Paper:
Expression and Identification of Confidence Based on Individual Verbal and Non-Verbal Features in Human-Robot Interaction
Youdi Li, Wei Fen Hsieh, Eri Sato-Shimokawara, and Toru Yamaguchi
Tokyo Metropolitan University
6-6 Asahigaoka, Hino, Tokyo 191-0065, Japan
In our daily life, it is inevitable to confront the condition which we feel confident or unconfident. Under these conditions, we might have different expressions and responses. Not to mention under the situation when a human communicates with a robot. It is necessary for robots to behave in various styles to show adaptive confidence degree, for example, in previous work, when the robot made mistakes during the interaction, different certainty expression styles have shown influence on humans’ truthfulness and acceptance. On the other hand, when human feel uncertain on the robot’s utterance, the approach of how the robot recognizes human’s uncertainty is crucial. However, relative researches are still scarce and ignore individual characteristics. In current study, we designed an experiment to obtain human verbal and non-verbal features under certain and uncertain condition. From the certain/uncertain answer experiment, we extracted the head movement and voice factors as features to investigate if we can classify these features correctly. From the result, we have found that different people had distinct features to show different certainty degree but some participants might have a similar pattern considering their relatively close psychological feature value. We aim to explore different individuals’ certainty expression patterns because it can not only facilitate humans’ confidence status detection but also is expected to be utilized on robot side to give the proper response adaptively and thus spice up the Human-Robot Interaction.
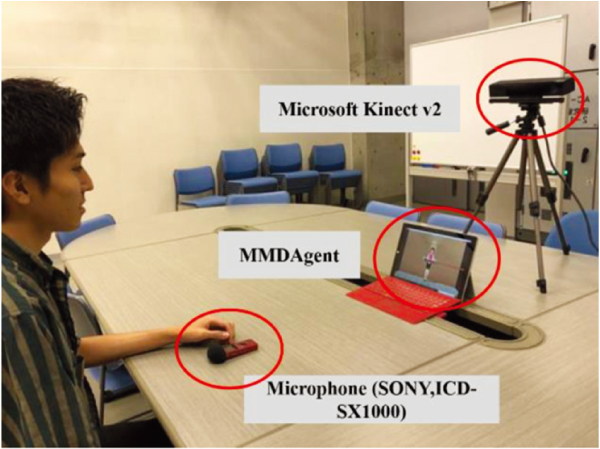
A participant is answering questions
- [1] P. Liu, D. F. Glas, T. Kanda, and H. Ishiguro, “Data-Driven HRI: Learning Social Behaviors by Example from Human–Human Interaction,” IEEE Trans. on Robotics, Vol.32, Issue 4, pp. 988-1008, 2016.
- [2] I. Leite, M. McCoy, D. Ullman, N. Salomons, and B. Scassellati, “Comparing Models of Disengagement in Individual and Group Interactions,” Proc. of the 10th Annual ACM/IEEE Int. Conf. on Human-Robot Interaction (HRI’15), pp. 99-105, 2015.
- [3] D. Matsui, T. Minato, K. F. MacDorman, and H. Ishiguro, “Generating Natural Motion in an Android by Mapping Human Motion,” H. Ishiguro and F. Dalla Libera (Eds.), “Geminoid Studies: Science and Technologies for Humanlike Teleoperated Androids,” pp. 57-73, Springer, 2018.
- [4] T. Minato, M. Shimada, H. Ishiguro, and S. Itakura, “Development of an Android Robot for Studying Human-Robot Interaction,” Proc. of the 17th Int. Conf. on Industrial and Engineering Applications of Artificial Intelligence and Expert Systems (IEA/AIE 2004), pp. 424-434, 2004.
- [5] M. Pantic, R. Cowie, F. D’Errico, D. Heylen, M. Mehu, C. Pelachaud, I. Poggi, M. Schroeder, and A. Vinciarelli, “Social Signal Processing: The Research Agenda,” T. B. Moeslund, A. Hilton, V. Krüger, L. Sigal (Eds.), “Visual Analysis of Humans: Looking at People,” pp. 511-538, Springer, 2011.
- [6] S. Ivaldi, S. Lefort, J. Peters, M. Chetouani, J. Provasi, and E. Zibetti, “Towards Engagement Models that Consider Individual Factors in HRI: On the Relation of Extroversion and Negative Attitude Towards Robots to Gaze and Speech During a Human–Robot Assembly Task,” Int. J. of Social Robotics, Vol.9, Issue 1, pp. 63-86, 2017.
