L by varying rc: small values of rc give mostly short-distance couplings, large L values and essentially no hubs whereas large values of rc yield long-distance couplings with small L values and highly connected hubs. Figure 3B shows that the propagation range in scale-free networks is mostly given by the presence or absence of long-distance GJC-couplings, independently of the presence of hubs. Indeed, when L is small thus long-distance GJC and hubs are present, scale-free networks essentially behave like Erdős-Rényi and Shortcut networks (that display long-distance GJC but no hubs). Therefore the presence of long-distance GJC couplings in scale-free organizations with small L seems more influential than the presence of hubs. When L increases, scale-free networks, that progressively loose long-range couplings and hubs, crossover to the behavior of Link radius and Regular degree networks, i.e., the coupling organizations that feature only short-distance couplings. This evidences that spatial constraint and mean shortest path are the major topological determinants of ICW propagation, while the presence of hubs, for instance, is less influential. Figure 3C shows the propagation range in shortcut networks for increasing values of rewiring probability ps, i.e., progressively larger numbers of long-distance GJC couplings between astrocytes. The ICW propagation range decays very rapidly with increasing ps although all networks have the same mean degree. Similarly, in spatial scale free networks, allowing long distance links by increasing rc leads to decreases in ICW extent (Figure 3D). Hence, long-distance couplings strongly restricts the extent of ICW propagation.
In summary, our analysis suggests that the dependence of ICW propagation in astrocyte networks on mean degree and mean shortest paths is the exact opposite of the common view on signal propagation in complex networks: for all simulated coupling organizations, ICW propagation was improved when either the mean degree was small or the mean shortest path was large and strongly decreased when long-distance couplings were introduced. Note that these organization properties are interrelated, since the strict restriction of GJC coupling to short distances yields large mean shortest paths while the introduction of long-distance GJC coupling reduces the mean shortest path.
Influence of the Shell Structure
A characterization of the features of network topology that locally regulate ICW propagation may be obtained by the analysis of the shell structure of the network (see Figure 4A), where each shell r is defined as the set of cells at shortest path (topological) distance r from a reference cell that we choose as the cell that initiates the ICW. These shell structures are schematically exemplified in Figure 4B for two network topologies: cubic lattices (〈k〉 = 6, L = 11), and regular networks either with the same mean degree (〈k〉 = 6, L = 8.8) or with the same mean shortest path (〈k〉 = 4, L = 11). The procedures used to generate these networks were very similar: for the cubic lattice, the astrocytes were first connected to their k = 6 nearest neighbors, then their positions were jittered (as described in methods), leaving the connections unchanged. Conversely, regular networks were built first by jittering the cell position, and then by connecting the cells to their k = 6 nearest neighbors. Those cubic lattice and regular networks share identical topological parameters and the connections between their cells show only subtle differences. Even though these differences are minute, ICW propagation in these networks are remarkably distinct, with a number of cells activated by an ICW (Nact) that is up to ten-fold larger in cubic lattices than in regular networks (Figure 4C). This astonishing behavior can be explained by differences in the shell structure of these networks.
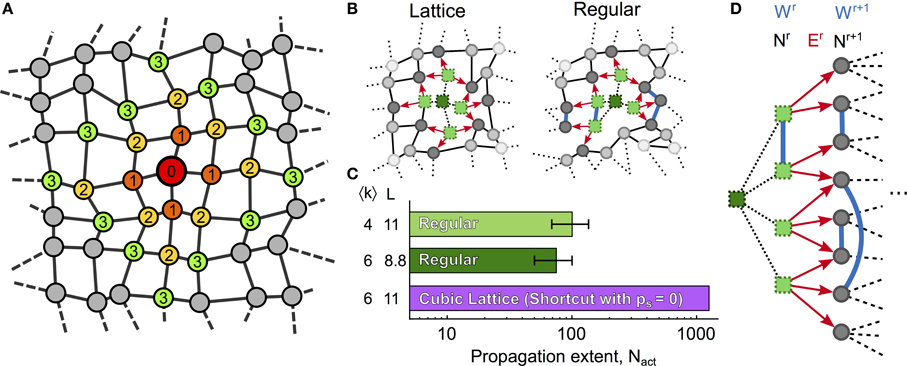
Figure 4. Shell analysis. (A) Ilustration of shells 1–3 in a generic network; nodes are colored according to their topological distance (numbers) from a red reference node. A shell is a set of nodes that have the same topological distance r to the reference node (thus the same color in the figure). (B) 2D representation of ICW propagation (red arrows) in a cubic lattice and a regular network with identical mean degree. Bright green squares represent activated cells while dark green squares denote cells that were previously activated by the ICW. (C) Despite sharing similar topological features, propagation in the cubic lattice may activate up to 10-fold more cells than in regular networks. (D) Shell analysis reveals that this difference in ICW propagation may be attributable to the connections between cells within the same r-th shell (Wr) in regular networks, which reduce the quantity of IP3 given to astrocytes in the next shell r + 1, thus resulting in earlier propagation failures than in cubic lattices. Definitions of Nr, Wr, and Er are given in the main text. Cubic lattices were built by shortcut networks with ps = 0 and mlatt = 1 (see “Methods”). Model parameters as in Table 1.
Let us denote by Nr the number of cells in the r-th shell, by Wr the number of links between cells within the same r-th shell, and by Er the number of links between cells in shell r and cells in next shell r + 1. Figure 4D illustrates the three quantities Nr, Wr, Er for two consecutive shells. While there are no links between cells belonging to the same shell in cubic lattices, regular networks feature significant amounts of intra-shell connections. Therefore, while Wr = 0 for every shell of the cubic lattice, Wr ≥ 0 in regular networks. This observation crucially accounts for the smaller ICW extent in regular networks compared to cubic lattices.
The forward propagation of an ICW away from its originating cell in fact can be regarded as a shell-by-shell activation process. Astrocytes inside a given shell however do not activate all at the same time so that, when only a fraction ρ of shell r is activated (i.e., ρ Nr astrocytes are activated in shell r), the IP3 quantity that they will produce will be distributed among: (1) Nr−1 astrocytes in the preceeding shell r − 1; (2) Nr+1 astrocytes in the following shell r + 1; and (3) unactivated astrocytes linked to the activated ones in shell r. This latter quantity can be expressed considering the number of unactivated astrocytes in shell r ((1 − ρ)Nr) and the probability for an unactivated astrocyte in shell r to be connected to an activated astrocyte in the same shell (≈ 2 ρ Wr/(Nr − 1), derived in Supplementary Material S3.3). The number of unactivated astrocytes in shell r connected to activated ones in the same shell thus reads:
Assuming that, for each activated astrocyte in shell r, the total amount Q0 of IP3 passed along the ki neighbors is constant (see Supplementary Material S3.1 and Supplementary Figure S6), the total amount of IP3 flowing out of the activated astrocytes in shell r can be approximated by Q0 ρ Nr. As IP3 going out of shell r will be divided among the Nr−1 astrocytes of the preceding shell, the Nr+1 astrocytes of the following shell and the 2ρ(1 − ρ)Wr unactivated astrocytes in the current shell, the mean IP3 supply to each of these unactivated astrocytes is then:
Since Wr = 0 in cubic lattices (see above), equation (6) predicts that the amount of IP3 supplied to unactivated astrocytes of shell r + 1 should be smaller in regular networks than in cubic lattices. Accordingly, the extent of ICW propagation is predicted to be smaller in regular networks than in the cubic lattices. This prediction agrees well with the simulations summarized in the histogram in Figure 4C (see also Supplementary Materials S3.3).
To conclude, the above analysis shows that the shell structure of the network has a crucial impact on ICW propagation, in addition to the general trends imposed by 〈k〉 and L. These results can be interpreted so as to define simple propagation rules that summarize the main properties of ICW propagation in astrocyte networks. These rules are given and tested in the last section.
