Unified classification of mouse retinal ganglion cells using function, morphology, and gene expression
- PMID: 35830791
- PMCID: PMC9364428
- DOI: 10.1016/j.celrep.2022.111040
Unified classification of mouse retinal ganglion cells using function, morphology, and gene expression
Abstract
Classification and characterization of neuronal types are critical for understanding their function and dysfunction. Neuronal classification schemes typically rely on measurements of electrophysiological, morphological, and molecular features, but aligning such datasets has been challenging. Here, we present a unified classification of mouse retinal ganglion cells (RGCs), the sole retinal output neurons. We use visually evoked responses to classify 1,859 mouse RGCs into 42 types. We also obtain morphological or transcriptomic data from subsets and use these measurements to align the functional classification to publicly available morphological and transcriptomic datasets. We create an online database that allows users to browse or download the data and to classify RGCs from their light responses using a machine learning algorithm. This work provides a resource for studies of RGCs, their upstream circuits in the retina, and their projections in the brain, and establishes a framework for future efforts in neuronal classification and open data distribution.
Keywords: CP: Neuroscience; retina, retinal ganglion cell, transcriptomics, morphology, light responses, classification.
Copyright © 2022 The Authors. Published by Elsevier Inc. All rights reserved.
Conflict of interest statement
Declaration of interests The authors declare no competing interests.
Figures
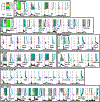
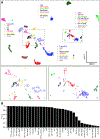
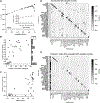
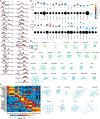
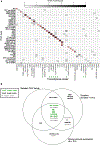
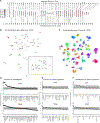
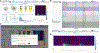
Similar articles
-
Three Small-Receptive-Field Ganglion Cells in the Mouse Retina Are Distinctly Tuned to Size, Speed, and Object Motion.J Neurosci. 2017 Jan 18;37(3):610-625. doi: 10.1523/JNEUROSCI.2804-16.2016. J Neurosci. 2017. PMID: 28100743 Free PMC article.
-
Light-evoked synaptic activity of retinal ganglion and amacrine cells is regulated in developing mouse retina.Eur J Neurosci. 2011 Jan;33(1):36-48. doi: 10.1111/j.1460-9568.2010.07484.x. Epub 2010 Nov 22. Eur J Neurosci. 2011. PMID: 21091802 Free PMC article.
-
Types and density of calretinin-containing retinal ganglion cells in mouse.Neurosci Res. 2010 Feb;66(2):141-50. doi: 10.1016/j.neures.2009.10.008. Epub 2009 Nov 4. Neurosci Res. 2010. PMID: 19895859
-
The types of retinal ganglion cells: current status and implications for neuronal classification.Annu Rev Neurosci. 2015 Jul 8;38:221-46. doi: 10.1146/annurev-neuro-071714-034120. Epub 2015 Apr 9. Annu Rev Neurosci. 2015. PMID: 25897874 Review.
-
Feature Detection by Retinal Ganglion Cells.Annu Rev Vis Sci. 2022 Sep 15;8:135-169. doi: 10.1146/annurev-vision-100419-112009. Epub 2022 Apr 6. Annu Rev Vis Sci. 2022. PMID: 35385673 Review.
Cited by
-
Applying Super-Resolution and Tomography Concepts to Identify Receptive Field Subunits in the Retina.PLoS Comput Biol. 2024 Sep 3;20(9):e1012370. doi: 10.1371/journal.pcbi.1012370. eCollection 2024 Sep. PLoS Comput Biol. 2024. PMID: 39226328 Free PMC article.
-
Signaling - transcription interactions in mouse retinal ganglion cells early axon pathfinding -a literature review.Front Ophthalmol (Lausanne). 2023 May 17;3:1180142. doi: 10.3389/fopht.2023.1180142. eCollection 2023. Front Ophthalmol (Lausanne). 2023. PMID: 38983012 Free PMC article. Review.
-
Modular interneuron circuits control motion sensitivity in the mouse retina.Nat Commun. 2023 Nov 27;14(1):7746. doi: 10.1038/s41467-023-43382-0. Nat Commun. 2023. PMID: 38008788 Free PMC article.
-
Differences in spike generation instead of synaptic inputs determine the feature selectivity of two retinal cell types.Neuron. 2022 Jul 6;110(13):2110-2123.e4. doi: 10.1016/j.neuron.2022.04.012. Epub 2022 May 3. Neuron. 2022. PMID: 35508174 Free PMC article.
-
Subcellular pathways through VGluT3-expressing mouse amacrine cells provide locally tuned object-motion-selective signals in the retina.Nat Commun. 2024 Apr 5;15(1):2965. doi: 10.1038/s41467-024-46996-0. Nat Commun. 2024. PMID: 38580652 Free PMC article.
References
Publication types
MeSH terms
Grants and funding
LinkOut - more resources
Full Text Sources
Molecular Biology Databases
Miscellaneous