Abstract
Large studies use genotype data to discover genetic contributions to complex traits and infer relationships between those traits. Co-incident geographical variation in genotypes and health traits can bias these analyses. Here we show that single genetic variants and genetic scores composed of multiple variants are associated with birth location within UK Biobank and that geographic structure in genotype data cannot be accounted for using routine adjustment for study centre and principal components derived from genotype data. We find that major health outcomes appear geographically structured and that coincident structure in health outcomes and genotype data can yield biased associations. Understanding and accounting for this phenomenon will be important when making inference from genotype data in large studies.
Similar content being viewed by others
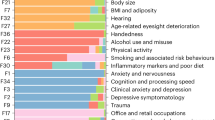
Introduction
Genotype data are used to answer an increasing number of research questions through an increasing number of methods. Latent structure within genetic data was originally identified as a problem in candidate gene association studies, where population stratification was “probably the most often cited reason for non-replication of genetic association results”1. With the advent of data at genome-wide scale, it became possible to parameterise the impact of latent structure on genetic association results using principal components (PCs)2 derived from genotype data among other approaches3. These methods, in conjunction with stringent genome-wide significance thresholds4 and the requirement for replication in an independent population, led to substantial improvement in replicability of genetic association studies.
In other contexts, detecting and accounting for latent structure remains problematic. Determining heritability of complex traits is challenging5,6 because population structure and polygenic traits both impart genome-wide signatures which may be truly (rather than spuriously) related. For example, genetic height7 varies amongst historical populations from which modern populations represent different mixtures. Stratification may be part of the explanation for different signatures of selection for height in UK Biobank compared to the GIANT consortium8,9. Analysis of rare genetic variation is another challenge, as the latent structure within this subset of genetic data may not reflect the latent structure of common variants used to generate PCs10.
An entirely different context for using genetic data is in epidemiological analyses which have developed substantially with the availability of reliable genetic association results from published sources and very large collections of genetic and phenotypic data such as UK Biobank11. A good example of this is Mendelian randomisation, which aims to escape confounding in associations by using genetic variation to proxy risk factors of interest12. Recent literature has focussed on maximising the use of the current wave of genetic association evidence and accounting for undesirable pleiotropic effects of single variants13. This activity has largely assumed that structure is addressed during the discovery of associated genetic variants, an assumption which now warrants closer examination. If present, latent structure within datasets used to perform epidemiological analyses would violate the requirement that genetic instrumental variables are not related to potentially confounding features14 and may result in biased epidemiological inference.
Here, we use geographical information in conjunction with genetic data to investigate latent structure (of unknown cause) in two population-based cohorts in the United Kingdom. We show that single genetic variants and polygenic scores incorporating multiple variants are associated with birth location in data from UK Biobank. Given regional differences in many health outcomes, this observed structure provides a source of covariance between genotypes and health outcomes which can bias epidemiological inference from genetic data. Understanding and accounting for this phenomenon will be important when making inference from genotype data in large studies.
Results
Alignment of educational attainment with ancestry
We examined whether there is previously under-appreciated structure in a well-understood ethnically and geographically homogenous resource using the the Avon Longitudinal Study of Parents and Children (ALSPAC)15,16 as an exemplar. We studied 7739 mothers who were recruited during pregnancy in the Bristol area (South West UK) in the early 1990s. We undertook chromosome painting17 to describe fine-scale relatedness between each mother and each of the regions of the Peopling of the British Isles (PoBI) project18, acting as an external source of geographical information. We summarised each mother’s ancestral lineage as a mixture of the PoBI regions, allowing us to estimate the educational attainment that those regions would have were the ALSPAC mothers’ education levels explained by this variation. In doing this a pattern for lower educational attainment in lineages originating from the regions immediately surrounding Bristol (Fig. 1) and higher educational attainment in more geographically distant lineages was observed. The patterns of educational attainment within the United Kingdom predicted by the ALSPAC sample are strikingly different from patterns of educational attainment observed in national surveys19. Distant lineages are likely only represented in ALSPAC by individuals or families who had migrated, and we anticipate that the educational attainment of people who migrate for economic reasons differs from people who do not. Educational attainment is therefore aligned to subtle genetic differences even in this apparently geographically and ethnically homogenous population and this is co-incident with axes of ancestry.
