City-Wide Traffic Flow Forecasting Using a Deep Convolutional Neural Network
- PMID: 31940830
- PMCID: PMC7014408
- DOI: 10.3390/s20020421
City-Wide Traffic Flow Forecasting Using a Deep Convolutional Neural Network
Abstract
City-wide traffic flow forecasting is a significant function of the Intelligent Transport System (ITS), which plays an important role in city traffic management and public travel safety. However, this remains a very challenging task that is affected by many complex factors, such as road network distribution and external factors (e.g., weather, accidents, and holidays). In this paper, we propose a deep-learning-based multi-branch model called TFFNet (Traffic Flow Forecasting Network) to forecast the short-term traffic status (flow) throughout a city. The model uses spatiotemporal traffic flow matrices and external factors as its input and then infers and outputs the future short-term traffic status (flow) of the whole road network. For modelling the spatial correlations of the traffic flows between current and adjacent road segments, we employ a multi-layer fully convolutional framework to perform cross-correlation calculation and extract the hierarchical spatial dependencies from local to global scales. Also, we extract the temporal closeness and periodicity of traffic flow from historical observations by constructing a high-dimensional tensor comprised of traffic flow matrices from three fragments of the time axis: recent time, near history, and distant history. External factors are also considered and trained with a fully connected neural network and then fused with the output of the main component of TFFNet. The multi-branch model is automatically trained to fit complex patterns hidden in the traffic flow matrices until reaching pre-defined convergent criteria via the back-propagation method. By constructing a rational model input and network architecture, TFFNet can capture spatial and temporal dependencies simultaneously from traffic flow matrices during model training and outperforms other typical traffic flow forecasting methods in the experimental dataset.
Keywords: city-wide traffic flow forecasting; deep learning; external factors fusion; multi-branch prediction network; taxicabs GPS trajectories.
Conflict of interest statement
The authors declare no conflict of interest.
Figures
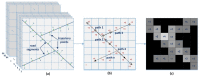
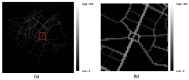
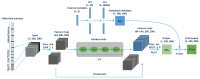
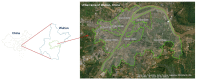
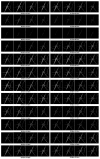
Similar articles
-
Exploiting dynamic spatio-temporal graph convolutional neural networks for citywide traffic flows prediction.Neural Netw. 2022 Jan;145:233-247. doi: 10.1016/j.neunet.2021.10.021. Epub 2021 Oct 28. Neural Netw. 2022. PMID: 34773899
-
Multitask Learning and GCN-Based Taxi Demand Prediction for a Traffic Road Network.Sensors (Basel). 2020 Jul 5;20(13):3776. doi: 10.3390/s20133776. Sensors (Basel). 2020. PMID: 32635669 Free PMC article.
-
Hybrid Deep Learning Approach for Traffic Speed Prediction.Big Data. 2024 Oct;12(5):377-389. doi: 10.1089/big.2021.0251. Epub 2022 Feb 2. Big Data. 2024. PMID: 35108088
-
Urban Safety: An Image-Processing and Deep-Learning-Based Intelligent Traffic Management and Control System.Sensors (Basel). 2021 Nov 19;21(22):7705. doi: 10.3390/s21227705. Sensors (Basel). 2021. PMID: 34833794 Free PMC article. Review.
-
Traffic safety and city structure: lessons for the future.Salud Publica Mex. 2008;50 Suppl 1:S93-100. doi: 10.1590/s0036-36342008000700014. Salud Publica Mex. 2008. PMID: 18373015 Review.
Cited by
-
Energy Based Logic Mining Analysis with Hopfield Neural Network for Recruitment Evaluation.Entropy (Basel). 2020 Dec 30;23(1):40. doi: 10.3390/e23010040. Entropy (Basel). 2020. PMID: 33396577 Free PMC article.
-
Deep learning on multi-view sequential data: a survey.Artif Intell Rev. 2023;56(7):6661-6704. doi: 10.1007/s10462-022-10332-z. Epub 2022 Nov 29. Artif Intell Rev. 2023. PMID: 36466765 Free PMC article.
-
Incorporating Multivariate Auxiliary Information for Traffic Prediction on Highways.Sensors (Basel). 2023 Mar 31;23(7):3631. doi: 10.3390/s23073631. Sensors (Basel). 2023. PMID: 37050690 Free PMC article.
-
FASTNN: A Deep Learning Approach for Traffic Flow Prediction Considering Spatiotemporal Features.Sensors (Basel). 2022 Sep 13;22(18):6921. doi: 10.3390/s22186921. Sensors (Basel). 2022. PMID: 36146272 Free PMC article.
-
Temporal Aggregation Effects in Spatiotemporal Traffic Modelling.Sensors (Basel). 2020 Dec 4;20(23):6931. doi: 10.3390/s20236931. Sensors (Basel). 2020. PMID: 33291588 Free PMC article.
References
-
- Karlaftis M.G., Vlahogianni E.I. Statistical methods versus neural networks in transportation research: Differences, similarities and some insights. Transp. Res. Part C Emerg. Technol. 2011;19:387–399. doi: 10.1016/j.trc.2010.10.004. - DOI
-
- Davis G.A., Nihan N.L. Nonparametric Regression and Short-Term Freeway Traffic Forecasting. J. Transp. Eng. 1991;117:178–188. doi: 10.1061/(ASCE)0733-947X(1991)117:2(178). - DOI
-
- Chang H., Lee Y., Yoon B., Baek S. Dynamic near-term traffic flow prediction: Systemoriented approach based on past experiences. IET Intell. Transp. Syst. 2012;6:292–305. doi: 10.1049/iet-its.2011.0123. - DOI
-
- Xia D., Wang B., Li H., Li Y., Zhang Z. A distributed spatial-temporal weighted model on MapReduce for short-term traffic flow forecasting. Neurocomputing. 2016;179:246–263. doi: 10.1016/j.neucom.2015.12.013. - DOI
-
- Wu C.H., Ho J.M., Lee D.T. Travel-time prediction with support vector regression. IEEE Trans. Intell. Transp. Syst. 2004;5:276–281. doi: 10.1109/TITS.2004.837813. - DOI
LinkOut - more resources
Full Text Sources