Machine-learning-based children's pathological gait classification with low-cost gait-recognition system
- PMID: 34158070
- PMCID: PMC8220846
- DOI: 10.1186/s12938-021-00898-0
Machine-learning-based children's pathological gait classification with low-cost gait-recognition system
Abstract
Background: Pathological gaits of children may lead to terrible diseases, such as osteoarthritis or scoliosis. By monitoring the gait pattern of a child, proper therapeutic measures can be recommended to avoid the terrible consequence. However, low-cost systems for pathological gait recognition of children automatically have not been on market yet. Our goal was to design a low-cost gait-recognition system for children with only pressure information.
Methods: In this study, we design a pathological gait-recognition system (PGRS) with an 8 × 8 pressure-sensor array. An intelligent gait-recognition method (IGRM) based on machine learning and pure plantar pressure information is also proposed in static and dynamic sections to realize high accuracy and good real-time performance. To verifying the recognition effect, a total of 17 children were recruited in the experiments wearing PGRS to recognize three pathological gaits (toe-in, toe-out, and flat) and normal gait. Children are asked to walk naturally on level ground in the dynamic section or stand naturally and comfortably in the static section. The evaluation of the performance of recognition results included stratified tenfold cross-validation with recall, precision, and a time cost as metrics.
Results: The experimental results show that all of the IGRMs have been identified with a practically applicable degree of average accuracy either in the dynamic or static section. Experimental results indicate that the IGRM has 92.41% and 97.79% intra-subject recognition accuracy, and 85.78% and 78.81% inter-subject recognition accuracy, respectively, in the static and dynamic sections. And we find methods in the static section have less recognition accuracy due to the unnatural gesture of children when standing.
Conclusions: In this study, a low-cost PGRS has been verified and realize feasibility, highly average precision, and good real-time performance of gait recognition. The experimental results reveal the potential for the computer supervision of non-pathological and pathological gaits in the plantar-pressure patterns of children and for providing feedback in the application of gait-abnormality rectification.
Keywords: Feature extraction; Gait classification; Pathological gait recognition; Pressure-sensor array.
Conflict of interest statement
The authors declare that they have no competing interests.
Figures
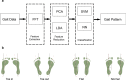
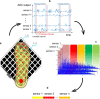
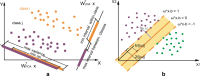
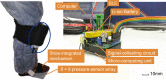
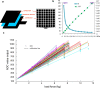
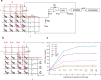
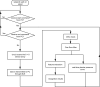
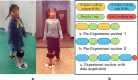
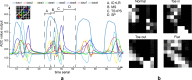
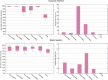
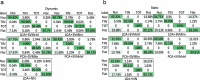
Similar articles
-
Computer Vision and Machine Learning-Based Gait Pattern Recognition for Flat Fall Prediction.Sensors (Basel). 2022 Oct 19;22(20):7960. doi: 10.3390/s22207960. Sensors (Basel). 2022. PMID: 36298311 Free PMC article.
-
Real-time feedback of dynamic foot pressure index for gait training of toe-walking children with spastic diplegia.Disabil Rehabil. 2017 Sep;39(19):1921-1925. doi: 10.1080/09638288.2016.1212114. Epub 2016 Aug 24. Disabil Rehabil. 2017. PMID: 27558231
-
[Experimental gait study based on the plantar pressure test for the young people].Sheng Wu Yi Xue Gong Cheng Xue Za Zhi. 2014 Dec;31(6):1278-82, 1293. Sheng Wu Yi Xue Gong Cheng Xue Za Zhi. 2014. PMID: 25868244 Chinese.
-
Single-Camera-Based Method for Step Length Symmetry Measurement in Unconstrained Elderly Home Monitoring.IEEE Trans Biomed Eng. 2017 Nov;64(11):2618-2627. doi: 10.1109/TBME.2017.2653246. IEEE Trans Biomed Eng. 2017. PMID: 28092516
-
Contributions to the understanding of gait control.Dan Med J. 2014 Apr;61(4):B4823. Dan Med J. 2014. PMID: 24814597 Review.
Cited by
-
Current developments of robotic hip exoskeleton toward sensing, decision, and actuation: A review.Wearable Technol. 2022 Jul 15;3:e15. doi: 10.1017/wtc.2022.11. eCollection 2022. Wearable Technol. 2022. PMID: 38486916 Free PMC article. Review.
-
Computer Vision and Machine Learning-Based Gait Pattern Recognition for Flat Fall Prediction.Sensors (Basel). 2022 Oct 19;22(20):7960. doi: 10.3390/s22207960. Sensors (Basel). 2022. PMID: 36298311 Free PMC article.
-
Flexible Machine Learning Algorithms for Clinical Gait Assessment Tools.Sensors (Basel). 2022 Jun 30;22(13):4957. doi: 10.3390/s22134957. Sensors (Basel). 2022. PMID: 35808456 Free PMC article.
-
Analysis of Gait Characteristics of Patients with Knee Arthritis Based on Human Posture Estimation.Biomed Res Int. 2022 Apr 14;2022:7020804. doi: 10.1155/2022/7020804. eCollection 2022. Biomed Res Int. 2022. Retraction in: Biomed Res Int. 2024 Mar 20;2024:9878053. doi: 10.1155/2024/9878053 PMID: 35463980 Free PMC article. Retracted.
References
MeSH terms
Grants and funding
LinkOut - more resources
Full Text Sources