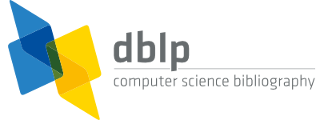


default search action
H. Brendan McMahan
Person information
- affiliation: Google
SPARQL queries 
Refine list

refinements active!
zoomed in on ?? of ?? records
view refined list in
export refined list as
2020 – today
- 2024
- [c51]Krishnamurthy Dj Dvijotham, H. Brendan McMahan, Krishna Pillutla, Thomas Steinke, Abhradeep Thakurta:
Efficient and Near-Optimal Noise Generation for Streaming Differential Privacy. FOCS 2024: 2306-2317 - [c50]Galen Andrew, Peter Kairouz, Sewoong Oh, Alina Oprea, Hugh Brendan McMahan, Vinith Menon Suriyakumar:
One-shot Empirical Privacy Estimation for Federated Learning. ICLR 2024 - [i58]Hubert Eichner, Daniel Ramage, Kallista A. Bonawitz, Dzmitry Huba, Tiziano Santoro, Brett McLarnon, Timon Van Overveldt, Nova Fallen, Peter Kairouz, Albert Cheu, Katharine Daly, Adrià Gascón, Marco Gruteser, Brendan McMahan:
Confidential Federated Computations. CoRR abs/2404.10764 (2024) - [i57]Krishnamurthy Dvijotham, H. Brendan McMahan, Krishna Pillutla, Thomas Steinke, Abhradeep Thakurta:
Efficient and Near-Optimal Noise Generation for Streaming Differential Privacy. CoRR abs/2404.16706 (2024) - [i56]Zachary Charles, Arun Ganesh, Ryan McKenna, H. Brendan McMahan, Nicole Mitchell, Krishna Pillutla, Keith Rush:
Fine-Tuning Large Language Models with User-Level Differential Privacy. CoRR abs/2407.07737 (2024) - [i55]H. Brendan McMahan, Zheng Xu, Yanxiang Zhang:
A Hassle-free Algorithm for Private Learning in Practice: Don't Use Tree Aggregation, Use BLTs. CoRR abs/2408.08868 (2024) - [i54]Katharine Daly, Hubert Eichner, Peter Kairouz, H. Brendan McMahan, Daniel Ramage, Zheng Xu:
Federated Learning in Practice: Reflections and Projections. CoRR abs/2410.08892 (2024) - 2023
- [j6]Natalia Ponomareva, Hussein Hazimeh, Alex Kurakin, Zheng Xu, Carson Denison, H. Brendan McMahan, Sergei Vassilvitskii, Steve Chien, Abhradeep Guha Thakurta:
How to DP-fy ML: A Practical Guide to Machine Learning with Differential Privacy. J. Artif. Intell. Res. 77: 1113-1201 (2023) - [c49]Zheng Xu, Yanxiang Zhang, Galen Andrew, Christopher A. Choquette-Choo, Peter Kairouz, H. Brendan McMahan, Jesse Rosenstock, Yuanbo Zhang:
Federated Learning of Gboard Language Models with Differential Privacy. ACL (industry) 2023: 629-639 - [c48]Zheng Xu, Maxwell D. Collins, Yuxiao Wang, Liviu Panait, Sewoong Oh, Sean Augenstein, Ting Liu, Florian Schroff, H. Brendan McMahan:
Learning to Generate Image Embeddings with User-Level Differential Privacy. CVPR 2023: 7969-7980 - [c47]Tian Li, Manzil Zaheer, Ken Liu, Sashank J. Reddi, Hugh Brendan McMahan, Virginia Smith:
Differentially Private Adaptive Optimization with Delayed Preconditioners. ICLR 2023 - [c46]Christopher A. Choquette-Choo, Hugh Brendan McMahan, J. Keith Rush, Abhradeep Guha Thakurta:
Multi-Epoch Matrix Factorization Mechanisms for Private Machine Learning. ICML 2023: 5924-5963 - [c45]Natalia Ponomareva
, Sergei Vassilvitskii
, Zheng Xu
, Brendan McMahan
, Alexey Kurakin
, Chiyaun Zhang
:
How to DP-fy ML: A Practical Tutorial to Machine Learning with Differential Privacy. KDD 2023: 5823-5824 - [c44]Christopher A. Choquette-Choo, Arun Ganesh, Ryan McKenna, H. Brendan McMahan, John Rush, Abhradeep Guha Thakurta, Zheng Xu:
(Amplified) Banded Matrix Factorization: A unified approach to private training. NeurIPS 2023 - [c43]Anastasia Koloskova, Ryan McKenna, Zachary Charles, John Keith Rush, H. Brendan McMahan:
Gradient Descent with Linearly Correlated Noise: Theory and Applications to Differential Privacy. NeurIPS 2023 - [c42]Krishna Pillutla, Galen Andrew, Peter Kairouz, H. Brendan McMahan, Alina Oprea, Sewoong Oh:
Unleashing the Power of Randomization in Auditing Differentially Private ML. NeurIPS 2023 - [i53]Anastasia Koloskova, Ryan McKenna, Zachary Charles, Keith Rush, Brendan McMahan:
Convergence of Gradient Descent with Linearly Correlated Noise and Applications to Differentially Private Learning. CoRR abs/2302.01463 (2023) - [i52]Galen Andrew, Peter Kairouz, Sewoong Oh, Alina Oprea, H. Brendan McMahan, Vinith M. Suriyakumar:
One-shot Empirical Privacy Estimation for Federated Learning. CoRR abs/2302.03098 (2023) - [i51]Natalia Ponomareva, Hussein Hazimeh, Alex Kurakin, Zheng Xu, Carson Denison, H. Brendan McMahan, Sergei Vassilvitskii, Steve Chien, Abhradeep Thakurta:
How to DP-fy ML: A Practical Guide to Machine Learning with Differential Privacy. CoRR abs/2303.00654 (2023) - [i50]Alekh Agarwal, H. Brendan McMahan, Zheng Xu:
An Empirical Evaluation of Federated Contextual Bandit Algorithms. CoRR abs/2303.10218 (2023) - [i49]Boxin Wang, Jacky Yibo Zhang, Yuan Cao, Bo Li, H. Brendan McMahan, Sewoong Oh, Zheng Xu, Manzil Zaheer:
Can Public Large Language Models Help Private Cross-device Federated Learning? CoRR abs/2305.12132 (2023) - [i48]Krishna Pillutla, Galen Andrew, Peter Kairouz, H. Brendan McMahan, Alina Oprea, Sewoong Oh:
Unleashing the Power of Randomization in Auditing Differentially Private ML. CoRR abs/2305.18447 (2023) - [i47]Zheng Xu, Yanxiang Zhang, Galen Andrew, Christopher A. Choquette-Choo, Peter Kairouz, H. Brendan McMahan, Jesse Rosenstock, Yuanbo Zhang:
Federated Learning of Gboard Language Models with Differential Privacy. CoRR abs/2305.18465 (2023) - [i46]Christopher A. Choquette-Choo, Arun Ganesh, Ryan McKenna, H. Brendan McMahan, Keith Rush, Abhradeep Guha Thakurta, Zheng Xu:
(Amplified) Banded Matrix Factorization: A unified approach to private training. CoRR abs/2306.08153 (2023) - 2022
- [j5]Kallista A. Bonawitz, Peter Kairouz, Brendan McMahan, Daniel Ramage:
Federated learning and privacy. Commun. ACM 65(4): 90-97 (2022) - [c41]Sergey Denisov, H. Brendan McMahan, John Rush, Adam D. Smith, Abhradeep Guha Thakurta:
Improved Differential Privacy for SGD via Optimal Private Linear Operators on Adaptive Streams. NeurIPS 2022 - [i45]Brendan McMahan, Keith Rush, Abhradeep Guha Thakurta:
Private Online Prefix Sums via Optimal Matrix Factorizations. CoRR abs/2202.08312 (2022) - [i44]Zachary Charles, Kallista A. Bonawitz, Stanislav Chiknavaryan, Brendan McMahan, Blaise Agüera y Arcas:
Federated Select: A Primitive for Communication- and Memory-Efficient Federated Learning. CoRR abs/2208.09432 (2022) - [i43]Christopher A. Choquette-Choo, H. Brendan McMahan, Keith Rush, Abhradeep Thakurta:
Multi-Epoch Matrix Factorization Mechanisms for Private Machine Learning. CoRR abs/2211.06530 (2022) - [i42]Zheng Xu, Maxwell D. Collins, Yuxiao Wang, Liviu Panait, Sewoong Oh, Sean Augenstein, Ting Liu, Florian Schroff, H. Brendan McMahan:
Learning to Generate Image Embeddings with User-level Differential Privacy. CoRR abs/2211.10844 (2022) - [i41]Tian Li, Manzil Zaheer, Ken Ziyu Liu, Sashank J. Reddi, H. Brendan McMahan, Virginia Smith:
Differentially Private Adaptive Optimization with Delayed Preconditioners. CoRR abs/2212.00309 (2022) - 2021
- [j4]Peter Kairouz, H. Brendan McMahan, Brendan Avent, Aurélien Bellet, Mehdi Bennis
, Arjun Nitin Bhagoji
, Kallista A. Bonawitz, Zachary Charles, Graham Cormode