Within-UK ancestry predicts migration that confounds education: estimated educational attainment of the United Kingdom, when seen only through the ALSPAC cohort based in Bristol. Scores are 1: vocational, 2: CSEs, 3: O-levels, 4: A-levels, 5: degree. CSE Certificate of Secondary Education. The predicted mean education for each region is given, along with 95% confidence intervals estimated by bootstrap resampling of individuals. Each region is coloured by predicted mean education, where predicted mean = 2 is shaded in red and predicted mean = 5 is shaded in white. See Methods for details. ALSPAC Avon Longitudinal Study of Parents and Children
Alignment of common genetic variants with geography
The structure in ALSPAC was detected here using a chromosome painting method, which is highly sensitive to ancestry. With greater power, it is entirely possible the same phenomena may become detectable in more routine analytical procedures for gene discovery or epidemiological analysis. We therefore turned to UK Biobank, an exceptional resource containing a catalogue of health, disease and genotype data of almost half a million participants11,20. Conceptually, UK Biobank is analogous to a super-imposition of multiple ALSPACs, each of which recruited participants living near a study assessment centre. This design not only gives UK Biobank the capacity to represent a broad spectrum of UK ancestry and structure, but also means that the study is sensitive to important sampling phenomena including self-selection. The hurdles of location and attendance (less than 6% of individuals contacted by UK Biobank chose to participate21) are likely to influence the nature of the resultant participant collection and are related to behaviours with heritable contributions22. This may create collider biases23,24 which have the ability to induce association between otherwise independent variables.
We examined whether there is geographic structure in the genetic data of UK Biobank using within-study geographical information by performing genome-wide association studies (GWASs) for birth location in PLINK25. The outcomes were North/South and East/West axes of birth location, both measured on a metre grid scale from an origin South West of the United Kingdom. Analysis of genetic data was performed within individuals of white British ancestry with non-missing data on birth location (n = 321,439). GWAS for birth location identified that single variants are associated with geography within UK Biobank. An unadjusted model produced distorted and inflated plots with evidence for association at variants across the autosome. After adjustment for genotyping array, 40 PCs and a factor variable representing UK Biobank assessment centre single variants remained associated with birth location (Supplementary Fig. 1).
Alignment of polygenic scores with geography
Rather than using single genetic variants, empirical epidemiological analyses often use polygenic scores (PS)26,27. As exemplars, we took genetic variants and weightings associated with educational attainment, height and body mass index (BMI) from published genome-wide meta-analyses28,29,30 excluding UK Biobank. Using an approach that is widespread in applied analyses, we used these externally derived variants and weightings in conjunction with the UK Biobank genetic data to create polygenic scores for the three traits. Aiming to understand the properties of these polygenic scores under a range of analytical contexts, we created both weighted and unweighted PS at a strict and more liberal threshold of association in the discovery sample (p < 5e−08 and p < 1e−05 respectively). We used general additive models31 in the ”mgcv” package (version 1.8)32 within R (version 3.3.1)33 to test for non-linear relationships between PS and geographical terms. All PS tested were associated with birth location in an unadjusted model and a model that adjusted only for genotyping array. These associations attenuated but were not extinguished in models incorporating adjustment for 40 PCs and study centre, especially for educational attainment and birth location on the North/South axis, where statistical adjustment had little impact on the fitted geographical distribution of the PS (Figs. 2 and 3 and Table 1). There is some irregularity in the pattern of geographical association when comparing the characteristics of weighted versus unweighted PS for the same trait or when comparing strictly defined versus liberally defined PS for the same trait, suggesting that the characteristics of these PS are sensitive to changes in composition. Sensitivity analyses using a PS for BMI trained in published data from Biobank Japan34 yielded similar findings to the PS for BMI trained in GIANT (Supplementary Table 1).
The relationship between polygenic scores (PS; right-hand label) and geographical terms (left-hand label) within the UK Biobank sample. Tiles are shaded by p value testing the null hypothesis of no association between PS and geographical term, where p = 0 is shaded in black and p = 2e−16 is shaded in red. Statistical adjustment was performed as follows: model 1: no adjustment; model 2: adjustment for genotyping array only; model 3: adjustment for genotyping array, 10 principal components (PCs) and study participation centre; model 4: adjustment for genotyping array, 40 PCs and study participation centre
Fitted spline regression plots showing the non-linear distribution of polygenic scores (PS) for educational attainment (weighted version, including variants with p < 1.0e−05) in unadjusted model (left) and model after adjustment for 40 principal components and study centre (right). The centre of major population centres is marked for reference. The shaded area represents 95% confidence intervals
Alignment of complex traits with geography
Having found evidence for association between genotypic variation and geography, we used general additive models to test for non-linear relationships between four exemplar complex traits and geography. Reported household income, measured BMI, reported age at completion of full-time education and reported number of siblings showed strong evidence for geographical stratification (p < 2e−16 for non-linear relationship between observed traits and axes of birth location).
