Improving High-Throughput Phenotyping Using Fusion of Close-Range Hyperspectral Camera and Low-Cost Depth Sensor
- PMID: 30126148
- PMCID: PMC6111299
- DOI: 10.3390/s18082711
Improving High-Throughput Phenotyping Using Fusion of Close-Range Hyperspectral Camera and Low-Cost Depth Sensor
Abstract
Hyperspectral sensors, especially the close-range hyperspectral camera, have been widely introduced to detect biological processes of plants in the high-throughput phenotyping platform, to support the identification of biotic and abiotic stress reactions at an early stage. However, the complex geometry of plants and their interaction with the illumination, severely affects the spectral information obtained. Furthermore, plant structure, leaf area, and leaf inclination distribution are critical indexes which have been widely used in multiple plant models. Therefore, the process of combination between hyperspectral images and 3D point clouds is a promising approach to solve these problems and improve the high-throughput phenotyping technique. We proposed a novel approach fusing a low-cost depth sensor and a close-range hyperspectral camera, which extended hyperspectral camera ability with 3D information as a potential tool for high-throughput phenotyping. An exemplary new calibration and analysis method was shown in soybean leaf experiments. The results showed that a 0.99 pixel resolution for the hyperspectral camera and a 3.3 millimeter accuracy for the depth sensor, could be achieved in a controlled environment using the method proposed in this paper. We also discussed the new capabilities gained using this new method, to quantify and model the effects of plant geometry and sensor configuration. The possibility of 3D reflectance models can be used to minimize the geometry-related effects in hyperspectral images, and to significantly improve high-throughput phenotyping. Overall results of this research, indicated that the proposed method provided more accurate spatial and spectral plant information, which helped to enhance the precision of biological processes in high-throughput phenotyping.
Keywords: close-range hyperspectral camera; fusion; high-throughput phenotyping; low-cost depth sensor; plant 3D model.
Conflict of interest statement
The authors declare no conflict of interest.
Figures
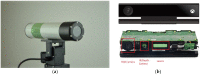
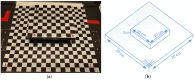
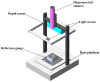
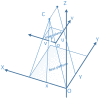
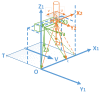
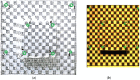
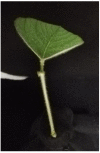
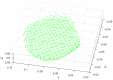
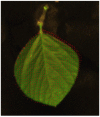
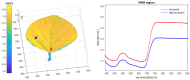
Similar articles
-
Crop 3D-a LiDAR based platform for 3D high-throughput crop phenotyping.Sci China Life Sci. 2018 Mar;61(3):328-339. doi: 10.1007/s11427-017-9056-0. Epub 2017 Dec 6. Sci China Life Sci. 2018. PMID: 28616808
-
Elimination of Leaf Angle Impacts on Plant Reflectance Spectra Using Fusion of Hyperspectral Images and 3D Point Clouds.Sensors (Basel). 2022 Dec 21;23(1):44. doi: 10.3390/s23010044. Sensors (Basel). 2022. PMID: 36616642 Free PMC article.
-
Technical workflows for hyperspectral plant image assessment and processing on the greenhouse and laboratory scale.Gigascience. 2020 Aug 1;9(8):giaa090. doi: 10.1093/gigascience/giaa090. Gigascience. 2020. PMID: 32815537 Free PMC article. Review.
-
Reflectance Measurement Method Based on Sensor Fusion of Frame-Based Hyperspectral Imager and Time-of-Flight Depth Camera.Sensors (Basel). 2022 Nov 10;22(22):8668. doi: 10.3390/s22228668. Sensors (Basel). 2022. PMID: 36433268 Free PMC article.
-
Image-Based High-Throughput Phenotyping in Horticultural Crops.Plants (Basel). 2023 May 22;12(10):2061. doi: 10.3390/plants12102061. Plants (Basel). 2023. PMID: 37653978 Free PMC article. Review.
Cited by
-
A hyperspectral plant health monitoring system for space crop production.Front Plant Sci. 2023 Jul 4;14:1133505. doi: 10.3389/fpls.2023.1133505. eCollection 2023. Front Plant Sci. 2023. PMID: 37469773 Free PMC article.
-
Rotational hyperspectral scanner and related image reconstruction algorithm.Sci Rep. 2021 Feb 8;11(1):3296. doi: 10.1038/s41598-021-82819-8. Sci Rep. 2021. PMID: 33558585 Free PMC article.
-
End-to-End Fusion of Hyperspectral and Chlorophyll Fluorescence Imaging to Identify Rice Stresses.Plant Phenomics. 2022 Aug 2;2022:9851096. doi: 10.34133/2022/9851096. eCollection 2022. Plant Phenomics. 2022. PMID: 36059603 Free PMC article.
-
The Development of Hyperspectral Distribution Maps to Predict the Content and Distribution of Nitrogen and Water in Wheat (Triticum aestivum).Front Plant Sci. 2019 Oct 30;10:1380. doi: 10.3389/fpls.2019.01380. eCollection 2019. Front Plant Sci. 2019. PMID: 31737009 Free PMC article.
-
Estimation of Leaf Nitrogen Content in Wheat Based on Fusion of Spectral Features and Deep Features from Near Infrared Hyperspectral Imagery.Sensors (Basel). 2021 Jan 17;21(2):613. doi: 10.3390/s21020613. Sensors (Basel). 2021. PMID: 33477350 Free PMC article.
References
-
- Bai G., Ge Y., Hussain W., Baenziger P.S., Graef G. A multi-sensor system for high throughput field phenotyping in soybean and wheat breeding. Comput. Electron. Agric. 2016;128:181–192. doi: 10.1016/j.compag.2016.08.021. - DOI
-
- Thenkabail P.S., Lyon J.G., Huete A. Hyperspectral Remote Sensing of Vegetation. CRC Press; Boca Raton, FL, USA: 2012. Advances in Hyperspectral Remote Sensing of Vegetation and Agricultural Croplands; pp. 3–32.
-
- Gonzalezdugo V., Hernandez P., Solis I., Zarcotejada P. Using high-resolution hyperspectral and thermal airborne imagery to assess physiological condition in the context of wheat phenotyping. Remote Sens. 2015;7:13586–13605. doi: 10.3390/rs71013586. - DOI
-
- Thomas S., Kuska M.T., Bohnenkamp D., Brugger A., Alisaac E., Wahabzada M. Benefits of hyperspectral imaging for plant disease detection and plant protection: A technical perspective. J. Plant Dis. Prot. 2018;125:5–20. doi: 10.1007/s41348-017-0124-6. - DOI
LinkOut - more resources
Full Text Sources
Other Literature Sources