Field-Based High-Throughput Phenotyping for Maize Plant Using 3D LiDAR Point Cloud Generated With a "Phenomobile"
- PMID: 31134110
- PMCID: PMC6514377
- DOI: 10.3389/fpls.2019.00554
Field-Based High-Throughput Phenotyping for Maize Plant Using 3D LiDAR Point Cloud Generated With a "Phenomobile"
Abstract
With the rapid rising of global population, the demand for improving breeding techniques to greatly increase the worldwide crop production has become more and more urgent. Most researchers believe that the key to new breeding techniques lies in genetic improvement of crops, which leads to a large quantity of phenotyping spots. Unfortunately, current phenotyping solutions are not powerful enough to handle so many spots with satisfying speed and accuracy. As a result, high-throughput phenotyping is drawing more and more attention. In this paper, we propose a new field-based sensing solution to high-throughput phenotyping. We mount a LiDAR (Velodyne HDL64-S3) on a mobile robot, making the robot a "phenomobile." We develop software for data collection and analysis under Robotic Operating System using open source components and algorithm libraries. Different from conducting phenotyping observations with an in-row and one-by-one manner, our new solution allows the robot to move around the parcel to collect data. Thus, the 3D and 360° view laser scanner can collect phenotyping data for a large plant group at the same time, instead of one by one. Furthermore, no touching interference from the robot would be imposed onto the crops. We conduct experiments for maize plant on two parcels. We implement point cloud merging with landmarks and Iterative Closest Points to cut down the time consumption. We then recognize and compute the morphological phenotyping parameters (row spacing and plant height) of maize plant using depth-band histograms and horizontal point density. We analyze the cloud registration and merging performances, the row spacing detection accuracy, and the single plant height computation accuracy. Experimental results verify the feasibility of the proposed solution.
Keywords: 3D LiDAR; field-based; high-throughput phenotyping; maize; mobile robot; point cloud.
Figures
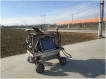
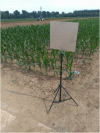
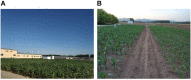
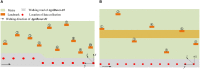
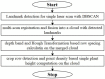

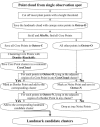
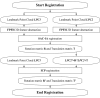
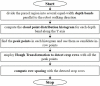
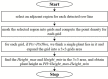
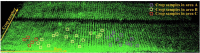
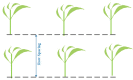
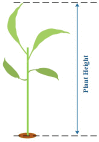
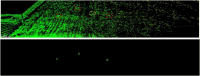
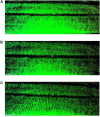
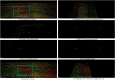
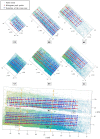
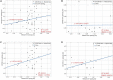
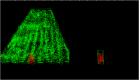
Similar articles
-
Dynamic detection of three-dimensional crop phenotypes based on a consumer-grade RGB-D camera.Front Plant Sci. 2023 Jan 27;14:1097725. doi: 10.3389/fpls.2023.1097725. eCollection 2023. Front Plant Sci. 2023. PMID: 36778701 Free PMC article.
-
Crop 3D-a LiDAR based platform for 3D high-throughput crop phenotyping.Sci China Life Sci. 2018 Mar;61(3):328-339. doi: 10.1007/s11427-017-9056-0. Epub 2017 Dec 6. Sci China Life Sci. 2018. PMID: 28616808
-
An Accurate Skeleton Extraction Approach From 3D Point Clouds of Maize Plants.Front Plant Sci. 2019 Mar 7;10:248. doi: 10.3389/fpls.2019.00248. eCollection 2019. Front Plant Sci. 2019. PMID: 30899271 Free PMC article.
-
Robotic Technologies for High-Throughput Plant Phenotyping: Contemporary Reviews and Future Perspectives.Front Plant Sci. 2021 Jun 25;12:611940. doi: 10.3389/fpls.2021.611940. eCollection 2021. Front Plant Sci. 2021. PMID: 34249028 Free PMC article. Review.
-
Measuring crops in 3D: using geometry for plant phenotyping.Plant Methods. 2019 Sep 3;15:103. doi: 10.1186/s13007-019-0490-0. eCollection 2019. Plant Methods. 2019. PMID: 31497064 Free PMC article. Review.
Cited by
-
High-Throughput Phenotyping: A Platform to Accelerate Crop Improvement.Phenomics. 2021 May 11;1(2):31-53. doi: 10.1007/s43657-020-00007-6. eCollection 2021 Apr. Phenomics. 2021. PMID: 36939738 Free PMC article. Review.
-
Genomics combined with UAS data enhances prediction of grain yield in winter wheat.Front Genet. 2023 Mar 29;14:1124218. doi: 10.3389/fgene.2023.1124218. eCollection 2023. Front Genet. 2023. PMID: 37065497 Free PMC article.
-
A Comprehensive Review of High Throughput Phenotyping and Machine Learning for Plant Stress Phenotyping.Phenomics. 2022 Apr 4;2(3):156-183. doi: 10.1007/s43657-022-00048-z. eCollection 2022 Jun. Phenomics. 2022. PMID: 36939773 Free PMC article. Review.
-
An overview of image-based phenotyping as an adaptive 4.0 technology for studying plant abiotic stress: A bibliometric and literature review.Heliyon. 2023 Nov 2;9(11):e21650. doi: 10.1016/j.heliyon.2023.e21650. eCollection 2023 Nov. Heliyon. 2023. PMID: 38027954 Free PMC article. Review.
-
Methods and Applications of 3D Ground Crop Analysis Using LiDAR Technology: A Survey.Sensors (Basel). 2023 Aug 16;23(16):7212. doi: 10.3390/s23167212. Sensors (Basel). 2023. PMID: 37631749 Free PMC article. Review.
References
-
- Andrade F. H., Calviño P., Cirilo A., Barbieri P. (2002). Yield responses to narrow rows depend on increased radiation interception. Agron. J. 94 975–980.
-
- Andújar D., Ribeiro A., Fernández-Quintanilla C., Dorado J. (2016). Using depth cameras to extract structural parameters to assess the growth state and yield of cauliflower crops. Comput. Electron. Agric. 122 67–73. 10.1016/j.compag.2016.01.018 - DOI
-
- Asaari M. S. M., Mishra P., Mertens S., Dhondt S., Inzé D., Wuyts N., et al. (2018). Close-range hyperspectral image analysis for the early detection of stress responses in individual plants in a high-throughput phenotyping platform. ISPRS J. Photogramm. Remote Sens. 138 121–138. 10.1016/j.isprsjprs.2018.02.003 - DOI
LinkOut - more resources
Full Text Sources
Research Materials