Co-incident latent structure produces biased estimates
Given these observations, we hypothesised that latent structure might act as a source of covariance between genotypes and health outcomes, leading us to explore the potential role of latent structure in confounding analysis. We tested for linear association between the PS and complex traits and examined whether the inclusion of non-linear terms for birth location as covariates altered the results, again using general additive models. The relationship between the BMI PS and BMI changed little with increasing statistical adjustment, but other relationships changed in magnitude with the addition of non-linear terms for birth location. For example, the association between the BMI PS and household income attenuated by over 30% in a fully adjusted model compared to an unadjusted model, suggesting that the unadjusted estimate was confounded by co-incident latent structure in this sample. Similar patterns of attenuation were seen for both weighted and unweighted PS, and for strictly defined (Table 2, Fig. 4) and liberally defined PS (Supplementary Table 2 and Supplementary Fig. 2). Birth location captures neither the full extent of variation in fine ancestral structure (which predicts PS) nor the full extent of geographically structured social and economic differences (which predict income). It is possible that these adjusted estimates therefore contain residual confounding and that the true impact of biases within this sample is larger than these results suggest. Similarly, lack of association between a PS and birth location may be insufficient to assert that the PS is free from stratifying bias.
Attenuation in linear relationship between polygenic scores (PS) and complex traits in the UK Biobank sample at varying degrees of statistical adjustment. N sibs refers to number of siblings. For each PS, the relationship with four traits was estimated using an unadjusted model (plotted in circle) and this estimate and its corresponding 95% confidence intervals were rescaled to a value of 1. Error bars represent 95% confidence intervals for the rescaled estimate. Adjustment was then performed for genotyping array only (triangles), genotyping array, 40 principal components (PCs) and study participation centre (cross) and 40 PCs, study participation centre and non-linear regression terms for North and East axes of birth location (square). A value of 0.5 on the y-axis would mean that 50% of the unadjusted effect estimate remained after adjustment. Lines are drawn at x = 1 (red) and y = 0 (black) for reference
As an alternative way to demonstrate the potential impact of such bias, we generated random complex traits matched to real complex traits, assigning each participant a plausible value based on typical values for the corresponding real traits in their region of the UK Biobank sample. This procedure aimed to produce traits recapitulating the coarse geographical distribution of the real complex traits, while greatly reducing the magnitude of biological genotype–phenotype pathways, as the matched traits were uncorrelated with either the real genotypes or real traits within any given region (Methods). In this analysis, each PS was associated with at least one complex trait and these associations attenuated with adjustment for latent structure, collectively suggesting PS predict geographical location within the UK Biobank sample (Supplementary Table 3).
Discussion
The presence of structure within the genetic data of UK Biobank has several potential explanations, including a legacy of ancient ancestral groups that are not fully admixed18,35, a consequence of non-random mating or polygenic selection36,37,38, a study artefact induced by selection bias24 or a combination of all these explanations. Regardless of origin, this phenomenon is important, both as a source of ecological-level covariance between genotypes and geographically heterogeneous complex traits, and because of its apparent persistence across different analytical contexts and modes of statistical adjustment. Recent evidence from an investigation in the United States39 also illustrates associations between PS and complex traits at the ecological level. Now manifest, this property should be added to the growing list of limitations to naive use of PS—including horizontal pleiotropy12, high false discovery rate40, association with coarse ancestral groups41 and prediction of inter-generational phenotypes which complicates interpretation42.
The ability of very large studies to detect effects indistinguishable from artefactual biases or ancestral differences demands reworked approaches to exploit43, or at least account for, structure. Exciting recent developments aim to improve statistical models44 or leverage information from family-based study designs for unbiased inference45. Until such methods have developed further, we hope this short article draws attention to an important phenomenon and illustrates the ongoing relevance of basic epidemiolocal principles in an era of increasingly sophisticated analyses.
Methods
ALSPAC
The ALSPAC is a birth cohort which recruited 14,541 pregnant women living in the former county of Avon (surrounding Bristol, UK) with expected delivery dates between 1 April 1991 and 31 December 1992. Since then, participating mothers and their children have been followed up with serial clinical data, questionnaire data and biosample collection. A nested cohort following children of the index offspring has been formed and data on fathers of the index offspring are also available. Further information on the index offspring and mothers cohorts are available15,16. The study website contains details of all data that are available through a fully searchable data dictionary at http://www.bris.ac.uk/alspac/researchers/data-access/data-dictionary/.