, Rachel Cummings, Rafael G. L. D'Oliveira, Hubert Eichner, Salim El Rouayheb, David Evans, Josh Gardner, Zachary Garrett, Adrià Gascón, Badih Ghazi, Phillip B. Gibbons, Marco Gruteser, Zaïd Harchaoui, Chaoyang He, Lie He, Zhouyuan Huo, Ben Hutchinson, Justin Hsu, Martin Jaggi, Tara Javidi
, Gauri Joshi, Mikhail Khodak, Jakub Konecný, Aleksandra Korolova, Farinaz Koushanfar, Sanmi Koyejo, Tancrède Lepoint, Yang Liu, Prateek Mittal, Mehryar Mohri, Richard Nock, Ayfer Özgür, Rasmus Pagh
, Hang Qi, Daniel Ramage, Ramesh Raskar, Mariana Raykova, Dawn Song, Weikang Song, Sebastian U. Stich, Ziteng Sun, Ananda Theertha Suresh, Florian Tramèr
, Praneeth Vepakomma
, Jianyu Wang, Li Xiong, Zheng Xu, Qiang Yang, Felix X. Yu, Han Yu
, Sen Zhao:
Advances and Open Problems in Federated Learning. Found. Trends Mach. Learn. 14(1-2): 1-210 (2021) - [j3]Kallista A. Bonawitz, Peter Kairouz, Brendan McMahan, Daniel Ramage:
Federated Learning and Privacy: Building privacy-preserving systems for machine learning and data science on decentralized data. ACM Queue 19(5): 87-114 (2021) - [c40]Sashank J. Reddi, Zachary Charles, Manzil Zaheer, Zachary Garrett, Keith Rush, Jakub Konecný, Sanjiv Kumar, Hugh Brendan McMahan:
Adaptive Federated Optimization. ICLR 2021 - [c39]Peter Kairouz, Brendan McMahan, Shuang Song, Om Thakkar, Abhradeep Thakurta, Zheng Xu:
Practical and Private (Deep) Learning Without Sampling or Shuffling. ICML 2021: 5213-5225 - [c38]Galen Andrew, Om Thakkar, Brendan McMahan, Swaroop Ramaswamy:
Differentially Private Learning with Adaptive Clipping. NeurIPS 2021: 17455-17466 - [i40]Peter Kairouz, Brendan McMahan, Shuang Song, Om Thakkar, Abhradeep Thakurta, Zheng Xu:
Practical and Private (Deep) Learning without Sampling or Shuffling. CoRR abs/2103.00039 (2021) - [i39]Jianyu Wang, Zachary Charles, Zheng Xu, Gauri Joshi, H. Brendan McMahan, Blaise Agüera y Arcas, Maruan Al-Shedivat, Galen Andrew, Salman Avestimehr, Katharine Daly, Deepesh Data, Suhas N. Diggavi, Hubert Eichner, Advait Gadhikar, Zachary Garrett, Antonious M. Girgis, Filip Hanzely, Andrew Hard, Chaoyang He, Samuel Horváth
, Zhouyuan Huo, Alex Ingerman, Martin Jaggi, Tara Javidi, Peter Kairouz, Satyen Kale, Sai Praneeth Karimireddy, Jakub Konecný, Sanmi Koyejo, Tian Li, Luyang Liu, Mehryar Mohri, Hang Qi, Sashank J. Reddi, Peter Richtárik
, Karan Singhal, Virginia Smith, Mahdi Soltanolkotabi, Weikang Song, Ananda Theertha Suresh, Sebastian U. Stich, Ameet Talwalkar, Hongyi Wang, Blake E. Woodworth, Shanshan Wu, Felix X. Yu, Honglin Yuan, Manzil Zaheer, Mi Zhang, Tong Zhang, Chunxiang Zheng, Chen Zhu, Wennan Zhu:
A Field Guide to Federated Optimization. CoRR abs/2107.06917 (2021) - 2020
- [c37]Wennan Zhu, Peter Kairouz, Brendan McMahan, Haicheng Sun, Wei Li:
Federated Heavy Hitters Discovery with Differential Privacy. AISTATS 2020: 3837-3847 - [c36]Sean Augenstein, H. Brendan McMahan, Daniel Ramage, Swaroop Ramaswamy, Peter Kairouz, Mingqing Chen, Rajiv Mathews, Blaise Agüera y Arcas:
Generative Models for Effective ML on Private, Decentralized Datasets. ICLR 2020 - [c35]Blake E. Woodworth, Kumar Kshitij Patel, Sebastian U. Stich, Zhen Dai, Brian Bullins, H. Brendan McMahan, Ohad Shamir, Nathan Srebro:
Is Local SGD Better than Minibatch SGD? ICML 2020: 10334-10343 - [c34]Borja Balle, Peter Kairouz, Brendan McMahan, Om Dipakbhai Thakkar, Abhradeep Thakurta:
Privacy Amplification via Random Check-Ins. NeurIPS 2020 - [i38]Blake E. Woodworth, Kumar Kshitij Patel
