Lack of Transparency and Potential Bias in Artificial Intelligence Data Sets and Algorithms: A Scoping Review
- PMID: 34550305
- PMCID: PMC9379852
- DOI: 10.1001/jamadermatol.2021.3129
Lack of Transparency and Potential Bias in Artificial Intelligence Data Sets and Algorithms: A Scoping Review
Abstract
Importance: Clinical artificial intelligence (AI) algorithms have the potential to improve clinical care, but fair, generalizable algorithms depend on the clinical data on which they are trained and tested.
Objective: To assess whether data sets used for training diagnostic AI algorithms addressing skin disease are adequately described and to identify potential sources of bias in these data sets.
Data sources: In this scoping review, PubMed was used to search for peer-reviewed research articles published between January 1, 2015, and November 1, 2020, with the following paired search terms: deep learning and dermatology, artificial intelligence and dermatology, deep learning and dermatologist, and artificial intelligence and dermatologist.
Study selection: Studies that developed or tested an existing deep learning algorithm for triage, diagnosis, or monitoring using clinical or dermoscopic images of skin disease were selected, and the articles were independently reviewed by 2 investigators to verify that they met selection criteria.
Consensus process: Data set audit criteria were determined by consensus of all authors after reviewing existing literature to highlight data set transparency and sources of bias.
Results: A total of 70 unique studies were included. Among these studies, 1 065 291 images were used to develop or test AI algorithms, of which only 257 372 (24.2%) were publicly available. Only 14 studies (20.0%) included descriptions of patient ethnicity or race in at least 1 data set used. Only 7 studies (10.0%) included any information about skin tone in at least 1 data set used. Thirty-six of the 56 studies developing new AI algorithms for cutaneous malignant neoplasms (64.3%) met the gold standard criteria for disease labeling. Public data sets were cited more often than private data sets, suggesting that public data sets contribute more to new development and benchmarks.
Conclusions and relevance: This scoping review identified 3 issues in data sets that are used to develop and test clinical AI algorithms for skin disease that should be addressed before clinical translation: (1) sparsity of data set characterization and lack of transparency, (2) nonstandard and unverified disease labels, and (3) inability to fully assess patient diversity used for algorithm development and testing.
Figures
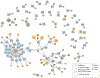
Comment in
-
Risk of Bias and Error From Data Sets Used for Dermatologic Artificial Intelligence.JAMA Dermatol. 2021 Nov 1;157(11):1271-1273. doi: 10.1001/jamadermatol.2021.3128. JAMA Dermatol. 2021. PMID: 34550304 No abstract available.
Similar articles
-
Artificial Intelligence for the Prediction and Early Diagnosis of Pancreatic Cancer: Scoping Review.J Med Internet Res. 2023 Mar 31;25:e44248. doi: 10.2196/44248. J Med Internet Res. 2023. PMID: 37000507 Free PMC article. Review.
-
Artificial Intelligence for Skin Cancer Detection: Scoping Review.J Med Internet Res. 2021 Nov 24;23(11):e22934. doi: 10.2196/22934. J Med Internet Res. 2021. PMID: 34821566 Free PMC article. Review.
-
Ethical considerations for artificial intelligence in dermatology: a scoping review.Br J Dermatol. 2024 May 17;190(6):789-797. doi: 10.1093/bjd/ljae040. Br J Dermatol. 2024. PMID: 38330217 Review.
-
Transparency in Artificial Intelligence Reporting in Ophthalmology-A Scoping Review.Ophthalmol Sci. 2024 Jan 18;4(4):100471. doi: 10.1016/j.xops.2024.100471. eCollection 2024 Jul-Aug. Ophthalmol Sci. 2024. PMID: 38591048 Free PMC article.
-
Comparison of Chest Radiograph Interpretations by Artificial Intelligence Algorithm vs Radiology Residents.JAMA Netw Open. 2020 Oct 1;3(10):e2022779. doi: 10.1001/jamanetworkopen.2020.22779. JAMA Netw Open. 2020. PMID: 33034642 Free PMC article.
Cited by
-
Building machines that learn and think with people.Nat Hum Behav. 2024 Oct;8(10):1851-1863. doi: 10.1038/s41562-024-01991-9. Epub 2024 Oct 22. Nat Hum Behav. 2024. PMID: 39438684 Review.
-
Transformative Potential of AI in Healthcare: Definitions, Applications, and Navigating the Ethical Landscape and Public Perspectives.Healthcare (Basel). 2024 Jan 5;12(2):125. doi: 10.3390/healthcare12020125. Healthcare (Basel). 2024. PMID: 38255014 Free PMC article. Review.
-
A survey of skin tone assessment in prospective research.NPJ Digit Med. 2024 Jul 17;7(1):191. doi: 10.1038/s41746-024-01176-8. NPJ Digit Med. 2024. PMID: 39014060 Free PMC article. Review.
-
A scoping review of neurodegenerative manifestations in explainable digital phenotyping.NPJ Parkinsons Dis. 2023 Mar 30;9(1):49. doi: 10.1038/s41531-023-00494-0. NPJ Parkinsons Dis. 2023. PMID: 36997573 Free PMC article. Review.
-
Deep learning-aided decision support for diagnosis of skin disease across skin tones.Nat Med. 2024 Feb;30(2):573-583. doi: 10.1038/s41591-023-02728-3. Epub 2024 Feb 5. Nat Med. 2024. PMID: 38317019 Free PMC article.
References
-
- Holland S, Hosny A, Newman S, Joseph J, Chmielinski K. The dataset nutrition label: a framework to drive higher data quality standards. arXiv. Preprint posted online May 9, 2018. 1805.03677.
-
- Gebru T, Morgenstern J, Vecchione B, et al. Datasheets for datasets. arXiv. Preprint posted online March 19, 2020. 1803.09010.
-
- Deng J, Dong W, Socher R, Li L-J, Li K, Fei-Fei L. ImageNet: a large-scale hierarchical image database. In: 2009 IEEE Conference on Computer Vision and Pattern Recognition. IEEE; 2009:248–255.
Publication types
MeSH terms
Grants and funding
LinkOut - more resources
Full Text Sources
Miscellaneous