Ethical approval for the study was obtained from the ALSPAC Ethics and Law Committee and the Local Research Ethics Committees, and this research complied with all relevant ethical regulations. Participants gave informed consent. This publication is the work of the authors and N.T. and S.H. who will serve as guarantors for the contents of this paper.
Genotypes
Genotype data for ALSPAC mothers were generated using the Illumina human660w genotyping array and genotypes were called with Illumina GenomeStudio, yielding 557,124 directly genotyped single-nucleotide polymorphisms (SNPs) in 10,015 participants. Participants and SNPs were carried through to imputation if they passed quality control measures implemented in PLINK (v1.07). SNP-level quality control removed variants with more than 5% missingness, or p value for Hardy–Weinberg equilibrium smaller than 1e−6. Participant-level quality control removed variants with uncertain X chromosome heterozygosity, extreme autosomal heterozygosity or more than 5% overall missingness. Next, multi-dimensional scaling of genome-wide data was performed including reference data from HapMap populations. Samples which clustered outside the CEU population were removed. Following these measures, data for 9048 participants and 526,688 SNPs were available. Related participants were identified by estimating inheritance by descent, where an estimate of greater than 0.125 was considered to represent cryptic relatedness. Data from these participants were included in phasing and imputation, but prior to analysis related individuals were removed from the dataset until no cryptically related pairs were present, yielding a final sample size of 8196 mothers.
Chromosome painting
To infer within-UK ancestral origin of these mothers, we used Chromosome Painting17 coupled with high-resolution spatial data from the PoBI18. We first merged the ALSPAC mothers and PoBI data into a set of shared SNPs (using the ALSPAC imputed data). We then performed phasing using Shapeit46 and then Impute247 to impute any remaining missing SNPs. Finally, we used ChromoPainter17 to paint each individual against all individuals from the 35 labelled populations given in PoBI to obtain a genome-wide estimate of haplotype sharing.
We then constructed a reference panel for the PoBI data by averaging the painting for all individuals in each of the labelled populations. We then used the Non-Negative Least Squares method for estimating ancestry48 for each of the ALSPAC participants, in terms of the 35 labels. In total, 7739 ALSPAC mothers and 2,039 PoBI participants were included in analyses.
We did not observe many individuals with ancestry from only a single PoBI region. Therefore, we have to infer the underlying population averages that, given the mixture that we observed, would have given rise to the data. We use the method of Lawson et al.49 for this. Let AB=C, where A is an N by K matrix of admixture estimates, B is the population phenotypes to be inferred, and C is the observed individual phenotype estimates, i.e., measured education. Then, we can solve B=(ATA)−1ATC.
We note that this procedure is solely used to generate a visualisation of the relationship between genetic information and migration status and is not intended to reflect inference regarding individual-level ancestry. At the individual-level, using genetically non-distinct populations (i.e. based on labels rather than genetic distinctness as was done in Leslie et al.18) could result in the inference being unidentifiable. For this question regarding average phenotype, this lack of identifiability does not matter since populations are represented in the correct proportions, on average. This is evidenced by the clear structure visible in Fig. 1.
UK Biobank methods
The UK Biobank study assessment centre sites targeted densely populated areas of England, Scotland and Wales, where a large eligible population could attend in-person assessment with a journey of less than 10 miles50. Participants gave informed consent, and the UK Biobank was approved by the North West Multi-centre Research Ethics Committee. This research was conducted using the UK Biobank Resource applications 8786 and 15825, and complied with all relevant ethical regulations.
Geographical data
The assessment centre at which a participant consented was assigned a numerical code (field 54 in the UK Biobank data). In analyses adjusted for assessment centre, these codes were treated as factor variables.
Participants who were born in the United Kingdom were asked to name their place of birth during a verbal interview at study assessment centres. These answers were used to derive approximate North and East co-ordinates (rounded values, recorded on a metre grid scale from an origin South-West of the UK, fields 129 and 130 in the UK Biobank data). Values less than zero were coded as missing for both variables.
Complex traits
Household income was obtained from baseline data in UK Biobank. Participants were asked to report the annual income for their household in pounds sterling using one of the following options (1: less than 18,000; 2: 18,000 to 30,999; 3: 31,000 to 51,999; 4: 52,000 to 100,000; 5: greater than 100,000; −1: do not know; −3: prefer not to answer) (field 738). To allow inclusion in a linear regression model these categories were recoded to values in pounds sterling in the midpoint of each category. For categories with only an upper or lower bound, the difference between the midpoint and boundary of the next adjacent category was used to estimate a midpoint as follows (1: 11,505; 2: 22,495; 3: 41,499; 4: 76,000; 5: 124,000). Household income was coded as missing for participants who preferred not to answer or did not know their household income.