The Range of ICW Propagation is Dictated by the Local Balance Between IP3 Accumulation and Diffusion
ICW propagation from an activated to an unactivated astrocyte relies both on GJC–mediated intercellular IP3 transport and on IP3 accumulation in the destination cell up to a threshold concentration that triggers CICR therein, thus locally regenerating the Ca2+ wave (Goldberg et al., 2010). The above analysis revealed that the most significant obstacle to ICW propagation is IP3 dilution to unactivated astrocytes. Indeed, when an astrocyte activates, the excess intracellular IP3 diffuses to every unactivated cell it is coupled to. However, the total amount of IP3 that is distributed by an activated astrocyte does not depend on the number of cells to which it is coupled. Therefore, the larger the number of cells coupled to an activated astrocyte (i.e., the larger its degree k), the smaller the amount of IP3 that diffuses to every individual unactivated cell. As a result, when IP3 flows from a source (activated) astrocyte to a destination (unactivated) one, the probability that the destination astrocyte effectively gets activated decreases when the source astrocyte is GJC-coupled to many unactivated cells.
This observation accounts for the simulation results of Figure 3A, where the propagation range decreases when the mean degree 〈k〉 of the GJC network increases. It also explains why long–distance connections between cells tend to hamper ICW propagation (see Supplementary Material S3). Figure 5 illustrates ICW propagation in time for three networks with identical mean degrees but different organization of the GJC couplings: a shortcut network (top panels), a regular network (middle panels) and a square lattice (bottom panels). Note that all the analyses and quantifications in this study were obtained with 3-dimensional networks, except in this figure where we show 2-dimensional networks for reasons of readability. The stimulation of an astrocyte in the center of the network (red circles in the leftmost panels) triggers an ICW that propagates toward the periphery. Whereas the ICW keeps on propagating long after the end of stimulation (at t = 25 s, red bar), both in the regular network and in the square lattice, it abruptly aborts (at t = 29 s) in the shortcut network. When IP3 is transported along a long–distance coupling, the destination astrocyte is very likely located far away from the propagation front, in a zone where most astrocytes are unactivated. It is thus likely that most of the astrocytes coupled to the destination astrocyte are unactivated, so that even though the destination astrocyte eventually gets activated, it cannot propagate the ICW further. This dilution effect, that hampers propagation, is much more reduced in networks with only short-distance couplings. Indeed, the destination cell of a short–distance GJC-coupling is by definition located in the vicinity of the propagation front and therefore is likely to be GJC-coupled to several activated astrocytes.
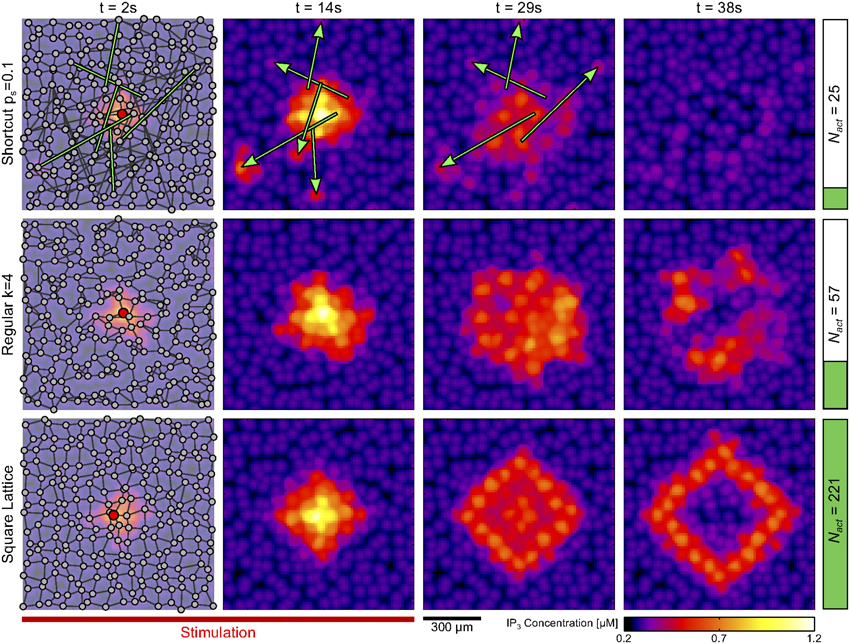
Figure 5. Effect of long-distance GJC-coupling on ICW propagation. Snapshots of IP3 concentration (see Supplementary Material S1.4) at different time instants in three 2D networks (shown in the leftmost panels): a shortcut network with ps = 0.1 (top row); a regular network with 〈k〉 = 4 (middle row); and a square lattice also with 〈k〉 = 4 (bottom row). The presence of long-distance connections (green edges) in the shortcut network causes IP3 transport away from the wave front, hampering ICW propagation. This is reflected by a considerably lower value of the number of cells activated by the ICW (Nact, right vertical bars) in the shortcut network compared to the other two networks. The ICW was triggered by stimulating the astrocyte marked in red (leftmost panels) from 0 s to 25 s (red bar). Astrocyte parameters as in Table 1.
The above rules can be expressed in a concise way by a simplified model of ICW propagation. To build this simplified model, we forget about the complex dynamics and interactions between Ca2+, IP3 and calcium stores that take place inside each astrocyte. Instead, we consider that an astrocyte is characterized by a single signaling state that can have only three values: (U)nactivated, (A)ctivated or (R)efractory (see Figure 6). The default (basal) state is the (U)nactivated one. When the ICW reaches astrocyte number i, i can become (A)ctivated with probability pU→Ai (that we define below). Once in the A state, the astrocyte first switches to the (R)refractory state with rate kA→R, then back to the U state, with rate kR→U.
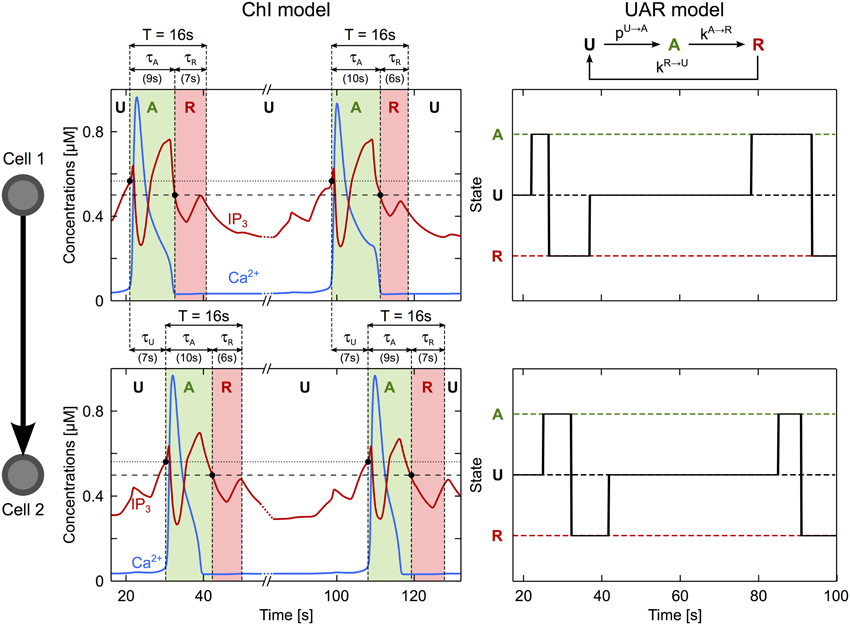
Figure 6. A simplified description of ICW propagation. The propagation of an ICW through an astrocyte may be regarded as a three-state process, as illustrated here for the case of two connected astrocytes (cell 1, top panel; cell 2, bottom panel). These astrocytes are in the unactivated state (U) when at rest. Upon arrival of an ICW (when the IP3 level crosses the threshold for CICR initiation, dotted line), the transient increase of intracellular Ca2+ (blue traces) occurring first in cell 1 then in cell 2, activates these cells (A, green regions). Following activation, each cell recovers to rest through a refractory period (R) when their IP3 value falls below the threshold gradient for intercellular diffusion (dashed line). The time constant of each transition may be estimated accordingly. τU coincides with the delay between the Ca2+ increases in cell 1 and in cell 2. τA is estimated by the time interval from the beginning of the Ca2+ elevation to the point where IP3 gets below the diffusion threshold. Finally, τR is estimated by τA + τR = T where T (= 16 s in this example) represents the minimum period of Ca2+ oscillations in a single cell. Astrocyte parameters as in Table 1.
