Quantitative estimation of soil salinity by means of different modeling methods and visible-near infrared (VIS-NIR) spectroscopy, Ebinur Lake Wetland, Northwest China
- PMID: 29736341
- PMCID: PMC5936634
- DOI: 10.7717/peerj.4703
Quantitative estimation of soil salinity by means of different modeling methods and visible-near infrared (VIS-NIR) spectroscopy, Ebinur Lake Wetland, Northwest China
Abstract
Soil salinization is one of the most common forms of land degradation. The detection and assessment of soil salinity is critical for the prevention of environmental deterioration especially in arid and semi-arid areas. This study introduced the fractional derivative in the pretreatment of visible and near infrared (VIS-NIR) spectroscopy. The soil samples (n = 400) collected from the Ebinur Lake Wetland, Xinjiang Uyghur Autonomous Region (XUAR), China, were used as the dataset. After measuring the spectral reflectance and salinity in the laboratory, the raw spectral reflectance was preprocessed by means of the absorbance and the fractional derivative order in the range of 0.0-2.0 order with an interval of 0.1. Two different modeling methods, namely, partial least squares regression (PLSR) and random forest (RF) with preprocessed reflectance were used for quantifying soil salinity. The results showed that more spectral characteristics were refined for the spectrum reflectance treated via fractional derivative. The validation accuracies showed that RF models performed better than those of PLSR. The most effective model was established based on RF with the 1.5 order derivative of absorbance with the optimal values of R2 (0.93), RMSE (4.57 dS m-1), and RPD (2.78 ≥ 2.50). The developed RF model was stable and accurate in the application of spectral reflectance for determining the soil salinity of the Ebinur Lake wetland. The pretreatment of fractional derivative could be useful for monitoring multiple soil parameters with higher accuracy, which could effectively help to analyze the soil salinity.
Keywords: Ebinur Lake; Machine learning; PLSR; RF; Soil salinity; VIS–NIR; Wetland.
Conflict of interest statement
The authors declare there are no competing interests.
Figures
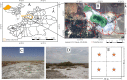
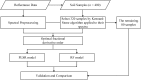
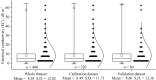
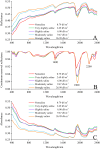
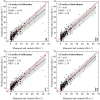
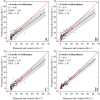
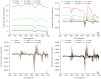
Similar articles
-
Machine-learning-based quantitative estimation of soil organic carbon content by VIS/NIR spectroscopy.PeerJ. 2018 Oct 17;6:e5714. doi: 10.7717/peerj.5714. eCollection 2018. PeerJ. 2018. PMID: 30357023 Free PMC article.
-
Estimation of Soil Organic Matter in Arid Zones with Coupled Environmental Variables and Spectral Features.Sensors (Basel). 2022 Feb 4;22(3):1194. doi: 10.3390/s22031194. Sensors (Basel). 2022. PMID: 35161939 Free PMC article.
-
Desert soil clay content estimation using reflectance spectroscopy preprocessed by fractional derivative.PLoS One. 2017 Sep 21;12(9):e0184836. doi: 10.1371/journal.pone.0184836. eCollection 2017. PLoS One. 2017. PMID: 28934274 Free PMC article.
-
Spectral prediction of soil salinity and alkalinity indicators using visible, near-, and mid-infrared spectroscopy.J Environ Manage. 2023 Nov 1;345:118854. doi: 10.1016/j.jenvman.2023.118854. Epub 2023 Aug 28. J Environ Manage. 2023. PMID: 37647733
-
Quantifying salinity in calcareous soils through advanced spectroscopic models: A comparative study of random forests and regression techniques across diverse land use systems.PLoS One. 2024 Aug 22;19(8):e0307853. doi: 10.1371/journal.pone.0307853. eCollection 2024. PLoS One. 2024. PMID: 39173042 Free PMC article.
Cited by
-
Classifying and Predicting Salinization Level in Arid Area Soil Using a Combination of Chua's Circuit and Fractional Order Sprott Chaotic System.Sensors (Basel). 2019 Oct 17;19(20):4517. doi: 10.3390/s19204517. Sensors (Basel). 2019. PMID: 31627421 Free PMC article.
-
Machine-learning-based quantitative estimation of soil organic carbon content by VIS/NIR spectroscopy.PeerJ. 2018 Oct 17;6:e5714. doi: 10.7717/peerj.5714. eCollection 2018. PeerJ. 2018. PMID: 30357023 Free PMC article.
-
Assessing Soil Organic Matter Content in a Coal Mining Area through Spectral Variables of Different Numbers of Dimensions.Sensors (Basel). 2020 Mar 24;20(6):1795. doi: 10.3390/s20061795. Sensors (Basel). 2020. PMID: 32213967 Free PMC article.
-
Estimation of Soil Organic Carbon Content in the Ebinur Lake Wetland, Xinjiang, China, Based on Multisource Remote Sensing Data and Ensemble Learning Algorithms.Sensors (Basel). 2022 Mar 31;22(7):2685. doi: 10.3390/s22072685. Sensors (Basel). 2022. PMID: 35408299 Free PMC article.
-
Estimation of soybean yield parameters under lodging conditions using RGB information from unmanned aerial vehicles.Front Plant Sci. 2022 Dec 13;13:1012293. doi: 10.3389/fpls.2022.1012293. eCollection 2022. Front Plant Sci. 2022. PMID: 36589058 Free PMC article.
References
-
- Abliz A, Tiyip T, Ghulam A, Halik Ü, Ding J-L, Sawut M, Zhang F, Nurmemet I, Abliz A. Effects of shallow groundwater table and salinity on soil salt dynamics in the Keriya Oasis, Northwestern China. Environmental Earth Sciences. 2016;75:260. doi: 10.1007/s12665-015-4794-8. - DOI
-
- Akramkhanov A, Martius C, Park SJ, Hendrickx JMH. Environmental factors of spatial distribution of soil salinity on flat irrigated terrain. Geoderma. 2011;163:55–62. doi: 10.1016/j.geoderma.2011.04.001. - DOI
-
- Allbed A, Kumar L, Aldakheel YY. Assessing soil salinity using soil salinity and vegetation indices derived from IKONOS high-spatial resolution imageries: applications in a date palm dominated region. Geoderma. 2014;230–231:1–8. doi: 10.1016/j.geoderma.2014.03.025. - DOI
-
- Bao S. Soil and agricultural chemistry analysis. Beijing: China Agricultural Science and Technology; 2000. (in Chinese)
-
- Ben-Dor E, Banin A. Near-infrared analysis as a aapid method to simultaneously evaluate several soil properties. Soil Science Society of America Journal. 1995;59:364–372. doi: 10.2136/sssaj1995.03615995005900020014x. - DOI
Grants and funding
LinkOut - more resources
Full Text Sources
Other Literature Sources
Miscellaneous