Number of siblings was obtained from baseline data. Participants were asked to report how many full brothers they had (including those who have died and twin brothers, but excluding half-brothers, step-brothers and adopted brothers) (field 1873). A matching question was asked for sisters (field 1883). The responses for these two questions were combined to create a number of full siblings. This variable was coded as missing for participants who preferred not to answer or did not know for either source question.
BMI was derived by UK Biobank from height and weight measures during the initial assessment centre visit (field 21001) as weight divided by height squared (units kg/m2). This variable was coded as missing if either height or weight measures were missing.
Age at completion of full-time education was taken from baseline data (field 845). Participants were asked to report their age (in years) when they completed full-time education. This variable was set to missing for participants who responded that they never went to school, did not know or preferred not to answer. This question was not asked for participants who had previously indicated they had a college or university degree and this is reflected in the smaller sample size compared to other complex traits.
Randomly assigned traits
Participants were ranked by North/South axis of birth location within the United Kingdom, and divided into 100 bins, each with an equal number of participants. Within each bin, the mean and standard deviation of each complex trait (income, number of siblings, BMI and age at completion of full-time education) was summarised, then new values for that trait were drawn from a random distribution with the same mean and standard deviation. The procedure was repeated for East/West axis of birth location, yielding two new values for each simulated trait. These values were combined with equal weighting, producing a total of four simulated traits which aimed to preserve coarse geographical variation across the sampling frame of UK Biobank whilst greatly reducing or eliminating direct biological effects.
Genetic data
We used the UK Biobank 500k (July 2017) genotype release, for which pre-imputation quality control, phasing and imputation are described elsewhere20. Following imputation we removed variants that were not present within the haplotype reference consortium (HRC) imputation panel and applied a graded filtering on imputation quality. Rarer variants were required to have a higher imputation INFO score (Info > 0.3 for minor allele frequency (MAF) > 3%; Info > 0.6 for MAF 1–3%; Info > 0.8 for MAF 0.5–1% and Info > 0.9 for MAF 0.1–0.5%). We removed 378 individuals with a mismatch between genetic sex and reported sex and 352 individuals with putative sex chromosome aneuploidy. We performed analysis within individuals who self-reported as “British” and had similar ancestral background from genetic PCs (n = 409,703). We applied an exclusion list containing 79,448 individuals, whilst preferentially removing individuals related to the greatest number of other individuals so that no related pairs remained in the final sample used for analysis. A comprehensive description of quality control methods has been published online51.
Genetic principal components
We used genetic PCs supplied by UK Biobank (field 22009). These were calculated using a set of 407,219 unrelated, high-quality samples and 147,604 high confidence markers after pruning for linkage disequilibrium. Participants with missing PCs were excluded from analysis.
Genome-wide association studies
GWAS for birth location were performed using PLINK (v2.0, August 2017 release)25. A full description of the analytical pipeline has been published online52. All models included adjustment for genotyping array and sex. Assessment centre was treated as a factor variable (where included) and PCs were treated as linear covariables.
Polygenic scores
We took variants and weights associated with educational attainment from the discovery phase of a recent genome-wide meta-analysis (excluding the replication phase in UK Biobank)28. Variants and weights for height and BMI were taken from the entire meta-analysis results from the GIANT consortium29,30. For each of these three traits, we obtained clumped instruments from the MRBase repository using the “extract_instruments” option in the TwoSampleMR R package53. Effect allele dosage was extracted for these variants from the filtered UK Biobank genotype data. Effect allele dosage was weighted by reported genetic effect (beta) and then summarised across all contributing variants to create per-individual PS. Unweighted PS were created in parallel, which included the same variants but only considered direction of effect, not reported effect size. PS were z-transformed after application of all exclusion criteria. For sensitivity analysis, variants and weights for BMI were taken from published results in Biobank Japan34, clumped using reference data from East Asian ancestry participants in 1000 genomes and then used to derive PS following the same workflow as the main analysis.
Generalised additive models
The relationship between complex traits and geographical parameters was modelled using the ‘mgcv’ package (version 1.8)32 in R (version 3.3.1)33. Traits were modelled against a spline function for either birth northings or birth eastings, in the form t ~ s(location). Approximate statistical significance for non-linear terms was taken from the model summary, which estimates a suitable number of degrees of freedom from cross validation.
The relationship between PS and geographical parameters was modelled in a similar way, but incorporated a variable for genotyping array as the minimum adjustment, in the form ps ~ s(location) + array. Fully adjusted models included factor variables for study centre and up to 40 genetic PCs.