- [7] S. M. Anzalone, Y. Yoshikawa, H. Ishiguro, E. Menegatti, E. Pagello, and R. Sorbello, “Towards Partners Profiling in Human Robot Interaction Contexts,” Proc. of the 3rd Int. Conf. on Simulation, Modeling, and Programming for Autonomous Robots (SIMPAR 2012), pp. 4-15, 2012.
- [8] M. Leo and G. M. Farinella (Eds.), “Computer Vision for Assistive Healthcare,” Academic Press, 2018.
- [9] A. Vinciarelli, M. Pantic, and H. Bourlard, “Social signal processing: Survey of an emerging domain,” Image and Vision Computing, Vol.27, Issue 12, pp. 1743-1759, 2009.
- [10] S. Ali Etemad and A. Arya, “Classification and translation of style and affect in human motion using RBF neural networks,” Neurocomputing, Vol.129, pp. 585-595, 2014.
- [11] A. Thomaz, G. Hoffman, and M. Cakmak, “Computational Human-Robot Interaction,” Foundations and Trends in Robotics, Vol.4, No.2-3, pp. 105-223, 2016.
- [12] B. Lepri, R. Subramanian, K. Kalimeri, J. Staiano, F. Pianesi, and N. Sebe, “Employing social gaze and speaking activity for automatic determination of the Extraversion trait,” Proc. of the Int. Conf. on Multimodal Interfaces and the Workshop on Machine Learning for Multimodal Interaction (ICMI-MLMI’10), Article No.7, 2010.
- [13] H. Ritschel and E. André, “Real-Time Robot Personality Adaptation based on Reinforcement Learning and Social Signals,” Proc. of the Companion of the 2017 ACM/IEEE Int. Conf. on Human-Robot Interaction, pp. 265-266, 2017.
- [14] K. M. Lee, W. Peng, S. Jin, and C. Yan, “Can Robots Manifest Personality?: An Empirical Test of Personality Recognition, Social Responses, and Social Presence in Human–Robot Interaction,” J. of Communication, Vol.56, Issue 4, pp. 754-772, 2006.
- [15] A. Aly and A. Tapus, “A model for synthesizing a combined verbal and nonverbal behavior based on personality traits in human-robot interaction,” Proc. of the 8th ACM/IEEE Int. Conf. on Human-Robot Interaction, pp.325-332, 2013.
- [16] S. Woods, K. Dautenhahn, C. Kaouri, R. Boekhorst, and K. L. Koay, “Is this robot like me? Links between human and robot personality traits,” Proc. of the 5th IEEE-RAS Int. Conf. on Humanoid Robots, pp. 375-380, 2005.
- [17] Y. Li, W. F. Hsieh, A. Matsufuji, E. Sato-Shimokawara, and T. Yamaguchi, “Be Certain or Uncertain for the Erroneous Situation in Human-Robot Interaction: A Dialogue Experiment Focused on the Verbal Factors,” The Joint Int. Conf. of ISCIIA2018 and ITCA2018 (ISCIIA&ITCA2018), 2018.
- [18] N. Mavridis, “A review of verbal and non-verbal human–robot interactive communication,” Robotics and Autonomous Systems, Vol.63, Part 1, pp. 22-35, 2015.
- [19] A. Aly and A. Tapus, “Towards an intelligent system for generating an adapted verbal and nonverbal combined behavior in human–robot interaction,” Autonomous Robots, Vol.40, Issue 2, pp. 193-209, 2016.
- [20] M. L. Knapp, J. A. Hall, and T. G. Horgan, “Nonverbal Communication in Human Interaction,” 8th edition, Wadsworth Cengage Learning, 2013.
- [21] V. Chu, K. Bullard, and A. L. Thomaz, “Multimodal real-time contingency detection for HRI,” Proc. of the 2014 IEEE/RSJ Int. Conf. on Intelligent Robots and Systems, pp. 3327-3332, 2014.
- [22] P. Cofta, “Confidence, trust and identity,” BT Technology J., Vol.25, Issue 2, pp. 173-178, 2007.
- [23] L. Takayama, D. Dooley, and W. Ju, “Expressing thought: Improving robot readability with animation principles,” Proc. of the 6th ACM/IEEE Int. Conf. on Human-Robot Interaction (HRI’11), pp. 69-76, 2011.
