The state of artificial intelligence-based FDA-approved medical devices and algorithms: an online database
- PMID: 32984550
- PMCID: PMC7486909
- DOI: 10.1038/s41746-020-00324-0
The state of artificial intelligence-based FDA-approved medical devices and algorithms: an online database
Abstract
At the beginning of the artificial intelligence (AI)/machine learning (ML) era, the expectations are high, and experts foresee that AI/ML shows potential for diagnosing, managing and treating a wide variety of medical conditions. However, the obstacles for implementation of AI/ML in daily clinical practice are numerous, especially regarding the regulation of these technologies. Therefore, we provide an insight into the currently available AI/ML-based medical devices and algorithms that have been approved by the US Food & Drugs Administration (FDA). We aimed to raise awareness of the importance of regulatory bodies, clearly stating whether a medical device is AI/ML based or not. Cross-checking and validating all approvals, we identified 64 AI/ML based, FDA approved medical devices and algorithms. Out of those, only 29 (45%) mentioned any AI/ML-related expressions in the official FDA announcement. The majority (85.9%) was approved by the FDA with a 510(k) clearance, while 8 (12.5%) received de novo pathway clearance and one (1.6%) premarket approval (PMA) clearance. Most of these technologies, notably 30 (46.9%), 16 (25.0%), and 10 (15.6%) were developed for the fields of Radiology, Cardiology and Internal Medicine/General Practice respectively. We have launched the first comprehensive and open access database of strictly AI/ML-based medical technologies that have been approved by the FDA. The database will be constantly updated.
Keywords: Health services; Outcomes research.
© The Author(s) 2020.
Conflict of interest statement
Competing interestsThe authors declare no competing interests.
Figures
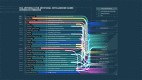
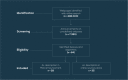
Similar articles
-
The 2021 landscape of FDA-approved artificial intelligence/machine learning-enabled medical devices: An analysis of the characteristics and intended use.Int J Med Inform. 2022 Sep;165:104828. doi: 10.1016/j.ijmedinf.2022.104828. Epub 2022 Jul 1. Int J Med Inform. 2022. PMID: 35780651
-
Trends in clinical validation and usage of US Food and Drug Administration-cleared artificial intelligence algorithms for medical imaging.Clin Radiol. 2023 Feb;78(2):123-129. doi: 10.1016/j.crad.2022.09.122. Epub 2022 Oct 28. Clin Radiol. 2023. PMID: 36625218
-
Artificial intelligence and remote patient monitoring in US healthcare market: a literature review.J Mark Access Health Policy. 2023 May 3;11(1):2205618. doi: 10.1080/20016689.2023.2205618. eCollection 2023. J Mark Access Health Policy. 2023. PMID: 37151736 Free PMC article.
-
Approval of artificial intelligence and machine learning-based medical devices in the USA and Europe (2015-20): a comparative analysis.Lancet Digit Health. 2021 Mar;3(3):e195-e203. doi: 10.1016/S2589-7500(20)30292-2. Epub 2021 Jan 18. Lancet Digit Health. 2021. PMID: 33478929 Review.
-
FDA-cleared artificial intelligence and machine learning-based medical devices and their 510(k) predicate networks.Lancet Digit Health. 2023 Sep;5(9):e618-e626. doi: 10.1016/S2589-7500(23)00126-7. Lancet Digit Health. 2023. PMID: 37625896 Review.
Cited by
-
Systematic analysis of the test design and performance of AI/ML-based medical devices approved for triage/detection/diagnosis in the USA and Japan.Sci Rep. 2022 Oct 7;12(1):16874. doi: 10.1038/s41598-022-21426-7. Sci Rep. 2022. PMID: 36207474 Free PMC article. Clinical Trial.
-
Algorithmovigilance, lessons from pharmacovigilance.NPJ Digit Med. 2024 Oct 2;7(1):270. doi: 10.1038/s41746-024-01237-y. NPJ Digit Med. 2024. PMID: 39358559 Free PMC article. Review.
-
Introducing AI to the molecular tumor board: one direction toward the establishment of precision medicine using large-scale cancer clinical and biological information.Exp Hematol Oncol. 2022 Oct 31;11(1):82. doi: 10.1186/s40164-022-00333-7. Exp Hematol Oncol. 2022. PMID: 36316731 Free PMC article. Review.
-
Assessment of blood perfusion quality in laparoscopic colorectal surgery by means of Machine Learning.Sci Rep. 2022 Aug 29;12(1):14682. doi: 10.1038/s41598-022-16030-8. Sci Rep. 2022. PMID: 36038561 Free PMC article.
-
Artificial Intelligence in Obstetrics and Gynecology: Transforming Care and Outcomes.Cureus. 2024 Jul 17;16(7):e64725. doi: 10.7759/cureus.64725. eCollection 2024 Jul. Cureus. 2024. PMID: 39156405 Free PMC article. Review.
References
-
- Wang, X. et al. Chest X-ray: hospital-scale chest X-ray database and benchmarks on weakly supervised classification and localization of common thorax diseases. In: Advances in Computer Vision and Pattern Recognition, Springer (2019).
LinkOut - more resources
Full Text Sources