A low-complexity metabolic network model for the respiratory and fermentative metabolism of Escherichia coli
- PMID: 30157229
- PMCID: PMC6114798
- DOI: 10.1371/journal.pone.0202565
A low-complexity metabolic network model for the respiratory and fermentative metabolism of Escherichia coli
Abstract
Over the last decades, predictive microbiology has made significant advances in the mathematical description of microbial spoiler and pathogen dynamics in or on food products. Recently, the focus of predictive microbiology has shifted from a (semi-)empirical population-level approach towards mechanistic models including information about the intracellular metabolism in order to increase model accuracy and genericness. However, incorporation of this subpopulation-level information increases model complexity and, consequently, the required run time to simulate microbial cell and population dynamics. In this paper, results of metabolic flux balance analyses (FBA) with a genome-scale model are used to calibrate a low-complexity linear model describing the microbial growth and metabolite secretion rates of Escherichia coli as a function of the nutrient and oxygen uptake rate. Hence, the required information about the cellular metabolism (i.e., biomass growth and secretion of cell products) is selected and included in the linear model without incorporating the complete intracellular reaction network. However, the applied FBAs are only representative for microbial dynamics under specific extracellular conditions, viz., a neutral medium without weak acids at a temperature of 37℃. Deviations from these reference conditions lead to metabolic shifts and adjustments of the cellular nutrient uptake or maintenance requirements. This metabolic dependency on extracellular conditions has been taken into account in our low-complex metabolic model. In this way, a novel approach is developed to take the synergistic effects of temperature, pH, and undissociated acids on the cell metabolism into account. Consequently, the developed model is deployable as a tool to describe, predict and control E. coli dynamics in and on food products under various combinations of environmental conditions. To emphasize this point,three specific scenarios are elaborated: (i) aerobic respiration without production of weak acid extracellular metabolites, (ii) anaerobic fermentation with secretion of mixed acid fermentation products into the food environment, and (iii) respiro-fermentative metabolic regimes in between the behaviors at aerobic and anaerobic conditions.
Conflict of interest statement
The authors have declared that no competing interests exist.
Figures
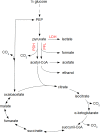
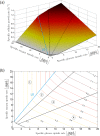
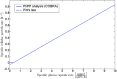
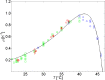
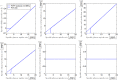
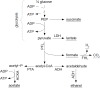
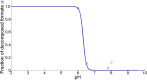
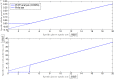
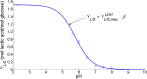
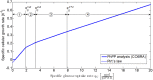
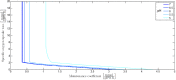
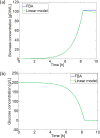
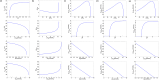
Similar articles
-
A hybrid model of anaerobic E. coli GJT001: combination of elementary flux modes and cybernetic variables.Biotechnol Prog. 2008 Sep-Oct;24(5):993-1006. doi: 10.1002/btpr.73. Biotechnol Prog. 2008. PMID: 19194908
-
Modelling overflow metabolism in Escherichia coli with flux balance analysis incorporating differential proteomic efficiencies of energy pathways.BMC Syst Biol. 2019 Jan 10;13(1):3. doi: 10.1186/s12918-018-0677-4. BMC Syst Biol. 2019. PMID: 30630470 Free PMC article.
-
NetRed, an algorithm to reduce genome-scale metabolic networks and facilitate the analysis of flux predictions.Metab Eng. 2021 May;65:207-222. doi: 10.1016/j.ymben.2020.11.003. Epub 2020 Nov 6. Metab Eng. 2021. PMID: 33161143
-
Upflow anaerobic sludge blanket reactor--a review.Indian J Environ Health. 2001 Apr;43(2):1-82. Indian J Environ Health. 2001. PMID: 12397675 Review.
-
Flux balance analysis of metabolic networks for efficient engineering of microbial cell factories.Biotechnol Genet Eng Rev. 2024 Dec;40(4):3682-3715. doi: 10.1080/02648725.2022.2152631. Epub 2022 Dec 7. Biotechnol Genet Eng Rev. 2024. PMID: 36476223 Review.
Cited by
-
Lung but not brain cancer cell malignancy inhibited by commonly used anesthetic propofol during surgery: Implication of reducing cancer recurrence risk.J Adv Res. 2021 Jan 6;31:1-12. doi: 10.1016/j.jare.2020.12.007. eCollection 2021 Jul. J Adv Res. 2021. PMID: 34194828 Free PMC article.
-
StressME: Unified computing framework of Escherichia coli metabolism, gene expression, and stress responses.PLoS Comput Biol. 2024 Feb 12;20(2):e1011865. doi: 10.1371/journal.pcbi.1011865. eCollection 2024 Feb. PLoS Comput Biol. 2024. PMID: 38346086 Free PMC article.
References
Publication types
MeSH terms
Grants and funding
LinkOut - more resources
Full Text Sources
Other Literature Sources