Person Re-Identification Using Deep Modeling of Temporally Correlated Inertial Motion Patterns
- PMID: 32050728
- PMCID: PMC7039239
- DOI: 10.3390/s20030949
Person Re-Identification Using Deep Modeling of Temporally Correlated Inertial Motion Patterns
Abstract
Person re-identification (re-ID) is among the essential components that play an integral role in constituting an automated surveillance environment. Majorly, the problem is tackled using data acquired from vision sensors using appearance-based features, which are strongly dependent on visual cues such as color, texture, etc., consequently limiting the precise re-identification of an individual. To overcome such strong dependence on visual features, many researchers have tackled the re-identification problem using human gait, which is believed to be unique and provide a distinctive biometric signature that is particularly suitable for re-ID in uncontrolled environments. However, image-based gait analysis often fails to extract quality measurements of an individual's motion patterns owing to problems related to variations in viewpoint, illumination (daylight), clothing, worn accessories, etc. To this end, in contrast to relying on image-based motion measurement, this paper demonstrates the potential to re-identify an individual using inertial measurements units (IMU) based on two common sensors, namely gyroscope and accelerometer. The experiment was carried out over data acquired using smartphones and wearable IMUs from a total of 86 randomly selected individuals including 49 males and 37 females between the ages of 17 and 72 years. The data signals were first segmented into single steps and strides, which were separately fed to train a sequential deep recurrent neural network to capture implicit arbitrary long-term temporal dependencies. The experimental setup was devised in a fashion to train the network on all the subjects using data related to half of the step and stride sequences only while the inference was performed on the remaining half for the purpose of re-identification. The obtained experimental results demonstrate the potential to reliably and accurately re-identify an individual based on one's inertial sensor data.
Keywords: deep learning; gait-based person re-ID; human re-identification; human-gait analysis; inertial sensors; inertial-based person re-identification.
Conflict of interest statement
The authors declare no conflict of interest.
Figures
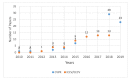
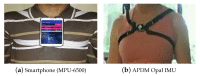
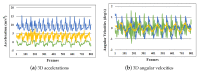
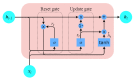
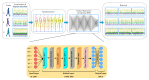
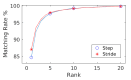
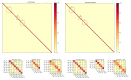
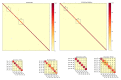
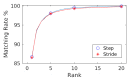
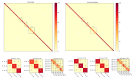
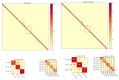
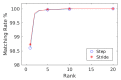
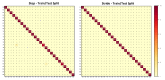
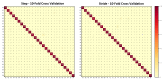
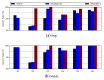
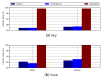
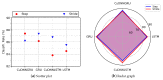
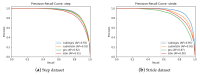
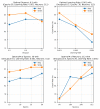
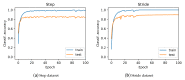
Similar articles
-
IMU-Based Gait Recognition Using Convolutional Neural Networks and Multi-Sensor Fusion.Sensors (Basel). 2017 Nov 27;17(12):2735. doi: 10.3390/s17122735. Sensors (Basel). 2017. PMID: 29186887 Free PMC article.
-
IMU-to-Segment Assignment and Orientation Alignment for the Lower Body Using Deep Learning.Sensors (Basel). 2018 Jan 19;18(1):302. doi: 10.3390/s18010302. Sensors (Basel). 2018. PMID: 29351262 Free PMC article.
-
Identification of Gait Motion Patterns Using Wearable Inertial Sensor Network.Sensors (Basel). 2019 Nov 18;19(22):5024. doi: 10.3390/s19225024. Sensors (Basel). 2019. PMID: 31752136 Free PMC article.
-
Inertial Sensor-Based Gait Recognition: A Review.Sensors (Basel). 2015 Sep 2;15(9):22089-127. doi: 10.3390/s150922089. Sensors (Basel). 2015. PMID: 26340634 Free PMC article. Review.
-
Person Re-Identification with RGB-D and RGB-IR Sensors: A Comprehensive Survey.Sensors (Basel). 2023 Jan 29;23(3):1504. doi: 10.3390/s23031504. Sensors (Basel). 2023. PMID: 36772548 Free PMC article. Review.
Cited by
-
An Acceleration Based Fusion of Multiple Spatiotemporal Networks for Gait Phase Detection.Int J Environ Res Public Health. 2020 Aug 5;17(16):5633. doi: 10.3390/ijerph17165633. Int J Environ Res Public Health. 2020. PMID: 32764244 Free PMC article.
-
Characterizing Human Box-Lifting Behavior Using Wearable Inertial Motion Sensors.Sensors (Basel). 2020 Apr 18;20(8):2323. doi: 10.3390/s20082323. Sensors (Basel). 2020. PMID: 32325739 Free PMC article.
-
Generisch-Net: A Generic Deep Model for Analyzing Human Motion with Wearable Sensors in the Internet of Health Things.Sensors (Basel). 2024 Sep 24;24(19):6167. doi: 10.3390/s24196167. Sensors (Basel). 2024. PMID: 39409207 Free PMC article.
-
Person Re-ID by Fusion of Video Silhouettes and Wearable Signals for Home Monitoring Applications.Sensors (Basel). 2020 May 1;20(9):2576. doi: 10.3390/s20092576. Sensors (Basel). 2020. PMID: 32369960 Free PMC article.
-
Bodyprint-A Meta-Feature Based LSTM Hashing Model for Person Re-Identification.Sensors (Basel). 2020 Sep 18;20(18):5365. doi: 10.3390/s20185365. Sensors (Basel). 2020. PMID: 32962168 Free PMC article.
References
-
- Nambiar A., Bernardino A., Nascimento J.C. Gait-based Person Re-identification: A Survey. ACM Comput. Surv. (CSUR) 2019;52:33. doi: 10.1145/3243043. - DOI
-
- Zheng L., Yang Y., Hauptmann A.G. Person Re-identification: Past, Present and Future. arXiv. 20161610.02984
-
- Perwaiz N., Fraz M.M., Shahzad M. Person re-identification using hybrid representation reinforced by metric learning. IEEE Access. 2018;6:77334–77349. doi: 10.1109/ACCESS.2018.2882254. - DOI
-
- Sun Y., Xu Q., Li Y., Zhang C., Li Y., Wang S., Sun J. Perceive Where to Focus: Learning Visibility-aware Part-level Features for Partial Person Re-identification; Proceedings of the IEEE Conference on Computer Vision and Pattern Recognition; Long Beach, CA, USA. 16–20 June 2019; pp. 393–402.
-
- Liu J., Zha Z.J., Chen D., Hong R., Wang M. Adaptive Transfer Network for Cross-Domain Person Re-Identification; Proceedings of the IEEE Conference on Computer Vision and Pattern Recognition (CVPR); Long Beach, CA, USA. 16–20 June 2019.
LinkOut - more resources
Full Text Sources