The relationship between complex traits and PS was modelled as a linear relationship to obtain indicative effect sizes, and took the form t ~ ps. Where relevant, non-linear covariables were included as spline terms in the form t ~ ps + s(birth_location) + other covariables. Simulated complex traits were modelled in exactly the same way as observed complex traits.
Reporting summary
Further information on experimental design is available in the Nature Research Reporting Summary linked to this article.
Data availability
ALSPAC data are available through an access procedure described at http://www.bristol.ac.uk/alspac/researchers/access/. UK Biobank data are available through a procedure described at http://www.ukbiobank.ac.uk/using-the-resource/. POBI genotype data and location information are available via the European Genotype Archive (https://www.ebi.ac.uk/ega/) under accession numbers EGAS00001000672 and EGAD00010000632. Summary results of genome-wide association analysis are available at the University of Bristol data repository, data.bris, at https://doi.org/10.5523/bris.15pdhgrio8d6u2f2brgaahah9, and can also be queried using the LD-Hub (http://ldsc.broadinstitute.org/ldhub/) resource.
References
Cardon, L. R. & Palmer, L. J. Population stratification and spurious allelic association. Lancet 361, 598–604 (2003).
Price, A. L. et al. Principal components analysis corrects for stratification in genome-wide association studies. Nat. Genet. 38, 904 (2006).
Bouaziz, M., Ambroise, C. & Guedj, M. Accounting for population stratification in practice: a comparison of the main strategies dedicated to genome-wide association studies. PLoS One 6, e28845 (2011).
Pe'er, I., Yelensky, R., Altshule, D. & Daly, M. J. Estimation of the multiple testing burden for genomewide association studies of nearly all common variants. Genet. Epidemiol. 32, 381–385 (2008).
Browning, S. R. & Browning, B. L. Population structure can inflate SNP-based heritability estimates. Am. J. Hum. Genet. 89, 191–193 (2011).
Dandine-Roulland, C. et al. Accuracy of heritability estimations in presence of hidden population stratification. Sci. Rep. 6, 26471 (2016).
Mathieson, I. et al. Genome-wide patterns of selection in 230 ancient Eurasians. Nature 528, 499 (2015).
Sohail, M. et al. Signals of polygenic adaptation on height have been overestimated due to uncorrected population structure in genome-wide association studies. Preprint at bioRxiv https://doi.org/10.1101/355057 (2018).
Berg, J. J. et al. Reduced signal for polygenic adaptation of height in UK Biobank. Preprint at bioRxiv https://doi.org/10.1101/354951 (2018).
Mathieson, I. & McVean, G. Differential confounding of rare and common variants in spatially structured populations. Nat. Genet. 44, 243–246 (2012).
Collins, R. What makes UK Biobank special? Lancet 379, 1173–1174 (2012).
Smith, G. D. & Hemani, G. Mendelian randomization: genetic anchors for causal inference in epidemiological studies. Hum. Mol. Genet. 23, R89–R98 (2014).
Paaby, A. B. & Rockman, M. V. The many faces of pleiotropy. Trends Genet. 29, 66–73 (2013).
Lawlor, D. A., Harbord, R. M., Sterne, J. A. C., Timpson, N. & Smith, G. D. Mendelian randomization: using genes as instruments for making causal inferences in epidemiology. Stat. Med. 27, 1133–1163 (2008).
Boyd, A. et al. Cohort Profile: The ‘Children of the 90s’-the index offspring of the Avon Longitudinal Study of Parents and Children. Int. J. Epidemiol. 42, 111–127 (2013).
Fraser, A. et al. Cohort Profile: The Avon Longitudinal Study of Parents and Children: ALSPAC mothers cohort. Int. J. Epidemiol. 42, 97–110 (2013).
Lawson, D. J., Hellenthal, G., Myers, S. & Falush, D. Inference of population structure using dense haplotype data. PLoS Genet. 8, e1002453 (2012).
Leslie, S. et al. The fine-scale genetic structure of the British population. Nature 519, 309 (2015). +.
Office for National Statistics. 2011 Census: Key Statistics and Quick Statistics for Local Authorities in the United Kingdom. https://www.ons.gov.uk/peoplepopulationandcommunity/populationandmigration/populationestimates/datasets/2011censuskeystatisticsandquickstatisticsforlocalauthoritiesintheunitedkingdompart1 (2013).
Bycroft, C. et al. Genome-wide genetic data on ~500,000 UK Biobank participants. Preprint at bioRxiv https://doi.org/10.1101/166298 (2017).
