Simple discrete-time self-exciting models can describe complex dynamic processes: A case study of COVID-19
- PMID: 33836020
- PMCID: PMC8034752
- DOI: 10.1371/journal.pone.0250015
Simple discrete-time self-exciting models can describe complex dynamic processes: A case study of COVID-19
Abstract
Hawkes processes are a form of self-exciting process that has been used in numerous applications, including neuroscience, seismology, and terrorism. While these self-exciting processes have a simple formulation, they can model incredibly complex phenomena. Traditionally Hawkes processes are a continuous-time process, however we enable these models to be applied to a wider range of problems by considering a discrete-time variant of Hawkes processes. We illustrate this through the novel coronavirus disease (COVID-19) as a substantive case study. While alternative models, such as compartmental and growth curve models, have been widely applied to the COVID-19 epidemic, the use of discrete-time Hawkes processes allows us to gain alternative insights. This paper evaluates the capability of discrete-time Hawkes processes by modelling daily mortality counts as distinct phases in the COVID-19 outbreak. We first consider the initial stage of exponential growth and the subsequent decline as preventative measures become effective. We then explore subsequent phases with more recent data. Various countries that have been adversely affected by the epidemic are considered, namely, Brazil, China, France, Germany, India, Italy, Spain, Sweden, the United Kingdom and the United States. These countries are all unique concerning the spread of the virus and their corresponding response measures. However, we find that this simple model is useful in accurately capturing the dynamics of the process, despite hidden interactions that are not directly modelled due to their complexity, and differences both within and between countries. The utility of this model is not confined to the current COVID-19 epidemic, rather this model could explain many other complex phenomena. It is of interest to have simple models that adequately describe these complex processes with unknown dynamics. As models become more complex, a simpler representation of the process can be desirable for the sake of parsimony.
Conflict of interest statement
The authors have declared that no competing interests exist.
Figures
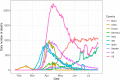
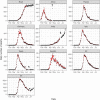
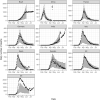
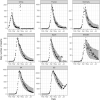
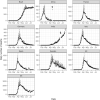
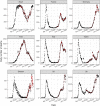
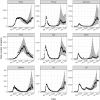
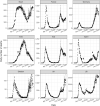
Similar articles
-
A non-parametric Hawkes model of the spread of Ebola in west Africa.J Appl Stat. 2020 Sep 26;49(3):621-637. doi: 10.1080/02664763.2020.1825646. eCollection 2022. J Appl Stat. 2020. PMID: 35706773 Free PMC article.
-
Travel-related control measures to contain the COVID-19 pandemic: a rapid review.Cochrane Database Syst Rev. 2020 Oct 5;10:CD013717. doi: 10.1002/14651858.CD013717. Cochrane Database Syst Rev. 2020. Update in: Cochrane Database Syst Rev. 2021 Mar 25;3:CD013717. doi: 10.1002/14651858.CD013717.pub2 PMID: 33502002 Updated.
-
Modelling the initial epidemic trends of COVID-19 in Italy, Spain, Germany, and France.PLoS One. 2020 Nov 9;15(11):e0241743. doi: 10.1371/journal.pone.0241743. eCollection 2020. PLoS One. 2020. PMID: 33166344 Free PMC article.
-
A time-modulated Hawkes process to model the spread of COVID-19 and the impact of countermeasures.Annu Rev Control. 2021;51:551-563. doi: 10.1016/j.arcontrol.2021.02.002. Epub 2021 Mar 12. Annu Rev Control. 2021. PMID: 33746561 Free PMC article. Review.
-
Authors' response: Occupation and SARS-CoV-2 infection risk among workers during the first pandemic wave in Germany: potential for bias.Scand J Work Environ Health. 2022 Sep 1;48(7):588-590. doi: 10.5271/sjweh.4061. Epub 2022 Sep 25. Scand J Work Environ Health. 2022. PMID: 36153787 Free PMC article. Review.
Cited by
-
Using a latent Hawkes process for epidemiological modelling.PLoS One. 2023 Mar 1;18(3):e0281370. doi: 10.1371/journal.pone.0281370. eCollection 2023. PLoS One. 2023. PMID: 36857340 Free PMC article.
-
Networks of necessity: Simulating COVID-19 mitigation strategies for disabled people and their caregivers.PLoS Comput Biol. 2022 May 18;18(5):e1010042. doi: 10.1371/journal.pcbi.1010042. eCollection 2022 May. PLoS Comput Biol. 2022. PMID: 35584133 Free PMC article.
References
-
- World Health Organisation. Weekly Epidemiological Update for Coronavirus disease 2019 (COVID-19)—9 March 2021; 2021. https://www.who.int/docs/default-source/coronaviruse/situation-reports/2....
-
- Plank MJ, Binny RN, Hendy SC, Lustig A, James A, Steyn N. A stochastic model for COVID-19 spread and the effects of Alert Level 4 in Aotearoa New Zealand. medRxiv. 2020;.
MeSH terms
Grants and funding
LinkOut - more resources
Full Text Sources
Other Literature Sources
Medical
Research Materials