The challenges of modeling and forecasting the spread of COVID-19
- PMID: 32616574
- PMCID: PMC7382213
- DOI: 10.1073/pnas.2006520117
The challenges of modeling and forecasting the spread of COVID-19
Abstract
The coronavirus disease 2019 (COVID-19) pandemic has placed epidemic modeling at the forefront of worldwide public policy making. Nonetheless, modeling and forecasting the spread of COVID-19 remains a challenge. Here, we detail three regional-scale models for forecasting and assessing the course of the pandemic. This work demonstrates the utility of parsimonious models for early-time data and provides an accessible framework for generating policy-relevant insights into its course. We show how these models can be connected to each other and to time series data for a particular region. Capable of measuring and forecasting the impacts of social distancing, these models highlight the dangers of relaxing nonpharmaceutical public health interventions in the absence of a vaccine or antiviral therapies.
Keywords: COVID-19; branching process; compartmental models; pandemic.
Copyright © 2020 the Author(s). Published by PNAS.
Conflict of interest statement
The authors declare no competing interest.
Figures
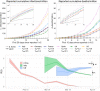
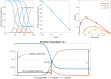
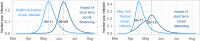
Similar articles
-
A vision for actionable science in a pandemic.Nat Commun. 2020 Sep 30;11(1):4960. doi: 10.1038/s41467-020-18056-w. Nat Commun. 2020. PMID: 32999293 Free PMC article.
-
Strategies and recommendations for the safe implementation of vascular surgery during the pandemic period of novel coronavirus pneumonia.J Vasc Surg. 2020 Jul;72(1):377-378. doi: 10.1016/j.jvs.2020.04.466. Epub 2020 Apr 22. J Vasc Surg. 2020. PMID: 32334048 Free PMC article. No abstract available.
-
Covid-19 exposes the gaps in infection prevention and control.Infect Dis Health. 2020 Nov;25(4):223-226. doi: 10.1016/j.idh.2020.08.005. Epub 2020 Aug 19. Infect Dis Health. 2020. PMID: 32863200 Free PMC article.
-
A Practical Approach to the Management of Cancer Patients During the Novel Coronavirus Disease 2019 (COVID-19) Pandemic: An International Collaborative Group.Oncologist. 2020 Jun;25(6):e936-e945. doi: 10.1634/theoncologist.2020-0213. Epub 2020 Apr 27. Oncologist. 2020. PMID: 32243668 Free PMC article. Review.
-
The Pathogenesis of Coronavirus Disease 2019 (COVID-19): Evaluation and Prevention.J Immunol Res. 2020 Jul 10;2020:1357983. doi: 10.1155/2020/1357983. eCollection 2020. J Immunol Res. 2020. PMID: 32671115 Free PMC article. Review.
Cited by
-
Estimating, monitoring, and forecasting COVID-19 epidemics: a spatiotemporal approach applied to NYC data.Sci Rep. 2021 Apr 27;11(1):9089. doi: 10.1038/s41598-021-88281-w. Sci Rep. 2021. PMID: 33907222 Free PMC article.
-
Simple discrete-time self-exciting models can describe complex dynamic processes: A case study of COVID-19.PLoS One. 2021 Apr 9;16(4):e0250015. doi: 10.1371/journal.pone.0250015. eCollection 2021. PLoS One. 2021. PMID: 33836020 Free PMC article.
-
Competing control scenarios in probabilistic SIR epidemics on social-contact networks.Ann Oper Res. 2022 Oct 19:1-24. doi: 10.1007/s10479-022-05031-5. Online ahead of print. Ann Oper Res. 2022. PMID: 36281317 Free PMC article.
-
Predicting COVID-19 pandemic waves including vaccination data with deep learning.Front Public Health. 2023 Dec 15;11:1279364. doi: 10.3389/fpubh.2023.1279364. eCollection 2023. Front Public Health. 2023. PMID: 38162619 Free PMC article.
-
Multiscale heterogeneous optimal lockdown control for COVID-19 using geographic information.Sci Rep. 2022 Mar 10;12(1):3970. doi: 10.1038/s41598-022-07692-5. Sci Rep. 2022. PMID: 35273215 Free PMC article.
References
-
- Phelan A. L., Katz R., Gostin L. O., The novel coronavirus originating in Wuhan, China: Challenges for global health governance. JAMA 323, 709–710 (2020). - PubMed
-
- Landler M., Castle S., Behind the virus report that jarred the U.S. and the U.K. to action. NY Times, 17 March 2020. https://www.nytimes.com/2020/03/17/world/europe/coronavirus-imperial-col.... Accessed 24 March 2020.
-
- Imai N., et al. , “Transmissibility of 2019-nCoV” (Report 3, Imperial College London, London, United Kingdom, 2020; 10.25561/77148). - DOI
Publication types
MeSH terms
LinkOut - more resources
Full Text Sources
Medical