Fry, A. et al. Comparison of sociodemographic and health-related characteristics of UK Biobank participants with those of the general population. Am. J. Epidemiol. 186, 1026–1034 (2017).
Taylor, A. E. et al. Exploring the association of genetic factors with participation in the Avon Longitudinal Study of Parents and Children. Int. J. Epidemiol. 47, 1207–1216 (2018).
Cole, S. R. et al. Illustrating bias due to conditioning on a collider. Int. J. Epidemiol. 39, 417–420 (2010).
Munafò, M. R., Tilling, K., Taylor, A. E., Evans, D. M. & Davey Smith, G. Collider scope: when selection bias can substantially influence observed associations. Int. J. Epidemiol. 47, 226–235 (2018).
Chang, C. C. et al. Second-generation PLINK: rising to the challenge of larger and richer datasets. Gigascience 4, 7 (2015).
Mistry, S., Harrison, J. R., Smith, D. J., Escott-Price, V. & Zammit, S. The use of polygenic risk scores to identify phenotypes associated with genetic risk of schizophrenia: Systematic review. Schizophr Res. 197, 2–8 (2017).
Abraham, G. et al. Genomic prediction of coronary heart disease. Eur. Heart J. 37, 3267–3278 (2016).
Okbay, A. et al. Genome-wide association study identifies 74 loci associated with educational attainment. Nature 533, 539 (2016).
Locke, A. E. et al. Genetic studies of body mass index yield new insights for obesity biology. Nature 518, 197–U401 (2015).
Wood, A. R. et al. Defining the role of common variation in the genomic and biological architecture of adult human height. Nat. Genet. 46, 1173–1186 (2014).
Wood, S. N. Stable and efficient multiple smoothing parameter estimation for generalized additive models. J. Am. Stat. Assoc. 99, 673–686 (2004).
Wood, S. Mixed GAM computation vehicle with automatic smoothness estimation. https://cran.r-project.org/web/packages/mgcv/ (2017).
R Core Team. R: A Language and Environment for Statistical Computing (R Foundation for Statistical Computing, Vienna, 2016).
Kanai, M. et al. Genetic analysis of quantitative traits in the Japanese population links cell types to complex human diseases. Nat. Genet. 50, 390–400 (2018).
Robinson, M. R. et al. Population genetic differentiation of height and body mass index across Europe. Nat. Genet 47, 1357 (2015).
Turchin, M. C. et al. Evidence of widespread selection on standing variation in Europe at height-associated SNPs. Nat. Genet. 44, 1015–1019 (2012).
Berg, J. J. & Coop, G. A population genetic signal of polygenic adaptation. PLoS Genet. 10, e1004412 (2014).
Kong, A. et al. Selection against variants in the genome associated with educational attainment. Proc. Natl. Acad. Sci. USA 114, E727–E732 (2017).
Domingue, B. W., Rehkopf, D. H., Conley, D. & Boardman, J. D. Geographic clustering of polygenic scores at different stages of the life course. RSF 4, 137–149 (2018).
Hemani, G. et al. Automating Mendelian randomization through machine learning to construct a putative causal map of the human phenome. Preprint at bioRxiv https://doi.org/10.1101/173682 (2017).
Curtis, D. Polygenic risk score for schizophrenia is more strongly associated with ancestry than with schizophrenia. Psychiatr. Genet. 28, 85–89 (2018).
Kong, A. et al. The nature of nurture: effects of parental genotypes. Science 359, 424–428 (2018).
Smith, M. W. & O’Brien, S. J. Mapping by admixture linkage disequilibrium: advances, limitations and guidelines. Nat. Rev. Genet. 6, 623–632 (2005).
Heckerman, D. et al. Linear mixed model for heritability estimation that explicitly addresses environmental variation. Proc. Natl. Acad. Sci. USA 113, 7377–7382 (2016).
Young, A. I. et al. Relatedness disequilibrium regression estimates heritability without environmental bias. Nat. Genet. 50, 1304–1310 (2018).
Delaneau, O., Marchini, J. & Zagury, J. F. A linear complexity phasing method for thousands of genomes. Nat. Methods 9, 179–181 (2012).
Howie, B., Fuchsberger, C., Stephens, M., Marchini, J. & Abecasis, G. R. Fast and accurate genotype imputation in genome-wide association studies through pre-phasing. Nat. Genet. 44, 955 (2012). +.
Hellenthal, G. et al. A genetic atlas of human admixture history. Science 343, 747–751 (2014).
Lawson, D., van Dorp, L. & Falush, D. A tutorial on how not to over-interpret STRUCTURE and ADMIXTURE bar plots. Nat. Commun. 9, 3258 (2018).