At every time step, the model computes the propagation efficiency of each astrocyte according to the above mentioned rule: (i) an astrocyte can propagate the ICW only if it is activated by the ICW, and (ii) its efficiency to propagate the ICW decreases when the number of unactivated astrocytes to which it is GJC-coupled increases. Therefore, the propagation efficiency of astrocyte number i, βi, is computed according to
where Nui(t) is the number of astrocytes that are GJC-coupled to i and are not in the activated state.
Now, to determine whether an astrocyte i, which is in the U state at time t will be activated by the ICW at time t + 1, the model computes the sum of the propagation efficiencies of all the astrocytes that are coupled to i, ∑j∈i βj(t) (with
i the set of astrocytes that are GJC-coupled to i). If this sum is larger than a threshold ϑi, i gets activated (i.e., switch from U to A states) with rate kU→A. If the sum of efficiencies is not larger than ϑi, the astrocyte remains in the U state. Formally, we thus define the global probability that an unactivated astrocyte i gets activated by the ICW as:

In the model, the threshold ϑi increases linearly with the astrocyte degree ki (the number of cells it is coupled to), that is ϑi = a · ki + b where the constants a and b where estimated from the ChI model (Supplementary Material S3 and Figure S5B). The other parameters of the simplified model, i.e., the rates kU→A, kA→R and kR→U were estimated from simulations of the ChI model as, respectively, the inverse of the time needed to transmit ICW between two cells (τU), and the inverse of the time spent in the activated (τA) or refractory (τR) states (see Figure 6, parameter values reported in Supplementary Table S1).
The three-state UAR description introduced above is reminiscent of Susceptible–Excited–Refractory (SER) models widely adopted to study network signal propagation (Dodds and Watts, 2004; Müller-Linow et al., 2006; Centola et al., 2007; Müller-Linow et al., 2008; Hütt et al., 2012) except that our definition of pU→Ai takes into account the two-hop neighborhood of each astrocytes, i.e., the activation state of neighbors of the cells coupled to each astrocyte. If the status of activation of the two-hop neighborhood is indeed crucial in ICW propagation, then we expect that the essence of ICW dynamics in the astrocyte networks considered so far, will be reproduced if we substitute the ChI astrocyte model by the UAR description. To test this, we simulated 3D networks with the same topologies than those in Figure 3 except that now, the internal dynamics inside each node are given by the simple stochastic UAR model.
Figure 7 shows the extent of ICW propagation simulated by the simplified UAR model in the same networks as those considered in Figure 3. It is apparent that the UAR model produces a good qualitative match of the results on ICW propagation previously obtained by the ChI model (Figure 3). The number of activated cells (Nact) indeed decreases for either large values of the mean degree (〈k〉, Figure 7A) or small values of the mean shortest path (L, Figure 7B). As in Figure 3, the dependence on the mean shortest path L shows two distinct groups: the propagation ranges of all the coupling organizations where the GJC couplings are strictly restricted to short distance collapse roughly to the same curve, whereas the networks with long-range GJC couplings form another group. As with the detailed ChI model, the propagation range on scale-free networks crossovers from the behavior typical of long-distance couplings to that observed for organizations with short-distances couplings only. Data analysis also shows a comparable decrease of the propagation extent when the fraction of long-distance GJC couplings (ps) increases (Figure 7C). Remarkably, the UAR description even reproduces the distinct propagation extents between cubic lattices and regular networks (Figure 7D).
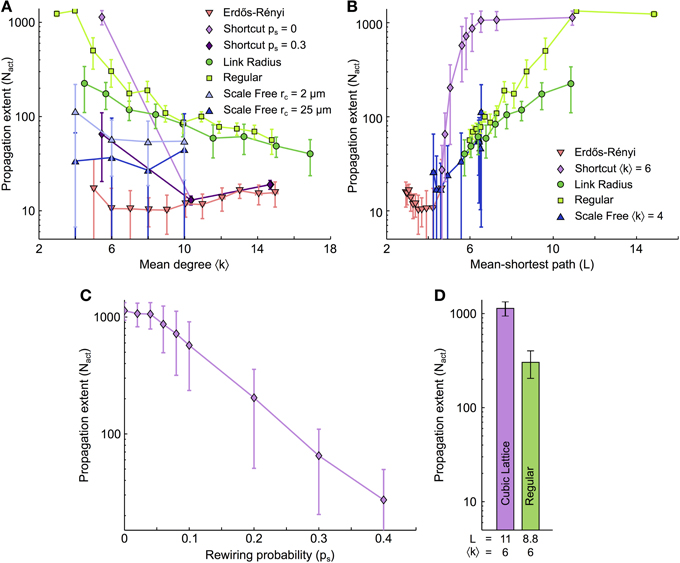
Figure 7. ICW propagation simulated in the simplified model. (A) The extent of ICW propagation (Nact) as a function of 〈k〉 and (B) L was recomputed for the same coupling networks as in Figure 3 but with astrocytes that were modeled with the simplified three–state UAR model. The similarity of Nact values thus obtained compared to those obtained in Figure 3 with the ChI model provides the simplified model with biophysical consistency. This supports our statement that the activation state of the two–hop neighborhood of individual cells (“the cells that are connected to the cells that are connected to me”) is crucial to ICW propagation. (C) In shortcut networks, a large density of long–distance couplings between astrocytes hampers ICW propagation, like with the ChI model in Figure 3. (D) Propagation extent for cubic lattices and regular networks of same mean degree. Data points ± errorbars correspond to mean values ± standard deviation over 20 networks of similar topology. Parameters of the simplified model (see text) as in Supplementary Table S1 (τ values were estimated like in Figure 6).
To conclude, these results confirm that the basic ingredients expressed in the UAR model are sufficient to explain the propagation extent of ICW based on the full bio-realistic ChI model. This confirms that the probability for an astrocyte to propagate an incoming ICW does not only depend on the number of activated cells to which it is coupled (i.e., its 1-hop neighbors), but also on its 2-hop neighborhood, i.e., the cells that are coupled to the cells coupled to this astrocyte.
The Influence of the Coupling Strength is Non–Monotonous
Thus far, our simulations consisted in changing the topology of the GJC network, keeping GJC strength (or conductance) constant and identical for all cell-cell couplings and all stimulations. In this final section, we turn to estimate how ICW propagation range changes when the overall coupling strength varies. We used Link Radius networks for the coupling topology since the properties of this topology (spatially constrained and distributed degrees) are likely closest to real astrocyte networks. All the other simulation parameters were kept unchanged compared to the above results.
We first changed the strength F of all GJC. In order to compare the resulting networks where both the mean degree 〈k〉 and the coupling strength per connection, F, vary, we used the mean GJC strength per cell 〈ΣF〉 = 〈k〉F. As shown in Figure 8, this quantity was found to be a very good predictor of ICW extent. Figure 8A illustrates the propagation range for the case where the coupling strength is the same for every coupled astrocyte pairs. Whatever the mean degree 〈k〉, these results show a non-monotonous behavior of the ICW propagation, with optimal ICW propagation at intermediate coupling strengths. Actually, large coupling strengths (i.e., 〈ΣF〉 > 1 μM.s−1) severely hinder propagation, in agreement with our conclusions above with constant F values and increasing mean degrees 〈k〉. For very low values of F (〈ΣF〉 < 0.1 μM.s−1), ICW propagation is as well blocked because IP3 diffusion is much slower than its degradation. In between those two regimes (i.e., for 0.1 <〈ΣF〉 < 1 μM.s−1), ICW propagation is optimal and actually reaches the whole astrocyte network (regenerative propagation). Therefore, one expects from these data that ICW propagation should be optimal in networks with intermediate coupling strength since large and small strengths both hinder it. Note however that the regenerative propagation observed at intermediate values of 〈ΣF〉 is qualitatively different from the propagation observed at large values (and in the simulations presented in the article thus far). With such intermediate values, the activation of a cell by a Ca2+ wave switches the cell to a “Up” state, with much larger values of IP3 and Ca2+ than before the activation (Figure S11A). The biological relevance of this “Up” state is not firmly ascertained yet.
