Abstract
In this paper we present a novel method for recognizing all kinds of abnormalities in digital mammograms using Independent Component Analysis mixture models and two sets of statistical features based on texture analysis.Our approach is concentrated on finding the ICA mixture model parameters that describe in an exclusive and effective way the abnormal and the normal tissue, and with the aid of a supervised probabilistic classifier we are able to successfully recognize suspicious regions in mammograms. Extensive experiments using the MIAS database have shown great accuracy of 98.33% in classifying an unknown regions of suspicion as abnormal and 62.71% as healthy tissue.
Preview
Unable to display preview. Download preview PDF.
Similar content being viewed by others
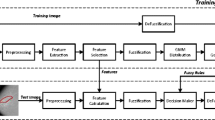
References
Martin, J., Moskowitz, M. and Milbrath, J.: Breast cancer missed by mammography. AJR, Vol. 132. (1979) 737
Kalisher, L.: Factors influencing false negative rates in xero-mammography. Radiology, Vol. 133. (1979) 297
Tabar, L. and Dean, B.P.: Teaching Atlas of Mammography. 2nd edition, Thieme, NY (1985)
Christoyianni, I., Dermatas, E., and Kokkinakis, G.: Fast Detection of Masses in Computer-Aided Mammography. IEEE Signal Processing Magazine, vol. 17, no 1. (2000) 54–64
Sonka, M., Fitzpatrick, M.,: Handbook of Medical Imaging, Vol 2, SPIE Press (2000).
Doi, K., Giger, M., Nishikawa, R., and Schmidt, R. (eds.): Digital Mammography 96. Elsevier Amsterdam(1996)
Lee, Te-Won: Independent Component Analysis: Theory and Applications. Kluwer Academic Publishers (1998)
Lee, T.-W., Lewicki, M: The Generalized Gaussian Mixture Model Using ICA. International Workshop on Independent Component Analysis. Helsinki (2000) 239–244
Haralick, R.: Statistical and Structural Approaches to Texture. Proc. IEEE, Vol. 67, No. 4. (1979) 786–804
Lee, T.-W., Lewicki, M and Sejnowski, T.: ICA Mixture Models for Unsupervised Classification of Non-Gaussian Sources and Automatic Context Switching in Blind Signal Separation. IEEE Trans. on Pattern Recognition and Machine Intelligence 22(10) (2000) 1–12
Duda, R and Hart, P.: Pattern Classification and Scene Analysis. New York Wiley (1973)
Cardoso, J.: Blind Signal Separation: statistical principles. IEEE Proceedings 9(10)(1998) 2009–2025
Author information
Authors and Affiliations
Editor information
Editors and Affiliations
Rights and permissions
Copyright information
© 2001 Springer-Verlag Berlin Heidelberg
About this paper
Cite this paper
Christoyianni, I., Koutras, A., Dermatas, E., Kokkinakis, G. (2001). Breast Tissue Classification in Mammograms Using ICA Mixture Models. In: Dorffner, G., Bischof, H., Hornik, K. (eds) Artificial Neural Networks — ICANN 2001. ICANN 2001. Lecture Notes in Computer Science, vol 2130. Springer, Berlin, Heidelberg. https://doi.org/10.1007/3-540-44668-0_78
Download citation
DOI: https://doi.org/10.1007/3-540-44668-0_78
Published:
Publisher Name: Springer, Berlin, Heidelberg
Print ISBN: 978-3-540-42486-4
Online ISBN: 978-3-540-44668-2
eBook Packages: Springer Book Archive