Entropy Weight Ensemble Framework for Yield Prediction of Winter Wheat Under Different Water Stress Treatments Using Unmanned Aerial Vehicle-Based Multispectral and Thermal Data
- PMID: 34987529
- PMCID: PMC8721222
- DOI: 10.3389/fpls.2021.730181
Entropy Weight Ensemble Framework for Yield Prediction of Winter Wheat Under Different Water Stress Treatments Using Unmanned Aerial Vehicle-Based Multispectral and Thermal Data
Abstract
Crop breeding programs generally perform early field assessments of candidate selection based on primary traits such as grain yield (GY). The traditional methods of yield assessment are costly, inefficient, and considered a bottleneck in modern precision agriculture. Recent advances in an unmanned aerial vehicle (UAV) and development of sensors have opened a new avenue for data acquisition cost-effectively and rapidly. We evaluated UAV-based multispectral and thermal images for in-season GY prediction using 30 winter wheat genotypes under 3 water treatments. For this, multispectral vegetation indices (VIs) and normalized relative canopy temperature (NRCT) were calculated and selected by the gray relational analysis (GRA) at each growth stage, i.e., jointing, booting, heading, flowering, grain filling, and maturity to reduce the data dimension. The elastic net regression (ENR) was developed by using selected features as input variables for yield prediction, whereas the entropy weight fusion (EWF) method was used to combine the predicted GY values from multiple growth stages. In our results, the fusion of dual-sensor data showed high yield prediction accuracy [coefficient of determination (R 2) = 0.527-0.667] compared to using a single multispectral sensor (R 2 = 0.130-0.461). Results showed that the grain filling stage was the optimal stage to predict GY with R 2 = 0.667, root mean square error (RMSE) = 0.881 t ha-1, relative root-mean-square error (RRMSE) = 15.2%, and mean absolute error (MAE) = 0.721 t ha-1. The EWF model outperformed at all the individual growth stages with R 2 varying from 0.677 to 0.729. The best prediction result (R 2 = 0.729, RMSE = 0.831 t ha-1, RRMSE = 14.3%, and MAE = 0.684 t ha-1) was achieved through combining the predicted values of all growth stages. This study suggests that the fusion of UAV-based multispectral and thermal IR data within an ENR-EWF framework can provide a precise and robust prediction of wheat yield.
Keywords: UAV; machine learning; multispectral indices; remote sensing; thermal infrared; wheat yield.
Copyright © 2021 Fei, Hassan, Ma, Shu, Cheng, Li, Chen and Xiao.
Conflict of interest statement
The authors declare that the research was conducted in the absence of any commercial or financial relationships that could be construed as a potential conflict of interest. The reviewer AR declared a past co-authorship with the authors MH, YX to the handling editor.
Figures
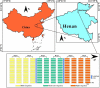
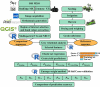
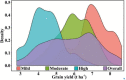
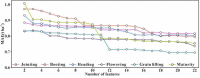
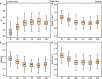
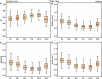
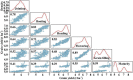
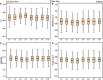
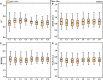
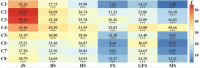
Similar articles
-
A rapid monitoring of NDVI across the wheat growth cycle for grain yield prediction using a multi-spectral UAV platform.Plant Sci. 2019 May;282:95-103. doi: 10.1016/j.plantsci.2018.10.022. Epub 2018 Nov 1. Plant Sci. 2019. PMID: 31003615
-
Inversion of Winter Wheat Growth Parameters and Yield Under Different Water Treatments Based on UAV Multispectral Remote Sensing.Front Plant Sci. 2021 May 20;12:609876. doi: 10.3389/fpls.2021.609876. eCollection 2021. Front Plant Sci. 2021. PMID: 34093601 Free PMC article.
-
Prediction of Chlorophyll Content in Multi-Temporal Winter Wheat Based on Multispectral and Machine Learning.Front Plant Sci. 2022 May 27;13:896408. doi: 10.3389/fpls.2022.896408. eCollection 2022. Front Plant Sci. 2022. PMID: 35712585 Free PMC article.
-
Perspectives for Remote Sensing with Unmanned Aerial Vehicles in Precision Agriculture.Trends Plant Sci. 2019 Feb;24(2):152-164. doi: 10.1016/j.tplants.2018.11.007. Epub 2018 Dec 15. Trends Plant Sci. 2019. PMID: 30558964 Review.
-
Methodological evolution of potato yield prediction: a comprehensive review.Front Plant Sci. 2023 Jul 26;14:1214006. doi: 10.3389/fpls.2023.1214006. eCollection 2023. Front Plant Sci. 2023. PMID: 37564384 Free PMC article. Review.
Cited by
-
Research hotspots and frontiers in agricultural multispectral technology: Bibliometrics and scientometrics analysis of the Web of Science.Front Plant Sci. 2022 Aug 11;13:955340. doi: 10.3389/fpls.2022.955340. eCollection 2022. Front Plant Sci. 2022. PMID: 36035687 Free PMC article.
-
Evaluation of Physicochemical Properties of Sustained-Release Membranes Based on Analytic Hierarchy.Membranes (Basel). 2023 Mar 9;13(3):313. doi: 10.3390/membranes13030313. Membranes (Basel). 2023. PMID: 36984701 Free PMC article.
-
Soybean leaf estimation based on RGB images and machine learning methods.Plant Methods. 2023 Jun 17;19(1):59. doi: 10.1186/s13007-023-01023-z. Plant Methods. 2023. PMID: 37330499 Free PMC article.
-
Rapid prediction of winter wheat yield and nitrogen use efficiency using consumer-grade unmanned aerial vehicles multispectral imagery.Front Plant Sci. 2022 Oct 24;13:1032170. doi: 10.3389/fpls.2022.1032170. eCollection 2022. Front Plant Sci. 2022. PMID: 36352879 Free PMC article.
-
Establishing a knowledge structure for yield prediction in cereal crops using unmanned aerial vehicles.Front Plant Sci. 2024 Aug 9;15:1401246. doi: 10.3389/fpls.2024.1401246. eCollection 2024. Front Plant Sci. 2024. PMID: 39184579 Free PMC article.
References
-
- Appeltans S., Guerrero A., Nawar S., Pieters J., Mouazen A. M. (2020). Practical recommendations for hyperspectral and thermal proximal disease sensing in potato and leek fields. Remote Sens. 12:1939. 10.3390/rs12121939 - DOI
-
- Aslan N., Shahrivar A. A., Abdollahi H. (2012). Multi-objective optimization of some process parameters of a lab-scale thickener using gray relational analysis. Sep. Purif. Technol. 90 189–195. 10.1016/j.seppur.2012.02.033 - DOI
-
- Ba Ret F., Guyot G. (1991). Potentials and limits of vegetation indices for LAI and APAR assessment. Remote Sens. Environ. 35 161–173. 10.1016/0034-4257(91)90009-U - DOI
-
- Bradley J. B. (1995). Neural networks: A comprehensive foundation. Inf. Process. Manage. 31 786. 10.1016/0306-4573(95)90003-9 - DOI
-
- Breiman L. (2001). Random forest. Mach. Learn. 45 5–32. 10.1023/a:101093340432 - DOI
LinkOut - more resources
Full Text Sources
Research Materials