UK Biobank. Protocol for a large-scale prospective epidemiological resource. http://www.ukbiobank.ac.uk/wp-content/uploads/2011/11/UK-Biobank-Protocol.pdf (2007).
Mitchell, R., Hemani, G., Dudding, T. & Paternoster, L. UK Biobank Genetic Data: MRC-IEU Quality Control, Version 1 (University of Bristol, Bristol, 2017).
Elsworth, B. et al. MRC IEU UK Biobank GWAS pipeline version 1 (University of Bristol, Bristol, 2017).
Hemani, G. et al. The MR-Base platform supports systematic causal inference across the human phenome. eLife 7, e34408 (2018).
Acknowledgements
We are extremely grateful to all the families who took part in this study, the midwives for their help in recruiting them, and the whole ALSPAC team, which includes interviewers, computer and laboratory technicians, clerical workers, research scientists, volunteers, managers, receptionists and nurses.The UK Medical Research Council and Wellcome (Grant ref: 102215/2/13/2) and the University of Bristol provide core support for ALSPAC. A comprehensive list of grants funding is available on the ALSPAC website at http://www.bristol.ac.uk/alspac/external/documents/grant-acknowledgements.pdf. The UK Medical Research Council (MRC) and the University of Bristol support the MRC IEU. N.T. is a Wellcome Trust Investigator (202802/Z/16/Z), a work-package lead in the Integrative Cancer Epidemiology Programme (ICEP) that is supported by a Cancer Research UK programme grant (C18281/A19169) and works within the University of Bristol NIHR Biomedical Research Centre (BRC). D.J.L. is funded by Wellcome Trust and Royal Society (Grant ref: WT104125MA). The Economics and Social Research Council (ESRC) support N.D. via a Future Research Leaders grant (Grant ref: ES/N000757/1). G.D.S. is the director of and a programme lead in the MRC-IEU (MC_UU_12013/1). G.H. receives funding from the Wellcome Trust (Grant Ref: 208806/Z/17/Z). K.H.W. is funded by programmes 3 and 4 of the MRC IEU (Grant refs; MC_UU_12013/3 and MC_UU_12013/4), and by Wellcome Trust funding (Grant ref: 202802/Z/16/Z awarded to N.T.). S.H. receives support from Wellcome (Grant ref: 201237/Z/16/Z). No funding body has influenced data analysis or interpretation. This work was carried out using the computation facilities of the Advanced Computing Research Centre http://www.bris.ac.uk/acrc/, and the Research Data Storage Facility of the University of Bristol http://www.bris.ac.uk/acrc/storage/. We wish to acknowledge the contributions of Professor Augustine Kong of the Big Data Institute, Oxford University. Professor Kong helped in the preparation of this manuscript through discussion and development of themes central this work. This work arose from discussion within the MRC IEU dry lab meeting group, which is a community of users of genetic data at the MRC IEU. This group meets regularly to discuss analysis of genetic data and observations during these meetings formed the starting point for this work. We are very grateful to all the members of this group for their input.
Author information
Authors and Affiliations
Contributions
N.T., S.H. and N.D. conceived the study; S.H., D.J.L., R.M. and N.D. performed the analysis; S.H., D.J.L. and N.T. wrote the paper. All authors discussed the result and commented on the paper.
Corresponding author
Ethics declarations
Competing interests
D.J.L. is a director of and shareholder in GENSCI LTD. The authors declare no competing interests.
Additional information
Publisher’s note: Springer Nature remains neutral with regard to jurisdictional claims in published maps and institutional affiliations.
Supplementary information
Rights and permissions
Open Access This article is licensed under a Creative Commons Attribution 4.0 International License, which permits use, sharing, adaptation, distribution and reproduction in any medium or format, as long as you give appropriate credit to the original author(s) and the source, provide a link to the Creative Commons license, and indicate if changes were made. The images or other third party material in this article are included in the article’s Creative Commons license, unless indicated otherwise in a credit line to the material. If material is not included in the article’s Creative Commons license and your intended use is not permitted by statutory regulation or exceeds the permitted use, you will need to obtain permission directly from the copyright holder. To view a copy of this license, visit http://creativecommons.org/licenses/by/4.0/.
About this article
Cite this article
Haworth, S., Mitchell, R., Corbin, L. et al. Apparent latent structure within the UK Biobank sample has implications for epidemiological analysis. Nat Commun 10, 333 (2019). https://doi.org/10.1038/s41467-018-08219-1
Received:
Accepted:
Published:
DOI: https://doi.org/10.1038/s41467-018-08219-1