Complementary water quality observations from high and medium resolution Sentinel sensors by aligning chlorophyll- a and turbidity algorithms
- PMID: 34732943
- PMCID: PMC8507437
- DOI: 10.1016/j.rse.2021.112651
Complementary water quality observations from high and medium resolution Sentinel sensors by aligning chlorophyll- a and turbidity algorithms
Abstract
High resolution imaging spectrometers are prerequisite to address significant data gaps in inland optical water quality monitoring. In this work, we provide a data-driven alignment of chlorophyll-a and turbidity derived from the Sentinel-2 MultiSpectral Imager (MSI) with corresponding Sentinel-3 Ocean and Land Colour Instrument (OLCI) products. For chlorophyll-a retrieval, empirical 'ocean colour' blue-green band ratios and a near infra-red (NIR) band ratio algorithm, as well as a semi-analytical three-band NIR-red ratio algorithm, were included in the analysis. Six million co-registrations with MSI and OLCI spanning 24 lakes across five continents were analysed. Following atmospheric correction with POLYMER, the reflectance distributions of the red and NIR bands showed close similarity between the two sensors, whereas the distribution for blue and green bands was positively skewed in the MSI results compared to OLCI. Whilst it is not possible from this analysis to determine the accuracy of reflectance retrieved with either MSI or OLCI results, optimizing water quality algorithms for MSI against those previously derived for the Envisat Medium Resolution Imaging Spectrometer (MERIS) and its follow-on OLCI, supports the wider use of MSI for aquatic applications. Chlorophyll-a algorithms were thus tuned for MSI against concurrent OLCI observations, resulting in significant improvements against the original algorithm coefficients. The mean absolute difference (MAD) for the blue-green band ratio algorithm decreased from 1.95 mg m-3 to 1.11 mg m-3, whilst the correlation coefficient increased from 0.61 to 0.80. For the NIR-red band ratio algorithms improvements were modest, with the MAD decreasing from 4.68 to 4.64 mg m-3 for the empirical red band ratio algorithm, and 3.73 to 3.67 for the semi-analytical 3-band algorithm. Three implementations of the turbidity algorithm showed improvement after tuning with the resulting distributions having reduced bias. The MAD reduced from 0.85 to 0.72, 1.22 to 1.10 and 1.93 to 1.55 FNU for the 665, 708 and 778 nm implementations respectively. However, several sources of uncertainty remain: adjacent land showed high divergence between the sensors, suggesting that high product uncertainty near land continues to be an issue for small water bodies, while it cannot be stated at this point whether MSI or OLCI results are differentially affected. The effect of spectrally wider bands of the MSI on algorithm sensitivity to chlorophyll-a and turbidity cannot be fully established without further availability of in situ optical measurements.
© 2021 The Authors.
Conflict of interest statement
The authors declare that they have no known competing financial interests or personal relationships that could have appeared to influence the work reported in this paper.
Figures
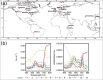
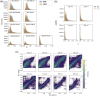
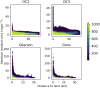
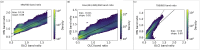
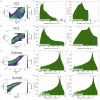
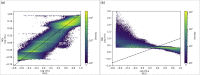
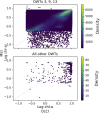
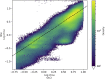
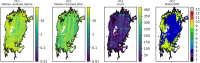
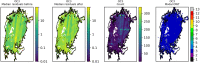
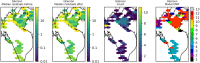
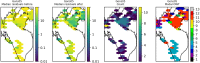
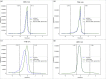
Similar articles
-
Validation and Comparison of Water Quality Products in Baltic Lakes Using Sentinel-2 MSI and Sentinel-3 OLCI Data.Sensors (Basel). 2020 Jan 29;20(3):742. doi: 10.3390/s20030742. Sensors (Basel). 2020. PMID: 32013214 Free PMC article.
-
A new approach to quantify chlorophyll-a over inland water targets based on multi-source remote sensing data.Sci Total Environ. 2024 Jan 1;906:167631. doi: 10.1016/j.scitotenv.2023.167631. Epub 2023 Oct 6. Sci Total Environ. 2024. PMID: 37806589
-
Seamless observations of chlorophyll-a from OLCI and VIIRS measurements in inland lakes.Water Res. 2025 Feb 15;270:122825. doi: 10.1016/j.watres.2024.122825. Epub 2024 Nov 20. Water Res. 2025. PMID: 39580942
-
Evaluation of Sentinel-3A OLCI Products Derived Using the Case-2 Regional CoastColour Processor over the Baltic Sea.Sensors (Basel). 2019 Aug 19;19(16):3609. doi: 10.3390/s19163609. Sensors (Basel). 2019. PMID: 31430993 Free PMC article.
-
Remote sensing of the chlorophyll-a based on OLI/Landsat-8 and MSI/Sentinel-2A (Barra Bonita reservoir, Brazil).An Acad Bras Cienc. 2018 Aug;90(2 suppl 1):1987-2000. doi: 10.1590/0001-3765201720170125. Epub 2017 Aug 31. An Acad Bras Cienc. 2018. PMID: 28876398
Cited by
-
Linking land use and precipitation changes to water quality changes in Lake Victoria using earth observation data.Environ Monit Assess. 2024 Oct 25;196(11):1104. doi: 10.1007/s10661-024-13261-2. Environ Monit Assess. 2024. PMID: 39453572 Free PMC article.
References
-
- Alikas K., Vabson V., Ansko I., Tilstone G.H., Dall’Olmo G., Nencioli F., Vendt R., Donlon C., Casal T. Comparison of above-water seabird and TriOS radiometers along an Atlantic meridional transect. Remote Sens. 2020;12(10):1669. doi: 10.3390/rs12101669. - DOI
-
- Alikas K., Ansko I., Vabson V., Ansper A., Kangro K., Uudeberg K., Ligi M. Consistency of radiometric satellite data over lakes and coastal waters with local field measurements. Remote Sens. 2020;12(4):616. doi: 10.3390/rs12040616. - DOI
-
- Attila J., Kauppila P., Kallio K.Y., Alasalmi H., Keto V., Bruun E., Koponen S. Applicability of earth observation chlorophyll-a data in assessment of water status via MERIS — with implications for the use of OLCI sensors. Remote Sens. Environ. 2018;212:273–287. doi: 10.1016/j.rse.2018.02.043. - DOI
-
- Binding C., Stumpf R.P., Schaeffer B.A., Tyler A., Hunter P. In: Earth Observations in Support of Global Water Quality Monitoring. Greb S., Dekker A., Binding C., editors. 2018. Introduction to deriving water quality measures from satellites. IOCCG Report Number 172018.
-
- Blondeau-Patissier D., Gower J.F.R., Dekker A.G., Phinn S.R., Brando V.E. A review of ocean color remote sensing methods and statistical techniques for the detection, mapping and analysis of phytoplankton blooms in coastal and open oceans. Prog. Oceanogr. 2014;123:123–144. doi: 10.1016/j.pocean.2013.12.008. - DOI
LinkOut - more resources
Full Text Sources
Miscellaneous