Abstract
The growing awareness of property safety inspections among governments and the public has fueled the demand for efficient, automated methods of damage assessment. Despite this, there is a notable scarcity of datasets specifically designed for house damage classification tasks. To address this gap, this paper presents the Build Damage Classification (BDC) Dataset, an enhanced dataset built upon xBD, incorporating three distinct sub-datasets for building damage classification. Additionally, to assess the impact of noise and low-quality data on model performance, two contrastive learning methods-DINOv2 and MoCo v2-are applied to classify property damage resulting from natural disasters. Experimental results reveal that DINOv2 significantly outperforms traditional CNNs and MoCo v2, with a notable improvement of approximately 20% in precision, recall, and F1 scores on the highly imbalanced and low-quality BDC dataset. Moreover, attention maps and gradient visualization techniques are used to explain the performance differences between the models.
Access this chapter
Tax calculation will be finalised at checkout
Purchases are for personal use only
Similar content being viewed by others
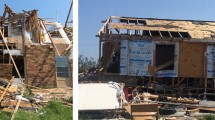
References
Stepinac, M., et al.: Damage classification of residential buildings in historical downtown after the ML5.5 earthquake in Zagreb, Croatia in 2020. Int. J. Disast. Risk Reduct. 56, 102140 (2021). https://doi.org/10.1016/j.ijdrr.2021.102140
Goyal, P.K.: Cyclonic damage assessment of rural houses for the east coastal region of India. Prog. Disast. Sci. 15, 100238 (2022). https://doi.org/10.1016/j.pdisas.2022.100238
Habibi, A., Delavar, M.R., Nazari, B., Pirasteh, S., Sadeghian, M.S.: A novel approach for flood hazard assessment using hybridized ensemble models and feature selection algorithms. Int. J. Appl. Earth Obs. Geoinf. 122, 103443 (2023). https://doi.org/10.1016/j.jag.2023.103443
Islam, M.M., Matsushita, S., Noguchi, R., Ahamed, T.: A damage-based crop insurance system for flash flooding: a satellite remote sensing and econometric approach. Asia-Pacific J. Reg. Sci. 6(1), 47–89 (2022). https://doi.org/10.1007/s41685-021-00220-9
Shrestha, B.B., Kawasaki, A., Zin, W.W.: Development of flood damage assessment method for residential areas considering various house types for Bago Region of Myanmar. Int. J. Disast. Risk Reduct. 66, 102602 (2021). https://doi.org/10.1016/j.ijdrr.2021.102602
Van Der Sande, C., Soudarissanane, S., Khoshelham, K.: Assessment of relative accuracy of AHN-2 laser scanning data using planar features. Sensors (Basel) 10(9), 8198–8214 (2010). https://doi.org/10.3390/s100908198
Yamazaki, F., Kouchi, K., Kohiyama, M., Muraoka, N., Matsuoka, M.: Earthquake damage detection using high-resolution satellite images. In: IGARSS 2004 IEEE International Geoscience and Remote Sensing Symposium, pp. 2280–2283. IEEE (2004)
Bai, Y., Chen, X., Kirillov, A., Yuille, A., Berg, A.C.: Point-level region contrast for object detection pre-training. In: Proceedings of the IEEE/CVF Conference on Computer Vision and Pattern Recognition, pp. 16061–16070 (2022)
Saito, K., Spence, R.J., Going, C., Markus, M.: Using high-resolution satellite images for post-earthquake building damage assessment: a study following the 26 January 2001 Gujarat earthquake. Earthq. Spectra 20(1), 145–169 (2004)
Rastiveis, H., Hosseini-Zirdoo, E., Eslamizade, F.: Automatic blocked roads assessment after earthquake using high resolution satellite imagery. Int. Arch. Photogram. Remote Sens. Spatial Inf. Sci. XL-1/W5, 601–605 (2015). https://doi.org/10.5194/isprsarchives-XL-1-W5-601-2015
Elberink, S.O., Shoko, M., Fathi, S.A., Rutzinger, M.: Detection of collapsed buildings by classifying segmented airborne laser scanner data. Int. Arch. Photogram. Remote Sens. Spatial Inf. Sci. 38(5), W12 (2011)
Chehata, N., Guo, L., Mallet, C.: Airborne lidar feature selection for urban classification using random forests. In: Laserscanning 2009 (2009)
Gong, L., Wang, C., Wu, F., Zhang, J., Zhang, H., Li, Q.: Earthquake-induced building damage detection with post-event sub-meter VHR TerraSAR-X staring spotlight imagery. Remote Sens. 8(11) (2016). https://doi.org/10.3390/rs8110887
Fujita, A., Sakurada, K., Imaizumi, T., Ito, R., Hikosaka, S., Nakamura, R.:Damage detection from aerial images via convolutional neural networks. In: 2017 Fifteenth IAPR International Conference on Machine Vision Applications (MVA), pp. 5–8. IEEE (2017)
Kaur, S., Gupta, S., Singh, S., Koundal, D., Zaguia, A.: Convolutional neural network based hurricane damage detection using satellite images. Soft. Comput. 26(16), 7831–7845 (2022). https://doi.org/10.1007/s00500-022-06805-6
