Tomek Link and SMOTE Approaches for Machine Fault Classification with an Imbalanced Dataset
- PMID: 35590937
- PMCID: PMC9099503
- DOI: 10.3390/s22093246
Tomek Link and SMOTE Approaches for Machine Fault Classification with an Imbalanced Dataset
Abstract
Data-driven methods have prominently featured in the progressive research and development of modern condition monitoring systems for electrical machines. These methods have the advantage of simplicity when it comes to the implementation of effective fault detection and diagnostic systems. Despite their many advantages, the practical implementation of data-driven approaches still faces challenges such as data imbalance. The lack of sufficient and reliable labeled fault data from machines in the field often poses a challenge in developing accurate supervised learning-based condition monitoring systems. This research investigates the use of a Naïve Bayes classifier, support vector machine, and k-nearest neighbors together with synthetic minority oversampling technique, Tomek link, and the combination of these two resampling techniques for fault classification with simulation and experimental imbalanced data. A comparative analysis of these techniques is conducted for different imbalanced data cases to determine the suitability thereof for condition monitoring on a wound-rotor induction generator. The precision, recall, and f1-score matrices are applied for performance evaluation. The results indicate that the technique combining the synthetic minority oversampling technique with the Tomek link provides the best performance across all tested classifiers. The k-nearest neighbors, together with this combination resampling technique yielded the most accurate classification results. This research is of interest to researchers and practitioners working in the area of condition monitoring in electrical machines, and the findings and presented approach of the comparative analysis will assist with the selection of the most suitable technique for handling imbalanced fault data. This is especially important in the practice of condition monitoring on electrical rotating machines, where fault data are very limited.
Keywords: Bayesian classification; Tomek link; imbalanced data; k-nearest neighbor; support vector machine; synthetic minority over-sampling sampling; wound-rotor induction generator.
Conflict of interest statement
The authors declare no conflict of interest.
Figures
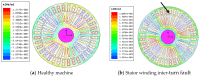
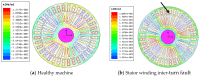
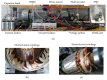
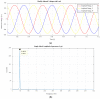
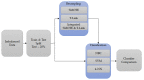
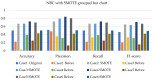
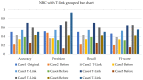
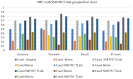
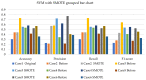
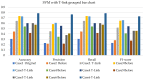
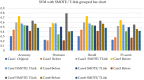
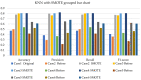
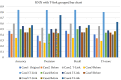
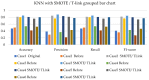
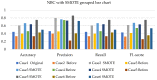
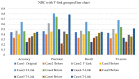
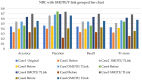
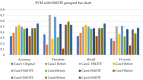
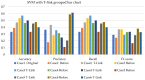
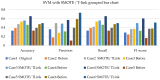
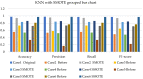
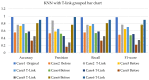
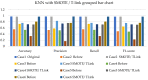
Similar articles
-
Churn prediction in telecommunication industry using kernel Support Vector Machines.PLoS One. 2022 May 24;17(5):e0267935. doi: 10.1371/journal.pone.0267935. eCollection 2022. PLoS One. 2022. PMID: 35609023 Free PMC article.
-
Comparing Sampling Strategies for Tackling Imbalanced Data in Human Activity Recognition.Sensors (Basel). 2022 Feb 11;22(4):1373. doi: 10.3390/s22041373. Sensors (Basel). 2022. PMID: 35214275 Free PMC article.
-
Classification of Imbalanced Data by Oversampling in Kernel Space of Support Vector Machines.IEEE Trans Neural Netw Learn Syst. 2018 Sep;29(9):4065-4076. doi: 10.1109/TNNLS.2017.2751612. Epub 2017 Oct 10. IEEE Trans Neural Netw Learn Syst. 2018. PMID: 29028213
-
Comparative Studies on Resampling Techniques in Machine Learning and Deep Learning Models for Drug-Target Interaction Prediction.Molecules. 2023 Feb 9;28(4):1663. doi: 10.3390/molecules28041663. Molecules. 2023. PMID: 36838652 Free PMC article. Review.
-
A comprehensive data level analysis for cancer diagnosis on imbalanced data.J Biomed Inform. 2019 Feb;90:103089. doi: 10.1016/j.jbi.2018.12.003. Epub 2019 Jan 3. J Biomed Inform. 2019. PMID: 30611011 Review.
Cited by
-
An Oversampling Method of Unbalanced Data for Mechanical Fault Diagnosis Based on MeanRadius-SMOTE.Sensors (Basel). 2022 Jul 10;22(14):5166. doi: 10.3390/s22145166. Sensors (Basel). 2022. PMID: 35890845 Free PMC article.
-
Investigation of bacterial DNA gyrase Inhibitor classification models and structural requirements utilizing multiple machine learning methods.Mol Divers. 2024 Aug;28(4):2119-2133. doi: 10.1007/s11030-024-10806-y. Epub 2024 Feb 19. Mol Divers. 2024. PMID: 38372837
-
Predicting cysteine reactivity changes upon phosphorylation using XGBoost.FEBS Open Bio. 2024 Jan;14(1):51-62. doi: 10.1002/2211-5463.13737. Epub 2023 Nov 20. FEBS Open Bio. 2024. PMID: 37964470 Free PMC article.
-
Platelet Metabolites as Candidate Biomarkers in Sepsis Diagnosis and Management Using the Proposed Explainable Artificial Intelligence Approach.J Clin Med. 2024 Aug 23;13(17):5002. doi: 10.3390/jcm13175002. J Clin Med. 2024. PMID: 39274215 Free PMC article.
-
A Long-Tailed Image Classification Method Based on Enhanced Contrastive Visual Language.Sensors (Basel). 2023 Jul 26;23(15):6694. doi: 10.3390/s23156694. Sensors (Basel). 2023. PMID: 37571481 Free PMC article.
References
-
- Khan M.U., Imtiaz M.A., Aziz S., Kareem Z., Waseem A., Akram M.A. System design for early fault diagnosis of machines using vibration features; Proceedings of the IEEE 5th International Conference on Power Generation Systems and Renewable Energy Technologies; Istanbul, Turkey. 26–27 August 2019.
-
- Spyropoulos D.V., Mitronikas E.D. A review on the faults of electric machines used in electric ships. Adv. Power Electron. 2013;2013:216870. doi: 10.1155/2013/216870. - DOI
-
- Salomon C.P., Ferreira C., Sant’Ana W., Lambert-Torres G., Borges da Silva L.E., Bonaldi E.L., Oliveira L., Torres B. A study of fault diagnosis based on electrical signature analysis for synchronous generators predictive maintenance in bulk electric systems. Energies. 2019;12:1506. doi: 10.3390/en12081506. - DOI
-
- Rehman A.U., Chen Y., Wang L., Zhao Y., Yonghong Y., Yonghong C., Tanaka T. Experimental research and analysis on rotor winding inter-turn circuit fault in DFIG; Proceedings of the IEEE International Conference on Condition Monitoring and Diagnosis; Xi’an, China. 25–28 September 2016.
-
- Sun W., Zhao R., Yan R., Shao S., Chen X. Convolutional discriminative feature learning for induction motor fault diagnosis. IEEE Trans. Ind. Inform. 2017;13:1350–1359. doi: 10.1109/TII.2017.2672988. - DOI
MeSH terms
LinkOut - more resources
Full Text Sources