On the Feasibility of Using an Ear-EEG to Develop an Endogenous Brain-Computer Interface
- PMID: 30158505
- PMCID: PMC6165202
- DOI: 10.3390/s18092856
On the Feasibility of Using an Ear-EEG to Develop an Endogenous Brain-Computer Interface
Abstract
Brain-computer interface (BCI) studies based on electroencephalography (EEG) measured around the ears (ear-EEGs) have mostly used exogenous paradigms involving brain activity evoked by external stimuli. The objective of this study is to investigate the feasibility of ear-EEGs for development of an endogenous BCI system that uses self-modulated brain activity. We performed preliminary and main experiments where EEGs were measured on the scalp and behind the ears to check the reliability of ear-EEGs as compared to scalp-EEGs. In the preliminary and main experiments, subjects performed eyes-open and eyes-closed tasks, and they performed mental arithmetic (MA) and light cognitive (LC) tasks, respectively. For data analysis, the brain area was divided into four regions of interest (ROIs) (i.e., frontal, central, occipital, and ear area). The preliminary experiment showed that the degree of alpha activity increase of the ear area with eyes closed is comparable to those of other ROIs (occipital > ear > central > frontal). In the main experiment, similar event-related (de)synchronization (ERD/ERS) patterns were observed between the four ROIs during MA and LC, and all ROIs showed the mean classification accuracies above 70% required for effective binary communication (MA vs. LC) (occipital = ear = central = frontal). From the results, we demonstrated that ear-EEG can be used to develop an endogenous BCI system based on cognitive tasks without external stimuli, which allows the usability of ear-EEGs to be extended.
Keywords: brain-computer interface (BCI); ear-EEG; electroencephalography (EEG); endogenous BCI; mental arithmetic.
Conflict of interest statement
The authors declare that the research was conducted in the absence of any commercial or financial relationships that could be constructed as a potential conflict of interest.
Figures
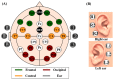
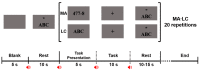
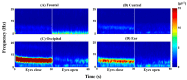
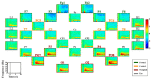
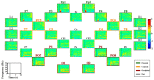
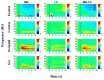
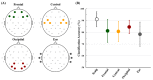
Similar articles
-
Effects of Different Re-referencing Methods on Spontaneously Generated Ear-EEG.Front Neurosci. 2019 Aug 7;13:822. doi: 10.3389/fnins.2019.00822. eCollection 2019. Front Neurosci. 2019. PMID: 31440129 Free PMC article.
-
Evaluation of Real-Time Endogenous Brain-Computer Interface Developed Using Ear-Electroencephalography.Front Neurosci. 2022 Mar 24;16:842635. doi: 10.3389/fnins.2022.842635. eCollection 2022. Front Neurosci. 2022. PMID: 35401092 Free PMC article.
-
Mobile BCI dataset of scalp- and ear-EEGs with ERP and SSVEP paradigms while standing, walking, and running.Sci Data. 2021 Dec 20;8(1):315. doi: 10.1038/s41597-021-01094-4. Sci Data. 2021. PMID: 34930915 Free PMC article.
-
EEG-based classification of imaginary left and right foot movements using beta rebound.Clin Neurophysiol. 2013 Nov;124(11):2153-60. doi: 10.1016/j.clinph.2013.05.006. Epub 2013 Jun 10. Clin Neurophysiol. 2013. PMID: 23757379
-
A comprehensive review of EEG-based brain-computer interface paradigms.J Neural Eng. 2019 Feb;16(1):011001. doi: 10.1088/1741-2552/aaf12e. Epub 2018 Nov 15. J Neural Eng. 2019. PMID: 30523919 Review.
Cited by
-
Effects of Different Re-referencing Methods on Spontaneously Generated Ear-EEG.Front Neurosci. 2019 Aug 7;13:822. doi: 10.3389/fnins.2019.00822. eCollection 2019. Front Neurosci. 2019. PMID: 31440129 Free PMC article.
-
EEG Dataset for the Recognition of Different Emotions Induced in Voice-User Interaction.Sci Data. 2024 Oct 3;11(1):1084. doi: 10.1038/s41597-024-03887-9. Sci Data. 2024. PMID: 39362909 Free PMC article.
-
Evaluation of Real-Time Endogenous Brain-Computer Interface Developed Using Ear-Electroencephalography.Front Neurosci. 2022 Mar 24;16:842635. doi: 10.3389/fnins.2022.842635. eCollection 2022. Front Neurosci. 2022. PMID: 35401092 Free PMC article.
-
EEG Headset Evaluation for Detection of Single-Trial Movement Intention for Brain-Computer Interfaces.Sensors (Basel). 2020 May 14;20(10):2804. doi: 10.3390/s20102804. Sensors (Basel). 2020. PMID: 32423133 Free PMC article.
References
-
- Allison B.Z., Dunne S., Leeb R., Millán J., Nijholt A. Towards Practical Brain-Computer Interfaces: Bridging the Gap from Research to Real-World Applications. Springer; Berlin, Germany: 2013. Recent and upcoming BCI progress: Overview, analysis, and recommendations; pp. 1–13.
-
- Eric C.L., Gerwin S., Jonathan R.W., Jeffrey G.O., Daniel W.M. A brain-computer interface using electrocorticographic signals in humans. J. Neural Eng. 2004;1:63. - PubMed
Publication types
MeSH terms
LinkOut - more resources
Full Text Sources
Other Literature Sources