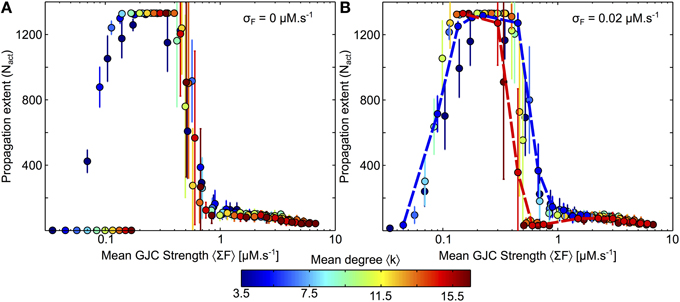
Figure 8. Changes of GJC strength and mean degree in Link Radius networks. (A) In a first approach the strength F of the GJC couplings between two coupled cells are identical for every coupled cell pairs. Whatever the mean degree of the network, the ICW extent is dictated by the mean GJC strength per node 〈ΣF〉 = 〈k〉 F. (B) When the GJC strengths are randomly chosen from a normal distribution, the dependance on 〈ΣF〉 is essentially preserved, except that the optimal range of 〈ΣF〉 values diminishes when the network mean degree is large (dashed red lines). All points represent 20 realization of a given parameter combination. Curves and points are color-coded according to the mean degree 〈k〉. Astrocyte parameters as in Table 1 except for F that was varied between 0 and 0.05 by steps of 0.01 and between 0.1 and 0.4 by steps of 0.05. Link Radius parameters as in Table 2.
Finally, we investigated the effect of randomly distributed GJC strength on ICW propagation. To this end, we picked each GJC strength independently according to a normal distribution with mean F and variance σ2F. To avoid negative values and preserve constant mean, we restricted the random values to [0, 2F]. Figure 8B shows that this distribution of the GJC over cell pairs does not substantially affect ICW propagation. The only notable change is seen for high-degree networks (red dashed curve) for which the end of the optimal (regenerative) regime occurs earlier, i.e., for smaller GJC strengths. The dependence on the network mean degree is amplified when the variability of the GJC strength increases (Figure S11B): the variability of the GJC strength hardly has an effect on low-degree networks, but tends to hinder ICW propagation in high-degree networks. Having low connectivity is thus beneficial for ICW propagation in two ways: (1) it increases ICW extent, for all GJC strength F; (2) it makes the network more robust to variability in GJC strengths.
Discussion
In many instances, the variability observed in the propagation range of intercellular calcium waves (ICW) in astrocyte populations (Charles, 1998; Scemes and Giaume, 2006; Kuga et al., 2011; Sasaki et al., 2011) cannot be accounted for by the type of preparation or by stimulation protocol (Scemes and Giaume, 2006). Our model of ICW in 3D coupling networks suggests that this variability can be due to a mere change in the spatial organization (or topology) of the astrocyte network. In our simulations, the mean degree 〈k〉 and mean-shortest path L of the coupling networks were found to be the main topological characteristics controlling propagation. Quite surprisingly, increasing the number of cells coupled to each astrocyte or adding long–distance GJC couplings actually reduced the extent of ICW propagation. Moreover, all types of ICW propagations ranges could be reproduced in our model with spatially constrained networks (Link Radius and Regular) just by changing 〈k〉. Our model therefore predicts that variations in the organization of the GJC couplings control the range of ICW propagation.
A first experimental element in favor of our hypothesis is the observation that regenerative ICW are far more frequent in cell cultures than in slices or in vivo experiments (Scemes and Giaume, 2006). Since a 2D embedding imposes a lower mean degree (compared to 3D), this observation supports our hypothesis. In vivo, the organization of astrocyte coupling networks has recently attracted attention as several articles demonstrated variability between brain regions (Giaume et al., 2010). For instance, heterogeneities in coupling organization were found in mouse olfactory glomeruli (Roux et al., 2011), and somatosensory cortex (Houades et al., 2008). Locally, astrocyte density may control the astrocyte coupling organization, as in the stratum pyramidale of the hippocampus (Rouach et al., 2008). These local heterogeneities are also reflected in the observation that the total number of coupled astrocytes (obtained via e.g., biocytin-coupling experiments) vary a lot between brain regions: cortical astrocytes can be organized in networks of hundreds of cells (Nimmerjahn et al., 2004) while in the hippocampus, astrocytes in the CA3 region are much less coupled than in CA1 (D'Ambrosio et al., 1998). These variations in coupling can also be at least partially attributed to variations in the expression of connexins (Cx) 43 and 30, which also displays high heterogeneities (Giaume and Theis, 2010); for instance, hypothalamus and hippocampus display higher Cx43 levels than cortex and brain stem astrocytes (Blomstrand et al., 1999). According to our hypothesis, this regional variability of the organization of the astrocyte coupling network could explain the regional variability of the extent of ICW propagations.
While the heterogeneity of the coupling organization is being increasingly recognized (Giaume et al., 2010), only a few studies have addressed the relationship between the coupling properties of the astrocytes (or their Cx expression) and ICW propagation. According to our hypothesis, in regions in which GJC intercellular communication is the main ICW pathway (retina (Newman, 2001), striatum (Venance et al., 1997) and cerebral cortex (Iwabuchi et al., 2002; Haas et al., 2006)), one should observe increased ICW extent when the astrocytes are less coupled, or when Cx expression is lower. Blomstrand et al. (1999) quantified both the extent of dye coupling and ICW propagation as well as Cx43 expression in astrocyte cultures from different brain regions. In accordance with our hypothesis, an inverse relationship between ICW extent and GJC coupling was reported for two brain regions: hypothalamus was found to be highly GJC-coupled and to support small extent ICW whereas the neocortex, that was less GJC-coupled, exhibited larger ICW (Blomstrand et al., 1999). More generally, GJC coupling in regions where intercellular GJC is the predominant pathway for ICW propagation is often reported to be lower than in other brain regions like in the striatum (Rouach et al., 2002) and cortex (Aberg et al., 1999; Blomstrand et al., 1999). In the CA3 region of the hippocampus, known to be less coupled than CA1 (D'Ambrosio et al., 1998), neuronal activity is able to trigger long range ICW (Dani et al., 1992). On the contrary, increased coupling induced by forced expression of Cx43 was found to decrease ICW extent in human 1321N1 astrocytoma cells (Suadicani et al., 2004). Taken together, these articles comfirm our hypothesis: highly coupled astrocyte networks display small extent ICW while less coupled ones display larger ICW.
If astrocytes are indeed organized in independent non–overlapping domains (Bushong et al., 2002), the organization of their coupling can be expected to be close to a Voronoi diagram of the cell centers (Aurenhammer, 1991). The mean degree of a Voronoi diagram in three dimensions is ≈ 15, a value that should prevent ICW propagation according to our simulation results (see Figure 3A for 〈k〉 = 15). Actual astrocyte networks however differ from a pure Voronoi diagram because some of the astrocytes can be disconnected from the GJC network (Theis and Giaume, 2012). For instance, in cocultures of rat striatal neurons and astrocytes, 21% of the astrocytes were found to be disconnected from the network (Rouach et al., 2000). This figure even increases to 40% of disconnection in cultures with only astrocytes. Removing 21 or 40% of the nodes from a Voronoi diagram leads to a mean degree 〈k〉 ≈ 11.7 or 8.9, respectivley. Interestingly, these values of the mean degree are close to values reported in situ: 〈k〉 ≈ 11 neighbors in CA1 rat hippocampus (Xu et al., 2010). Our simulations show a strong increase in the ICW propagation range when the mean degree becomes smaller than ≈ 8 − 10 (Figure 3A). Interestingly, both Cx30 and Cx43 expression and permeability can be regulated by neurons (Rouach et al., 2000; Koulakoff et al., 2008; Roux et al., 2011), possibly via extracellular K+ (Pina-Benabou et al., 2001). This K+-triggered increase in GJC communication was also recently reported to decrease ICW extent in astrocyte networks [see Figure 3 in Scemes and Spray (2012)], in accordance with our hypothesis. Therefore, neurons could modulate mean degree of the astrocyte coupling network and even trigger a switch between 〈k〉 ≈ 12 and 〈k〉 ≈ 8 thus allowing the propagation of ICW to long ranges.