, Sebastian U. Stich, Zhen Dai, Brian Bullins, H. Brendan McMahan, Ohad Shamir, Nathan Srebro:
Is Local SGD Better than Minibatch SGD? CoRR abs/2002.07839 (2020) - [i37]Sashank J. Reddi, Zachary Charles, Manzil Zaheer, Zachary Garrett
, Keith Rush, Jakub Konecný, Sanjiv Kumar, H. Brendan McMahan:
Adaptive Federated Optimization. CoRR abs/2003.00295 (2020) - [i36]Borja Balle, Peter Kairouz, H. Brendan McMahan, Om Thakkar, Abhradeep Thakurta:
Privacy Amplification via Random Check-Ins. CoRR abs/2007.06605 (2020) - [i35]Swaroop Ramaswamy, Om Thakkar, Rajiv Mathews, Galen Andrew, H. Brendan McMahan, Françoise Beaufays:
Training Production Language Models without Memorizing User Data. CoRR abs/2009.10031 (2020)
2010 – 2019
- 2019
- [c33]Kallista A. Bonawitz, Fariborz Salehi, Jakub Konecný, Brendan McMahan, Marco Gruteser:
Federated Learning with Autotuned Communication-Efficient Secure Aggregation. ACSSC 2019: 1222-1226 - [c32]Hubert Eichner, Tomer Koren, Brendan McMahan, Nathan Srebro, Kunal Talwar:
Semi-Cyclic Stochastic Gradient Descent. ICML 2019: 1764-1773 - [c31]Kallista A. Bonawitz, Hubert Eichner, Wolfgang Grieskamp, Dzmitry Huba, Alex Ingerman, Vladimir Ivanov, Chloé Kiddon, Jakub Konecný, Stefano Mazzocchi, Brendan McMahan, Timon Van Overveldt, David Petrou, Daniel Ramage, Jason Roselander:
Towards Federated Learning at Scale: System Design. SysML 2019 - [i34]Kallista A. Bonawitz, Hubert Eichner, Wolfgang Grieskamp, Dzmitry Huba, Alex Ingerman
, Vladimir Ivanov, Chloé Kiddon, Jakub Konecný, Stefano Mazzocchi, H. Brendan McMahan, Timon Van Overveldt, David Petrou, Daniel Ramage, Jason Roselander:
Towards Federated Learning at Scale: System Design. CoRR abs/1902.01046 (2019) - [i33]Wennan Zhu, Peter Kairouz, Haicheng Sun, Brendan McMahan, Wei Li:
Federated Heavy Hitters Discovery with Differential Privacy. CoRR abs/1902.08534 (2019) - [i32]Alexander Ratner, Dan Alistarh, Gustavo Alonso, David G. Andersen, Peter Bailis, Sarah Bird, Nicholas Carlini, Bryan Catanzaro, Eric S. Chung, Bill Dally, Jeff Dean, Inderjit S. Dhillon, Alexandros G. Dimakis, Pradeep Dubey, Charles Elkan, Grigori Fursin, Gregory R. Ganger, Lise Getoor, Phillip B. Gibbons, Garth A. Gibson, Joseph E. Gonzalez, Justin Gottschlich, Song Han, Kim M. Hazelwood, Furong Huang, Martin Jaggi, Kevin G. Jamieson, Michael I. Jordan, Gauri Joshi, Rania Khalaf, Jason Knight, Jakub Konecný, Tim Kraska, Arun Kumar, Anastasios Kyrillidis, Jing Li
, Samuel Madden, H. Brendan McMahan, Erik Meijer, Ioannis Mitliagkas, Rajat Monga, Derek Gordon Murray, Dimitris S. Papailiopoulos, Gennady Pekhimenko, Theodoros Rekatsinas, Afshin Rostamizadeh, Christopher Ré, Christopher De Sa, Hanie Sedghi, Siddhartha Sen, Virginia Smith, Alex Smola, Dawn Song, Evan Randall Sparks, Ion Stoica, Vivienne Sze, Madeleine Udell, Joaquin Vanschoren, Shivaram Venkataraman, Rashmi Vinayak, Markus Weimer, Andrew Gordon Wilson, Eric P. Xing, Matei Zaharia, Ce Zhang, Ameet Talwalkar:
SysML: The New Frontier of Machine Learning Systems. CoRR abs/1904.03257 (2019) - [i31]Hubert Eichner, Tomer Koren, H. Brendan McMahan, Nathan Srebro, Kunal Talwar:
Semi-Cyclic Stochastic Gradient Descent. CoRR abs/1904.10120 (2019) - [i30]Om Thakkar, Galen Andrew, H. Brendan McMahan:
Differentially Private Learning with Adaptive Clipping. CoRR abs/1905.03871 (2019) - [i29]Sean Augenstein, H. Brendan McMahan, Daniel Ramage, Swaroop Ramaswamy, Peter Kairouz, Mingqing Chen, Rajiv Mathews, Blaise Agüera y Arcas:
Generative Models for Effective ML on Private, Decentralized Datasets. CoRR abs/1911.06679 (2019) - [i28]Ziteng Sun, Peter Kairouz, Ananda Theertha Suresh, H. Brendan McMahan:
Can You Really Backdoor Federated Learning? CoRR abs/1911.07963 (2019) - [i27]Kallista A. Bonawitz, Fariborz Salehi, Jakub Konecný, Brendan McMahan, Marco Gruteser:
Federated Learning with Autotuned Communication-Efficient Secure Aggregation. CoRR abs/1912.00131 (2019) - [i26]Peter Kairouz, H. Brendan McMahan, Brendan Avent, Aurélien Bellet, Mehdi Bennis, Arjun Nitin Bhagoji, Kallista A. Bonawitz, Zachary Charles, Graham Cormode, Rachel Cummings, Rafael G. L. D'Oliveira, Salim El Rouayheb, David Evans, Josh Gardner, Zachary Garrett
, Adrià Gascón, Badih Ghazi, Phillip B. Gibbons, Marco Gruteser, Zaïd Harchaoui, Chaoyang He, Lie He, Zhouyuan Huo, Ben Hutchinson, Justin Hsu, Martin Jaggi, Tara Javidi, Gauri Joshi, Mikhail Khodak, Jakub Konecný, Aleksandra Korolova, Farinaz Koushanfar, Sanmi Koyejo, Tancrède Lepoint, Yang Liu, Prateek Mittal, Mehryar Mohri, Richard Nock, Ayfer Özgür, Rasmus Pagh, Mariana Raykova, Hang Qi, Daniel Ramage, Ramesh Raskar, Dawn Song, Weikang Song, Sebastian U. Stich, Ziteng Sun, Ananda Theertha Suresh, Florian Tramèr, Praneeth Vepakomma, Jianyu Wang, Li Xiong, Zheng Xu, Qiang Yang, Felix X. Yu, Han Yu, Sen Zhao:
Advances and Open Problems in Federated Learning. CoRR abs/1912.04977 (2019) - 2018
- [c30]H. Brendan McMahan, Daniel Ramage, Kunal Talwar, Li Zhang:
Learning Differentially Private Recurrent Language Models. ICLR (Poster) 2018 - [c29]Naman Agarwal, Ananda Theertha Suresh, Felix X. Yu, Sanjiv Kumar, Brendan McMahan:
cpSGD: Communication-efficient and differentially-private distributed SGD. NeurIPS 2018: 7575-7586 - [c28]Blake E. Woodworth, Jialei Wang, Adam D. Smith, Brendan McMahan, Nati Srebro:
Graph Oracle Models, Lower Bounds, and Gaps for Parallel Stochastic Optimization. NeurIPS 2018: 8505-8515 - [i25]Blake E. Woodworth, Jialei Wang, Brendan McMahan, Nathan Srebro:
Graph Oracle Models, Lower Bounds, and Gaps for Parallel Stochastic Optimization. CoRR abs/1805.10222 (2018) - [i24]Naman Agarwal, Ananda Theertha Suresh, Felix X. Yu, Sanjiv Kumar, H. Brendan McMahan:
cpSGD: Communication-efficient and differentially-private distributed SGD. CoRR abs/1805.10559 (2018) - [i23]Sebastian Caldas, Peter Wu, Tian Li, Jakub Konecný, H. Brendan McMahan, Virginia Smith, Ameet Talwalkar:
LEAF: A Benchmark for Federated Settings. CoRR abs/1812.01097 (2018) - [i22]H. Brendan McMahan, Galen Andrew:
A General Approach to Adding Differential Privacy to Iterative Training Procedures. CoRR abs/1812.06210 (2018) - [i21]Sebastian Caldas, Jakub Konecný, H. Brendan McMahan, Ameet Talwalkar:
Expanding the Reach of Federated Learning by Reducing Client Resource Requirements. CoRR abs/1812.07210 (2018) - 2017
- [j2]H. Brendan McMahan:
A survey of Algorithms and Analysis for Adaptive Online Learning. J. Mach. Learn. Res. 18: 90:1-90:50 (2017) - [c27]Brendan McMahan, Eider Moore, Daniel Ramage, Seth Hampson, Blaise Agüera y Arcas:
Communication-Efficient Learning of Deep Networks from Decentralized Data. AISTATS 2017: 1273-1282 - [c26]Kallista A. Bonawitz, Vladimir Ivanov, Ben Kreuter, Antonio Marcedone, H. Brendan McMahan, Sarvar Patel, Daniel Ramage, Aaron Segal, Karn Seth:
Practical Secure Aggregation for Privacy-Preserving Machine Learning. CCS 2017: 1175-1191 - [c25]Martín Abadi, Úlfar Erlingsson, Ian J. Goodfellow, H. Brendan McMahan, Ilya Mironov
, Nicolas Papernot, Kunal Talwar, Li Zhang:
On the Protection of Private Information in Machine Learning Systems: Two Recent Approches. CSF 2017: 1-6 - [c24]Ananda Theertha Suresh, Felix X. Yu, Sanjiv Kumar, H. Brendan McMahan:
Distributed Mean Estimation with Limited Communication. ICML 2017: 3329-3337 - [i20]Martín Abadi, Úlfar Erlingsson, Ian J. Goodfellow, H. Brendan McMahan, Ilya Mironov, Nicolas Papernot, Kunal Talwar, Li Zhang:
On the Protection of Private Information in Machine Learning Systems: Two Recent Approaches. CoRR abs/1708.08022 (2017) - [i19]H. Brendan McMahan, Daniel Ramage, Kunal Talwar, Li Zhang:
Learning Differentially Private Language Models Without Losing Accuracy. CoRR abs/1710.06963 (2017) - [i18]Kallista A. Bonawitz, Vladimir Ivanov, Ben Kreuter, Antonio Marcedone, H. Brendan McMahan, Sarvar Patel, Daniel Ramage, Aaron Segal, Karn Seth:
Practical Secure Aggregation for Privacy Preserving Machine Learning. IACR Cryptol. ePrint Arch. 2017: 281 (2017) - 2016
- [c23]Martín Abadi, Andy Chu, Ian J. Goodfellow, H. Brendan McMahan, Ilya Mironov
, Kunal Talwar, Li Zhang:
Deep Learning with Differential Privacy. CCS 2016: 308-318 - [i17]H. Brendan McMahan, Eider Moore, Daniel Ramage, Blaise Agüera y Arcas:
Federated Learning of Deep Networks using Model Averaging. CoRR abs/1602.05629 (2016) - [i16]Martín Abadi, Andy Chu, Ian J. Goodfellow, H. Brendan McMahan, Ilya Mironov, Kunal Talwar, Li Zhang:
Deep Learning with Differential Privacy. CoRR abs/1607.00133 (2016) - [i15]Jakub Konecný, H. Brendan McMahan, Daniel Ramage, Peter Richtárik:
Federated Optimization: Distributed Machine Learning for On-Device Intelligence. CoRR abs/1610.02527 (2016) - [i14]Jakub Konecný, H. Brendan McMahan, Felix X. Yu, Peter Richtárik, Ananda Theertha Suresh, Dave Bacon:
Federated Learning: Strategies for Improving Communication Efficiency. CoRR abs/1610.05492 (2016) - [i13]Ananda Theertha Suresh, Felix X. Yu, H. Brendan McMahan, Sanjiv Kumar:
Distributed Mean Estimation with Limited Communication. CoRR abs/1611.00429 (2016) - [i12]Kallista A. Bonawitz, Vladimir Ivanov, Ben Kreuter, Antonio Marcedone, H. Brendan McMahan, Sarvar Patel, Daniel Ramage, Aaron Segal, Karn Seth:
Practical Secure Aggregation for Federated Learning on User-Held Data. CoRR abs/1611.04482 (2016) - 2015
- [i11]Jakub Konecný, Brendan McMahan, Daniel Ramage:
Federated Optimization: Distributed Optimization Beyond the Datacenter. CoRR abs/1511.03575 (2015) - 2014
- [c22]H. Brendan McMahan, Francesco Orabona:
Unconstrained Online Linear Learning in Hilbert Spaces: Minimax Algorithms and Normal Approximations. COLT 2014: 1020-1039 - [c21]H. Brendan McMahan, Matthew J. Streeter:
Delay-Tolerant Algorithms for Asynchronous Distributed Online Learning. NIPS 2014: 2915-2923 - [i10]H. Brendan McMahan, Francesco Orabona:
Unconstrained Online Linear Learning in Hilbert Spaces: Minimax Algorithms and Normal Approximations. CoRR abs/1403.0628 (2014) - [i9]H. Brendan McMahan:
Analysis Techniques for Adaptive Online Learning. CoRR abs/1403.3465 (2014) - 2013
- [c20]Daniel Golovin, D. Sculley, H. Brendan McMahan, Michael Young:
Large-Scale Learning with Less RAM via Randomization. ICML (2) 2013: 325-333 - [c19]H. Brendan McMahan, Gary Holt, David Sculley, Michael Young, Dietmar Ebner, Julian Grady, Lan Nie, Todd Phillips, Eugene Davydov, Daniel Golovin, Sharat Chikkerur, Dan Liu, Martin Wattenberg, Arnar Mar Hrafnkelsson, Tom Boulos, Jeremy Kubica:
Ad click prediction: a view from the trenches. KDD 2013: 1222-1230 - [c18]H. Brendan McMahan, Jacob D. Abernethy:
