Computational models in cardiology
- PMID: 30361497
- PMCID: PMC6556062
- DOI: 10.1038/s41569-018-0104-y
Computational models in cardiology
Abstract
The treatment of individual patients in cardiology practice increasingly relies on advanced imaging, genetic screening and devices. As the amount of imaging and other diagnostic data increases, paralleled by the greater capacity to personalize treatment, the difficulty of using the full array of measurements of a patient to determine an optimal treatment seems also to be paradoxically increasing. Computational models are progressively addressing this issue by providing a common framework for integrating multiple data sets from individual patients. These models, which are based on physiology and physics rather than on population statistics, enable computational simulations to reveal diagnostic information that would have otherwise remained concealed and to predict treatment outcomes for individual patients. The inherent need for patient-specific models in cardiology is clear and is driving the rapid development of tools and techniques for creating personalized methods to guide pharmaceutical therapy, deployment of devices and surgical interventions.
Conflict of interest statement
Competing interests
N.A.T. declares no competing interests.
Figures
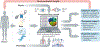
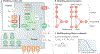
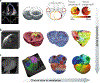
Similar articles
-
Human-based approaches to pharmacology and cardiology: an interdisciplinary and intersectorial workshop.Europace. 2016 Sep;18(9):1287-98. doi: 10.1093/europace/euv320. Epub 2015 Nov 29. Europace. 2016. PMID: 26622055 Free PMC article.
-
Precision medicine in cardiology.Nat Rev Cardiol. 2016 Oct;13(10):591-602. doi: 10.1038/nrcardio.2016.101. Epub 2016 Jun 30. Nat Rev Cardiol. 2016. PMID: 27356875 Review.
-
["Made-to-measure cardiology", personalized cardiology for the patient!].Presse Med. 2015 Jul-Aug;44(7-8):728-9. doi: 10.1016/j.lpm.2015.03.021. Epub 2015 Jul 2. Presse Med. 2015. PMID: 26142949 French. No abstract available.
-
Nuclear Cardiology in the Era of Precision Medicine: Tailoring Treatment to the Individual Patient.Cureus. 2024 Apr 24;16(4):e58960. doi: 10.7759/cureus.58960. eCollection 2024 Apr. Cureus. 2024. PMID: 38800181 Free PMC article. Review.
-
Personalized Interventions: A Reality in the Next 20 Years or Pie in the Sky.Pediatr Cardiol. 2020 Mar;41(3):486-502. doi: 10.1007/s00246-020-02303-4. Epub 2020 Mar 20. Pediatr Cardiol. 2020. PMID: 32198592 Review.
Cited by
-
Progress of Conductivity and Conduction Velocity Measured in Human and Animal Hearts.Rev Cardiovasc Med. 2024 Oct 11;25(10):364. doi: 10.31083/j.rcm2510364. eCollection 2024 Oct. Rev Cardiovasc Med. 2024. PMID: 39484125 Free PMC article. Review.
-
A deep learning algorithm for the detection of aortic dissection on non-contrast-enhanced computed tomography via the identification and segmentation of the true and false lumens of the aorta.Quant Imaging Med Surg. 2024 Oct 1;14(10):7365-7378. doi: 10.21037/qims-24-533. Epub 2024 Sep 26. Quant Imaging Med Surg. 2024. PMID: 39429578 Free PMC article.
-
The Ithildin library for efficient numerical solution of anisotropic reaction-diffusion problems in excitable media.PLoS One. 2024 Sep 19;19(9):e0303674. doi: 10.1371/journal.pone.0303674. eCollection 2024. PLoS One. 2024. PMID: 39298417 Free PMC article.
-
A guide for assessment of myocardial stiffness in health and disease.Nat Cardiovasc Res. 2022 Jan;1(1):8-22. doi: 10.1038/s44161-021-00007-3. Epub 2022 Jan 12. Nat Cardiovasc Res. 2022. PMID: 39196108 Review.
-
Uncertainty Quantification of Fiber Orientation and Epicardial Activation.Comput Cardiol (2010). 2023 Oct;50:10.22489/cinc.2023.137. doi: 10.22489/cinc.2023.137. Epub 2023 Dec 26. Comput Cardiol (2010). 2023. PMID: 39193483 Free PMC article.
References
-
- Antman EM & Loscalzo J Precision medicine in cardiology. Nat. Rev. Cardiol 13, 591–602 (2016). - PubMed
-
- Horiuchi Y et al. Identifying novel phenotypes of acute heart failure using cluster analysis of clinical variables. Int. J. Cardiol 262, 57–63 (2018). - PubMed
-
- Stanley K Design of randomized controlled trials. Circulation 115, 1164–1169 (2007). - PubMed
Publication types
MeSH terms
Grants and funding
LinkOut - more resources
Full Text Sources
Other Literature Sources
Research Materials