Indoor Visual-Based Localization System for Multi-Rotor UAVs
- PMID: 35957353
- PMCID: PMC9370993
- DOI: 10.3390/s22155798
Indoor Visual-Based Localization System for Multi-Rotor UAVs
Abstract
Industry 4.0, smart homes, and the Internet of Things are boosting the employment of autonomous aerial vehicles in indoor environments, where localization is still challenging, especially in the case of close and cluttered areas. In this paper, we propose a Visual Inertial Odometry localization method based on fiducial markers. Our approach enables multi-rotor aerial vehicle navigation in indoor environments and tackles the most challenging aspects of image-based indoor localization. In particular, we focus on a proper and continuous pose estimation, working from take-off to landing, at several different flying altitudes. With this aim, we designed a map of fiducial markers that produces results that are both dense and heterogeneous. Narrowly placed tags lead to minimal information loss during rapid aerial movements while four different classes of marker size provide consistency when the camera zooms in or out according to the vehicle distance from the ground. We have validated our approach by comparing the output of the localization algorithm with the ground-truth information collected through an optoelectronic motion capture system, using two different platforms in different flying conditions. The results show that error mean and standard deviation can remain constantly lower than 0.11 m, so not degrading when the aerial vehicle increases its altitude and, therefore, strongly improving similar state-of-the-art solutions.
Keywords: Visual Inertial Odometry; aerial vehicles; fiducial markers; indoor localization.
Conflict of interest statement
The authors declare no conflict of interest.
Figures
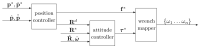
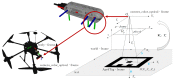
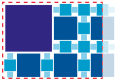
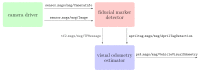
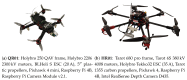
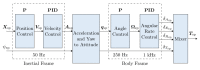
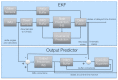
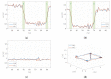
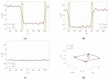
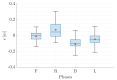
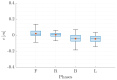
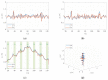
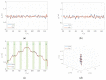
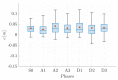
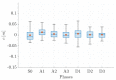
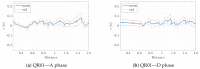
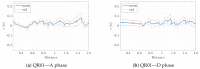
Similar articles
-
VIAE-Net: An End-to-End Altitude Estimation through Monocular Vision and Inertial Feature Fusion Neural Networks for UAV Autonomous Landing.Sensors (Basel). 2021 Sep 20;21(18):6302. doi: 10.3390/s21186302. Sensors (Basel). 2021. PMID: 34577508 Free PMC article.
-
Marker-Based Multi-Sensor Fusion Indoor Localization System for Micro Air Vehicles.Sensors (Basel). 2018 May 25;18(6):1706. doi: 10.3390/s18061706. Sensors (Basel). 2018. PMID: 29799441 Free PMC article.
-
Smart Artificial Markers for Accurate Visual Mapping and Localization.Sensors (Basel). 2021 Jan 18;21(2):625. doi: 10.3390/s21020625. Sensors (Basel). 2021. PMID: 33477398 Free PMC article.
-
Visual SLAM for Unmanned Aerial Vehicles: Localization and Perception.Sensors (Basel). 2024 May 8;24(10):2980. doi: 10.3390/s24102980. Sensors (Basel). 2024. PMID: 38793834 Free PMC article. Review.
-
Sensors and Sensor Fusion Methodologies for Indoor Odometry: A Review.Polymers (Basel). 2022 May 15;14(10):2019. doi: 10.3390/polym14102019. Polymers (Basel). 2022. PMID: 35631899 Free PMC article. Review.
Cited by
-
Analytical Models for Pose Estimate Variance of Planar Fiducial Markers for Mobile Robot Localisation.Sensors (Basel). 2023 Jun 20;23(12):5746. doi: 10.3390/s23125746. Sensors (Basel). 2023. PMID: 37420909 Free PMC article.
References
-
- Hamandi M., Usai F., Sablé Q., Staub N., Tognon M., Franchi A. Design of Multirotor Aerial Vehicles: A Taxonomy Based on Input Allocation. Int. J. Robot. Res. 2021;40:1015–1044. doi: 10.1177/02783649211025998. - DOI
-
- Shakeri R., Al-Garadi M.A., Badawy A., Mohamed A., Khattab T., Al-Ali A.K., Harras K.A., Guizani M. Design challenges of multi-UAV systems in cyber-physical applications: A comprehensive survey and future directions. IEEE Commun. Surv. Tutor. 2019;21:3340–3385. doi: 10.1109/COMST.2019.2924143. - DOI
-
- Shakhatreh H., Sawalmeh A.H., Al-Fuqaha A., Dou Z., Almaita E., Khalil I., Othman N.S., Khreishah A., Guizani M. Unmanned aerial vehicles (UAVs): A survey on civil applications and key research challenges. IEEE Access. 2019;7:48572–48634. doi: 10.1109/ACCESS.2019.2909530. - DOI
-
- Islam N., Rashid M.M., Pasandideh F., Ray B., Moore S., Kadel R. A Review of Applications and Communication Technologies for Internet of Things (IoT) and Unmanned Aerial Vehicle (UAV) Based Sustainable Smart Farming. Sustainability. 2021;13:1821. doi: 10.3390/su13041821. - DOI
-
- Dastgheibifard S., Asnafi M. A review on potential applications of unmanned aerial vehicle for construction industry. Sustain. Struct. Mater. 2018;1:44–53.
MeSH terms
Grants and funding
LinkOut - more resources
Full Text Sources