Open Access
ARTICLE
Toward Secure Software-Defined Networks Using Machine Learning: A Review, Research Challenges, and Future Directions
1 Faculty of Information and Communication Technology, Universiti Tunku Abdul Rahman, Kampar, Perak, Malaysia
2 Higher Colleges of Technology, Fujairah Men’s Campus, Fujairah, UAE
* Corresponding Author: Muhammad Waqas Nadeem. Email:
Computer Systems Science and Engineering 2023, 47(2), 2201-2217. https://doi.org/10.32604/csse.2023.039893
Received 22 February 2023; Accepted 09 May 2023; Issue published 28 July 2023
Abstract
Over the past few years, rapid advancements in the internet and communication technologies have led to increasingly intricate and diverse networking systems. As a result, greater intelligence is necessary to effectively manage, optimize, and maintain these systems. Due to their distributed nature, machine learning models are challenging to deploy in traditional networks. However, Software-Defined Networking (SDN) presents an opportunity to integrate intelligence into networks by offering a programmable architecture that separates data and control planes. SDN provides a centralized network view and allows for dynamic updates of flow rules and software-based traffic analysis. While the programmable nature of SDN makes it easier to deploy machine learning techniques, the centralized control logic also makes it vulnerable to cyberattacks. To address these issues, recent research has focused on developing powerful machine-learning methods for detecting and mitigating attacks in SDN environments. This paper highlighted the countermeasures for cyberattacks on SDN and how current machine learning-based solutions can overcome these emerging issues. We also discuss the pros and cons of using machine learning algorithms for detecting and mitigating these attacks. Finally, we highlighted research issues, gaps, and challenges in developing machine learning-based solutions to secure the SDN controller, to help the research and network community to develop more robust and reliablesolutions.Keywords
Cite This Article
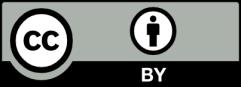
This work is licensed under a Creative Commons Attribution 4.0 International License , which permits unrestricted use, distribution, and reproduction in any medium, provided the original work is properly cited.