The coupling organization in astrocyte networks also changes during development. During the first postnatal weeks, astrocytes show large increases in Cx43 expression that persists until adulthood (Aberg et al., 1999; Montoro and Yuste, 2004). ICW are frequently observed during development (Parri et al., 2001; Weissman et al., 2004; Fiacco and McCarthy, 2006; Scemes and Giaume, 2006; Kunze et al., 2009) and are thought to be much less frequent in adults under non–pathological conditions (Fiacco and McCarthy, 2006; Scemes and Giaume, 2006). In most parts of the brain, Cx43 becomes strongly expressed between postnatal day 10 (Aberg et al., 1999) and the third postnatal week (Rouach et al., 2002). In agreement with our hypothesis, spontaneous astrocyte calcium activity in the rat ventrobasal thalamus decreases by five fold between postanatal day 10 and 14 (Parri et al., 2001). In mice CA1 stratum radiatum in the hippocampus, realistic stimulation with caged IP3 failed to elicit ICW in individuals ranging from postnatal day 10–14 (Fiacco and McCarthy, 2004). The astrocytes of the neocortex are known to be sparsely coupled during postnatal days 1–3 (Aberg et al., 1999), where ICW were found to be propagating (Iwabuchi et al., 2002). On the opposite, in the CA1 region of the hippocampus, astrocytes are highly coupled during postnatal day 10–14 (Aberg et al., 1999) and they do not support wave propagation with similar stimulation protocols (Fiacco and McCarthy, 2004). Taken together, these observations support our hypothesis, since ICW activity seems to be predominant during early postnatal development when astrocytes are less coupled, and tends to diminish with age as Cx expression gets stronger.
In several regions of the brain, intercellular IP3 transfer through GJC coupling is however not the major pathway for ICW. Indeed, ICW propagation through the activation of P2Y receptors by diffusing extracellular ATP is the dominant pathway in the corpus callosum (Schipke et al., 2002), CA1 hippocampus (Haas et al., 2006) and the cerebellum (Hoogland et al., 2009). In these regions, ICW can be elicited through ATP application and are strongly diminished by P2Y receptor antagonists (Hoogland et al., 2009; Kuga et al., 2011). ATP activation of P2Y1 receptors leads to IP3 production via PLCβ activation but ATP also has a P2Y-mediated effect on GJC permeability, that was reported to decrease gap junction coupling (Rouach et al., 2002; Même et al., 2004; Orellana et al., 2013). A network with initially strong GJC-coupling would not support long range ICW propagation via the intercellular GJC pathway according to our model. However, the release of ATP in such a network, by down-regulating GJC permeability, would decrease the coupling and facilitate ICW propagation. In support of this hypothesis, the expression of P2Y1R in Cx43 expressing cells increases ICW extent while the expression of Cx43 in the absence of P2Y1 reduced it (Suadicani et al., 2004). Collectively, these articles also support our hypothesis and offer an attractive perspective: long-range ICW can be elicited in an astrocyte network (i) if its mean degree of GJC coupling is low - for regions in which GJC is the predominant pathway; or (ii) if the mean degree is large, for regions in which ATP is the predominant pathway, but if propagation is rescued by the down-regulation of GJC permeability by ATP.
The topological determinants of signal propagation in astrocyte networks thus seem different from those at play in neuronal networks. Signal is propagated from one astrocyte to the other by diffusion from a single IP3 pool (the astrocyte) while neurons communicating through chemical synapses use distinct pools of neurotransmitters located in each of their synapses. This difference is actually the strongest one in our view: while increasing the number of neighbors in astrocyte networks dilutes away IP3 and decreases ICW propagation, increasing the number of neighbors in neuronal networks only implies the addition of new synapses and can thus only increase the network excitability. In agreement with this view, increased connectivity (i.e., mean degree) has been shown to promote synchronization in model networks of excitable neurons (Wang et al., 1995; Golomb and Hansel, 2000) and to control the switch between asynchronized states and partially synchronized (or coherent) states (Olmi et al., 2010; Luccioli et al., 2012; Tattini et al., 2012). In the present study, the presence of hubs and long range connections between astrocytes impaired ICW extent. In contrast, broad in-degree distributions (allowing the presence of hubs) has been shown to increase the mean activity in model neuronal networks while broad out-degree distributions increased the amplitude of cross-correlation in synaptic currents (Roxin, 2011). Finally, Dyhrfjeld-Johnsen et al. (2007) showed that network hyperexcitability during simulated sclerosis can be directly linked to the presence of long distance links in the network: when these long distance links were removed during maximal sclerosis, network hyperexcitability decreased. Theoretical studies suggested that to keep network activity balanced, synaptic weights of each neuron should be rescaled by some function of the neuron degree (Van Vreeswijk and Sompolinsky, 1996, 1998; Lerchner et al., 2006). This synaptic scaling mechanism has mainly been observed experimentally for post-synaptic terminals (Turrigiano, 2008). In astrocyte networks, one could wonder whether such a mechanism would influence ICW propagation. Unfortunately, because of the bidirectionality of these networks, rescaling GJC strength is not as straightforward as for neuronal networks (conductances in both direction should be equal gij = gji but ki can be different from kj). Neuronal networks can also be bidirectional when neurons are coupled by GJC-mediated electrical synapses. While being restricted to certain adult neuronal subpopulations (Söhl et al., 2005), these GJC are still functionally relevant and have been shown to mediate synchronization between neurons both experimentally (Connors and Long, 2004) and in modeling studies (Chow and Kopell, 2000; Lewis and Rinzel, 2003). The effect of GJC topological properties such as connectivity and rewiring on signal propagation could however be more subtle. Because of the similarity between the term governing the diffusion of IP3 between astrocytes (F × (Ii − Ij)) and the term governing GJC-coupling at electrical synapses (g × (Vi − Vj)), GJC-coupled neuronal networks are of particular relevance to our study. Accordingly, the effects of network topology on signal propagation in GJC-coupled neuronal networks bear some resemblance with the effects described here for astrocyte networks. In Volman et al. (2011), it is shown that increasing GJC conductance or connectivity could help reduce epileptic seizures by mechanisms similar to what we observed in astrocyte networks (subthreshold activity gets diluted among neighbors). Further increases in connectivity however enhanced seizure activity (and thus signal propagation), in contrast with what we observed in astrocyte networks. Increased rewiring of GJC-coupled model neurons has been shown to impair signal detection but, differently from astrocyte networks, weak connectivity and coupling, while enhancing signal detection, impaired signal propagation (Volman and Perc, 2010). Altogether, those behaviors reported for neuronal networks do not match the observations we reported here for model astrocyte networks. These differences in the dynamics-topology relationships bring new light on the well-known observation that neurons form highly connected networks with long distance links while astrocytes usually restrict their couplings to a handful of nearby neighbors (Bushong et al., 2002). The topology of each of these cellular networks thus appears adapted for optimal signal transmission.
Fundings
This study was funded by the High Council for Scientific and Technological Cooperation between France and Israel (Jules Lallouette, Hugues Berry), by the ERCIM “Alain Bensoussan” Fellowship (Maurizio De Pittà), and by the Italian–Israeli Joint Neuroscience Lab (Eshel Ben-Jacob, Maurizio De Pittà). The authors also acknowledge the support of the computing center IN2P3 at CNRS (http://cc.in2p3.fr), where the simulations were performed.
Conflict of Interest Statement
The authors declare that the research was conducted in the absence of any commercial or financial relationships that could be construed as a potential conflict of interest.
