Generation of Structural MR Images from Amyloid PET: Application to MR-Less Quantification
- PMID: 29217736
- PMCID: PMC6910644
- DOI: 10.2967/jnumed.117.199414
Generation of Structural MR Images from Amyloid PET: Application to MR-Less Quantification
Abstract
Structural MR images concomitantly acquired with PET images can provide crucial anatomic information for precise quantitative analysis. However, in the clinical setting, not all the subjects have corresponding MR images. Here, we developed a model to generate structural MR images from amyloid PET using deep generative networks. We applied our model to quantification of cortical amyloid load without structural MR. Methods: We used florbetapir PET and structural MR data from the Alzheimer Disease Neuroimaging Initiative database. The generative network was trained to generate realistic structural MR images from florbetapir PET images. After the training, the model was applied to the quantification of cortical amyloid load. PET images were spatially normalized to the template space using the generated MR, and then SUV ratio (SUVR) of the target regions was measured by predefined regions of interest. A real MR-based quantification was used as the gold standard to measure the accuracy of our approach. Other MR-less methods-a normal PET template-based, a multiatlas PET template-based, and a PET segmentation-based normalization/quantification-were also tested. We compared the performance of quantification methods using generated MR with that of MR-based and MR-less quantification methods. Results: Generated MR images from florbetapir PET showed signal patterns that were visually similar to the real MR. The structural similarity index between real and generated MR was 0.91 ± 0.04. The mean absolute error of SUVR of cortical composite regions estimated by the generated MR-based method was 0.04 ± 0.03, which was significantly smaller than other MR-less methods (0.29 ± 0.12 for the normal PET template, 0.12 ± 0.07 for the multiatlas PET template, and 0.08 ± 0.06 for the PET segmentation-based methods). Bland-Altman plots revealed that the generated MR-based SUVR quantification was the closest to the SUVRs estimated by the real MR-based method. Conclusion: Structural MR images were successfully generated from amyloid PET images using deep generative networks. Generated MR images could be used as templates for accurate and precise amyloid quantification. This generative method might be used to generate multimodal images of various organs for further quantitative analyses.
Keywords: MR generation; PET quantification; deep learning; florbetapir PET; generative adversarial network.
© 2018 by the Society of Nuclear Medicine and Molecular Imaging.
Figures
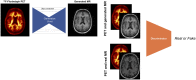
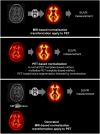
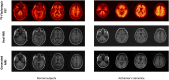
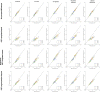
Similar articles
-
Automated PET-only quantification of amyloid deposition with adaptive template and empirically pre-defined ROI.Phys Med Biol. 2016 Aug 7;61(15):5768-80. doi: 10.1088/0031-9155/61/15/5768. Epub 2016 Jul 13. Phys Med Biol. 2016. PMID: 27405579
-
Perfusion-like template and standardized normalization-based brain image analysis using 18F-florbetapir (AV-45/Amyvid) PET.Eur J Nucl Med Mol Imaging. 2013 Jun;40(6):908-20. doi: 10.1007/s00259-013-2350-x. Epub 2013 Feb 15. Eur J Nucl Med Mol Imaging. 2013. PMID: 23412134
-
Implementation and validation of an adaptive template registration method for 18F-flutemetamol imaging data.J Nucl Med. 2013 Aug;54(8):1472-8. doi: 10.2967/jnumed.112.115006. Epub 2013 Jun 5. J Nucl Med. 2013. PMID: 23740104
-
Spatial normalization and quantification approaches of PET imaging for neurological disorders.Eur J Nucl Med Mol Imaging. 2022 Sep;49(11):3809-3829. doi: 10.1007/s00259-022-05809-6. Epub 2022 May 28. Eur J Nucl Med Mol Imaging. 2022. PMID: 35624219 Review.
-
Creating Artificial Images for Radiology Applications Using Generative Adversarial Networks (GANs) - A Systematic Review.Acad Radiol. 2020 Aug;27(8):1175-1185. doi: 10.1016/j.acra.2019.12.024. Epub 2020 Feb 5. Acad Radiol. 2020. PMID: 32035758 Review.
Cited by
-
Amyloid PET Quantification Via End-to-End Training of a Deep Learning.Nucl Med Mol Imaging. 2019 Oct;53(5):340-348. doi: 10.1007/s13139-019-00610-0. Epub 2019 Oct 14. Nucl Med Mol Imaging. 2019. PMID: 31723364 Free PMC article.
-
Discovering Digital Tumor Signatures-Using Latent Code Representations to Manipulate and Classify Liver Lesions.Cancers (Basel). 2021 Jun 22;13(13):3108. doi: 10.3390/cancers13133108. Cancers (Basel). 2021. PMID: 34206336 Free PMC article.
-
Accurate Automated Quantification of Dopamine Transporter PET Without MRI Using Deep Learning-based Spatial Normalization.Nucl Med Mol Imaging. 2024 Oct;58(6):354-363. doi: 10.1007/s13139-024-00869-y. Epub 2024 Jul 22. Nucl Med Mol Imaging. 2024. PMID: 39308485
-
Contrast-enhanced to non-contrast-enhanced image translation to exploit a clinical data warehouse of T1-weighted brain MRI.BMC Med Imaging. 2024 Mar 20;24(1):67. doi: 10.1186/s12880-024-01242-3. BMC Med Imaging. 2024. PMID: 38504179 Free PMC article.
-
Semantic Segmentation of White Matter in FDG-PET Using Generative Adversarial Network.J Digit Imaging. 2020 Aug;33(4):816-825. doi: 10.1007/s10278-020-00321-5. J Digit Imaging. 2020. PMID: 32043177 Free PMC article.
References
-
- Werner P, Barthel H, Drzezga A, Sabri O. Current status and future role of brain PET/MRI in clinical and research settings. Eur J Nucl Med Mol Imaging. 2015;42:512–516. - PubMed
-
- Pichler BJ, Kolb A, Nägele T, Schlemmer H-P. PET/MRI: paving the way for the next generation of clinical multimodality imaging applications. J Nucl Med. 2010;51:333–336. - PubMed
-
- Johnson KA, Minoshima S, Bohnen NI, et al. Appropriate use criteria for amyloid PET: a report of the Amyloid Imaging Task Force, the Society of Nuclear Medicine and Molecular Imaging, and the Alzheimer’s Association. J Nucl Med. 2013;54:476–490. - PubMed
-
- Lopresti BJ, Klunk WE, Mathis CA, et al. Simplified quantification of Pittsburgh compound B amyloid imaging PET studies: a comparative analysis. J Nucl Med. 2005;46:1959–1972. - PubMed
Publication types
MeSH terms
Substances
LinkOut - more resources
Full Text Sources
Other Literature Sources
Medical