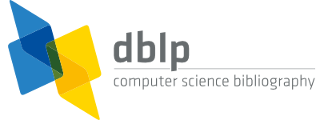


default search action
Sham M. Kakade
Person information
- affiliation: Harvard University, Cambridge, MA, USA
- affiliation (former): University of Washington, Department of Statistics, Seattle, WA, USA
- affiliation (former): Microsoft Research New England, Cambridge, MA, USA
- affiliation (former): Toyota Technological Institute at Chicago, IL, USA
- affiliation (former): University of Pennsylvania, Department of Statistics, Philadelphia, PA, USA
- affiliation: University College London, Gatsby Computational Neuroscience Unit, UK
SPARQL queries 
Refine list

refinements active!
zoomed in on ?? of ?? records
view refined list in
export refined list as
2020 – today
- 2024
- [j31]Motoya Ohnishi, Isao Ishikawa, Kendall Lowrey, Masahiro Ikeda, Sham M. Kakade, Yoshinobu Kawahara:
Koopman Spectrum Nonlinear Regulators and Efficient Online Learning. Trans. Mach. Learn. Res. 2024 (2024) - [c157]Depen Morwani, Benjamin L. Edelman, Costin-Andrei Oncescu, Rosie Zhao, Sham M. Kakade:
Feature emergence via margin maximization: case studies in algebraic tasks. ICLR 2024 - [c156]Nikhil Vyas, Depen Morwani, Rosie Zhao, Gal Kaplun, Sham M. Kakade, Boaz Barak:
Beyond Implicit Bias: The Insignificance of SGD Noise in Online Learning. ICML 2024 - [c155]Kenneth Li, Samy Jelassi, Hugh Zhang, Sham M. Kakade, Martin Wattenberg, David Brandfonbrener:
Q-Probe: A Lightweight Approach to Reward Maximization for Language Models. ICML 2024 - [c154]Samy Jelassi, David Brandfonbrener, Sham M. Kakade, Eran Malach:
Repeat After Me: Transformers are Better than State Space Models at Copying. ICML 2024 - [c153]Hanlin Zhang, Yifan Zhang, Yaodong Yu, Dhruv Madeka, Dean P. Foster, Eric P. Xing, Himabindu Lakkaraju, Sham M. Kakade:
A Study on the Calibration of In-context Learning. NAACL-HLT 2024: 6118-6136 - [i153]Samy Jelassi, David Brandfonbrener, Sham M. Kakade, Eran Malach:
Repeat After Me: Transformers are Better than State Space Models at Copying. CoRR abs/2402.01032 (2024) - [i152]Kenneth Li, Samy Jelassi, Hugh Zhang, Sham M. Kakade, Martin Wattenberg, David Brandfonbrener:
Q-Probe: A Lightweight Approach to Reward Maximization for Language Models. CoRR abs/2402.14688 (2024) - [i151]Zhenting Qi, Hanlin Zhang, Eric P. Xing, Sham M. Kakade, Himabindu Lakkaraju:
Follow My Instruction and Spill the Beans: Scalable Data Extraction from Retrieval-Augmented Generation Systems. CoRR abs/2402.17840 (2024) - [i150]Yiwen Kou, Zixiang Chen, Quanquan Gu, Sham M. Kakade:
Matching the Statistical Query Lower Bound for k-sparse Parity Problems with Stochastic Gradient Descent. CoRR abs/2404.12376 (2024) - [i149]Ethan Shen, Alan Fan, Sarah M. Pratt, Jae Sung Park, Matthew Wallingford, Sham M. Kakade, Ari Holtzman, Ranjay Krishna, Ali Farhadi, Aditya Kusupati:
Superposed Decoding: Multiple Generations from a Single Autoregressive Inference Pass. CoRR abs/2405.18400 (2024) - [i148]Licong Lin, Jingfeng Wu, Sham M. Kakade, Peter L. Bartlett, Jason D. Lee:
Scaling Laws in Linear Regression: Compute, Parameters, and Data. CoRR abs/2406.08466 (2024) - [i147]David Brandfonbrener, Hanlin Zhang, Andreas Kirsch, Jonathan Richard Schwarz, Sham M. Kakade:
CoLoR-Filter: Conditional Loss Reduction Filtering for Targeted Language Model Pre-training. CoRR abs/2406.10670 (2024) - [i146]Edwin Zhang, Vincent Zhu, Naomi Saphra, Anat Kleiman, Benjamin L. Edelman, Milind Tambe, Sham M. Kakade, Eran Malach:
Transcendence: Generative Models Can Outperform The Experts That Train Them. CoRR abs/2406.11741 (2024) - [i145]Jeffrey Li, Alex Fang, Georgios Smyrnis, Maor Ivgi, Matt Jordan, Samir Yitzhak Gadre, Hritik Bansal, Etash Kumar Guha, Sedrick Keh, Kushal Arora, Saurabh Garg, Rui Xin, Niklas Muennighoff, Reinhard Heckel, Jean Mercat, Mayee Chen, Suchin Gururangan, Mitchell Wortsman, Alon Albalak, Yonatan Bitton, Marianna Nezhurina, Amro Abbas, Cheng-Yu Hsieh, Dhruba Ghosh, Josh Gardner, Maciej Kilian, Hanlin Zhang, Rulin Shao, Sarah M. Pratt, Sunny Sanyal, Gabriel Ilharco, Giannis Daras, Kalyani Marathe, Aaron Gokaslan, Jieyu Zhang, Khyathi Raghavi Chandu, Thao Nguyen, Igor Vasiljevic, Sham M. Kakade, Shuran Song, Sujay Sanghavi, Fartash Faghri, Sewoong Oh, Luke Zettlemoyer, Kyle Lo, Alaaeldin El-Nouby, Hadi Pouransari, Alexander Toshev, Stephanie Wang, Dirk Groeneveld, Luca Soldaini, Pang Wei Koh, Jenia Jitsev, Thomas Kollar, Alexandros G. Dimakis, Yair Carmon, Achal Dave, Ludwig Schmidt, Vaishaal Shankar:
DataComp-LM: In search of the next generation of training sets for language models. CoRR abs/2406.11794 (2024) - [i144]Depen Morwani, Itai Shapira, Nikhil Vyas, Eran Malach, Sham M. Kakade, Lucas Janson:
A New Perspective on Shampoo's Preconditioner. CoRR abs/2406.17748 (2024) - [i143]Ziqi Wang, Hanlin Zhang, Xiner Li, Kuan-Hao Huang, Chi Han, Shuiwang Ji, Sham M. Kakade, Hao Peng, Heng Ji:
Eliminating Position Bias of Language Models: A Mechanistic Approach. CoRR abs/2407.01100 (2024) - [i142]Kaiying Hou, David Brandfonbrener, Sham M. Kakade, Samy Jelassi, Eran Malach:
Universal Length Generalization with Turing Programs. CoRR abs/2407.03310 (2024) - [i141]Rosie Zhao, Depen Morwani, David Brandfonbrener, Nikhil Vyas, Sham M. Kakade:
Deconstructing What Makes a Good Optimizer for Language Models. CoRR abs/2407.07972 (2024) - [i140]Natalia Zhang, Xinqi Wang, Qiwen Cui, Runlong Zhou, Sham M. Kakade, Simon S. Du:
Multi-Agent Reinforcement Learning from Human Feedback: Data Coverage and Algorithmic Techniques. CoRR abs/2409.00717 (2024) - [i139]Nikhil Vyas, Depen Morwani, Rosie Zhao, Itai Shapira, David Brandfonbrener, Lucas Janson, Sham M. Kakade:
SOAP: Improving and Stabilizing Shampoo using Adam. CoRR abs/2409.11321 (2024) - [i138]Carson Eisenach, Udaya Ghai, Dhruv Madeka, Kari Torkkola, Dean P. Foster, Sham M. Kakade:
Neural Coordination and Capacity Control for Inventory Management. CoRR abs/2410.02817 (2024) - [i137]Costin-Andrei Oncescu, Sanket Purandare, Stratos Idreos, Sham M. Kakade:
Flash Inference: Near Linear Time Inference for Long Convolution Sequence Models and Beyond. CoRR abs/2410.12982 (2024) - [i136]Akshara Prabhakar, Yuanzhi Li, Karthik Narasimhan, Sham M. Kakade, Eran Malach, Samy Jelassi:
LoRA Soups: Merging LoRAs for Practical Skill Composition Tasks. CoRR abs/2410.13025 (2024) - [i135]Samy Jelassi, Clara Mohri, David Brandfonbrener, Alex Gu, Nikhil Vyas, Nikhil Anand, David Alvarez-Melis, Yuanzhi Li, Sham M. Kakade, Eran Malach:
Mixture of Parrots: Experts improve memorization more than reasoning. CoRR abs/2410.19034 (2024) - [i134]Hanlin Zhang, Depen Morwani, Nikhil Vyas, Jingfeng Wu, Difan Zou, Udaya Ghai, Dean P. Foster, Sham M. Kakade:
How Does Critical Batch Size Scale in Pre-training? CoRR abs/2410.21676 (2024) - 2023
- [j30]Kaiqing Zhang, Sham M. Kakade, Tamer Basar, Lin F. Yang:
Model-Based Multi-Agent RL in Zero-Sum Markov Games with Near-Optimal Sample Complexity. J. Mach. Learn. Res. 24: 175:1-175:53 (2023) - [j29]Juan C. Perdomo, Akshay Krishnamurthy, Peter L. Bartlett, Sham M. Kakade:
A Complete Characterization of Linear Estimators for Offline Policy Evaluation. J. Mach. Learn. Res. 24: 284:1-284:50 (2023) - [j28]Difan Zou, Jingfeng Wu, Vladimir Braverman, Quanquan Gu, Sham M. Kakade:
Benign Overfitting of Constant-Stepsize SGD for Linear Regression. J. Mach. Learn. Res. 24: 326:1-326:58 (2023) - [c152]Gaurav Mahajan, Sham M. Kakade, Akshay Krishnamurthy, Cyril Zhang:
Learning Hidden Markov Models Using Conditional Samples. COLT 2023: 2014-2066 - [c151]Tengyang Xie, Dylan J. Foster, Yu Bai, Nan Jiang, Sham M. Kakade:
The Role of Coverage in Online Reinforcement Learning. ICLR 2023 - [c150]Dylan J. Foster, Noah Golowich, Sham M. Kakade:
Hardness of Independent Learning and Sparse Equilibrium Computation in Markov Games. ICML 2023: 10188-10221 - [c149]Nikhil Vyas, Sham M. Kakade, Boaz Barak:
On Provable Copyright Protection for Generative Models. ICML 2023: 35277-35299 - [c148]Jingfeng Wu, Difan Zou, Zixiang Chen, Vladimir Braverman, Quanquan Gu, Sham M. Kakade:
Finite-Sample Analysis of Learning High-Dimensional Single ReLU Neuron. ICML 2023: 37919-37951 - [c147]Benjamin L. Edelman, Surbhi Goel, Sham M. Kakade, Eran Malach, Cyril Zhang:
Pareto Frontiers in Deep Feature Learning: Data, Compute, Width, and Luck. NeurIPS 2023 - [c146]Aniket Rege, Aditya Kusupati, Sharan Ranjit S, Alan Fan, Qingqing Cao, Sham M. Kakade, Prateek Jain, Ali Farhadi:
AdANNS: A Framework for Adaptive Semantic Search. NeurIPS 2023 - [c145]Krishna Pillutla, Vincent Roulet, Sham M. Kakade, Zaïd Harchaoui:
Modified Gauss-Newton Algorithms under Noise. SSP 2023: 51-55 - [i133]Nikhil Vyas, Sham M. Kakade, Boaz Barak:
Provable Copyright Protection for Generative Models. CoRR abs/2302.10870 (2023) - [i132]Sham M. Kakade, Akshay Krishnamurthy, Gaurav Mahajan, Cyril Zhang:
Learning Hidden Markov Models Using Conditional Samples. CoRR abs/2302.14753 (2023) - [i131]Jingfeng Wu, Difan Zou, Zixiang Chen, Vladimir Braverman, Quanquan Gu, Sham M. Kakade:
Learning High-Dimensional Single-Neuron ReLU Networks with Finite Samples. CoRR abs/2303.02255 (2023) - [i130]Dylan J. Foster, Noah Golowich, Sham M. Kakade:
Hardness of Independent Learning and Sparse Equilibrium Computation in Markov Games. CoRR abs/2303.12287 (2023) - [i129]Krishna Pillutla, Vincent Roulet, Sham M. Kakade, Zaïd Harchaoui:
Modified Gauss-Newton Algorithms under Noise. CoRR abs/2305.10634 (2023) - [i128]Aniket Rege, Aditya Kusupati, Sharan Ranjit S, Alan Fan, Qingqing Cao, Sham M. Kakade, Prateek Jain, Ali Farhadi:
AdANNS: A Framework for Adaptive Semantic Search. CoRR abs/2305.19435 (2023) - [i127]Nikhil Vyas, Depen Morwani, Rosie Zhao, Gal Kaplun, Sham M. Kakade, Boaz Barak:
Beyond Implicit Bias: The Insignificance of SGD Noise in Online Learning. CoRR abs/2306.08590 (2023) - [i126]Jens Tuyls, Dhruv Madeka, Kari Torkkola, Dean P. Foster, Karthik Narasimhan, Sham M. Kakade:
Scaling Laws for Imitation Learning in NetHack. CoRR abs/2307.09423 (2023) - [i125]Benjamin L. Edelman, Surbhi Goel, Sham M. Kakade, Eran Malach, Cyril Zhang:
Pareto Frontiers in Neural Feature Learning: Data, Compute, Width, and Luck. CoRR abs/2309.03800 (2023) - [i124]Devvrit, Sneha Kudugunta, Aditya Kusupati, Tim Dettmers, Kaifeng Chen, Inderjit S. Dhillon, Yulia Tsvetkov, Hannaneh Hajishirzi, Sham M. Kakade, Ali Farhadi, Prateek Jain:
MatFormer: Nested Transformer for Elastic Inference. CoRR abs/2310.07707 (2023) - [i123]Sohrab Andaz, Carson Eisenach, Dhruv Madeka, Kari Torkkola, Randy Jia, Dean P. Foster, Sham M. Kakade:
Learning an Inventory Control Policy with General Inventory Arrival Dynamics. CoRR abs/2310.17168 (2023) - [i122]Depen Morwani, Benjamin L. Edelman, Costin-Andrei Oncescu, Rosie Zhao, Sham M. Kakade:
Feature emergence via margin maximization: case studies in algebraic tasks. CoRR abs/2311.07568 (2023) - [i121]Hanlin Zhang, Yifan Zhang, Yaodong Yu, Dhruv Madeka, Dean P. Foster, Eric P. Xing, Himabindu Lakkaraju, Sham M. Kakade:
A Study on the Calibration of In-context Learning. CoRR abs/2312.04021 (2023) - 2022
- [j27]Krishna Pillutla
, Sham M. Kakade, Zaïd Harchaoui:
Robust Aggregation for Federated Learning. IEEE Trans. Signal Process. 70: 1142-1154 (2022) - [c144]Jordan T. Ash, Cyril Zhang, Surbhi Goel, Akshay Krishnamurthy, Sham M. Kakade:
Anti-Concentrated Confidence Bonuses For Scalable Exploration. ICLR 2022 - [c143]Jens Tuyls, Shunyu Yao, Sham M. Kakade, Karthik Narasimhan:
Multi-Stage Episodic Control for Strategic Exploration in Text Games. ICLR 2022 - [c142]Benjamin L. Edelman
, Surbhi Goel, Sham M. Kakade, Cyril Zhang:
Inductive Biases and Variable Creation in Self-Attention Mechanisms. ICML 2022: 5793-5831 - [c141]Yonathan Efroni, Sham M. Kakade, Akshay Krishnamurthy, Cyril Zhang:
Sparsity in Partially Controllable Linear Systems. ICML 2022: 5851-5860 - [c140]Nikunj Saunshi, Jordan T. Ash, Surbhi Goel, Dipendra Misra, Cyril Zhang, Sanjeev Arora, Sham M. Kakade, Akshay Krishnamurthy:
Understanding Contrastive Learning Requires Incorporating Inductive Biases. ICML 2022: 19250-19286 - [c139]Jingfeng Wu, Difan Zou, Vladimir Braverman, Quanquan Gu, Sham M. Kakade:
Last Iterate Risk Bounds of SGD with Decaying Stepsize for Overparameterized Linear Regression. ICML 2022: 24280-24314 - [c138]Abhishek Gupta, Aldo Pacchiano, Yuexiang Zhai, Sham M. Kakade, Sergey Levine:
Unpacking Reward Shaping: Understanding the Benefits of Reward Engineering on Sample Complexity. NeurIPS 2022 - [c137]Boaz Barak, Benjamin L. Edelman, Surbhi Goel, Sham M. Kakade, Eran Malach, Cyril Zhang:
Hidden Progress in Deep Learning: SGD Learns Parities Near the Computational Limit. NeurIPS 2022 - [c136]Surbhi Goel, Sham M. Kakade, Adam Kalai, Cyril Zhang:
Recurrent Convolutional Neural Networks Learn Succinct Learning Algorithms. NeurIPS 2022 - [c135]Aditya Kusupati, Gantavya Bhatt, Aniket Rege, Matthew Wallingford, Aditya Sinha, Vivek Ramanujan, William Howard-Snyder, Kaifeng Chen, Sham M. Kakade, Prateek Jain, Ali Farhadi:
Matryoshka Representation Learning. NeurIPS 2022 - [c134]Jingfeng Wu, Difan Zou, Vladimir Braverman, Quanquan Gu, Sham M. Kakade:
The Power and Limitation of Pretraining-Finetuning for Linear Regression under Covariate Shift. NeurIPS 2022 - [c133]Difan Zou, Jingfeng Wu, Vladimir Braverman, Quanquan Gu, Sham M. Kakade:
Risk Bounds of Multi-Pass SGD for Least Squares in the Interpolation Regime. NeurIPS 2022 - [i120]Jens Tuyls, Shunyu Yao, Sham M. Kakade, Karthik Narasimhan:
Multi-Stage Episodic Control for Strategic Exploration in Text Games. CoRR abs/2201.01251 (2022) - [i119]Nikunj Saunshi, Jordan T. Ash, Surbhi Goel, Dipendra Misra, Cyril Zhang, Sanjeev Arora, Sham M. Kakade, Akshay Krishnamurthy:
Understanding Contrastive Learning Requires Incorporating Inductive Biases. CoRR abs/2202.14037 (2022) - [i118]Difan Zou, Jingfeng Wu, Vladimir Braverman, Quanquan Gu, Sham M. Kakade:
Risk Bounds of Multi-Pass SGD for Least Squares in the Interpolation Regime. CoRR abs/2203.03159 (2022) - [i117]Juan C. Perdomo, Akshay Krishnamurthy, Peter L. Bartlett, Sham M. Kakade:
A Sharp Characterization of Linear Estimators for Offline Policy Evaluation. CoRR abs/2203.04236 (2022) - [i116]Aditya Kusupati, Gantavya Bhatt, Aniket Rege, Matthew Wallingford, Aditya Sinha, Vivek Ramanujan, William Howard-Snyder
, Kaifeng Chen, Sham M. Kakade, Prateek Jain, Ali Farhadi:
Matryoshka Representations for Adaptive Deployment. CoRR abs/2205.13147 (2022) - [i115]Boaz Barak, Benjamin L. Edelman, Surbhi Goel, Sham M. Kakade, Eran Malach, Cyril Zhang:
Hidden Progress in Deep Learning: SGD Learns Parities Near the Computational Limit. CoRR abs/2207.08799 (2022) - [i114]Jingfeng Wu, Difan Zou, Vladimir Braverman, Quanquan Gu, Sham M. Kakade:
The Power and Limitation of Pretraining-Finetuning for Linear Regression under Covariate Shift. CoRR abs/2208.01857 (2022) - [i113]Surbhi Goel, Sham M. Kakade, Adam Tauman Kalai, Cyril Zhang:
Recurrent Convolutional Neural Networks Learn Succinct Learning Algorithms. CoRR abs/2209.00735 (2022) - [i112]Tengyang Xie, Dylan J. Foster, Yu Bai, Nan Jiang, Sham M. Kakade:
The Role of Coverage in Online Reinforcement Learning. CoRR abs/2210.04157 (2022) - [i111]Abhishek Gupta, Aldo Pacchiano, Yuexiang Zhai, Sham M. Kakade, Sergey Levine:
Unpacking Reward Shaping: Understanding the Benefits of Reward Engineering on Sample Complexity. CoRR abs/2210.09579 (2022) - 2021
