Nondestructive Classification of Soybean Seed Varieties by Hyperspectral Imaging and Ensemble Machine Learning Algorithms
- PMID: 33297289
- PMCID: PMC7731448
- DOI: 10.3390/s20236980
Nondestructive Classification of Soybean Seed Varieties by Hyperspectral Imaging and Ensemble Machine Learning Algorithms
Abstract
During the processing and planting of soybeans, it is greatly significant that a reliable, rapid, and accurate technique is used to detect soybean varieties. Traditional chemical analysis methods of soybean variety sampling (e.g., mass spectrometry and high-performance liquid chromatography) are destructive and time-consuming. In this paper, a robust and accurate method for nondestructive soybean classification is developed through hyperspectral imaging and ensemble machine learning algorithms. Image acquisition, preprocessing, and feature selection are used to obtain different types of soybean hyperspectral features. Based on these features, one of ensemble classifiers-random subspace linear discriminant (RSLD) algorithm is used to classify soybean seeds. Compared with the linear discrimination (LD) and linear support vector machine (LSVM) methods, the results show that the RSLD algorithm in this paper is more stable and reliable. In classifying soybeans in 10, 15, 20, and 25 categories, the RSLD method achieves the highest classification accuracy. When 155 features are used to classify 15 types of soybeans, the classification accuracy of the RSLD method reaches 99.2%, while the classification accuracies of the LD and LSVM methods are only 98.6% and 69.7%, respectively. Therefore, the ensemble classification algorithm RSLD can maintain high classification accuracy when different types and different classification features are used.
Keywords: correlation coefficient matrix; ensemble machine learning algorithms; hyperspectral imaging; random subspace linear discriminant.
Conflict of interest statement
The authors declare no conflict of interest.
Figures
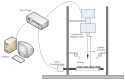
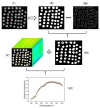
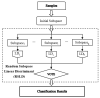
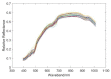
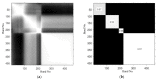
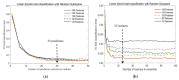
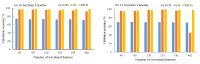
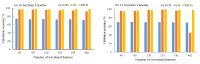
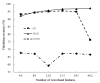
Similar articles
-
Nondestructive Classification of Maize Moldy Seeds by Hyperspectral Imaging and Optimal Machine Learning Algorithms.Sensors (Basel). 2022 Aug 13;22(16):6064. doi: 10.3390/s22166064. Sensors (Basel). 2022. PMID: 36015825 Free PMC article.
-
Identification of heat damage in imported soybeans based on hyperspectral imaging technology.J Sci Food Agric. 2020 Mar 15;100(4):1775-1786. doi: 10.1002/jsfa.10214. Epub 2020 Jan 13. J Sci Food Agric. 2020. PMID: 31849057
-
Rapid and nondestructive watermelon (Citrullus lanatus) seed viability detection based on visible near-infrared hyperspectral imaging technology and machine learning algorithms.J Food Sci. 2024 Jul;89(7):4403-4418. doi: 10.1111/1750-3841.17151. Epub 2024 Jun 21. J Food Sci. 2024. PMID: 38957090
-
A review of the medical hyperspectral imaging systems and unmixing algorithms' in biological tissues.Photodiagnosis Photodyn Ther. 2021 Mar;33:102165. doi: 10.1016/j.pdpdt.2020.102165. Epub 2020 Dec 28. Photodiagnosis Photodyn Ther. 2021. PMID: 33383204 Review.
-
Machine learning techniques for analysis of hyperspectral images to determine quality of food products: A review.Curr Res Food Sci. 2021 Feb 3;4:28-44. doi: 10.1016/j.crfs.2021.01.002. eCollection 2021. Curr Res Food Sci. 2021. PMID: 33659896 Free PMC article. Review.
Cited by
-
A non-destructive testing method for early detection of ginseng root diseases using machine learning technologies based on leaf hyperspectral reflectance.Front Plant Sci. 2022 Nov 17;13:1031030. doi: 10.3389/fpls.2022.1031030. eCollection 2022. Front Plant Sci. 2022. PMID: 36466253 Free PMC article.
-
Recognition of maize seed varieties based on hyperspectral imaging technology and integrated learning algorithms.PeerJ Comput Sci. 2023 May 10;9:e1354. doi: 10.7717/peerj-cs.1354. eCollection 2023. PeerJ Comput Sci. 2023. PMID: 37346683 Free PMC article.
-
Dropout Deep Belief Network Based Chinese Ancient Ceramic Non-Destructive Identification.Sensors (Basel). 2021 Feb 12;21(4):1318. doi: 10.3390/s21041318. Sensors (Basel). 2021. PMID: 33673248 Free PMC article.
-
Soybean cyst nematode detection and management: a review.Plant Methods. 2022 Sep 7;18(1):110. doi: 10.1186/s13007-022-00933-8. Plant Methods. 2022. PMID: 36071455 Free PMC article. Review.
-
Classification of multi-year and multi-variety pumpkin seeds using hyperspectral imaging technology and three-dimensional convolutional neural network.Plant Methods. 2023 Aug 10;19(1):82. doi: 10.1186/s13007-023-01057-3. Plant Methods. 2023. PMID: 37563698 Free PMC article.
References
-
- Corassa G.M., Santi A.L., Amado T.J.C., Reimche G.B., Gaviraghi R., Bisognin M.B., Pires J.L.F. Performance of soybean varieties differs according to yield class: A case study from Southern Brazil. Precis. Agric. 2019;20:520–540. doi: 10.1007/s11119-018-9595-0. - DOI
-
- Ye S., Wang Y., Huang D.-Q., Li J., Gong Y., Xu L., Liu L. Genetic purity test of F1 hybrid seed with molecular markers in cabbage (Brassica oleracea var. capitata) Sci. Hortic. 2013;155:92–96. doi: 10.1016/j.scienta.2013.03.016. - DOI
-
- Rao P.S., Bharathi M., Reddy K.B., Keshavulu K., Rao L.V.S., Neeraja C.N. Varietal identification in rice (Oryza sativa) through chemical tests and gel electrophoresis of soluble seed proteins. Indian J. Agric. Sci. 2012;82:304–311.
Publication types
MeSH terms
Grants and funding
LinkOut - more resources
Full Text Sources
Research Materials