dropClust: efficient clustering of ultra-large scRNA-seq data
- PMID: 29361178
- PMCID: PMC5888655
- DOI: 10.1093/nar/gky007
dropClust: efficient clustering of ultra-large scRNA-seq data
Abstract
Droplet based single cell transcriptomics has recently enabled parallel screening of tens of thousands of single cells. Clustering methods that scale for such high dimensional data without compromising accuracy are scarce. We exploit Locality Sensitive Hashing, an approximate nearest neighbour search technique to develop a de novo clustering algorithm for large-scale single cell data. On a number of real datasets, dropClust outperformed the existing best practice methods in terms of execution time, clustering accuracy and detectability of minor cell sub-types.
Figures
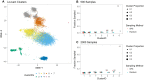
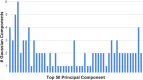
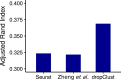
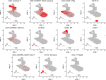
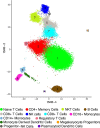
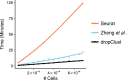
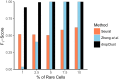
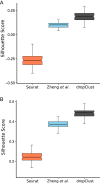
Similar articles
-
A multitask clustering approach for single-cell RNA-seq analysis in Recessive Dystrophic Epidermolysis Bullosa.PLoS Comput Biol. 2018 Apr 9;14(4):e1006053. doi: 10.1371/journal.pcbi.1006053. eCollection 2018 Apr. PLoS Comput Biol. 2018. PMID: 29630593 Free PMC article.
-
A Bayesian mixture model for clustering droplet-based single-cell transcriptomic data from population studies.Nat Commun. 2019 Apr 9;10(1):1649. doi: 10.1038/s41467-019-09639-3. Nat Commun. 2019. PMID: 30967541 Free PMC article.
-
DIMM-SC: a Dirichlet mixture model for clustering droplet-based single cell transcriptomic data.Bioinformatics. 2018 Jan 1;34(1):139-146. doi: 10.1093/bioinformatics/btx490. Bioinformatics. 2018. PMID: 29036318 Free PMC article.
-
Identifying cell types to interpret scRNA-seq data: how, why and more possibilities.Brief Funct Genomics. 2020 Jul 29;19(4):286-291. doi: 10.1093/bfgp/elaa003. Brief Funct Genomics. 2020. PMID: 32232401 Review.
-
Supervised application of internal validation measures to benchmark dimensionality reduction methods in scRNA-seq data.Brief Bioinform. 2021 Nov 5;22(6):bbab304. doi: 10.1093/bib/bbab304. Brief Bioinform. 2021. PMID: 34374742 Review.
Cited by
-
Single-Cell Transcriptomics: Current Methods and Challenges in Data Acquisition and Analysis.Front Neurosci. 2021 Apr 22;15:591122. doi: 10.3389/fnins.2021.591122. eCollection 2021. Front Neurosci. 2021. PMID: 33967674 Free PMC article. Review.
-
How does the structure of data impact cell-cell similarity? Evaluating how structural properties influence the performance of proximity metrics in single cell RNA-seq data.Brief Bioinform. 2022 Nov 19;23(6):bbac387. doi: 10.1093/bib/bbac387. Brief Bioinform. 2022. PMID: 36151725 Free PMC article.
-
Accuracy, robustness and scalability of dimensionality reduction methods for single-cell RNA-seq analysis.Genome Biol. 2019 Dec 10;20(1):269. doi: 10.1186/s13059-019-1898-6. Genome Biol. 2019. PMID: 31823809 Free PMC article.
-
Analysis of immunogenic cell death in ascending thoracic aortic aneurysms based on single-cell sequencing data.Front Immunol. 2023 May 3;14:1087978. doi: 10.3389/fimmu.2023.1087978. eCollection 2023. Front Immunol. 2023. PMID: 37207221 Free PMC article.
-
scGAL: unmask tumor clonal substructure by jointly analyzing independent single-cell copy number and scRNA-seq data.BMC Genomics. 2024 Apr 22;25(1):393. doi: 10.1186/s12864-024-10319-w. BMC Genomics. 2024. PMID: 38649804 Free PMC article.
References
-
- Li H., Courtois E.T., Sengupta D., Tan Y., Chen K.H., Goh J.J.L., Kong S.L., Chua C., Hon L.K., Tan W.S. et al. . Reference component analysis of single-cell transcriptomes elucidates cellular heterogeneity in human colorectal tumors. Nat. Genet. 2017; 49:708–718. - PubMed
-
- Zeisel A., Munoz-Manchado A.B., Codeluppi S., Lonnerberg P., La Manno G., Jureus A., Marques S., Munguba H., He L., Betsholtz C. et al. . Cell types in the mouse cortex and hippocampus revealed by single-cell RNA-seq. Science. 2015; 347:1138–1142. - PubMed
Publication types
MeSH terms
Substances
LinkOut - more resources
Full Text Sources
Other Literature Sources