Abstract
The understanding of the relation between structure and function in the brain requires theoretical frameworks capable of dealing with a large variety of complex experimental data. Likewise neural computation strives to design structures from which complex functionality should emerge. The framework of information theory has been partially successful in explaining certain brain structures with respect to sensory transformations under restricted conditions. Yet classical measures of information have not taken an explicit account of some of the fundamental concepts in brain theory and neural computation: namely that optimal coding depends on the specific task(s) to be solved by the system, and that autonomy and goal orientedness also depend on extracting relevant information from the environment and specific knowledge from the receiver to be able to affect it in the desired way. This paper presents a general (i.e. implementation independent) new information processing measure that takes into account the previously mentioned issues. It is based on measuring the transformations required to go from the original alphabet in which the sensory messages are represented, to the objective alphabet which depends on the implicit task(s) imposed by the environment-system relation.
Access this chapter
Tax calculation will be finalised at checkout
Purchases are for personal use only
Preview
Unable to display preview. Download preview PDF.
Similar content being viewed by others
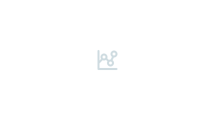
References
Atick, J.J.: Could information theory provide an ecological theory of sensory processing? Network 3 (1992) 213–251
Barlow, H.B.: Unsupervised learning. Neur. Comput. 1 (1989) 295–311
Borst, A., Theunissen, F.E.: Information theory and neural coding. Nat. Neurosc. 2 (1999) 947–57
Cover, T.M., Thomas, J.A.: Elements of Information Theory. John Wiley, New York (1991)
Fisher, R.A.: Statistical Methods and Scientific Inference, 2nd edition. Oliver and Boyd: London (1959)
Kolmogorov, A.: Three approaches to the quantitative definition of information. Problems of Information Transmission 1 (1965) 1–11
Linsker, R.: Self-organization in a perceptual network. IEEE Computer 21 (1988) 105–117
MacKay, D.J.C.: Information Theory, Inference and Learning Algorithms. Textbook in preparation, to be published by Cambridge University Press. Available from http://www.inference.phy.cam.ac.uk/mackay/itprnn/ (1999)
López de Mántaras, R.: A Distance-based Attribute Selection Measure for Decision Tree Induction. Machine Learning Journal 6 (1991) 81–92
Prechelt, L.: PROBEN1: A Set of Neural Network Benchmark Problems and Benchmarking Rules, Technical Report 21/94, (1994), Fakultat fur Informatik, Universität Karlsruhe, Germany
Ruderman, D. L.: The statistics of natural images. Network 5 (1994) 517–548
Sánchez-Montañés, M.A., Corbacho, F.: Towards a new information processing measure for neural computation. Internal Report, (2002), ETS de Informática, Universidad Autńoma de Madrid, Spain. Available at http://www.ii.uam.es/~msanchez/papers (2002)
Shannon, C.E., Weaver, W.: The Mathematical Theory of Communication. University of Illinois Press, Urbana (1949)
Author information
Authors and Affiliations
Editor information
Editors and Affiliations
Rights and permissions
Copyright information
© 2002 Springer-Verlag Berlin Heidelberg
About this paper
Cite this paper
Sánchez-Montañés, M.A., Corbacho, F.J. (2002). Towards a New Information Processing Measure for Neural Computation. In: Dorronsoro, J.R. (eds) Artificial Neural Networks — ICANN 2002. ICANN 2002. Lecture Notes in Computer Science, vol 2415. Springer, Berlin, Heidelberg. https://doi.org/10.1007/3-540-46084-5_104
Download citation
DOI: https://doi.org/10.1007/3-540-46084-5_104
Published:
Publisher Name: Springer, Berlin, Heidelberg
Print ISBN: 978-3-540-44074-1
Online ISBN: 978-3-540-46084-8
eBook Packages: Springer Book Archive