- [j26]Chi Jin
, Praneeth Netrapalli, Rong Ge, Sham M. Kakade, Michael I. Jordan:
On Nonconvex Optimization for Machine Learning: Gradients, Stochasticity, and Saddle Points. J. ACM 68(2): 11:1-11:29 (2021) - [j25]Alekh Agarwal, Sham M. Kakade, Jason D. Lee, Gaurav Mahajan:
On the Theory of Policy Gradient Methods: Optimality, Approximation, and Distribution Shift. J. Mach. Learn. Res. 22: 98:1-98:76 (2021) - [c132]Difan Zou, Jingfeng Wu, Vladimir Braverman, Quanquan Gu, Sham M. Kakade:
Benign Overfitting of Constant-Stepsize SGD for Linear Regression. COLT 2021: 4633-4635 - [c131]Simon Shaolei Du, Wei Hu, Sham M. Kakade, Jason D. Lee, Qi Lei:
Few-Shot Learning via Learning the Representation, Provably. ICLR 2021 - [c130]Preetum Nakkiran, Prayaag Venkat, Sham M. Kakade, Tengyu Ma:
Optimal Regularization can Mitigate Double Descent. ICLR 2021 - [c129]Ruosong Wang, Dean P. Foster, Sham M. Kakade:
What are the Statistical Limits of Offline RL with Linear Function Approximation? ICLR 2021 - [c128]Yu Bai, Minshuo Chen, Pan Zhou, Tuo Zhao, Jason D. Lee, Sham M. Kakade, Huan Wang, Caiming Xiong:
How Important is the Train-Validation Split in Meta-Learning? ICML 2021: 543-553 - [c127]Simon S. Du, Sham M. Kakade, Jason D. Lee, Shachar Lovett, Gaurav Mahajan, Wen Sun, Ruosong Wang:
Bilinear Classes: A Structural Framework for Provable Generalization in RL. ICML 2021: 2826-2836 - [c126]Ruosong Wang, Yifan Wu, Ruslan Salakhutdinov, Sham M. Kakade:
Instabilities of Offline RL with Pre-Trained Neural Representation. ICML 2021: 10948-10960 - [c125]Xiyang Liu, Weihao Kong, Sham M. Kakade, Sewoong Oh:
Robust and differentially private mean estimation. NeurIPS 2021: 3887-3901 - [c124]Difan Zou, Jingfeng Wu, Vladimir Braverman, Quanquan Gu, Dean P. Foster, Sham M. Kakade:
The Benefits of Implicit Regularization from SGD in Least Squares Problems. NeurIPS 2021: 5456-5468 - [c123]Jordan T. Ash, Surbhi Goel, Akshay Krishnamurthy, Sham M. Kakade:
Gone Fishing: Neural Active Learning with Fisher Embeddings. NeurIPS 2021: 8927-8939 - [c122]Baihe Huang, Kaixuan Huang, Sham M. Kakade, Jason D. Lee, Qi Lei, Runzhe Wang, Jiaqi Yang:
Going Beyond Linear RL: Sample Efficient Neural Function Approximation. NeurIPS 2021: 8968-8983 - [c121]Yuanhao Wang, Ruosong Wang, Sham M. Kakade:
An Exponential Lower Bound for Linearly Realizable MDP with Constant Suboptimality Gap. NeurIPS 2021: 9521-9533 - [c120]Aditya Kusupati, Matthew Wallingford, Vivek Ramanujan, Raghav Somani, Jae Sung Park, Krishna Pillutla, Prateek Jain, Sham M. Kakade, Ali Farhadi:
LLC: Accurate, Multi-purpose Learnt Low-dimensional Binary Codes. NeurIPS 2021: 23900-23913 - [c119]Baihe Huang, Kaixuan Huang, Sham M. Kakade, Jason D. Lee, Qi Lei, Runzhe Wang, Jiaqi Yang:
Optimal Gradient-based Algorithms for Non-concave Bandit Optimization. NeurIPS 2021: 29101-29115 - [i110]Xiyang Liu, Weihao Kong, Sham M. Kakade, Sewoong Oh:
Robust and Differentially Private Mean Estimation. CoRR abs/2102.09159 (2021) - [i109]Ruosong Wang, Yifan Wu, Ruslan Salakhutdinov, Sham M. Kakade:
Instabilities of Offline RL with Pre-Trained Neural Representation. CoRR abs/2103.04947 (2021) - [i108]Simon S. Du, Sham M. Kakade, Jason D. Lee, Shachar Lovett, Gaurav Mahajan, Wen Sun, Ruosong Wang:
Bilinear Classes: A Structural Framework for Provable Generalization in RL. CoRR abs/2103.10897 (2021) - [i107]Yuanhao Wang, Ruosong Wang, Sham M. Kakade:
An Exponential Lower Bound for Linearly-Realizable MDPs with Constant Suboptimality Gap. CoRR abs/2103.12690 (2021) - [i106]Difan Zou, Jingfeng Wu, Vladimir Braverman, Quanquan Gu, Sham M. Kakade:
Benign Overfitting of Constant-Stepsize SGD for Linear Regression. CoRR abs/2103.12692 (2021) - [i105]Aditya Kusupati, Matthew Wallingford, Vivek Ramanujan, Raghav Somani, Jae Sung Park, Krishna Pillutla, Prateek Jain, Sham M. Kakade, Ali Farhadi:
LLC: Accurate, Multi-purpose Learnt Low-dimensional Binary Codes. CoRR abs/2106.01487 (2021) - [i104]Jordan T. Ash, Surbhi Goel, Akshay Krishnamurthy, Sham M. Kakade:
Gone Fishing: Neural Active Learning with Fisher Embeddings. CoRR abs/2106.09675 (2021) - [i103]Motoya Ohnishi, Isao Ishikawa, Kendall Lowrey, Masahiro Ikeda, Sham M. Kakade, Yoshinobu Kawahara:
Koopman Spectrum Nonlinear Regulator and Provably Efficient Online Learning. CoRR abs/2106.15775 (2021) - [i102]Kaixuan Huang, Sham M. Kakade, Jason D. Lee, Qi Lei:
A Short Note on the Relationship of Information Gain and Eluder Dimension. CoRR abs/2107.02377 (2021) - [i101]Baihe Huang, Kaixuan Huang, Sham M. Kakade, Jason D. Lee, Qi Lei, Runzhe Wang, Jiaqi Yang:
Optimal Gradient-based Algorithms for Non-concave Bandit Optimization. CoRR abs/2107.04518 (2021) - [i100]Baihe Huang, Kaixuan Huang, Sham M. Kakade, Jason D. Lee, Qi Lei, Runzhe Wang, Jiaqi Yang:
Going Beyond Linear RL: Sample Efficient Neural Function Approximation. CoRR abs/2107.06466 (2021) - [i99]Difan Zou, Jingfeng Wu, Vladimir Braverman, Quanquan Gu, Dean P. Foster, Sham M. Kakade:
The Benefits of Implicit Regularization from SGD in Least Squares Problems. CoRR abs/2108.04552 (2021) - [i98]Yonathan Efroni, Sham M. Kakade, Akshay Krishnamurthy, Cyril Zhang:
Sparsity in Partially Controllable Linear Systems. CoRR abs/2110.06150 (2021) - [i97]Jingfeng Wu, Difan Zou, Vladimir Braverman, Quanquan Gu, Sham M. Kakade:
Last Iterate Risk Bounds of SGD with Decaying Stepsize for Overparameterized Linear Regression. CoRR abs/2110.06198 (2021) - [i96]Benjamin L. Edelman, Surbhi Goel, Sham M. Kakade, Cyril Zhang:
Inductive Biases and Variable Creation in Self-Attention Mechanisms. CoRR abs/2110.10090 (2021) - [i95]Jordan T. Ash, Cyril Zhang, Surbhi Goel, Akshay Krishnamurthy, Sham M. Kakade:
Anti-Concentrated Confidence Bonuses for Scalable Exploration. CoRR abs/2110.11202 (2021) - [i94]Dylan J. Foster, Sham M. Kakade, Jian Qian, Alexander Rakhlin:
The Statistical Complexity of Interactive Decision Making. CoRR abs/2112.13487 (2021) - 2020
- [j24]Justin Chan, Landon P. Cox, Dean P. Foster, Shyam Gollakota, Eric Horvitz, Joseph Jaeger
, Sham M. Kakade, Tadayoshi Kohno, John Langford, Jonathan Larson, Puneet Sharma, Sudheesh Singanamalla
, Jacob E. Sunshine, Stefano Tessaro:
PACT: Privacy-Sensitive Protocols And Mechanisms for Mobile Contact Tracing. IEEE Data Eng. Bull. 43(2): 15-35 (2020) - [j23]Damek Davis, Dmitriy Drusvyatskiy, Sham M. Kakade, Jason D. Lee:
Stochastic Subgradient Method Converges on Tame Functions. Found. Comput. Math. 20(1): 119-154 (2020) - [c118]Naman Agarwal, Sham M. Kakade, Rahul Kidambi, Yin Tat Lee, Praneeth Netrapalli, Aaron Sidford:
Leverage Score Sampling for Faster Accelerated Regression and ERM. ALT 2020: 22-47 - [c117]Elad Hazan, Sham M. Kakade, Karan Singh:
The Nonstochastic Control Problem. ALT 2020: 408-421 - [c116]Alekh Agarwal, Sham M. Kakade, Jason D. Lee, Gaurav Mahajan:
Optimality and Approximation with Policy Gradient Methods in Markov Decision Processes. COLT 2020: 64-66 - [c115]Alekh Agarwal, Sham M. Kakade, Lin F. Yang
:
Model-Based Reinforcement Learning with a Generative Model is Minimax Optimal. COLT 2020: 67-83 - [c114]Simon S. Du, Sham M. Kakade, Ruosong Wang, Lin F. Yang
:
Is a Good Representation Sufficient for Sample Efficient Reinforcement Learning? ICLR 2020 - [c113]Sanjeev Arora, Simon S. Du, Sham M. Kakade, Yuping Luo, Nikunj Saunshi:
Provable Representation Learning for Imitation Learning via Bi-level Optimization. ICML 2020: 367-376 - [c112]Mark Braverman, Xinyi Chen, Sham M. Kakade, Karthik Narasimhan, Cyril Zhang, Yi Zhang:
Calibration, Entropy Rates, and Memory in Language Models. ICML 2020: 1089-1099 - [c111]Weihao Kong, Raghav Somani, Zhao Song, Sham M. Kakade, Sewoong Oh:
Meta-learning for Mixed Linear Regression. ICML 2020: 5394-5404 - [c110]Aditya Kusupati, Vivek Ramanujan, Raghav Somani, Mitchell Wortsman, Prateek Jain, Sham M. Kakade, Ali Farhadi:
Soft Threshold Weight Reparameterization for Learnable Sparsity. ICML 2020: 5544-5555 - [c109]Colin Wei, Sham M. Kakade, Tengyu Ma:
The Implicit and Explicit Regularization Effects of Dropout. ICML 2020: 10181-10192 - [c108]Alekh Agarwal, Mikael Henaff, Sham M. Kakade, Wen Sun:
PC-PG: Policy Cover Directed Exploration for Provable Policy Gradient Learning. NeurIPS 2020 - [c107]Alekh Agarwal, Sham M. Kakade, Akshay Krishnamurthy, Wen Sun:
FLAMBE: Structural Complexity and Representation Learning of Low Rank MDPs. NeurIPS 2020 - [c106]Chi Jin, Sham M. Kakade, Akshay Krishnamurthy, Qinghua Liu:
Sample-Efficient Reinforcement Learning of Undercomplete POMDPs. NeurIPS 2020 - [c105]Sham M. Kakade, Akshay Krishnamurthy, Kendall Lowrey, Motoya Ohnishi, Wen Sun:
Information Theoretic Regret Bounds for Online Nonlinear Control. NeurIPS 2020 - [c104]Weihao Kong, Raghav Somani, Sham M. Kakade, Sewoong Oh:
Robust Meta-learning for Mixed Linear Regression with Small Batches. NeurIPS 2020 - [c103]Ruosong Wang, Simon S. Du, Lin F. Yang
, Sham M. Kakade:
Is Long Horizon RL More Difficult Than Short Horizon RL? NeurIPS 2020 - [c102]Kaiqing Zhang, Sham M. Kakade, Tamer Basar, Lin F. Yang
:
Model-Based Multi-Agent RL in Zero-Sum Markov Games with Near-Optimal Sample Complexity. NeurIPS 2020 - [i93]Aditya Kusupati, Vivek Ramanujan, Raghav Somani
, Mitchell Wortsman, Prateek Jain, Sham M. Kakade, Ali Farhadi:
Soft Threshold Weight Reparameterization for Learnable Sparsity. CoRR abs/2002.03231 (2020) - [i92]Weihao Kong, Raghav Somani
, Zhao Song, Sham M. Kakade, Sewoong Oh:
Meta-learning for mixed linear regression. CoRR abs/2002.08936 (2020) - [i91]Simon S. Du, Wei Hu, Sham M. Kakade, Jason D. Lee, Qi Lei:
Few-Shot Learning via Learning the Representation, Provably. CoRR abs/2002.09434 (2020) - [i90]Sanjeev Arora, Simon S. Du, Sham M. Kakade, Yuping Luo, Nikunj Saunshi:
Provable Representation Learning for Imitation Learning via Bi-level Optimization. CoRR abs/2002.10544 (2020) - [i89]Colin Wei, Sham M. Kakade, Tengyu Ma:
The Implicit and Explicit Regularization Effects of Dropout. CoRR abs/2002.12915 (2020) - [i88]Preetum Nakkiran, Prayaag Venkat, Sham M. Kakade, Tengyu Ma:
Optimal Regularization Can Mitigate Double Descent. CoRR abs/2003.01897 (2020) - [i87]Justin Chan, Dean P. Foster, Shyam Gollakota, Eric Horvitz, Joseph Jaeger, Sham M. Kakade, Tadayoshi Kohno, John Langford, Jonathan Larson, Sudheesh Singanamalla, Jacob E. Sunshine, Stefano Tessaro:
PACT: Privacy Sensitive Protocols and Mechanisms for Mobile Contact Tracing. CoRR abs/2004.03544 (2020) - [i86]Ruosong Wang, Simon S. Du, Lin F. Yang, Sham M. Kakade:
Is Long Horizon Reinforcement Learning More Difficult Than Short Horizon Reinforcement Learning? CoRR abs/2005.00527 (2020) - [i85]Weihao Kong, Raghav Somani
, Sham M. Kakade, Sewoong Oh:
Robust Meta-learning for Mixed Linear Regression with Small Batches. CoRR abs/2006.09702 (2020) - [i84]Alekh Agarwal, Sham M. Kakade, Akshay Krishnamurthy, Wen Sun:
FLAMBE: Structural Complexity and Representation Learning of Low Rank MDPs. CoRR abs/2006.10814 (2020) - [i83]Sham M. Kakade, Akshay Krishnamurthy, Kendall Lowrey, Motoya Ohnishi, Wen Sun:
Information Theoretic Regret Bounds for Online Nonlinear Control. CoRR abs/2006.12466 (2020) - [i82]Chi Jin, Sham M. Kakade, Akshay Krishnamurthy, Qinghua Liu:
Sample-Efficient Reinforcement Learning of Undercomplete POMDPs. CoRR abs/2006.12484 (2020) - [i81]Kaiqing Zhang, Sham M. Kakade, Tamer Basar, Lin F. Yang:
Model-Based Multi-Agent RL in Zero-Sum Markov Games with Near-Optimal Sample Complexity. CoRR abs/2007.07461 (2020) - [i80]Alekh Agarwal, Mikael Henaff, Sham M. Kakade, Wen Sun:
PC-PG: Policy Cover Directed Exploration for Provable Policy Gradient Learning. CoRR abs/2007.08459 (2020) - [i79]Yu Bai, Minshuo Chen, Pan Zhou, Tuo Zhao, Jason D. Lee, Sham M. Kakade, Huan Wang, Caiming Xiong:
How Important is the Train-Validation Split in Meta-Learning? CoRR abs/2010.05843 (2020) - [i78]Ruosong Wang, Dean P. Foster, Sham M. Kakade:
What are the Statistical Limits of Offline RL with Linear Function Approximation? CoRR abs/2010.11895 (2020)
2010 – 2019
- 2019
- [c101]Rong Ge, Prateek Jain, Sham M. Kakade, Rahul Kidambi, Dheeraj M. Nagaraj, Praneeth Netrapalli:
Open Problem: Do Good Algorithms Necessarily Query Bad Points? COLT 2019: 3190-3193 - [c100]Kendall Lowrey, Aravind Rajeswaran, Sham M. Kakade, Emanuel Todorov, Igor Mordatch:
Plan Online, Learn Offline: Efficient Learning and Exploration via Model-Based Control. ICLR (Poster) 2019 - [c99]Naman Agarwal, Brian Bullins, Elad Hazan, Sham M. Kakade, Karan Singh:
Online Control with Adversarial Disturbances. ICML 2019: 111-119 - [c98]Chelsea Finn, Aravind Rajeswaran, Sham M. Kakade, Sergey Levine:
Online Meta-Learning. ICML 2019: 1920-1930 - [c97]Elad Hazan, Sham M. Kakade, Karan Singh, Abby Van Soest:
Provably Efficient Maximum Entropy Exploration. ICML 2019: 2681-2691 - [c96]Ramya Korlakai Vinayak, Weihao Kong, Gregory Valiant, Sham M. Kakade:
Maximum Likelihood Estimation for Learning Populations of Parameters. ICML 2019: 6448-6457 - [c95]John Thickstun, Zaïd Harchaoui, Dean P. Foster, Sham M. Kakade:
Coupled Recurrent Models for Polyphonic Music Composition. ISMIR 2019: 311-318 - [c94]Aravind Rajeswaran, Chelsea Finn, Sham M. Kakade, Sergey Levine:
Meta-Learning with Implicit Gradients. NeurIPS 2019: 113-124 - [c93]Rong Ge, Sham M. Kakade, Rahul Kidambi, Praneeth Netrapalli:
The Step Decay Schedule: A Near Optimal, Geometrically Decaying Learning Rate Procedure For Least Squares. NeurIPS 2019: 14951-14962 - [c92]Gabriel Cadamuro, Ramya Korlakai Vinayak, Joshua Blumenstock, Sham M. Kakade, Jacob Shapiro
:
The Illusion of Change: Correcting for Biases in Change Inference for Sparse, Societal-Scale Data. WWW 2019: 2608-2615 - [i77]Venkata Krishna Pillutla, Vincent Roulet, Sham M. Kakade, Zaïd Harchaoui:
A Smoother Way to Train Structured Prediction Models. CoRR abs/1902.03228 (2019) - [i76]Chi Jin, Praneeth Netrapalli, Rong Ge, Sham M. Kakade, Michael I. Jordan:
A Short Note on Concentration Inequalities for Random Vectors with SubGaussian Norm. CoRR abs/1902.03736 (2019) - [i75]Ramya Korlakai Vinayak, Weihao Kong, Gregory Valiant, Sham M. Kakade:
Maximum Likelihood Estimation for Learning Populations of Parameters. CoRR abs/1902.04553 (2019) - [i74]Chi Jin, Praneeth Netrapalli, Rong Ge, Sham M. Kakade, Michael I. Jordan:
Stochastic Gradient Descent Escapes Saddle Points Efficiently. CoRR abs/1902.04811 (2019) - [i73]Chelsea Finn, Aravind Rajeswaran, Sham M. Kakade, Sergey Levine:
Online Meta-Learning. CoRR abs/1902.08438 (2019) - [i72]Naman Agarwal, Brian Bullins, Elad Hazan, Sham M. Kakade, Karan Singh:
Online Control with Adversarial Disturbances. CoRR abs/1902.08721 (2019) - [i71]Rong Ge, Sham M. Kakade, Rahul Kidambi, Praneeth Netrapalli:
The Step Decay Schedule: A Near Optimal, Geometrically Decaying Learning Rate Procedure. CoRR abs/1904.12838 (2019) - [i70]Alekh Agarwal, Sham M. Kakade, Lin F. Yang:
On the Optimality of Sparse Model-Based Planning for Markov Decision Processes. CoRR abs/1906.03804 (2019) - [i69]Mark Braverman, Xinyi Chen, Sham M. Kakade, Karthik Narasimhan, Cyril Zhang, Yi Zhang:
Calibration, Entropy Rates, and Memory in Language Models. CoRR abs/1906.05664 (2019) - [i68]Alekh Agarwal, Sham M. Kakade, Jason D. Lee, Gaurav Mahajan:
Optimality and Approximation with Policy Gradient Methods in Markov Decision Processes. CoRR abs/1908.00261 (2019) - [i67]Aravind Rajeswaran, Chelsea Finn, Sham M. Kakade, Sergey Levine:
Meta-Learning with Implicit Gradients. CoRR abs/1909.04630 (2019) - [i66]Simon S. Du, Sham M. Kakade, Ruosong Wang, Lin F. Yang:
Is a Good Representation Sufficient for Sample Efficient Reinforcement Learning? CoRR abs/1910.03016 (2019) - [i65]Elad Hazan, Sham M. Kakade, Karan Singh:
The Nonstochastic Control Problem. CoRR abs/1911.12178 (2019) - [i64]Ramya Korlakai Vinayak, Weihao Kong, Sham M. Kakade:
Optimal Estimation of Change in a Population of Parameters. CoRR abs/1911.12568 (2019) - [i63]Venkata Krishna Pillutla, Sham M. Kakade, Zaïd Harchaoui:
Robust Aggregation for Federated Learning. CoRR abs/1912.13445 (2019) - 2018
- [c91]Prateek Jain, Sham M. Kakade, Rahul Kidambi, Praneeth Netrapalli, Aaron Sidford:
Accelerating Stochastic Gradient Descent for Least Squares Regression. COLT 2018: 545-604 - [c90]John Thickstun, Zaïd Harchaoui, Dean P. Foster, Sham M. Kakade:
Invariances and Data Augmentation for Supervised Music Transcription. ICASSP 2018: 2241-2245 - [c89]Rahul Kidambi, Praneeth Netrapalli, Prateek Jain, Sham M. Kakade:
On the insufficiency of existing momentum schemes for Stochastic Optimization. ICLR 2018 - [c88]Cathy Wu, Aravind Rajeswaran, Yan Duan, Vikash Kumar, Alexandre M. Bayen, Sham M. Kakade, Igor Mordatch, Pieter Abbeel:
Variance Reduction for Policy Gradient with Action-Dependent Factorized Baselines. ICLR 2018 - [c87]Maryam Fazel, Rong Ge, Sham M. Kakade, Mehran Mesbahi:
Global Convergence of Policy Gradient Methods for the Linear Quadratic Regulator. ICML 2018: 1466-1475 - [c86]Qingqing Huang, Sham M. Kakade, Weihao Kong, Gregory Valiant:
Recovering Structured Probability Matrices. ITCS 2018: 46:1-46:14 - [c85]Rahul Kidambi, Praneeth Netrapalli, Prateek Jain, Sham M. Kakade:
On the Insufficiency of Existing Momentum Schemes for Stochastic Optimization. ITA 2018: 1-9 - [c84]Venkata Krishna Pillutla, Vincent Roulet, Sham M. Kakade, Zaïd Harchaoui:
A Smoother Way to Train Structured Prediction Models. NeurIPS 2018: 4771-4783 - [c83]Sham M. Kakade, Jason D. Lee:
Provably Correct Automatic Sub-Differentiation for Qualified Programs. NeurIPS 2018: 7125-7135 - [c82]Vatsal Sharan, Sham M. Kakade, Percy Liang, Gregory Valiant:
Prediction with a short memory. STOC 2018: 1074-1087 - [i62]Maryam Fazel, Rong Ge, Sham M. Kakade, Mehran Mesbahi:
Global Convergence of Policy Gradient Methods for Linearized Control Problems. CoRR abs/1801.05039 (2018) - [i61]Rahul Kidambi, Praneeth Netrapalli, Prateek Jain, Sham M. Kakade:
On the insufficiency of existing momentum schemes for Stochastic Optimization. CoRR abs/1803.05591 (2018) - [i60]Cathy Wu, Aravind Rajeswaran, Yan Duan, Vikash Kumar, Alexandre M. Bayen, Sham M. Kakade, Igor Mordatch, Pieter Abbeel:
Variance Reduction for Policy Gradient with Action-Dependent Factorized Baselines. CoRR abs/1803.07246 (2018) - [i59]Damek Davis, Dmitriy Drusvyatskiy, Sham M. Kakade, Jason D. Lee:
Stochastic subgradient method converges on tame functions. CoRR abs/1804.07795 (2018) - [i58]Sham M. Kakade, Jason D. Lee:
Provably Correct Automatic Subdifferentiation for Qualified Programs. CoRR abs/1809.08530 (2018) - [i57]Kendall Lowrey, Aravind Rajeswaran, Sham M. Kakade, Emanuel Todorov, Igor Mordatch:
Plan Online, Learn Offline: Efficient Learning and Exploration via Model-Based Control. CoRR abs/1811.01848 (2018) - [i56]John Thickstun, Zaïd Harchaoui, Dean P. Foster, Sham M. Kakade:
Coupled Recurrent Models for Polyphonic Music Composition. CoRR abs/1811.08045 (2018) - [i55]Elad Hazan, Sham M. Kakade, Karan Singh, Abby Van Soest:
Provably Efficient Maximum Entropy Exploration. CoRR abs/1812.02690 (2018) - 2017
- [j22]Prateek Jain, Sham M. Kakade, Rahul Kidambi, Praneeth Netrapalli, Aaron Sidford:
Parallelizing Stochastic Gradient Descent for Least Squares Regression: Mini-batching, Averaging, and Model Misspecification. J. Mach. Learn. Res. 18: 223:1-223:42 (2017) - [c81]Prateek Jain, Chi Jin, Sham M. Kakade, Praneeth Netrapalli:
Global Convergence of Non-Convex Gradient Descent for Computing Matrix Squareroot. AISTATS 2017: 479-488 - [c80]Prateek Jain, Sham M. Kakade, Rahul Kidambi, Praneeth Netrapalli, Venkata Krishna Pillutla, Aaron Sidford:
A Markov Chain Theory Approach to Characterizing the Minimax Optimality of Stochastic Gradient Descent (for Least Squares). FSTTCS 2017: 2:1-2:10 - [c79]John Thickstun, Zaïd Harchaoui, Sham M. Kakade:
Learning Features of Music From Scratch. ICLR (Poster) 2017 - [c78]Chi Jin, Rong Ge, Praneeth Netrapalli, Sham M. Kakade, Michael I. Jordan:
How to Escape Saddle Points Efficiently. ICML 2017: 1724-1732 - [c77]Vatsal Sharan, Sham M. Kakade, Percy Liang, Gregory Valiant:
Learning Overcomplete HMMs. NIPS 2017: 940-949 - [c76]Aravind Rajeswaran, Kendall Lowrey, Emanuel Todorov, Sham M. Kakade:
Towards Generalization and Simplicity in Continuous Control. NIPS 2017: 6550-6561 - [i54]Chi Jin, Rong Ge, Praneeth Netrapalli, Sham M. Kakade, Michael I. Jordan:
How to Escape Saddle Points Efficiently. CoRR abs/1703.00887 (2017) - [i53]Aravind Rajeswaran, Kendall Lowrey, Emanuel Todorov, Sham M. Kakade:
Towards Generalization and Simplicity in Continuous Control. CoRR abs/1703.02660 (2017) - [i52]Prateek Jain, Sham M. Kakade, Rahul Kidambi, Praneeth Netrapalli, Aaron Sidford:
Accelerating Stochastic Gradient Descent. CoRR abs/1704.08227 (2017) - [i51]Prateek Jain, Sham M. Kakade, Rahul Kidambi, Praneeth Netrapalli, Venkata Krishna Pillutla, Aaron Sidford:
A Markov Chain Theory Approach to Characterizing the Minimax Optimality of Stochastic Gradient Descent (for Least Squares). CoRR abs/1710.09430 (2017) - [i50]Vatsal Sharan, Sham M. Kakade, Percy Liang, Gregory Valiant:
Learning Overcomplete HMMs. CoRR abs/1711.02309 (2017) - [i49]John Thickstun, Zaïd Harchaoui, Dean P. Foster, Sham M. Kakade:
Invariances and Data Augmentation for Supervised Music Transcription. CoRR abs/1711.04845 (2017) - [i48]Naman Agarwal, Sham M. Kakade, Rahul Kidambi, Yin Tat Lee, Praneeth Netrapalli, Aaron Sidford:
Leverage Score Sampling for Faster Accelerated Regression and ERM. CoRR abs/1711.08426 (2017) - 2016
- [j21]Qingqing Huang, Rong Ge, Sham M. Kakade, Munther A. Dahleh:
Minimal Realization Problems for Hidden Markov Models. IEEE Trans. Signal Process. 64(7): 1896-1904 (2016) - [c75]Prateek Jain, Chi Jin, Sham M. Kakade, Praneeth Netrapalli, Aaron Sidford:
Streaming PCA: Matching Matrix Bernstein and Near-Optimal Finite Sample Guarantees for Oja's Algorithm. COLT 2016: 1147-1164 - [c74]Dan Garber, Elad Hazan, Chi Jin, Sham M. Kakade, Cameron Musco, Praneeth Netrapalli, Aaron Sidford:
Faster Eigenvector Computation via Shift-and-Invert Preconditioning. ICML 2016: 2626-2634 - [c73]Rong Ge, Chi Jin, Sham M. Kakade, Praneeth Netrapalli, Aaron Sidford:
Efficient Algorithms for Large-scale Generalized Eigenvector Computation and Canonical Correlation Analysis. ICML 2016: 2741-2750 - [c72]Chi Jin, Sham M. Kakade, Praneeth Netrapalli:
Provable Efficient Online Matrix Completion via Non-convex Stochastic Gradient Descent. NIPS 2016: 4520-4528 - [i47]Qingqing Huang, Sham M. Kakade, Weihao Kong, Gregory Valiant:
Recovering Structured Probability Matrices. CoRR abs/1602.06586 (2016) - [i46]Prateek Jain, Chi Jin, Sham M. Kakade, Praneeth Netrapalli, Aaron Sidford:
Matching Matrix Bernstein with Little Memory: Near-Optimal Finite Sample Guarantees for Oja's Algorithm. CoRR abs/1602.06929 (2016) - [i45]Rong Ge, Chi Jin, Sham M. Kakade, Praneeth Netrapalli, Aaron Sidford:
Efficient Algorithms for Large-scale Generalized Eigenvector Computation and Canonical Correlation Analysis. CoRR abs/1604.03930 (2016) - [i44]Chi Jin, Sham M. Kakade, Praneeth Netrapalli:
Provable Efficient Online Matrix Completion via Non-convex Stochastic Gradient Descent. CoRR abs/1605.08370 (2016) - [i43]Dan Garber, Elad Hazan, Chi Jin, Sham M. Kakade, Cameron Musco, Praneeth Netrapalli, Aaron Sidford:
Faster Eigenvector Computation via Shift-and-Invert Preconditioning. CoRR abs/1605.08754 (2016) - [i42]Prateek Jain, Sham M. Kakade, Rahul Kidambi, Praneeth Netrapalli, Aaron Sidford:
Parallelizing Stochastic Approximation Through Mini-Batching and Tail-Averaging. CoRR abs/1610.03774 (2016) - [i41]John Thickstun, Zaïd Harchaoui, Sham M. Kakade:
Learning Features of Music from Scratch. CoRR abs/1611.09827 (2016) - [i40]Corinne L. Jones, Sham M. Kakade, Lucas W. Thornblade, David R. Flum, Abraham D. Flaxman:
Canonical Correlation Analysis for Analyzing Sequences of Medical Billing Codes. CoRR abs/1612.00516 (2016) - [i39]Sham M. Kakade, Percy Liang, Vatsal Sharan, Gregory Valiant:
Prediction with a Short Memory. CoRR abs/1612.02526 (2016) - 2015
- [j20]Anima Anandkumar, Dean P. Foster, Daniel J. Hsu, Sham M. Kakade, Yi-Kai Liu:
A Spectral Algorithm for Latent Dirichlet Allocation. Algorithmica 72(1): 193-214 (2015) - [j19]Animashree Anandkumar, Daniel J. Hsu, Majid Janzamin, Sham M. Kakade:
When are overcomplete topic models identifiable? uniqueness of tensor tucker decompositions with structured sparsity. J. Mach. Learn. Res. 16: 2643-2694 (2015) - [c71]Anima Anandkumar, Rong Ge, Daniel J. Hsu, Sham M. Kakade, Matus Telgarsky:
Tensor Decompositions for Learning Latent Variable Models (A Survey for ALT). ALT 2015: 19-38 - [c70]Roy Frostig, Rong Ge, Sham M. Kakade, Aaron Sidford:
Competing with the Empirical Risk Minimizer in a Single Pass. COLT 2015: 728-763 - [c69]David Belanger, Sham M. Kakade:
A Linear Dynamical System Model for Text. ICML 2015: 833-842 - [c68]Roy Frostig, Rong Ge, Sham M. Kakade, Aaron Sidford:
Un-regularizing: approximate proximal point and faster stochastic algorithms for empirical risk minimization. ICML 2015: 2540-2548 - [c67]Kamalika Chaudhuri, Sham M. Kakade, Praneeth Netrapalli, Sujay Sanghavi:
Convergence Rates of Active Learning for Maximum Likelihood Estimation. NIPS 2015: 1090-1098 - [c66]Qingqing Huang, Sham M. Kakade:
Super-Resolution Off the Grid. NIPS 2015: 2665-2673 - [c65]Rong Ge, Qingqing Huang, Sham M. Kakade:
Learning Mixtures of Gaussians in High Dimensions. STOC 2015: 761-770 - [i38]David Belanger, Sham M. Kakade:
A Linear Dynamical System Model for Text. CoRR abs/1502.04081 (2015) - [i37]Rong Ge, Qingqing Huang, Sham M. Kakade:
Learning Mixtures of Gaussians in High Dimensions. CoRR abs/1503.00424 (2015) - [i36]Kamalika Chaudhuri, Sham M. Kakade, Praneeth Netrapalli, Sujay Sanghavi:
Convergence Rates of Active Learning for Maximum Likelihood Estimation. CoRR abs/1506.02348 (2015) - [i35]Roy Frostig, Rong Ge, Sham M. Kakade, Aaron Sidford:
Un-regularizing: approximate proximal point and faster stochastic algorithms for empirical risk minimization. CoRR abs/1506.07512 (2015) - [i34]Prateek Jain, Chi Jin, Sham M. Kakade, Praneeth Netrapalli:
Computing Matrix Squareroot via Non Convex Local Search. CoRR abs/1507.05854 (2015) - [i33]Qingqing Huang, Sham M. Kakade:
Super-Resolution Off the Grid. CoRR abs/1509.07943 (2015) - [i32]Chi Jin, Sham M. Kakade, Cameron Musco, Praneeth Netrapalli, Aaron Sidford:
Robust Shift-and-Invert Preconditioning: Faster and More Sample Efficient Algorithms for Eigenvector Computation. CoRR abs/1510.08896 (2015) - 2014
- [j18]Daniel J. Hsu, Sham M. Kakade, Tong Zhang:
Random Design Analysis of Ridge Regression. Found. Comput. Math. 14(3): 569-600 (2014) - [j17]Animashree Anandkumar, Rong Ge, Daniel J. Hsu, Sham M. Kakade:
A tensor approach to learning mixed membership community models. J. Mach. Learn. Res. 15(1): 2239-2312 (2014) - [j16]Animashree Anandkumar, Rong Ge, Daniel J. Hsu, Sham M. Kakade, Matus Telgarsky:
Tensor decompositions for learning latent variable models. J. Mach. Learn. Res. 15(1): 2773-2832 (2014) - [c64]Qingqing Huang, Rong Ge, Sham M. Kakade, Munther A. Dahleh:
Minimal realization problem for Hidden Markov Models. Allerton 2014: 4-11 - [c63]Alekh Agarwal, Sham M. Kakade, Nikos Karampatziakis, Le Song, Gregory Valiant:
Least Squares Revisited: Scalable Approaches for Multi-class Prediction. ICML 2014: 541-549 - [i31]Qingqing Huang, Rong Ge, Sham M. Kakade, Munther A. Dahleh:
Minimal Realization Problems for Hidden Markov Models. CoRR abs/1411.3698 (2014) - [i30]Roy Frostig, Rong Ge, Sham M. Kakade, Aaron Sidford:
Competing with the Empirical Risk Minimizer in a Single Pass. CoRR abs/1412.6606 (2014) - 2013
- [j15]Sham M. Kakade, Ilan Lobel, Hamid Nazerzadeh:
Optimal Dynamic Mechanism Design and the Virtual-Pivot Mechanism. Oper. Res. 61(4): 837-854 (2013) - [j14]Paramveer S. Dhillon, Dean P. Foster, Sham M. Kakade, Lyle H. Ungar:
A risk comparison of ordinary least squares vs ridge regression. J. Mach. Learn. Res. 14(1): 1505-1511 (2013) - [j13]Alekh Agarwal, Dean P. Foster, Daniel J. Hsu, Sham M. Kakade, Alexander Rakhlin:
Stochastic Convex Optimization with Bandit Feedback. SIAM J. Optim. 23(1): 213-240 (2013) - [c62]Animashree Anandkumar, Rong Ge, Daniel J. Hsu, Sham M. Kakade:
A Tensor Spectral Approach to Learning Mixed Membership Community Models. COLT 2013: 867-881 - [c61]Animashree Anandkumar, Daniel J. Hsu, Adel Javanmard, Sham M. Kakade:
Learning Linear Bayesian Networks with Latent Variables. ICML (1) 2013: 249-257 - [c60]Daniel J. Hsu, Sham M. Kakade:
Learning mixtures of spherical gaussians: moment methods and spectral decompositions. ITCS 2013: 11-20 - [c59]Anima Anandkumar, Daniel J. Hsu, Majid Janzamin, Sham M. Kakade:
When are Overcomplete Topic Models Identifiable? Uniqueness of Tensor Tucker Decompositions with Structured Sparsity. NIPS 2013: 1986-1994 - [i29]Anima Anandkumar, Rong Ge, Daniel J. Hsu, Sham M. Kakade:
A Tensor Spectral Approach to Learning Mixed Membership Community Models. CoRR abs/1302.2684 (2013) - [i28]Animashree Anandkumar, Daniel J. Hsu, Majid Janzamin, Sham M. Kakade:
When are Overcomplete Topic Models Identifiable? Uniqueness of Tensor Tucker Decompositions with Structured Sparsity. CoRR abs/1308.2853 (2013) - [i27]Alekh Agarwal, Sham M. Kakade, Nikos Karampatziakis, Le Song, Gregory Valiant:
Least Squares Revisited: Scalable Approaches for Multi-class Prediction. CoRR abs/1310.1949 (2013) - 2012
- [j12]Daniel J. Hsu, Sham M. Kakade, Tong Zhang:
A spectral algorithm for learning Hidden Markov Models. J. Comput. Syst. Sci. 78(5): 1460-1480 (2012) - [j11]Sham M. Kakade, Shai Shalev-Shwartz, Ambuj Tewari:
Regularization Techniques for Learning with Matrices. J. Mach. Learn. Res. 13: 1865-1890 (2012) - [j10]Niranjan Srinivas, Andreas Krause
, Sham M. Kakade, Matthias W. Seeger:
Information-Theoretic Regret Bounds for Gaussian Process Optimization in the Bandit Setting. IEEE Trans. Inf. Theory 58(5): 3250-3265 (2012) - [c58]Anima Anandkumar, Dean P. Foster, Daniel J. Hsu, Sham M. Kakade, Yi-Kai Liu:
A Spectral Algorithm for Latent Dirichlet Allocation. NIPS 2012: 926-934 - [c57]Animashree Anandkumar, Daniel J. Hsu, Furong Huang, Sham M. Kakade:
Learning Mixtures of Tree Graphical Models. NIPS 2012: 1061-1069 - [c56]Daniel J. Hsu, Sham M. Kakade, Percy Liang:
Identifiability and Unmixing of Latent Parse Trees. NIPS 2012: 1520-1528 - [c55]Elad Hazan, Sham M. Kakade:
(weak) Calibration is Computationally Hard. COLT 2012: 3.1-3.10 - [c54]Daniel J. Hsu, Sham M. Kakade, Tong Zhang:
Random Design Analysis of Ridge Regression. COLT 2012: 9.1-9.24 - [c53]Animashree Anandkumar, Daniel J. Hsu, Sham M. Kakade:
A Method of Moments for Mixture Models and Hidden Markov Models. COLT 2012: 33.1-33.34 - [c52]Sébastien Bubeck, Nicolò Cesa-Bianchi, Sham M. Kakade:
Towards Minimax Policies for Online Linear Optimization with Bandit Feedback. COLT 2012: 41.1-41.14 - [c51]Ruslan Salakhutdinov, Sham M. Kakade, Dean P. Foster:
Domain Adaptation: A Small Sample Statistical Approach. AISTATS 2012: 960-968 - [i26]Sébastien Bubeck, Nicolò Cesa-Bianchi, Sham M. Kakade:
Towards minimax policies for online linear optimization with bandit feedback. CoRR abs/1202.3079 (2012) - [i25]Elad Hazan, Sham M. Kakade:
(weak) Calibration is Computationally Hard. CoRR abs/1202.4478 (2012) - [i24]Animashree Anandkumar, Daniel J. Hsu, Sham M. Kakade:
A Method of Moments for Mixture Models and Hidden Markov Models. CoRR abs/1203.0683 (2012) - [i23]Animashree Anandkumar, Daniel J. Hsu, Sham M. Kakade:
Learning High-Dimensional Mixtures of Graphical Models. CoRR abs/1203.0697 (2012) - [i22]Animashree Anandkumar, Dean P. Foster, Daniel J. Hsu, Sham M. Kakade, Yi-Kai Liu:
Two SVDs Suffice: Spectral decompositions for probabilistic topic modeling and latent Dirichlet allocation. CoRR abs/1204.6703 (2012) - [i21]Daniel J. Hsu, Sham M. Kakade, Percy Liang:
Identifiability and Unmixing of Latent Parse Trees. CoRR abs/1206.3137 (2012) - [i20]Daniel J. Hsu, Sham M. Kakade:
Learning Gaussian Mixture Models: Moment Methods and Spectral Decompositions. CoRR abs/1206.5766 (2012) - [i19]Eyal Even-Dar, Sham M. Kakade, Yishay Mansour:
Planning in POMDPs Using Multiplicity Automata. CoRR abs/1207.1388 (2012) - [i18]Animashree Anandkumar, Daniel J. Hsu, Adel Javanmard, Sham M. Kakade:
Learning Linear Bayesian Networks with Latent Variables. CoRR abs/1209.5350 (2012) - [i17]Anima Anandkumar, Rong Ge, Daniel J. Hsu, Sham M. Kakade, Matus Telgarsky:
Tensor decompositions for learning latent variable models. CoRR abs/1210.7559 (2012) - [i16]Daniel J. Hsu, Sham M. Kakade, Tong Zhang:
Analysis of a randomized approximation scheme for matrix multiplication. CoRR abs/1211.5414 (2012) - 2011
- [j9]Sham M. Kakade, Ilan Lobel, Hamid Nazerzadeh:
Optimal dynamic mechanism design via a virtual VCG mechanism. SIGecom Exch. 10(1): 27-30 (2011) - [j8]Daniel J. Hsu, Sham M. Kakade, Tong Zhang:
Robust Matrix Decomposition With Sparse Corruptions. IEEE Trans. Inf. Theory 57(11): 7221-7234 (2011) - [c50]Sham M. Kakade, Adam Kalai, Varun Kanade, Ohad Shamir:
Efficient Learning of Generalized Linear and Single Index Models with Isotonic Regression. NIPS 2011: 927-935 - [c49]Alekh Agarwal, Dean P. Foster, Daniel J. Hsu, Sham M. Kakade, Alexander Rakhlin:
Stochastic convex optimization with bandit feedback. NIPS 2011: 1035-1043 - [c48]Animashree Anandkumar, Kamalika Chaudhuri, Daniel J. Hsu, Sham M. Kakade, Le Song, Tong Zhang:
Spectral Methods for Learning Multivariate Latent Tree Structure. NIPS 2011: 2025-2033 - [c47]John Blitzer, Sham M. Kakade, Dean P. Foster:
Domain Adaptation with Coupled Subspaces. AISTATS 2011: 173-181 - [c46]Sham M. Kakade, Ulrike von Luxburg:
Preface. COLT 2011 - [e1]Sham M. Kakade, Ulrike von Luxburg:
COLT 2011 - The 24th Annual Conference on Learning Theory, June 9-11, 2011, Budapest, Hungary. JMLR Proceedings 19, JMLR.org 2011 [contents] - [i15]Daniel J. Hsu, Sham M. Kakade, Tong Zhang:
Dimension-free tail inequalities for sums of random matrices. CoRR abs/1104.1672 (2011) - [i14]Sham M. Kakade, Adam Tauman Kalai, Varun Kanade, Ohad Shamir:
Efficient Learning of Generalized Linear and Single Index Models with Isotonic Regression. CoRR abs/1104.2018 (2011) - [i13]Dean P. Foster, Sham M. Kakade, Ruslan Salakhutdinov:
Domain Adaptation: Overfitting and Small Sample Statistics. CoRR abs/1105.0857 (2011) - [i12]Daniel J. Hsu, Sham M. Kakade, Tong Zhang:
An Analysis of Random Design Linear Regression. CoRR abs/1106.2363 (2011) - [i11]Animashree Anandkumar, Kamalika Chaudhuri, Daniel J. Hsu, Sham M. Kakade, Le Song, Tong Zhang:
Spectral Methods for Learning Multivariate Latent Tree Structure. CoRR abs/1107.1283 (2011) - [i10]Alekh Agarwal, Dean P. Foster, Daniel J. Hsu, Sham M. Kakade, Alexander Rakhlin:
Stochastic convex optimization with bandit feedback. CoRR abs/1107.1744 (2011) - [i9]Daniel J. Hsu, Sham M. Kakade, Tong Zhang:
A tail inequality for quadratic forms of subgaussian random vectors. CoRR abs/1110.2842 (2011) - 2010
- [j7]Sham M. Kakade, Ping Li:
Guest editorial: special issue on learning theory. Mach. Learn. 80(2-3): 109-110 (2010) - [c45]Niranjan Srinivas, Andreas Krause, Sham M. Kakade, Matthias W. Seeger:
Gaussian Process Optimization in the Bandit Setting: No Regret and Experimental Design. ICML 2010: 1015-1022 - [c44]Alexander L. Strehl, John Langford, Lihong Li, Sham M. Kakade:
Learning from Logged Implicit Exploration Data. NIPS 2010: 2217-2225 - [c43]Sham M. Kakade, Ohad Shamir, Karthik Sindharan, Ambuj Tewari:
Learning Exponential Families in High-Dimensions: Strong Convexity and Sparsity. AISTATS 2010: 381-388 - [i8]Sham M. Kakade, Ilan Lobel, Hamid Nazerzadeh:
An Optimal Dynamic Mechanism for Multi-Armed Bandit Processes. CoRR abs/1001.4598 (2010) - [i7]Alexander L. Strehl, John Langford, Sham M. Kakade:
Learning from Logged Implicit Exploration Data. CoRR abs/1003.0120 (2010) - [i6]Daniel J. Hsu, Sham M. Kakade, Tong Zhang:
Robust Matrix Decomposition with Outliers. CoRR abs/1011.1518 (2010)
2000 – 2009
- 2009
- [j6]Eyal Even-Dar, Sham M. Kakade, Yishay Mansour:
Online Markov Decision Processes. Math. Oper. Res. 34(3): 726-736 (2009) - [j5]Sham M. Kakade, Adam Tauman Kalai, Katrina Ligett:
Playing Games with Approximation Algorithms. SIAM J. Comput. 39(3): 1088-1106 (2009) - [c42]Daniel J. Hsu, Sham M. Kakade, Tong Zhang:
A Spectral Algorithm for Learning Hidden Markov Models. COLT 2009 - [c41]Kamalika Chaudhuri, Sham M. Kakade, Karen Livescu
, Karthik Sridharan:
Multi-view clustering via canonical correlation analysis. ICML 2009: 129-136 - [c40]Daniel J. Hsu, Sham M. Kakade, John Langford, Tong Zhang:
Multi-Label Prediction via Compressed Sensing. NIPS 2009: 772-780 - [c39]Nikhil R. Devanur, Sham M. Kakade:
The price of truthfulness for pay-per-click auctions. EC 2009: 99-106 - [i5]Daniel J. Hsu, Sham M. Kakade, John Langford, Tong Zhang:
Multi-Label Prediction via Compressed Sensing. CoRR abs/0902.1284 (2009) - [i4]Sham M. Kakade, Shai Shalev-Shwartz, Ambuj Tewari:
Applications of strong convexity--strong smoothness duality to learning with matrices. CoRR abs/0910.0610 (2009) - [i3]Sham M. Kakade, Ohad Shamir, Karthik Sridharan, Ambuj Tewari:
Learning Exponential Families in High-Dimensions: Strong Convexity and Sparsity. CoRR abs/0911.0054 (2009) - [i2]Niranjan Srinivas, Andreas Krause, Sham M. Kakade, Matthias W. Seeger:
Gaussian Process Bandits without Regret: An Experimental Design Approach. CoRR abs/0912.3995 (2009) - 2008
- [j4]Sham M. Kakade, Dean P. Foster:
Deterministic calibration and Nash equilibrium. J. Comput. Syst. Sci. 74(1): 115-130 (2008) - [j3]Matthias W. Seeger, Sham M. Kakade, Dean P. Foster:
Information Consistency of Nonparametric Gaussian Process Methods. IEEE Trans. Inf. Theory 54(5): 2376-2382 (2008) - [c38]Peter L. Bartlett, Varsha Dani, Thomas P. Hayes, Sham M. Kakade, Alexander Rakhlin, Ambuj Tewari:
High-Probability Regret Bounds for Bandit Online Linear Optimization. COLT 2008: 335-342 - [c37]Varsha Dani, Thomas P. Hayes, Sham M. Kakade:
Stochastic Linear Optimization under Bandit Feedback. COLT 2008: 355-366 - [c36]Karthik Sridharan, Sham M. Kakade:
An Information Theoretic Framework for Multi-view Learning. COLT 2008: 403-414 - [c35]Sham M. Kakade, Shai Shalev-Shwartz, Ambuj Tewari:
