An Algorithm Based on Deep Learning for Predicting In-Hospital Cardiac Arrest
- PMID: 29945914
- PMCID: PMC6064911
- DOI: 10.1161/JAHA.118.008678
An Algorithm Based on Deep Learning for Predicting In-Hospital Cardiac Arrest
Abstract
Background: In-hospital cardiac arrest is a major burden to public health, which affects patient safety. Although traditional track-and-trigger systems are used to predict cardiac arrest early, they have limitations, with low sensitivity and high false-alarm rates. We propose a deep learning-based early warning system that shows higher performance than the existing track-and-trigger systems.
Methods and results: This retrospective cohort study reviewed patients who were admitted to 2 hospitals from June 2010 to July 2017. A total of 52 131 patients were included. Specifically, a recurrent neural network was trained using data from June 2010 to January 2017. The result was tested using the data from February to July 2017. The primary outcome was cardiac arrest, and the secondary outcome was death without attempted resuscitation. As comparative measures, we used the area under the receiver operating characteristic curve (AUROC), the area under the precision-recall curve (AUPRC), and the net reclassification index. Furthermore, we evaluated sensitivity while varying the number of alarms. The deep learning-based early warning system (AUROC: 0.850; AUPRC: 0.044) significantly outperformed a modified early warning score (AUROC: 0.603; AUPRC: 0.003), a random forest algorithm (AUROC: 0.780; AUPRC: 0.014), and logistic regression (AUROC: 0.613; AUPRC: 0.007). Furthermore, the deep learning-based early warning system reduced the number of alarms by 82.2%, 13.5%, and 42.1% compared with the modified early warning system, random forest, and logistic regression, respectively, at the same sensitivity.
Conclusions: An algorithm based on deep learning had high sensitivity and a low false-alarm rate for detection of patients with cardiac arrest in the multicenter study.
Keywords: artificial intelligence; cardiac arrest; deep learning; machine learning; rapid response system; resuscitation.
© 2018 The Authors. Published on behalf of the American Heart Association, Inc., by Wiley.
Figures
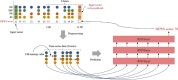
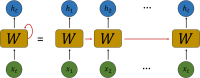
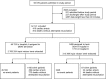
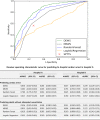
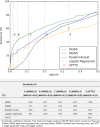
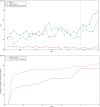
Similar articles
-
Detecting Patient Deterioration Using Artificial Intelligence in a Rapid Response System.Crit Care Med. 2020 Apr;48(4):e285-e289. doi: 10.1097/CCM.0000000000004236. Crit Care Med. 2020. PMID: 32205618
-
Explainable Artificial Intelligence Warning Model Using an Ensemble Approach for In-Hospital Cardiac Arrest Prediction: Retrospective Cohort Study.J Med Internet Res. 2023 Dec 22;25:e48244. doi: 10.2196/48244. J Med Internet Res. 2023. PMID: 38133922 Free PMC article.
-
Prospective, multicenter validation of the deep learning-based cardiac arrest risk management system for predicting in-hospital cardiac arrest or unplanned intensive care unit transfer in patients admitted to general wards.Crit Care. 2023 Sep 5;27(1):346. doi: 10.1186/s13054-023-04609-0. Crit Care. 2023. PMID: 37670324 Free PMC article.
-
Early warning system scores for clinical deterioration in hospitalized patients: a systematic review.Ann Am Thorac Soc. 2014 Nov;11(9):1454-65. doi: 10.1513/AnnalsATS.201403-102OC. Ann Am Thorac Soc. 2014. PMID: 25296111 Review.
-
Deep Learning in the Medical Domain: Predicting Cardiac Arrest Using Deep Learning.Acute Crit Care. 2018 Aug;33(3):117-120. doi: 10.4266/acc.2018.00290. Epub 2018 Aug 31. Acute Crit Care. 2018. PMID: 31723874 Free PMC article. Review.
Cited by
-
Korotkoff sounds dynamically reflect changes in cardiac function based on deep learning methods.Front Cardiovasc Med. 2022 Aug 26;9:940615. doi: 10.3389/fcvm.2022.940615. eCollection 2022. Front Cardiovasc Med. 2022. PMID: 36093170 Free PMC article. Review.
-
Early Prediction of Cardiac Arrest in the Intensive Care Unit Using Explainable Machine Learning: Retrospective Study.J Med Internet Res. 2024 Sep 17;26:e62890. doi: 10.2196/62890. J Med Internet Res. 2024. PMID: 39288404 Free PMC article.
-
Artificial Intelligence in Cardiology: Concepts, Tools and Challenges - "The Horse is the One Who Runs, You Must Be the Jockey".Arq Bras Cardiol. 2020 Apr;114(4):718-725. doi: 10.36660/abc.20180431. Epub 2020 May 29. Arq Bras Cardiol. 2020. PMID: 32491009 Free PMC article. English, Portuguese.
-
Predicting Patient Deterioration: A Review of Tools in the Digital Hospital Setting.J Med Internet Res. 2021 Sep 30;23(9):e28209. doi: 10.2196/28209. J Med Internet Res. 2021. PMID: 34591017 Free PMC article. Review.
-
2021 ISHNE/HRS/EHRA/APHRS collaborative statement on mHealth in Arrhythmia Management: Digital Medical Tools for Heart Rhythm Professionals: From the International Society for Holter and Noninvasive Electrocardiology/Heart Rhythm Society/European Heart Rhythm Association/Asia Pacific Heart Rhythm Society.J Arrhythm. 2021 Jan 29;37(2):271-319. doi: 10.1002/joa3.12461. eCollection 2021 Apr. J Arrhythm. 2021. PMID: 33850572 Free PMC article.
References
-
- Graham R, McCoy MA, Schultz AM; Committee on the Treatment of Cardiac Arrest: Current Status and Future Directions; Board on Health Sciences Policy; Institute of Medicine Strategies to Improve Cardiac Arrest Survival: A Time to Act. Washington, DC: National Academic Press; 2015. - PubMed
-
- Benjamin EJ, Blaha MJ, Chiuve SE, Cushman M, Das SR, Deo R, De Ferranti SD, Floyd J, Fornage M, Gillespie C, Isasi CR, Jim'nez MC, Jordan LC, Judd SE, Lackland D, Lichtman JH, Lisabeth L, Liu S, Longenecker CT, MacKey RH, Matsushita K, Mozaffarian D, Mussolino ME, Nasir K, Neumar RW, Palaniappan L, Pandey DK, Thiagarajan RR, Reeves MJ, Ritchey M, Rodriguez CJ, Roth GA, Rosamond WD, Sasson C, Towfghi A, Tsao CW, Turner MB, Virani SS, Voeks JH, Willey JZ, Wilkins JT, Wu JHY, Alger HM, Wong SS, Muntner P. Heart disease and stroke statistics—2017 update: a report from the American Heart Association. Circulation. 2017; 135:e146–e603. - PMC - PubMed
-
- Nadkarni VM. First documented rhythm and clinical outcome from in‐hospital cardiac arrest among children and adults. JAMA. 2006;295:50. - PubMed
Publication types
MeSH terms
LinkOut - more resources
Full Text Sources
Other Literature Sources
Medical
Research Materials