Lateral Motion Prediction of On-Road Preceding Vehicles: A Data-Driven Approach
- PMID: 31067760
- PMCID: PMC6539340
- DOI: 10.3390/s19092111
Lateral Motion Prediction of On-Road Preceding Vehicles: A Data-Driven Approach
Abstract
Drivers' behaviors and decision making on the road directly affect the safety of themselves, other drivers, and pedestrians. However, as distinct entities, people cannot predict the motions of surrounding vehicles and they have difficulty in performing safe reactionary driving maneuvers in a short time period. To overcome the limitations of making an immediate prediction, in this work, we propose a two-stage data-driven approach: classifying driving patterns of on-road surrounding vehicles using the Gaussian mixture models (GMM); and predicting vehicles' short-term lateral motions (i.e., left/right turn and left/right lane change) based on real-world vehicle mobility data, provided by the U.S. Department of Transportation, with different ensemble decision trees. We considered several important kinetic features and higher order kinematic variables. The research results of our proposed approach demonstrate the effectiveness of pattern classification and on-road lateral motion prediction. This methodology framework has the potential to be incorporated into current data-driven collision warning systems, to enable more practical on-road preprocessing in intelligent vehicles, and to be applied in autopilot-driving scenarios.
Keywords: data mining; data-driven intelligent vehicles; driver behavior classification; lateral motion prediction; vehicle mobility data.
Conflict of interest statement
The authors declare no conflict of interest regarding the publication of this paper.
Figures
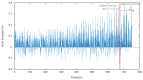
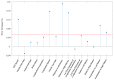
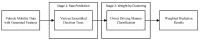
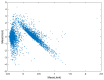
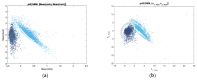
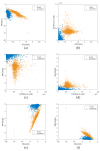
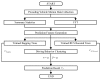
Similar articles
-
Does assisted driving behavior lead to safety-critical encounters with unequipped vehicles' drivers?Accid Anal Prev. 2016 Oct;95(Pt A):149-56. doi: 10.1016/j.aap.2016.07.003. Epub 2016 Jul 18. Accid Anal Prev. 2016. PMID: 27442594
-
Driver Behavior During Overtaking Maneuvers from the 100-Car Naturalistic Driving Study.Traffic Inj Prev. 2015;16 Suppl 2:S176-81. doi: 10.1080/15389588.2015.1057281. Traffic Inj Prev. 2015. PMID: 26436229
-
SafeDrive: Hybrid Recommendation System Architecture for Early Safety Predication Using Internet of Vehicles.Sensors (Basel). 2021 Jun 4;21(11):3893. doi: 10.3390/s21113893. Sensors (Basel). 2021. PMID: 34199981 Free PMC article.
-
Use Of Smartphones for Ensuring Vulnerable Road User Safety through Path Prediction and Early Warning: An In-Depth Review of Capabilities, Limitations and Their Applications in Cooperative Intelligent Transport Systems.Sensors (Basel). 2020 Feb 13;20(4):997. doi: 10.3390/s20040997. Sensors (Basel). 2020. PMID: 32069811 Free PMC article. Review.
-
Associating Vehicles Automation With Drivers Functional State Assessment Systems: A Challenge for Road Safety in the Future.Front Hum Neurosci. 2019 Apr 24;13:131. doi: 10.3389/fnhum.2019.00131. eCollection 2019. Front Hum Neurosci. 2019. PMID: 31114489 Free PMC article. Review.
Cited by
-
Conditional Artificial Potential Field-Based Autonomous Vehicle Safety Control with Interference of Lane Changing in Mixed Traffic Scenario.Sensors (Basel). 2019 Sep 27;19(19):4199. doi: 10.3390/s19194199. Sensors (Basel). 2019. PMID: 31569737 Free PMC article.
-
A Collision Relationship-Based Driving Behavior Decision-Making Method for an Intelligent Land Vehicle at a Disorderly Intersection via DRQN.Sensors (Basel). 2022 Jan 14;22(2):636. doi: 10.3390/s22020636. Sensors (Basel). 2022. PMID: 35062596 Free PMC article.
References
-
- Singh S. Traffic Safety Facts-Crash Stats. NHTSA’s National Center for Statistics and Analysis; Washington, DC, USA: 2015. Critical Reasons for Crashes Investigated in the National Motor Vehicle Crash Causation Survey.
-
- Nilsson J., Brännström M., Coelingh E., Fredriksson J. Lane Change Maneuvers for Automated Vehicles. IEEE Trans. Intell. Transp. Syst. 2017;18:1087–1096. doi: 10.1109/TITS.2016.2597966. - DOI
-
- Butakov V.A., Ioannou P. Personalized Driver/Vehicle Lane Change Models for ADAS. IEEE Trans. Veh. Technol. 2015;64:4422–4431. doi: 10.1109/TVT.2014.2369522. - DOI
-
- Zhang J., Wang F., Wang K., Lin W., Xu X., Chen C. Data-Driven Intelligent Transportation Systems: A Survey. IEEE Trans. Intell. Transp. Syst. 2011;12:1624–1639. doi: 10.1109/TITS.2011.2158001. - DOI
-
- Safety Pilot Model Deployment Data—Data.gov. [(accessed on 31 March 2019)]; Available online: https://catalog.data.gov/dataset/safety-pilot-model-deployment-data.
LinkOut - more resources
Full Text Sources