Supplementary Material
The Supplementary Material for this article can be found online at: http://www.frontiersin.org/journal/10.3389/fncom.2014.00045/abstract
References
Aberg, N. D., Rönnbäck, L., and Eriksson, P. S. (1999). Connexin43 mRNA and protein expression during postnatal development of defined brain regions. Dev. Brain Res. 115, 97–101. doi: 10.1016/S0165-3806(99)00052-8
Agulhon, C., Fiacco, T. A., and McCarthy, K. D. (2010). Hippocampal short- and long-term plasticity are not modulated by astrocyte Ca2+ signaling. Science 327, 1250–1254. doi: 10.1126/science.1184821
Agulhon, C., Petravicz, J., McMullen, A. B., Sweger, E. J., Minton, S. K., Taves, S. R., et al. (2008). What is the role of astrocyte calcium in neurophysiology? Neuron 59, 932–946. doi: 10.1016/j.neuron.2008.09.004
Albert, R., and Barabási, A.-L. (2002). Statistical mechanics of complex networks. Rev. Mod. Phys. 74, 47–97. doi: 10.1103/RevModPhys.74.47
Arcuino, G., Lin, J. H.-C., Takano, T., Liu, C., Jiang, L., Gao, Q., et al. (2002). Intercellular calcium signaling mediated by point-source burst release of ATP. Proc. Natl. Acad. Sci. U.S.A. 99, 9840–9845. doi: 10.1073/pnas.152588599
Aurenhammer, F. (1991). Voronoi diagramsa survey of a fundamental geometric data structure. ACM Comput. Surv. 23, 345–405. doi: 10.1145/116873.116880
Blomstrand, F., Aberg, N. D., Eriksson, P. S., Hansson, E., and Rönnbäck, L. (1999). Extent of intercellular calcium wave propagation is related to gap junction permeability and level of connexin-43 expression in astrocytes in primary cultures from four brain regions. Neuroscience 92, 255–265. doi: 10.1016/S0306-4522(98)00738-6
Boccaletti, S., Latora, V., Moreno, Y., Chavez, M., and Hwang, D.-U. (2006). Complex networks: structure and dynamics. Phys. Rep. 424, 175–308. doi: 10.1016/j.physrep.2005.10.009
Bushong, E. A., Martone, M. E., Jones, Y. Z., and Ellisman, M. H. (2002). Protoplasmic astrocyte in CA1 stratum radiatum occupy separate anatomical domains. J. Neurosci. 22, 183–192.
Carmignoto, G. (2000). Reciprocal communication systems between astrocytes and neurones. Prog. Neurobiol. 62, 561–581. doi: 10.1016/S0301-0082(00)00029-0
Centola, D., Eguíluz, V. M., and Macy, M. W. (2007). Cascade dynamics of complex propagation. Physica A 374, 449–456. doi: 10.1016/j.physa.2006.06.018
Charles, A. C., and Giaume, C. (2002). “Intercellular calcium waves in astrocytes: underlying mechanisms and functional significance,” in Tripartite Synapses: Synaptic Transmission With Glia, eds A. Volterra, P. Magistretti, and P. Haydon (Oxford: Oxford University Press), 110–126.
Chow, C. C., and Kopell, N. (2000). Dynamics of spiking neurons with electrical coupling. Neural Comput. 12, 1643–1678. doi: 10.1162/089976600300015295
Connors, B. W., and Long, M. A. (2004). Electrical synapses in the mammalian brain. Annu. Rev. Neurosci. 27, 393–418. doi: 10.1146/annurev.neuro.26.041002.131128
Costa, L. D. F., and da Rocha, L. E. C. (2006). A generalized approach to complex networks. Eur. Phys. J. B 50, 237–242. doi: 10.1140/epjb/e2006-00107-0
D'Ambrosio, R., Wenzel, J., Schwartzkroin, P. A., McKhann, G. M., and Janigro, D. (1998). Functional specialization and topographic segregation of hippocampal astrocytes. J. Neurosci. 18, 4425–4438.
Dani, J. W., Chernjavsky, A., and Smith, S. J. (1992). Neuronal activity triggers calcium waves in hippocampal astrocyte networks. Neuron 8, 429–440. doi: 10.1016/0896-6273(92)90271-E
De Pittà, M., Goldberg, M., Volman, V., Berry, H., and Ben-Jacob, E. (2009). Glutamate-dependent intracellular calcium and IP3 oscillating and pulsating dynamics in astrocytes. J. Biol. Phys. 35, 383–411. doi: 10.1007/s10867-009-9155-y
De Pittà, M., Volman, V., Berry, H., Parpura, V., Liaudet, N., Volterra, A., et al. (2012). Computational quest for understanding the role of astrocyte signaling in synaptic transmission and plasticity. Front. Comput. Neurosci. 6:98. doi: 10.3389/fncom.2012.00098
Dodds, P. S., and Watts, D. J. (2004). Universal behavior in a generalized model of contagion. Phys. Rev. Lett. 92, 218701. doi: 10.1103/PhysRevLett.92.218701
Dyhrfjeld-Johnsen, J., Santhakumar, V., Morgan, R. J., Huerta, R., Tsimring, L., and Soltesz, I. (2007). Topological determinants of epileptogenesis in large-scale structural and functional models of the dentate gyrus derived from experimental data. J. Neurophysiol. 97, 1566–1587. doi: 10.1152/jn.00950.2006
Feldt, S., Wang, J. X., Shtrahman, E., Dzakpasu, R., Olariu, E., and Zochowski, M. (2010). Functional clustering in hippocampal cultures: relating network structure and dynamics. Phys. Biol. 7, 046004. doi: 10.1088/1478-3975/7/4/046004
Fiacco, T. A., and McCarthy, K. D. (2004). Intracellular astrocyte calcium waves in situ increase the frequency of spontaneous AMPA receptor currents in CA1 pyramidal neurons. J. Neurosci. 24, 722–732. doi: 10.1523/JNEUROSCI.2859-03.2004
Fiacco, T. A., and McCarthy, K. D. (2006). Astrocyte calcium elevations: properties, propagation, and effects on brain signaling. Glia 54, 676–690. doi: 10.1002/glia.20396
Giaume, C., Koulakoff, A., Roux, L., Holcman, D., and Rouach, N. (2010). Astroglial networks: a step further in neuroglial and gliovascular interactions. Nat. Rev. Neurosci. 11, 87–99. doi: 10.1038/nrn2757
Giaume, C., and Theis, M. (2010). Pharmacological and genetic approaches to study connexin-mediated channels in glial cells of the central nervous system. Brain Res. Rev. 63, 160–176. doi: 10.1016/j.brainresrev.2009.11.005
Giaume, C., and Venance, L. (1998). Intracellular calcium signaling and gap junctional communication in astrocytes. Glia 24, 50–64.
Goldberg, M., De Pittà, M., Volman, V., Berry, H., and Ben-Jacob, E. (2010). Nonlinear gap junctions enable long-distance propagation of pulsating calcium waves in astrocyte networks. PLoS Comput. Biol. 6:e1000909. doi: 10.1371/journal.pcbi.1000909
Golomb, D., and Hansel, D. (2000). The number of synaptic inputs and the synchrony of large, sparse neuronal networks. Neural comput. 12, 1095–1139. doi: 10.1162/089976600300015529
Guthrie, P. B., Knappenberger, J., Segal, M., Bennett, M. V. L., Charles, A. C., and Kater, S. B. (1999). ATP released from astrocytes mediates glial calcium waves. J. Neurosci. 19, 520–528.
Haas, B., Schipke, C. G., Peters, O., SÃűhl, G., Willecke, K., and Kettenmann, H. (2006). Activity-dependent ATP-waves in the mouse neocortex are independent from astrocytic calcium waves. Cereb. Cortex 16, 237–246. doi: 10.1093/cercor/bhi101
Haydon, P. G. (2001). Glia: listening and talking to the synapse. Nat. Rev. Neurosci. 2, 185–193. doi: 10.1038/35058528
Haydon, P. G., and Carmignoto, G. (2006). Astrocyte control of synaptic transmission and neurovascular coupling. Physiol. Rev. 86, 1009–1031. doi: 10.1152/physrev.00049.2005
Hirase, H., Qian, L., Barthó, P., and Buzsáki, G. (2004). Calcium dynamics of cortical astrocytic networks in vivo. PLoS Biol. 2:E96. doi: 10.1371/journal.pbio.0020096
Höfer, T., Venance, L., and Giaume, C. (2002). Control and plasticity of intercellular calcium waves in astrocytes: a modeling approach. J. Neurosci. 22, 4850–4859.
