Paper:
A Robot in a Human–Robot Group Learns Group Norms and Makes Decisions Through Indirect Mutual Interaction with Humans
Yotaro Fuse*, Hiroshi Takenouchi**, and Masataka Tokumaru***
*Kansai University Graduate School
3-3-35 Yamate-cho, Suita-shi, Osaka 564-8680, Japan
**Fukuoka Institute of Technology
3-30-1 Wajiro-higashi, Higashi-ku, Fukuoka 811-0295, Japan
***Kansai University
3-3-35 Yamate-cho, Suita-shi, Osaka 564-8680, Japan
In this study, we investigate whether group norms occur in human–robot groups. At present, there are a number of studies that examine social robots’ ways of responding, gesturing, and displaying emotion. However, sociality implies that robots not only exhibit human-like behaviors, but also display the tendency to adapt to a group of individuals. For robots to exhibit sociality, they must adapt to group norms without being told by the group members how to behave. Group norms refer to the unwritten, unspoken, and informal rules that are present in a group of individuals. In a previous study, we demonstrated that a robot model learned group norms in human groups [1]. In the present study, we investigate whether group norms occur in human–robot groups. To this end, we prepared quizzes with unclear and vague answers, and instructed participants to take the quizzes with the robot. The results of the quiz experiments demonstrated that the robot considered group norms in human–robot groups when making decisions; thus, group norms occurred in human–robot groups.
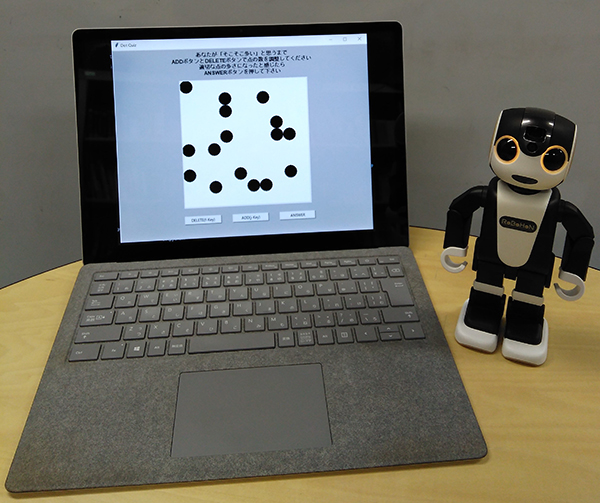
Robot and laptop used in experiment
- [1] Y. Fuse, H. Takenouchi, and M. Tokumaru, “A Robot Model that Obeys a Norm of a Human Group by Participating in the Group and Interacting with its Members,” IEICE Trans. on Information and Systems, Vol.E102.D, Issue 1, pp. 185-194, 2019.
- [2] F. Hegel, C. Muhl, B. Wrede, M. Hielscher-Fastabend, and G. Sagerer, “Understanding Social Robots,” 2009 2nd Int. Conf. on Advances in Computer-Human Interactions (ACHI’09), pp. 169-174, 2009.
- [3] T. Fong, I. Nourbakhsh, and K. Dautenhahn, “A survey of socially interactive robots,” Robotics and Autonomous Systems, Vol.42, Issues 3-4, pp. 143-166, 2003.
- [4] B. R. Duffy, “Anthropomorphism and the Social Robot,” Robotics and Autonomous Systems, Vol.42, Issues 3-4, pp. 177-190, 2003.
- [5] C. Breazeal, “Designing sociable robots,” MIT Press, 2004.
- [6] M. Sherif, “A study of some social factors in perception,” Archives of Psychology, Vol.27, Issue 187, pp. 1-60, 1935.
- [7] D. J. Terry and M. A. Hogg, “Group norms and the attitude-behavior relationship: A role for group identification,” Personality and Social Psychology Bulletin, Vol.22, Issue 8, pp. 776-793, 1996.
- [8] D. C. Feldman, “The development and enforcement of group norms,” Academy of Management Review, Vol.9, No.1, pp. 47-53, 1984.
- [9] E. M. Aminoff, D. Clewett, S. Freeman, A. Frithsen, C. Tipper, A. Johnson, S. T. Grafton, and M. B. Miller, “Individual differences in shifting decision criterion: A recognition memory study,” Memory & Cognition, Vol.40, Issue 7, pp. 1016-1030, 2012.
- [10] M. Deutsch and H. B. Gerard, “A study of normative and informational social influences upon individual judgement,” The J. of Abnormal and Social Psychology, Vol.51, Issue 3, pp. 629-636, 1955.
- [11] L. S. Rashotte, “Social Influence,” G. Ritzer and J. M. Ryan (Eds.), “The Blackwell Encyclopedia of Sociology,” Vol.IX, pp. 4426-4429, Oxford: Blackwell Publishing, 2007.
- [12] R. B. Cialdini, “Influence: science and practice,” HarperCollins, 2009.
- [13] P. Robinette et al., “Overtrust of robots in emergency evacuation scenarios,” Proc. of 2016 11th ACM/IEEE Int. Conf. on Human-Robot Interaction (HRI’16), pp. 101-108, 2016.
- [14] N. Salomons et al., “Humans Conform to Robots: Disambiguating Trust, Truth, and Conformity,” Proc. of the 2018 ACM/IEEE Int. Conf. on Human-Robot Interaction (HRI’18), pp. 187-195, 2018.
- [15] J. Brandstetter et al., “A peer pressure experiment: Recreation of the Asch conformity experiment with robots,” 2014 IEEE/RSJ Int. Conf. on Intelligent Robots and Systems, pp. 1335-1340, 2014.
- [16] R. Williams et al., ““My doll says it’s ok”: a study of children’s conformity to a talking doll,” Proc. of the 17th ACM Conf. on Interaction Design and Children, pp. 625-631, 2018.
- [17] N. Crichton, “Visual analogue scale (VAS),” J. of Clinical Nursing, Vol.10, Issue 5, pp. 697-706, 2001.
- [18] L. P. Kaelbling, M. L. Littman, and A. W. Moore, “Reinforcement learning: A survey,” J. of Artificial Intelligence Research, Vol.4, pp. 237-285, 1996.
- [19] J. Kober, J. A. Bagnell, and J. Peters, “Reinforcement learning in robotics: A survey,” The Int. J. of Robotics Research, Vol.32, Issue 11, pp. 1238-1274, 2013.
- [20] K. Arulkumaran, M. P. Deisenroth, M. Brundage, and A. A. Bharath, “A brief survey of deep reinforcement learning,” IEEE Signal Processing Magazine, Vol.34, No.6, pp. 26-38, arXiv preprint arXiv:1708.05866, 2017.
- [21] T. M. Moerland, J. Broekens, and C. M. Jonker, “Emotion in reinforcement learning agents and robots: A Survey,” Machine Learning, Vol.107, Issue 2, pp 443-480, 2018.
- [22] Y. Semet, “Interactive Evolutionary Computation: A Survey of Existing Theory,” University of Illinois, 2002.
- [23] H. Takagi and H. Iba, “Interactive evolutionary computation,” New Generation Computing, Vol.23, Issue 2, pp. 113-114, 2005.
- [24] K. Neubert and E. Brunner, “A studentized permutation test for the non-parametric Behrens-Fisher problem,” Computational Statistics & Data Analysis, Vol.51, Issue 10, pp. 5192-5204, 2007.
This article is published under a Creative Commons Attribution-NoDerivatives 4.0 Internationa License.