Enhancing Personalized Ads Using Interest Category Classification of SNS Users Based on Deep Neural Networks
- PMID: 33396796
- PMCID: PMC7795211
- DOI: 10.3390/s21010199
Enhancing Personalized Ads Using Interest Category Classification of SNS Users Based on Deep Neural Networks
Abstract
The classification and recommendation system for identifying social networking site (SNS) users' interests plays a critical role in various industries, particularly advertising. Personalized advertisements help brands stand out from the clutter of online advertisements while enhancing relevance to consumers to generate favorable responses. Although most user interest classification studies have focused on textual data, the combined analysis of images and texts on user-generated posts can more precisely predict a consumer's interests. Therefore, this research classifies SNS users' interests by utilizing both texts and images. Consumers' interests were defined using the Curlie directory, and various convolutional neural network (CNN)-based models and recurrent neural network (RNN)-based models were tested for our user interest classification system. In our hybrid neural network (NN) model, CNN-based classification models were used to classify images from users' SNS postings while RNN-based classification models were used to classify textual data. The results of our extensive experiments show that the classification of users' interests performed best when using texts and images together, at 96.55%, versus texts only, 41.38%, or images only, 93.1%. Our proposed system provides insights into personalized SNS advertising research and informs marketers on making (1) interest-based recommendations, (2) ranked-order recommendations, and (3) real-time recommendations.
Keywords: SNS; deep learning; interest classification; neural networks; personalized ads.
Conflict of interest statement
The authors declare no conflict of interest.
Figures
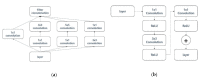
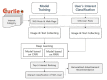
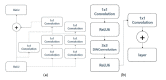
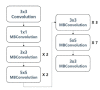
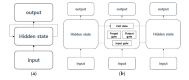
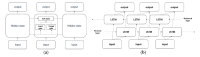
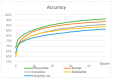
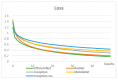
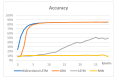
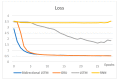
Similar articles
-
Multilevel Classification of Users' Needs in Chinese Online Medical and Health Communities: Model Development and Evaluation Based on Graph Convolutional Network.JMIR Form Res. 2023 Apr 20;7:e42297. doi: 10.2196/42297. JMIR Form Res. 2023. PMID: 37079346 Free PMC article.
-
Health Advertising on Short-Video Social Media: AStudy on User Attitudes Based on the ExtendedTechnology Acceptance Model.Int J Environ Res Public Health. 2020 Feb 26;17(5):1501. doi: 10.3390/ijerph17051501. Int J Environ Res Public Health. 2020. PMID: 32110950 Free PMC article.
-
Social Network Analysis of an Online Smoking Cessation Community to Identify Users' Smoking Status.Healthc Inform Res. 2021 Apr;27(2):116-126. doi: 10.4258/hir.2021.27.2.116. Epub 2021 Apr 30. Healthc Inform Res. 2021. PMID: 34015877 Free PMC article.
-
Knowledge-Aware Multispace Embedding Learning for Personalized Recommendation.Sensors (Basel). 2022 Mar 12;22(6):2212. doi: 10.3390/s22062212. Sensors (Basel). 2022. PMID: 35336383 Free PMC article.
-
Personal Interest Attention Graph Neural Networks for Session-Based Recommendation.Entropy (Basel). 2021 Nov 12;23(11):1500. doi: 10.3390/e23111500. Entropy (Basel). 2021. PMID: 34828197 Free PMC article.
Cited by
-
A Novel Hybrid Multi-Modal Deep Learning for Detecting Hashtag Incongruity on Social Media.Sensors (Basel). 2022 Dec 15;22(24):9870. doi: 10.3390/s22249870. Sensors (Basel). 2022. PMID: 36560237 Free PMC article.
-
Analysis of e-Mail Spam Detection Using a Novel Machine Learning-Based Hybrid Bagging Technique.Comput Intell Neurosci. 2022 Aug 9;2022:2500772. doi: 10.1155/2022/2500772. eCollection 2022. Comput Intell Neurosci. 2022. PMID: 35983156 Free PMC article.
-
Hardware implementation of memristor-based artificial neural networks.Nat Commun. 2024 Mar 4;15(1):1974. doi: 10.1038/s41467-024-45670-9. Nat Commun. 2024. PMID: 38438350 Free PMC article. Review.
-
HyAdamC: A New Adam-Based Hybrid Optimization Algorithm for Convolution Neural Networks.Sensors (Basel). 2021 Jun 12;21(12):4054. doi: 10.3390/s21124054. Sensors (Basel). 2021. PMID: 34204695 Free PMC article.
References
-
- Voorveld H. Brand communication in social media: A research agenda. J. Advert. 2019;48:14–26. doi: 10.1080/00913367.2019.1588808. - DOI
-
- Ashley C., Tracy T. Creative Strategies in Social Media Marketing: An Exploratory Study of Branded Social Content and Consumer Engagement. Psychol. Mark. 2015;32:15–27. doi: 10.1002/mar.20761. - DOI
-
- Gritten A. Forum-Media Proliferation and Demands for New Forms of Research. Int. J. Mark. Res. 2007;49:15–23. doi: 10.1177/147078530704900105. - DOI
-
- Kelly L., Kerr G., Drennan J. Avoidance of advertising in social networking sites: The teenage perspective. J. Interact. Advert. 2010;10:16–27. doi: 10.1080/15252019.2010.10722167. - DOI
-
- De Keyzer F., Dens N., De Pelsmacker P. Is this for me? How consumers respond to personalized advertising on social network sites. J. Interact. Advert. 2015;15:124–134. doi: 10.1080/15252019.2015.1082450. - DOI
Grants and funding
LinkOut - more resources
Full Text Sources
Other Literature Sources