Hoogland, T. M., Kuhn, B., Göbel, W., Huang, W., Nakai, J., Helmchen, F., et al. (2009). Radially expanding transglial calcium waves in the intact cerebellum. Proc. Natl. Acad. Sci. U.S.A. 106, 3496–3501. doi: 10.1073/pnas.0809269106
Houades, V., Koulakoff, A., Ezan, P., Seif, I., and Giaume, C. (2008). Gap junction-mediated astrocytic networks in the mouse barrel cortex. J. Neurosci. 28, 5207–5217. doi: 10.1523/JNEUROSCI.5100-07.2008
Houades, V., Rouach, N., Ezan, P., Kirchhoff, F., Koulakoff, A., and Giaume, C. (2006). Shapes of astrocyte networks in the juvenile brain. Neuron Glia Biol. 2, 3–14. doi: 10.1017/S1740925X06000081
Hütt, M.-T., Jain, M. K., Hilgetag, C. C., and Lesne, A. (2012). Stochastic resonance in discrete excitable dynamics on graphs. Chaos Solit. Fract. 45, 611–618. doi: 10.1016/j.chaos.2011.12.011
Iacobas, D. A., Suadicani, S. O., Spray, D. C., and Scemes, E. (2006). A stochastic two-dimensional model of intercellular Ca2+ wave spread in glia. Biophys. J. 90, 24–41. doi: 10.1529/biophysj.105.064378
Isham, V., Kaczmarska, J., and Nekovee, M. (2011). Spread of information and infection on finite random networks. Phys. Rev. E 83, 046128. doi: 10.1103/PhysRevE.83.046128
Iwabuchi, S., Kawahara, K., Makisaka, K., and Sato, H. (2002). Photolytic flash-induced intercellular calcium waves using caged calcium ionophore in cultured astrocytes from newborn rats. Exp. Brain. Res. 146, 103–116. doi: 10.1007/s00221-002-1149-y
Kettenmann, H., and Ransom, B. R. (2004). Neuroglia, The Concept of Neuroglia: A Historical Perspective, 1st Edn., New York, NY: Oxford University Press.
Koulakoff, A., Ezan, P., and Giaume, C. (2008). Neurons control the expression of connexin 30 and connexin 43 in mouse cortical astrocytes. Glia 56, 1299–1311. doi: 10.1002/glia.20698
Kuga, N., Sasaki, T., Takahara, Y., Matsuki, N., and Ikegaya, Y. (2011). Large-scale calcium waves traveling through astrocytic networks in vivo. J. Neurosci. 31, 2607–2614. doi: 10.1523/JNEUROSCI.5319-10.2011
Kunze, A., Congreso, M. R., Hartmann, C., Wallraff-Beck, A., Hüttmann, K., Bedner, P., et al. (2009). Connexin expression by radial glia-like cells is required for neurogenesis in the adult dentate gyrus. Proc. Natl. Acad. Sci. U.S.A. 106, 11336–11341. doi: 10.1073/pnas.0813160106
Lerchner, A., Ursta, C., Hertz, J., Ahmadi, M., Ruffiot, P., and Enemark, S. (2006). Response variability in balanced cortical networks. Neural comput. 18, 634–659. doi: 10.1162/neco.2006.18.3.634
Lewis, T. J., and Rinzel, J. (2003). Dynamics of spiking neurons connected by both inhibitory and electrical coupling. J. Comput. Neurosci. 14, 283–309. doi: 10.1023/A:1023265027714
Luccioli, S., Olmi, S., Politi, A., and Torcini, A. (2012). Collective dynamics in sparse networks. Phys. Rev. Lett. 109, 138103. doi: 10.1103/PhysRevLett.109.138103
Même, W., Ezan, P., Venance, L., Glowinski, J., and Giaume, C. (2004). ATP-induced inhibition of gap junctional communication is enhanced by interleukin-1 β treatment in cultured astrocytes. Neuroscience 126, 95–104. doi: 10.1016/j.neuroscience.2004.03.031
Montoro, R. J., and Yuste, R. (2004). Gap junctions in developing neocortex: a review. Brain Res. Rev. 47, 216–226. doi: 10.1016/j.brainresrev.2004.06.009
Müller-Linow, M., Hilgetag, C. C., and Hütt, M.-T. (2008). Organization of excitable dynamics in hierarchical biological networks. PLoS Comput. Biol. 4:e1000190. doi: 10.1371/journal.pcbi.1000190
Müller-Linow, M., Marr, C., and Hütt, M.-T. (2006). Topology regulates the distribution pattern of excitations in excitable dynamics on graphs. Phys. Rev. E 74, 016112. doi: 10.1103/PhysRevE.74.016112
Nagy, J. I., and Rash, J. E. (2000). Connexins and gap junctions of astrocytes and oligodendrocytes in the CNS. Brain Res. Rev. 32, 29–44. doi: 10.1016/S0165-0173(99)00066-1
Nedergaard, M., Ransom, B. R., and Goldman, S. A. (2003). New roles for astrocytes: redefining the functional architecture of the brain. Trends Neurosci. 26, 523–530. doi: 10.1016/j.tins.2003.08.008
Newman, E. A. (2001). Propagation of intercellular calcium waves in retinal astrocytes and müller cells. J. Neurosci. 21, 2215–2223.
Nimmerjahn, A. (2009). Astrocytes going live: advances and challenges. J. Physiol. 587, 1639–1647. doi: 10.1113/jphysiol.2008.167171
Nimmerjahn, A., Kirchhoff, F., Kerr, J. N. D., and Helmchen, F. (2004). Sulforhodamine 101 as a specific marker of astroglia in the neocortex in vivo. Nat. Methods 1, 31–37. doi: 10.1038/nmeth706
Olmi, S., Livi, R., Politi, A., and Torcini, A. (2010). Collective oscillations in disordered neural networks. Phys. Rev. E 81, 046119. doi: 10.1103/PhysRevE.81.046119
Orellana, J. A., Martinez, A. D., and Retamal, M. A. (2013). Gap junction channels and hemichannels in the CNS: regulation by signaling molecules. Neuropharmacology 75, 567–582. doi: 10.1016/j.neuropharm.2013.02.020
Pannasch, U., and Rouach, N. (2013). Emerging role for astroglial networks in information processing: from synapse to behavior. Trends. Neurosci. 36, 405–417. doi: 10.1016/j.tins.2013.04.004
Parri, H. R., Gould, T. M., and Crunelli, V. (2001). Spontaneous astrocytic Ca2+ oscillations in situ drive NMDAR-mediated neuronal excitation. Nat. Neurosci. 4, 803–812. doi: 10.1038/90507
Pasti, L., Volterra, A., Pozzan, T., and Carmignoto, G. (1997). Intracellular calcium oscillations in astrocytes: a highly plastic, bidirectional form of communication between neurons and astrocytes in situ. J. Neurosci. 17, 7817–7830.
Perea, G., Navarrete, M., and Araque, A. (2009). Tripartite synapse: astrocytes process and control synaptic information. Trends Neurosci. 32, 421–431. doi: 10.1016/j.tins.2009.05.001
Peters, J. L., Earnest, B. J., Tjalkens, R. B., Cassone, V. M., and Zoran, M. J. (2005). Modulation of intercellular calcium signaling by melatonin in avian and mammalian astrocytes is brain region-specific. J. Comp. Neurol. 493, 370–380. doi: 10.1002/cne.20779
Pina-Benabou, M. H. D., Srinivas, M., Spray, D. C., and Scemes, E. (2001). Calmodulin kinase pathway mediates the K+-induced increase in gap junctional communication between mouse spinal cord astrocytes. J. Neurosci. 21, 6635–6643.