Minimax Optimal Algorithms for Unconstrained Linear Optimization. NIPS 2013: 2724-2732 - [c17]John C. Duchi, Michael I. Jordan, H. Brendan McMahan:
Estimation, Optimization, and Parallelism when Data is Sparse. NIPS 2013: 2832-2840 - [i8]H. Brendan McMahan:
Minimax Optimal Algorithms for Unconstrained Linear Optimization. CoRR abs/1302.2176 (2013) - [i7]Daniel Golovin, D. Sculley, H. Brendan McMahan, Michael Young:
Large-Scale Learning with Less RAM via Randomization. CoRR abs/1303.4664 (2013) - 2012
- [c16]Matthew J. Streeter, H. Brendan McMahan:
No-Regret Algorithms for Unconstrained Online Convex Optimization. NIPS 2012: 2411-2419 - [c15]H. Brendan McMahan, Matthew J. Streeter:
Open Problem: Better Bounds for Online Logistic Regression. COLT 2012: 44.1-44.3 - [i6]Matthew J. Streeter, H. Brendan McMahan:
No-Regret Algorithms for Unconstrained Online Convex Optimization. CoRR abs/1211.2260 (2012) - [i5]H. Brendan McMahan, Omkar Muralidharan:
On Calibrated Predictions for Auction Selection Mechanisms. CoRR abs/1211.3955 (2012) - 2011
- [c14]H. Brendan McMahan:
Discussion of "Contextual Bandit Algorithms with Supervised Learning Guarantees". AISTATS 2011: 27-28 - [c13]H. Brendan McMahan:
Follow-the-Regularized-Leader and Mirror Descent: Equivalence Theorems and L1 Regularization. AISTATS 2011: 525-533 - 2010
- [c12]H. Brendan McMahan, Matthew J. Streeter:
Adaptive Bound Optimization for Online Convex Optimization. COLT 2010: 244-256 - [i4]Matthew J. Streeter, H. Brendan McMahan:
Less Regret via Online Conditioning. CoRR abs/1002.4862 (2010) - [i3]H. Brendan McMahan, Matthew J. Streeter:
Adaptive Bound Optimization for Online Convex Optimization. CoRR abs/1002.4908 (2010) - [i2]H. Brendan McMahan:
Follow-the-Regularized-Leader and Mirror Descent: Equivalence Theorems and Implicit Updates. CoRR abs/1009.3240 (2010)
2000 – 2009
- 2009
- [c11]H. Brendan McMahan, Matthew J. Streeter:
Tighter Bounds for Multi-Armed Bandits with Expert Advice. COLT 2009 - [c10]Varun Kanade, H. Brendan McMahan, Brent Bryan:
Sleeping Experts and Bandits with Stochastic Action Availability and Adversarial Rewards. AISTATS 2009: 272-279 - 2007
- [c9]H. Brendan McMahan, Geoffrey J. Gordon:
A Unification of Extensive-Form Games and Markov Decision Processes. AAAI 2007: 86-93 - [c8]Brent Bryan, H. Brendan McMahan, Chad M. Schafer, Jeff G. Schneider:
Efficiently computing minimax expected-size confidence regions. ICML 2007: 97-104 - [c7]Andreas Krause, H. Brendan McMahan, Carlos Guestrin, Anupam Gupta:
Selecting Observations against Adversarial Objectives. NIPS 2007: 777-784 - [c6]H. Brendan McMahan, Geoffrey J. Gordon:
A Fast Bundle-based Anytime Algorithm for Poker and other Convex Games. AISTATS 2007: 323-330 - 2005
- [c5]H. Brendan McMahan, Geoffrey J. Gordon:
Fast Exact Planning in Markov Decision Processes. ICAPS 2005: 151-160 - [c4]H. Brendan McMahan, Maxim Likhachev, Geoffrey J. Gordon:
Bounded real-time dynamic programming: RTDP with monotone upper bounds and performance guarantees. ICML 2005: 569-576 - [c3]Abraham Flaxman, Adam Tauman Kalai
, H. Brendan McMahan:
Online convex optimization in the bandit setting: gradient descent without a gradient. SODA 2005: 385-394 - 2004
- [j1]H. Brendan McMahan, Andrzej Proskurowski:
Multi-source spanning trees: algorithms for minimizing source eccentricities. Discret. Appl. Math. 137(2): 213-222 (2004) - [c2]H. Brendan McMahan, Avrim Blum:
Online Geometric Optimization in the Bandit Setting Against an Adaptive Adversary. COLT 2004: 109-123 - [i1]Abraham Flaxman, Adam Tauman Kalai, H. Brendan McMahan:
Online convex optimization in the bandit setting: gradient descent without a gradient. CoRR cs.LG/0408007 (2004) - 2003
- [c1]H. Brendan McMahan, Geoffrey J. Gordon, Avrim Blum:
Planning in the Presence of Cost Functions Controlled by an Adversary. ICML 2003: 536-543
Coauthor Index

manage site settings
To protect your privacy, all features that rely on external API calls from your browser are turned off by default. You need to opt-in for them to become active. All settings here will be stored as cookies with your web browser. For more information see our F.A.Q.
Unpaywalled article links
Add open access links from to the list of external document links (if available).
Privacy notice: By enabling the option above, your browser will contact the API of unpaywall.org to load hyperlinks to open access articles. Although we do not have any reason to believe that your call will be tracked, we do not have any control over how the remote server uses your data. So please proceed with care and consider checking the Unpaywall privacy policy.
Archived links via Wayback Machine
For web page which are no longer available, try to retrieve content from the of the Internet Archive (if available).
Privacy notice: By enabling the option above, your browser will contact the API of archive.org to check for archived content of web pages that are no longer available. Although we do not have any reason to believe that your call will be tracked, we do not have any control over how the remote server uses your data. So please proceed with care and consider checking the Internet Archive privacy policy.
Reference lists
Add a list of references from ,
, and
to record detail pages.
load references from crossref.org and opencitations.net
Privacy notice: By enabling the option above, your browser will contact the APIs of crossref.org, opencitations.net, and semanticscholar.org to load article reference information. Although we do not have any reason to believe that your call will be tracked, we do not have any control over how the remote server uses your data. So please proceed with care and consider checking the Crossref privacy policy and the OpenCitations privacy policy, as well as the AI2 Privacy Policy covering Semantic Scholar.
Citation data
Add a list of citing articles from and
to record detail pages.
load citations from opencitations.net
Privacy notice: By enabling the option above, your browser will contact the API of opencitations.net and semanticscholar.org to load citation information. Although we do not have any reason to believe that your call will be tracked, we do not have any control over how the remote server uses your data. So please proceed with care and consider checking the OpenCitations privacy policy as well as the AI2 Privacy Policy covering Semantic Scholar.
OpenAlex data
Load additional information about publications from .
Privacy notice: By enabling the option above, your browser will contact the API of openalex.org to load additional information. Although we do not have any reason to believe that your call will be tracked, we do not have any control over how the remote server uses your data. So please proceed with care and consider checking the information given by OpenAlex.
last updated on 2024-12-10 20:51 CET by the dblp team
all metadata released as open data under CC0 1.0 license
see also: Terms of Use | Privacy Policy | Imprint