- [24] A. D. Dragan, S. Bauman, J. Forlizzi, and S. S. Srinivasa, “Effects of Robot Motion on Human-Robot Collaboration,” Proc. of the 10th Annual ACM/IEEE Int. Conf. on Human-Robot Interaction (HRI’15), pp. 51-58, 2015.
- [25] P. E. Vernon, “Personality Assessment: A critical survey,” Routledge, 2014.
- [26] R. A. LeVine, “Culture, Behavior, and Personality: An Introduction to the Comparative Study of Psychosocial Adaptation,” 2nd edition, Routledge, 2018.
- [27] D. Cervone and L. A. Pervin, “Personality: Theory and Research,” 13th edition (Binder Ready Version), John Wiley & Sons, 2015.
- [28] I. Poggi and F. D’Errico, “Social Signals: A Psychological Perspective,” A. Ali Salah and T. Gevers (Eds.), “Computer Analysis of Human Behavior,” pp. 185-225, Springer, 2011.
- [29] O. P. John, L. P. Naumann, and C. J. Soto, “Paradigm Shift to the Integrative Big Five Trait Taxonomy: History, Measurement, and Conceptual Issues,” O. P. John, R. W. Robins, and L. A. Previn (Eds.), “Handbook of Personality: Theory and Research,” 3rd edition, pp. 114-158, The Guilford Press, 2008.
- [30] A. Gaschler, K. Huth, M. Giuliani, I. Kessler, J. De Ruiter, and A. Knoll, “Modelling State of Interaction from Head Poses for Social Human-Robot Interaction,” Proc. of the “Gaze in Human-Robot Interaction” Workshop held at the 7th ACM/IEEE Int. Conf. on Human-Robot Interaction (HRI2012), 2012.
- [31] M. Salem, F. Eyssel, K. Rohlfing, S. Kopp, and F. Joublin, “To Err is Human(-like): Effects of Robot Gesture on Perceived Anthropomorphism and Likability,” Int. J. of Social Robotic, Vol.5, Issue 3, pp. 313-323, 2013.
- [32] M. Giuliani, N. Mirnig, G. Stollnberger, S. Stadler, R. Buchner, and M. Tscheligi, “Systematic analysis of video data from different human–robot interaction studies: a categorization of social signals during error situations,” Frontiers in Psychology, Vol.6, Article No.931, 2015.
- [33] R. Stiefelhagen, C. Fugen, R. Gieselmann, H. Holzapfel, K. Nickel, and A. Waibel, “Natural human-robot interaction using speech, head pose and gestures,” Proc. of the 2004 IEEE/RSJ Int. Conf. on Intelligent Robots and Systems (IROS 2004), Vol.3, pp. 2422-2427, 2004.
- [34] L.-P. Morency, C. Sidner, C. Lee, and T. Darrell, “Head gestures for perceptual interfaces: The role of context in improving recognition,” Artificial Intelligence, Vol.171, Issue 8-9, pp. 568-585, 2007.
- [35] A. Frischen, A. P. Bayliss, and S. P. Tipper, “Gaze cueing of attention: Visual attention, social cognition, and individual differences,” Psychological Bulletin, Vol.133, Issue 4, pp. 694-724, 2007.
- [36] S. O. Ba and J.-M. Odobez, “Recognizing Visual Focus of Attention from Head Pose in Natural Meetings,” IEEE Trans. on Systems, Man, and Cybernetics, Part B (Cybernetics), Vol.39, Issue 1, pp. 16-33, 2009.
- [37] MMDAgent, http://www.mmdagent.jp/ [accessed August 16, 2019]
- [38] Praat, http://www.fon.hum.uva.nl/praat/ [accessed August 16, 2019]
- [39] E. Kasano, S. Muramatsu, A. Matsufuji, E. Sato-Shimokawara, and T. Yamaguchi, “Estimation of Speaker’s Confidence in Conversation Using Speech Information and Head Motion,” Proc. of the 2019 16th Int. Conf. on Ubiquitous Robots (UR), pp. 294-298, 2019.
- [40] R. Arora and Suman, “Comparative Analysis of Classification Algorithms on Different Datasets using WEKA,” Int. J. of Computer Applications, Vol.54, No.13, pp. 21-25, 2012.
This article is published under a Creative Commons Attribution-NoDerivatives 4.0 Internationa License.