Modeling COVID-19 spread in small colleges
- PMID: 34407115
- PMCID: PMC8372956
- DOI: 10.1371/journal.pone.0255654
Modeling COVID-19 spread in small colleges
Abstract
We develop an agent-based model on a network meant to capture features unique to COVID-19 spread through a small residential college. We find that a safe reopening requires strong policy from administrators combined with cautious behavior from students. Strong policy includes weekly screening tests with quick turnaround and halving the campus population. Cautious behavior from students means wearing facemasks, socializing less, and showing up for COVID-19 testing. We also find that comprehensive testing and facemasks are the most effective single interventions, building closures can lead to infection spikes in other areas depending on student behavior, and faster return of test results significantly reduces total infections.
Conflict of interest statement
The authors have declared that no competing interests exist.
Figures
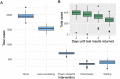
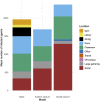
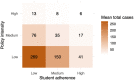
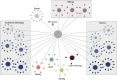
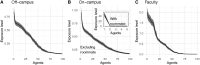
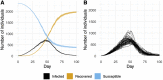
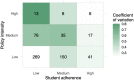
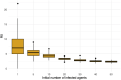
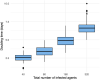
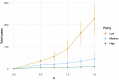
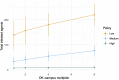
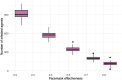
Similar articles
-
College Campuses and COVID-19 Mitigation: Clinical and Economic Value.Ann Intern Med. 2021 Apr;174(4):472-483. doi: 10.7326/M20-6558. Epub 2020 Dec 21. Ann Intern Med. 2021. PMID: 33347322 Free PMC article.
-
Assessment of a Multifaceted Approach, Including Frequent PCR Testing, to Mitigation of COVID-19 Transmission at a Residential Historically Black University.JAMA Netw Open. 2021 Dec 1;4(12):e2137189. doi: 10.1001/jamanetworkopen.2021.37189. JAMA Netw Open. 2021. PMID: 34902039 Free PMC article.
-
COVID-19 Testing and Case Rates and Social Contact Among Residential College Students in Connecticut During the 2020-2021 Academic Year.JAMA Netw Open. 2021 Dec 1;4(12):e2140602. doi: 10.1001/jamanetworkopen.2021.40602. JAMA Netw Open. 2021. PMID: 34940864 Free PMC article.
-
Physical interventions to interrupt or reduce the spread of respiratory viruses.Cochrane Database Syst Rev. 2023 Jan 30;1(1):CD006207. doi: 10.1002/14651858.CD006207.pub6. Cochrane Database Syst Rev. 2023. PMID: 36715243 Free PMC article. Review.
-
[Covid-19, september 2020: fall storm warning].Virologie (Montrouge). 2020 Dec 1;24(6):361-367. doi: 10.1684/vir.2020.0865. Virologie (Montrouge). 2020. PMID: 33252045 Review. French.
Cited by
-
Covid-19 Exposure Assessment Tool (CEAT): Easy-to-use tool to quantify exposure based on airflow, group behavior, and infection prevalence in the community.medRxiv [Preprint]. 2022 Mar 16:2022.03.02.22271806. doi: 10.1101/2022.03.02.22271806. medRxiv. 2022. Update in: Sci Adv. 2022 Sep 30;8(39):eabq0593. doi: 10.1126/sciadv.abq0593 PMID: 35291295 Free PMC article. Updated. Preprint.
-
Monitoring and responding to emerging infectious diseases in a university setting: A case study using COVID-19.PLoS One. 2023 May 17;18(5):e0280979. doi: 10.1371/journal.pone.0280979. eCollection 2023. PLoS One. 2023. PMID: 37196023 Free PMC article.
-
Information About COVID-19 Testing on College Websites in the New York City Metropolitan Area.J Community Health. 2021 Oct;46(5):887-892. doi: 10.1007/s10900-021-00970-9. Epub 2021 Feb 10. J Community Health. 2021. PMID: 33569669 Free PMC article.
-
Modeling for COVID-19 college reopening decisions: Cornell, a case study.Proc Natl Acad Sci U S A. 2022 Jan 11;119(2):e2112532119. doi: 10.1073/pnas.2112532119. Proc Natl Acad Sci U S A. 2022. PMID: 34969678 Free PMC article.
-
Estimating data-driven coronavirus disease 2019 mitigation strategies for safe university reopening.J R Soc Interface. 2022 Mar;19(188):20210920. doi: 10.1098/rsif.2021.0920. Epub 2022 Mar 14. J R Soc Interface. 2022. PMID: 35285285 Free PMC article.
References
-
- Long D, Graves D, Burton J, Kim C, Watson C, Mullen L, et al. COVID-19 Planning Guide and Self-Assessment for Higher Education. 2020.
-
- Guskiewicz K, Blouin R. Message from University leaders on important changes for Fall 2020 Roadmap;. Available from: https://www.unc.edu/posts/2020/08/17/shift-to-remote-cm/.
-
- News NDD. Notre Dame enacts two weeks of remote instruction;. Available from: https://news.nd.edu/news/notre-dame-enacts-two-weeks-of-remote-instruction/.
Publication types
MeSH terms
Grants and funding
LinkOut - more resources
Full Text Sources
Medical