Anomaly detection using edge computing in video surveillance system: review
- PMID: 35368446
- PMCID: PMC8963404
- DOI: 10.1007/s13735-022-00227-8
Anomaly detection using edge computing in video surveillance system: review
Abstract
The current concept of smart cities influences urban planners and researchers to provide modern, secured and sustainable infrastructure and gives a decent quality of life to its residents. To fulfill this need, video surveillance cameras have been deployed to enhance the safety and well-being of the citizens. Despite technical developments in modern science, abnormal event detection in surveillance video systems is challenging and requires exhaustive human efforts. In this paper, we focus on evolution of anomaly detection followed by survey of various methodologies developed to detect anomalies in intelligent video surveillance. Further, we revisit the surveys on anomaly detection in the last decade. We then present a systematic categorization of methodologies for anomaly detection. As the notion of anomaly depends on context, we identify different objects-of-interest and publicly available datasets in anomaly detection. Since anomaly detection is a time-critical application of computer vision, we explore the anomaly detection using edge devices and approaches explicitly designed for them. The confluence of edge computing and anomaly detection for real-time and intelligent surveillance applications is also explored. Further, we discuss the challenges and opportunities involved in anomaly detection using the edge devices.
Keywords: Anomaly detection; Edge computing; Machine learning; Video surveillance.
© The Author(s), under exclusive licence to Springer-Verlag London Ltd., part of Springer Nature 2022.
Conflict of interest statement
Conflicts of interestBoth the authors have checked the manuscript and have agreed to the submission in International Journal of Multimedia Information Retrieval. There is no conflict of interest between the authors.
Figures
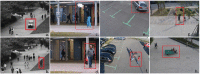
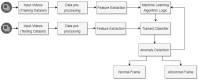
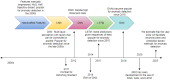
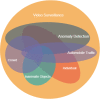
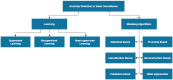
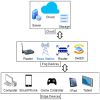
Similar articles
-
Embedded Vision Intelligence for the Safety of Smart Cities.J Imaging. 2022 Dec 14;8(12):326. doi: 10.3390/jimaging8120326. J Imaging. 2022. PMID: 36547491 Free PMC article.
-
An IoT Enable Anomaly Detection System for Smart City Surveillance.Sensors (Basel). 2023 Feb 20;23(4):2358. doi: 10.3390/s23042358. Sensors (Basel). 2023. PMID: 36850955 Free PMC article.
-
Deep Learning-Based Anomaly Detection in Video Surveillance: A Survey.Sensors (Basel). 2023 May 24;23(11):5024. doi: 10.3390/s23115024. Sensors (Basel). 2023. PMID: 37299751 Free PMC article. Review.
-
Smart Video Surveillance System Based on Edge Computing.Sensors (Basel). 2021 Apr 23;21(9):2958. doi: 10.3390/s21092958. Sensors (Basel). 2021. PMID: 33922548 Free PMC article.
-
Taxonomy of Anomaly Detection Techniques in Crowd Scenes.Sensors (Basel). 2022 Aug 14;22(16):6080. doi: 10.3390/s22166080. Sensors (Basel). 2022. PMID: 36015840 Free PMC article. Review.
Cited by
-
MC-ViViT: Multi-branch Classifier-ViViT to Detect Mild Cognitive Impairment in Older Adults Using Facial Videos.Expert Syst Appl. 2024 Mar 15;238(Pt B):121929. doi: 10.1016/j.eswa.2023.121929. Epub 2023 Oct 4. Expert Syst Appl. 2024. PMID: 39238945 Free PMC article.
-
Anomaly Detection on the Edge Using Smart Cameras under Low-Light Conditions.Sensors (Basel). 2024 Jan 24;24(3):772. doi: 10.3390/s24030772. Sensors (Basel). 2024. PMID: 38339490 Free PMC article.
-
Overlapping Shoeprint Detection by Edge Detection and Deep Learning.J Imaging. 2024 Jul 31;10(8):186. doi: 10.3390/jimaging10080186. J Imaging. 2024. PMID: 39194975 Free PMC article.
-
MDED-Framework: A Distributed Microservice Deep-Learning Framework for Object Detection in Edge Computing.Sensors (Basel). 2023 May 12;23(10):4712. doi: 10.3390/s23104712. Sensors (Basel). 2023. PMID: 37430625 Free PMC article.
-
Embedded Vision Intelligence for the Safety of Smart Cities.J Imaging. 2022 Dec 14;8(12):326. doi: 10.3390/jimaging8120326. J Imaging. 2022. PMID: 36547491 Free PMC article.
References
-
- Ahmed SA, Dogra DP, Kar S, Roy PP. Trajectory-based surveillance analysis: a survey. IEEE Trans Circuits Syst Video Technol. 2018;29(7):1985–1997. doi: 10.1109/TCSVT.2018.2857489. - DOI
-
- Ajay B, Rao M (2021) Binary neural network based real time emotion detection on an edge computing device to detect passenger anomaly, In: 2021 34th International conference on VLSI design and 2021 20th international conference on embedded systems (VLSID) pp 175–180, IEEE
-
- Angelini F, Yan J, Naqvi SM (2019) Privacy-preserving online human behaviour anomaly detection based on body movements and objects positions. In: ICASSP 2019-2019 IEEE international conference on acoustics, speech and signal processing (ICASSP) pp 8444–8448, IEEE
-
- Asad M, Yang J, He J, Shamsolmoali P, He X. Multi-frame feature-fusion-based model for violence detection. Visual Comput. 2021;37(6):1415–1431. doi: 10.1007/s00371-020-01878-6. - DOI
-
- Bansod SD, Nandedkar AV. Crowd anomaly detection and localization using histogram of magnitude and momentum. Vis Comput. 2020;36(3):609–620. doi: 10.1007/s00371-019-01647-0. - DOI
Publication types
LinkOut - more resources
Full Text Sources
Other Literature Sources
Research Materials