Fan, J., Xu, C., Zhang, J.: An ensemble learning approach of multi-model for classifying house damage. In: 2021 2nd International Conference on Big Data & Artificial Intelligence & Software Engineering (ICBASE), pp. 145–152 (2021)
Li, Y., Gu, S.: Detecting post hurricane house damage using geographic information related multi-resource classification model. In: 2021 2nd International Conference on Big Data & Artificial Intelligence & Software Engineering (ICBASE), pp. 492–501 (2021)
Krieger, J., Higgins, D.L.: Housing and health: time again for public health action. Am. J. Public Health 92(5), 758–768 (2002). https://doi.org/10.2105/ajph.92.5.758
Nix, E., Paulose, J., Shrubsole, C., Altamirano-Medina, H., Davies, M., Khosla, R., et al.: Evaluating housing health hazards: prevalence, practices and priorities in Delhi’s informal settlements. J. Urban Health 97(4), 502–518 (2020). https://doi.org/10.1007/s11524-020-00442-w
Standen, J.C., Morgan, G.G., Sowerbutts, T., Blazek, K., Gugusheff, J., Puntsag, O. et al.: Prioritising housing maintenance to improve health in indigenous communities in NSW over 20 years. Int. J. Environ. Res. Public Health 17(16) (2020). https://doi.org/10.3390/ijerph17165946
Zahs, V., Anders, K., Kohns, J., Stark, A., Höfle, B.: Classification of structural building damage grades from multi-temporal photogrammetric point clouds using a machine learning model trained on virtual laser scanning data. Int. J. Appl. Earth Obs. Geoinf. 122, 103406 (2023). https://doi.org/10.1016/j.jag.2023.103406
Mohd, T., Mohamed Saraf, M., Pin, S.F., Hasbullah, M.N.: Designing the invention house assessment form for Kuala Krai, Malaysia. Procedia Social Behav. Sci. 234, 317–325 (2016). https://doi.org/10.1016/j.sbspro.2016.10.248
Xu, J., Zeng, F., Liu, W., Takahashi, T.: Damage detection and level classification of roof damage after typhoon faxai based on aerial photos and deep learning. Appl. Sci. 12(10), 4912 (2022)
Oquab, M., Darcet, T., Moutakanni, T., Vo, H., Szafraniec, M., Khalidov, V., et al.: Dinov2: learning robust visual features without supervision. arXiv preprint arXiv:2304.07193 (2023)
Gupta, R., Goodman, B., Patel, N.N., Hosfelt, R., Sajeev, S., Heim, E.T., et al.: Creating xBD: a dataset for assessing building damage from satellite imagery. In: CVPR Workshops 2019 (2019)
Krizhevsky, A., Sutskever, I., Hinton, G.E.: ImageNet classification with deep convolutional neural networks. Adv. Neural Inf. Process. Syst. 25 (2012)
He, K., Zhang, X., Ren, S., Sun, J.: Deep residual learning for image recognition. In: Proceedings of the IEEE Conference on Computer Vision and Pattern Recognition, pp. 770–778 (2016)
Simonyan, K., Zisserman, A.: Very deep convolutional networks for large-scale image recognition. arXiv preprint arXiv:1409.1556 (2014)
Howard, A.G., Zhu, M., Chen, B., Kalenichenko, D., Wang, W., Weyand, T., et al.: MobileNets: efficient convolutional neural networks for mobile vision applications. arXiv preprint arXiv:1704.04861 (2017)
Huang, G., Liu, Z., Van Der Maaten, L., Weinberger, K.Q.: Densely connected convolutional networks. In: Proceedings of the IEEE Conference on Computer Vision and Pattern Recognition 2017, pp. 4700–4708 (2017)
Ma, N., Zhang, X., Zheng, H.-T., Sun, J.: ShuffleNet V2: practical guidelines for efficient CNN architecture design. In: Proceedings of the European Conference on Computer Vision (ECCV) 2018, pp. 116–131 (2018)
Iandola, F.N., Han, S., Moskewicz, M.W., Ashraf, K., Dally, W.J., Keutzer, K.: SqueezeNet: AlexNet-level accuracy with 50x fewer parameters and \({<}0.5\) MB model size. arXiv preprint arXiv:1602.07360 (2016)
Tan, M., Chen, B., Pang, R., Vasudevan, V., Sandler, M., Howard, A., et al.: MnasNet: platform-aware neural architecture search for mobile. In: Proceedings of the IEEE/CVF Conference on Computer Vision and Pattern Recognition, pp. 2820–2828 (2019)
Chen, X., Fan, H., Girshick, R., He, K.: Improved baselines with momentum contrastive learning. arXiv preprint arXiv:2003.04297 (2020)
Author information
Authors and Affiliations
Corresponding author
Editor information
Editors and Affiliations
Rights and permissions
Copyright information
© 2025 The Author(s), under exclusive license to Springer Nature Singapore Pte Ltd.
About this paper
Cite this paper
Zi, X. et al. (2025). BDC Dataset: A Comprehensive Dataset for Automated Build Damage Classification. In: Sheng, Q.Z., et al. Advanced Data Mining and Applications. ADMA 2024. Lecture Notes in Computer Science(), vol 15387. Springer, Singapore. https://doi.org/10.1007/978-981-96-0811-9_7
Download citation
DOI: https://doi.org/10.1007/978-981-96-0811-9_7
Published:
Publisher Name: Springer, Singapore
Print ISBN: 978-981-96-0810-2
Online ISBN: 978-981-96-0811-9
eBook Packages: Computer ScienceComputer Science (R0)