Evaluating the effect of demographic factors, socioeconomic factors, and risk aversion on mobility during the COVID-19 epidemic in France under lockdown: a population-based study
- PMID: 33163951
- PMCID: PMC7598368
- DOI: 10.1016/S2589-7500(20)30243-0
Evaluating the effect of demographic factors, socioeconomic factors, and risk aversion on mobility during the COVID-19 epidemic in France under lockdown: a population-based study
Abstract
Background: On March 17, 2020, French authorities implemented a nationwide lockdown to respond to the COVID-19 epidemic and curb the surge of patients requiring critical care. Assessing the effect of lockdown on individual displacements is essential to quantify achievable mobility reductions and identify the factors driving the changes in social dynamics that affected viral diffusion. We aimed to use mobile phone data to study how mobility in France changed before and during lockdown, breaking down our findings by trip distance, user age and residency, and time of day, and analysing regional data and spatial heterogeneities.
Methods: For this population-based study, we used temporally resolved travel flows among 1436 administrative areas of mainland France reconstructed from mobile phone trajectories. Data were stratified by age class (younger than 18 years, 18-64 years, and 65 years or older). We distinguished between residents and non-residents and used population data and regional socioeconomic indicators from the French National Statistical Institute. We measured mobility changes before and during lockdown at both local and country scales using a case-crossover framework. We analysed all trips combined and trips longer than 100 km (termed long trips), and separated trips by daytime or night-time, weekdays or weekends, and rush hours.
Findings: Lockdown caused a 65% reduction in the countrywide number of displacements (from about 57 million to about 20 million trips per day) and was particularly effective in reducing work-related short-range mobility, especially during rush hour, and long trips. Geographical heterogeneities showed anomalous increases in long-range movements even before lockdown announcement that were tightly localised in space. During lockdown, mobility drops were unevenly distributed across regions (eg, Île-de-France, the region of Paris, went from 585 000 to 117 000 outgoing trips per day). They were strongly associated with active populations, workers employed in sectors highly affected by lockdown, and number of hospitalisations per region, and moderately associated with the socioeconomic level of the regions. Major cities largely shrank their pattern of connectivity, reducing it mainly to short-range commuting (95% of traffic leaving Paris was contained in a 201 km radius before lockdown, which was reduced to 29 km during lockdown).
Interpretation: Lockdown was effective in reducing population mobility across scales. Caution should be taken in the timing of policy announcements and implementation, because anomalous mobility followed policy announcements, which might act as seeding events. Conversely, risk aversion might be beneficial in further decreasing mobility in highly affected regions. We also identified socioeconomic and demographic constraints to the efficacy of restrictions. The unveiled links between geography, demography, and timing of the response to mobility restrictions might help to design interventions that minimise invasiveness while contributing to the current epidemic response.
Funding: Agence Nationale de la Recherche, EU, REACTing.
© 2020 The Author(s). Published by Elsevier Ltd. This is an Open Access article under the CC BY-NC-ND 4.0 license.
Figures
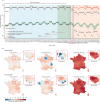
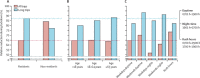
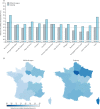
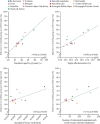
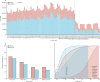
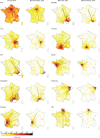
Similar articles
-
Factors affecting COVID-19 infected and death rates inform lockdown-related policymaking.PLoS One. 2020 Oct 23;15(10):e0241165. doi: 10.1371/journal.pone.0241165. eCollection 2020. PLoS One. 2020. PMID: 33095811 Free PMC article.
-
Does city lockdown prevent the spread of COVID-19? New evidence from the synthetic control method.Glob Health Res Policy. 2021 Jul 1;6(1):20. doi: 10.1186/s41256-021-00204-4. Glob Health Res Policy. 2021. PMID: 34193312 Free PMC article.
-
Lockdowns result in changes in human mobility which may impact the epidemiologic dynamics of SARS-CoV-2.Sci Rep. 2021 Mar 26;11(1):6995. doi: 10.1038/s41598-021-86297-w. Sci Rep. 2021. PMID: 33772076 Free PMC article.
-
Social inequalities in mobility during and following the COVID-19 associated lockdown of the Madrid metropolitan area in Spain.Health Place. 2021 Jul;70:102580. doi: 10.1016/j.healthplace.2021.102580. Epub 2021 May 19. Health Place. 2021. PMID: 34022543 Free PMC article. Review.
-
Addictovigilance contribution during COVID-19 epidemic and lockdown in France.Therapie. 2020 Jul-Aug;75(4):343-354. doi: 10.1016/j.therap.2020.06.006. Epub 2020 Jun 23. Therapie. 2020. PMID: 32660776 Free PMC article. Review.
Cited by
-
Anatomy of digital contact tracing: Role of age, transmission setting, adoption, and case detection.Sci Adv. 2021 Apr 9;7(15):eabd8750. doi: 10.1126/sciadv.abd8750. Print 2021 Apr. Sci Adv. 2021. PMID: 33712416 Free PMC article.
-
Evaluating the potential of suburban and rural areas for tourism and recreation, including individual short-term tourism under pandemic conditions.Sci Rep. 2022 Nov 27;12(1):20369. doi: 10.1038/s41598-022-24503-z. Sci Rep. 2022. PMID: 36437259 Free PMC article.
-
Modeling future spread of infections via mobile geolocation data and population dynamics. An application to COVID-19 in Brazil.PLoS One. 2020 Jul 16;15(7):e0235732. doi: 10.1371/journal.pone.0235732. eCollection 2020. PLoS One. 2020. PMID: 32673323 Free PMC article.
-
Population-scale dietary interests during the COVID-19 pandemic.Nat Commun. 2022 Feb 28;13(1):1073. doi: 10.1038/s41467-022-28498-z. Nat Commun. 2022. PMID: 35228539 Free PMC article.
-
COVID-19 lockdown policy and heterogeneous responses of urban mobility: Evidence from the Philippines.PLoS One. 2022 Jun 30;17(6):e0270555. doi: 10.1371/journal.pone.0270555. eCollection 2022. PLoS One. 2022. PMID: 35771739 Free PMC article.
References
-
- Di Domenico L, Pullano G, Sabbatini CE, Boëlle P-Y, Colizza V. Expected impact of reopening schools after lockdown on COVID-19 epidemic in Île-de-France. medRxiv. 2020 https://www.medrxiv.org/content/10.1101/2020.05.08.20095521v1 published online May 12. (preprint) - DOI - PMC - PubMed
Publication types
MeSH terms
LinkOut - more resources
Full Text Sources
Medical