Rela, L., Bordey, A., and Greer, C. A. (2010). Olfactory ensheathing cell membrane properties are shaped by connectivity. Glia 58, 665–678. doi: 10.1002/glia.20953
Rouach, N., Avignone, E., Même, W., Koulakoff, A., Venance, L., Blomstrand, F., et al. (2002). Gap junctions and connexin expression in the normal and pathological central nervous system. Biol. Cell. 94, 457–475. doi: 10.1016/S0248-4900(02)00016-3
Rouach, N., Glowinski, J., and Giaume, C. (2000). Activity-dependent neuronal control of gap-junctional communication in astrocytes. J. Cell. Biol. 149, 1513–1526. doi: 10.1083/jcb.149.7.1513
Rouach, N., Koulakoff, A., Abudara, V., Willecke, K., and Giaume, C. (2008). Astroglial metabolic networks sustain hippocampal synaptic transmission. Science 322, 1551–1555. doi: 10.1126/science.1164022
Roux, L., Benchenane, K., Rothstein, J. D., Bonvento, G., and Giaume, C. (2011). Plasticity of astroglial networks in olfactory glomeruli. Proc. Natl. Acad. Sci. U.S.A. 108, 18442–18446. doi: 10.1073/pnas.1107386108
Roxin, A. (2011). The role of degree distribution in shaping the dynamics in networks of sparsely connected spiking neurons. Front. Comput. Neurosci. 5:8. doi: 10.3389/fncom.2011.00008
Sasaki, T., Kuga, N., Namiki, S., Matsuki, N., and Ikegaya, Y. (2011). Locally synchronized astrocytes. Cereb. Cortex 21, 1889–1900. doi: 10.1093/cercor/bhq256
Scemes, E., and Giaume, C. (2006). Astrocyte calcium waves: what they are and what they do. Glia 54, 716–725. doi: 10.1002/glia.20374
Scemes, E., and Spray, D. C. (2012). Extracellular K+ and astrocyte signaling via connexin and pannexin channels. Neurochem. Res. 37, 2310–2316. doi: 10.1007/s11064-012-0759-4
Scemes, E., Suadicani, S. O., and Spray, D. C. (2000). Intercellular communication in spinal cord astrocytes: fine tuning between gap junctions and P2 nucleotide receptors in calcium wave propagation. J. Neurosci. 20, 1435–1445.
Schipke, C., Haas, B., and Kettenmann, H. (2008). Astrocytes discriminate and selectively respond to the activity of a subpopulation of neurons within the barrel cortex. Cereb. Cortex 18, 2450–2459. doi: 10.1093/cercor/bhn009
Schipke, C. G., Boucsein, C., Ohlemeyer, C., Kirchhoff, F., and Kettenmann, H. (2002). Astrocyte Ca2+ waves trigger responses in microglial cells in brain slices. FASEB J. 16, 255–257. doi: 10.1096/fj.01-0514fje
Söhl, G., Maxeiner, S., and Willecke, K. (2005). Expression and functions of neuronal gap junctions. Nat. Rev. Neurosci. 6, 191–200. doi: 10.1038/nrn1627
Suadicani, S. O., Flores, C. E., Urban-Maldonado, M., Beelitz, M., and Scemes, E. (2004). Gap junction channels coordinate the propagation of intercellular Ca2+ signals generated by P2Y receptor activation. Glia 48, 217–229. doi: 10.1002/glia.20071
Sul, J.-Y., Orosz, G., Givens, R. S., and Haydon, P. G. (2004). Astrocytic connectivity in the hippocampus. Neuron Glia Biol. 1, 3–11. doi: 10.1017/S1740925X04000031
Sun, W., McConnell, E., Pare, J.-F., Xu, Q., Chen, M., Peng, W., et al. (2013). Glutamate-dependent neuroglial calcium signaling differs between young and adult brain. Science 339, 197–200. doi: 10.1126/science.1226740
Tattini, L., Olmi, S., and Torcini, A. (2012). Coherent periodic activity in excitatory erdös-renyi neural networks: the role of network connectivity. Chaos 22, 023133. doi: 10.1063/1.4723839
Theis, M., and Giaume, C. (2012). Connexin-based intercellular communication and astrocyte heterogeneity. Brain Res. 1487, 88–98. doi: 10.1016/j.brainres.2012.06.045
Tian, G. F., Takano, T., Lin, J. H.-C., Wang, X., Bekar, L., and Nedergaard, M. (2006). Imaging of cortical astrocytes using 2-photon laser scanning microscopy in the intact mouse brain. Adv. Drug Deliv. Rev. 58, 773–787. doi: 10.1016/j.addr.2006.07.001
Turrigiano, G. G. (2008). The self-tuning neuron: synaptic scaling of excitatory synapses. Cell 135, 422–435. doi: 10.1016/j.cell.2008.10.008
Van Vreeswijk, C., and Sompolinsky, H. (1996). Chaos in neuronal networks with balanced excitatory and inhibitory activity. Science 274, 1724–1726. doi: 10.1126/science.274.5293.1724
Van Vreeswijk, C., and Sompolinsky, H. (1998). Chaotic balanced state in a model of cortical circuits. Neural Comput. 10, 1321–1371. doi: 10.1162/089976698300017214
Venance, L., Stella, N., Glowinski, J., and Giaume, C. (1997). Mechanism involved in initiation and propagation of receptor-induced intercellular calcium signaling in cultured rat astrocytes. J. Neurosci. 17, 1981–1992.
Volman, V., and Perc, M. (2010). Fast random rewiring and strong connectivity impair subthreshold signal detection in excitable networks. New J. Phys. 12, 043013. doi: 10.1088/1367-2630/12/4/043013
Volman, V., Perc, M., and Bazhenov, M. (2011). Gap junctions and epileptic seizures - two sides of the same coin? PLoS ONE 6:e20572. doi: 10.1371/journal.pone.0020572
Volterra, A., and Meldolesi, J. (2005). Astrocytes, from brain glue to communication elements: the revolution continues. Nat. Rev. Neurosci. 6, 626–640. doi: 10.1038/nrn1722
Wang, S. S.-H., Alousi, A. A., and Thompson, S. H. (1995). The lifetime of inositol 1,4,5-trisphosphate in single cells. J. Gen. Physiol. 105, 149–171. doi: 10.1085/jgp.105.1.149
Watts, D. J. (1999). Small Worlds: The Dynamics of Ne Between Order and Randomness, Chapter 2. Princeton: Princeton University Press, 33–36.
Weissman, T. A., Riquelme, P. A., Ivic, L., Flint, A. C., and Kriegstein, A. R. (2004). Calcium waves propagate through radial glial cells and modulate proliferation in the developing neocortex. Neuron 43, 647–661. doi: 10.1016/j.neuron.2004.08.015
Xu, G., Wang, W., Kimelberg, H. K., and Zhou, M. (2010). Electrical coupling of astrocytes in rat hippocampal slices under physiological and simulated ischemic conditions. Glia 58, 481–493. doi: 10.1002/glia.20939
Zanette, D. H. (2002). Dynamics of rumor propagation on small-world networks. Phys. Rev. E 65, 041908. doi: 10.1103/PhysRevE.65.041908
Zonta, M., and Carmignoto, G. (2002). Calcium oscillations encoding neuron-to-astrocyte communication. J. Physiol. Paris 96, 193–198. doi: 10.1016/S0928-4257(02)00006-2
Keywords: glial cells, astrocytes, gap-junctions, wave propagation, network topology
Citation: Lallouette J, De Pittà M, Ben-Jacob E and Berry H (2014) Sparse short-distance connections enhance calcium wave propagation in a 3D model of astrocyte networks. Front. Comput. Neurosci. 8:45. doi: 10.3389/fncom.2014.00045
Received: 18 December 2013; Accepted: 27 March 2013;
Published online: 16 April 2014.
Edited by:
David Hansel, University of Paris, FranceReviewed by:
Laurent Venance, Collège de France, FranceJulijana Gjorgjieva, Harvard University, USA
Copyright © 2014 Lallouette, De Pittà, Ben-Jacob and Berry. This is an open-access article distributed under the terms of the Creative Commons Attribution License (CC BY). The use, distribution or reproduction in other forums is permitted, provided the original author(s) or licensor are credited and that the original publication in this journal is cited, in accordance with accepted academic practice. No use, distribution or reproduction is permitted which does not comply with these terms.
*Correspondence: Hugues Berry, EPI Beagle, LIRIS, INRIA Rhône-Alpes, UMR5205, Université de Lyon, Batiment CEI-1, 66 Blvd Niels Bohr, 69100 Villeurbanne, France e-mail:aHVndWVzLmJlcnJ5QGlucmlhLmZy