Efficient bandit algorithms for online multiclass prediction. ICML 2008: 440-447 - [c34]Sham M. Kakade, Karthik Sridharan, Ambuj Tewari:
On the Complexity of Linear Prediction: Risk Bounds, Margin Bounds, and Regularization. NIPS 2008: 793-800 - [c33]Sham M. Kakade, Ambuj Tewari:
On the Generalization Ability of Online Strongly Convex Programming Algorithms. NIPS 2008: 801-808 - [c32]Shai Shalev-Shwartz, Sham M. Kakade:
Mind the Duality Gap: Logarithmic regret algorithms for online optimization. NIPS 2008: 1457-1464 - [i1]Daniel J. Hsu, Sham M. Kakade, Tong Zhang:
A Spectral Algorithm for Learning Hidden Markov Models. CoRR abs/0811.4413 (2008) - 2007
- [c31]Sham M. Kakade, Dean P. Foster:
Multi-view Regression Via Canonical Correlation Analysis. COLT 2007: 82-96 - [c30]Deva Ramanan
, Simon Baker, Sham M. Kakade:
Leveragingarchivalvideo for building face datasets. ICCV 2007: 1-8 - [c29]Eyal Even-Dar, Sham M. Kakade, Yishay Mansour:
The Value of Observation for Monitoring Dynamic Systems. IJCAI 2007: 2474-2479 - [c28]Varsha Dani, Thomas P. Hayes, Sham M. Kakade:
The Price of Bandit Information for Online Optimization. NIPS 2007: 345-352 - [c27]Sham M. Kakade, Adam Tauman Kalai, Katrina Ligett
:
Playing games with approximation algorithms. STOC 2007: 546-555 - [c26]Luis E. Ortiz, Robert E. Schapire, Sham M. Kakade:
Maximum Entropy Correlated Equilibria. AISTATS 2007: 347-354 - 2006
- [c25]Alina Beygelzimer, Sham M. Kakade, John Langford:
Cover trees for nearest neighbor. ICML 2006: 97-104 - [c24]Dean P. Foster, Sham M. Kakade:
Calibration via Regression. ITW 2006: 82-86 - [c23]Eyal Even-Dar, Sham M. Kakade, Michael J. Kearns, Yishay Mansour:
(In)Stability properties of limit order dynamics. EC 2006: 120-129 - 2005
- [c22]Sham M. Kakade, Michael J. Kearns:
Trading in Markovian Price Models. COLT 2005: 606-620 - [c21]Eyal Even-Dar, Sham M. Kakade, Yishay Mansour:
Reinforcement Learning in POMDPs Without Resets. IJCAI 2005: 690-695 - [c20]Sham M. Kakade, Adam Kalai:
From Batch to Transductive Online Learning. NIPS 2005: 611-618 - [c19]Sham M. Kakade, Matthias W. Seeger, Dean P. Foster:
Worst-Case Bounds for Gaussian Process Models. NIPS 2005: 619-626 - [c18]Eyal Even-Dar, Sham M. Kakade, Yishay Mansour:
Planning in POMDPs Using Multiplicity Automata. UAI 2005: 185-192 - 2004
- [c17]Sham M. Kakade, Michael J. Kearns, Luis E. Ortiz
:
Graphical Economics. COLT 2004: 17-32 - [c16]Sham M. Kakade, Dean P. Foster:
Deterministic Calibration and Nash Equilibrium. COLT 2004: 33-48 - [c15]Eyal Even-Dar, Sham M. Kakade, Yishay Mansour:
Experts in a Markov Decision Process. NIPS 2004: 401-408 - [c14]Sham M. Kakade, Michael J. Kearns, Luis E. Ortiz, Robin Pemantle, Siddharth Suri:
Economic Properties of Social Networks. NIPS 2004: 633-640 - [c13]Sham M. Kakade, Andrew Y. Ng:
Online Bounds for Bayesian Algorithms. NIPS 2004: 641-648 - [c12]Sham M. Kakade, Michael J. Kearns, Yishay Mansour, Luis E. Ortiz
:
Competitive algorithms for VWAP and limit order trading. EC 2004: 189-198 - 2003
- [c11]Sham M. Kakade, Michael J. Kearns, John Langford:
Exploration in Metric State Spaces. ICML 2003: 306-312 - [c10]J. Andrew Bagnell, Sham M. Kakade, Andrew Y. Ng, Jeff G. Schneider:
Policy Search by Dynamic Programming. NIPS 2003: 831-838 - [c9]Sham M. Kakade, Michael J. Kearns, John Langford, Luis E. Ortiz
:
Correlated equilibria in graphical games. EC 2003: 42-47 - 2002
- [j2]Sham M. Kakade, Peter Dayan
:
Dopamine: generalization and bonuses. Neural Networks 15(4-6): 549-559 (2002) - [j1]Nathaniel D. Daw
, Sham M. Kakade, Peter Dayan
:
Opponent interactions between serotonin and dopamine. Neural Networks 15(4-6): 603-616 (2002) - [c8]Sham M. Kakade, John Langford:
Approximately Optimal Approximate Reinforcement Learning. ICML 2002: 267-274 - [c7]Sham M. Kakade, Yee Whye Teh, Sam T. Roweis:
An Alternate Objective Function for Markovian Fields. ICML 2002: 275-282 - [c6]John Langford, Martin Zinkevich, Sham M. Kakade:
Competitive Analysis of the Explore/Exploit Tradeoff. ICML 2002: 339-346 - 2001
- [c5]Sham M. Kakade:
Optimizing Average Reward Using Discounted Rewards. COLT/EuroCOLT 2001: 605-615 - [c4]Sham M. Kakade:
A Natural Policy Gradient. NIPS 2001: 1531-1538 - 2000
- [c3]Sham M. Kakade, Peter Dayan:
Dopamine Bonuses. NIPS 2000: 131-137 - [c2]Peter Dayan, Sham M. Kakade:
Explaining Away in Weight Space. NIPS 2000: 451-457
1990 – 1999
- 1999
- [c1]Sham M. Kakade, Peter Dayan:
Acquisition in Autoshaping. NIPS 1999: 24-30
Coauthor Index
aka: Animashree Anandkumar
aka: Simon Shaolei Du
aka: Daniel J. Hsu

manage site settings
To protect your privacy, all features that rely on external API calls from your browser are turned off by default. You need to opt-in for them to become active. All settings here will be stored as cookies with your web browser. For more information see our F.A.Q.
Unpaywalled article links
Add open access links from to the list of external document links (if available).
Privacy notice: By enabling the option above, your browser will contact the API of unpaywall.org to load hyperlinks to open access articles. Although we do not have any reason to believe that your call will be tracked, we do not have any control over how the remote server uses your data. So please proceed with care and consider checking the Unpaywall privacy policy.
Archived links via Wayback Machine
For web page which are no longer available, try to retrieve content from the of the Internet Archive (if available).
Privacy notice: By enabling the option above, your browser will contact the API of archive.org to check for archived content of web pages that are no longer available. Although we do not have any reason to believe that your call will be tracked, we do not have any control over how the remote server uses your data. So please proceed with care and consider checking the Internet Archive privacy policy.
Reference lists
Add a list of references from ,
, and
to record detail pages.
load references from crossref.org and opencitations.net
Privacy notice: By enabling the option above, your browser will contact the APIs of crossref.org, opencitations.net, and semanticscholar.org to load article reference information. Although we do not have any reason to believe that your call will be tracked, we do not have any control over how the remote server uses your data. So please proceed with care and consider checking the Crossref privacy policy and the OpenCitations privacy policy, as well as the AI2 Privacy Policy covering Semantic Scholar.
Citation data
Add a list of citing articles from and
to record detail pages.
load citations from opencitations.net
Privacy notice: By enabling the option above, your browser will contact the API of opencitations.net and semanticscholar.org to load citation information. Although we do not have any reason to believe that your call will be tracked, we do not have any control over how the remote server uses your data. So please proceed with care and consider checking the OpenCitations privacy policy as well as the AI2 Privacy Policy covering Semantic Scholar.
OpenAlex data
Load additional information about publications from .
Privacy notice: By enabling the option above, your browser will contact the API of openalex.org to load additional information. Although we do not have any reason to believe that your call will be tracked, we do not have any control over how the remote server uses your data. So please proceed with care and consider checking the information given by OpenAlex.
last updated on 2024-12-01 00:17 CET by the dblp team
all metadata released as open data under CC0 1.0 license
see also: Terms of Use | Privacy Policy | Imprint