A machine learning approach to triaging patients with chronic obstructive pulmonary disease
- PMID: 29166411
- PMCID: PMC5699810
- DOI: 10.1371/journal.pone.0188532
A machine learning approach to triaging patients with chronic obstructive pulmonary disease
Abstract
COPD patients are burdened with a daily risk of acute exacerbation and loss of control, which could be mitigated by effective, on-demand decision support tools. In this study, we present a machine learning-based strategy for early detection of exacerbations and subsequent triage. Our application uses physician opinion in a statistically and clinically comprehensive set of patient cases to train a supervised prediction algorithm. The accuracy of the model is assessed against a panel of physicians each triaging identical cases in a representative patient validation set. Our results show that algorithm accuracy and safety indicators surpass all individual pulmonologists in both identifying exacerbations and predicting the consensus triage in a 101 case validation set. The algorithm is also the top performer in sensitivity, specificity, and ppv when predicting a patient's need for emergency care.
Conflict of interest statement
Figures
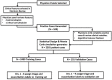
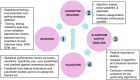
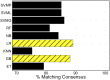
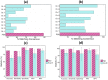
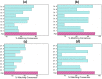
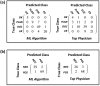
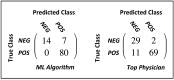
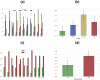
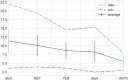
Similar articles
-
A Machine Learning Methodology for Identification and Triage of Heart Failure Exacerbations.J Cardiovasc Transl Res. 2022 Feb;15(1):103-115. doi: 10.1007/s12265-021-10151-7. Epub 2021 Aug 28. J Cardiovasc Transl Res. 2022. PMID: 34453676 Free PMC article.
-
Developing a Machine Learning Model to Predict Severe Chronic Obstructive Pulmonary Disease Exacerbations: Retrospective Cohort Study.J Med Internet Res. 2022 Jan 6;24(1):e28953. doi: 10.2196/28953. J Med Internet Res. 2022. PMID: 34989686 Free PMC article.
-
Acute Exacerbation of a Chronic Obstructive Pulmonary Disease Prediction System Using Wearable Device Data, Machine Learning, and Deep Learning: Development and Cohort Study.JMIR Mhealth Uhealth. 2021 May 6;9(5):e22591. doi: 10.2196/22591. JMIR Mhealth Uhealth. 2021. PMID: 33955840 Free PMC article.
-
A review on utilizing machine learning technology in the fields of electronic emergency triage and patient priority systems in telemedicine: Coherent taxonomy, motivations, open research challenges and recommendations for intelligent future work.Comput Methods Programs Biomed. 2021 Sep;209:106357. doi: 10.1016/j.cmpb.2021.106357. Epub 2021 Aug 16. Comput Methods Programs Biomed. 2021. PMID: 34438223 Review.
-
Decoding the Chronic Obstructive Pulmonary Disease (COPD) Puzzle: Investigating the Significance of Exacerbation Scores in Triage Decision-Making.Cureus. 2023 Jul 16;15(7):e41975. doi: 10.7759/cureus.41975. eCollection 2023 Jul. Cureus. 2023. PMID: 37593292 Free PMC article. Review.
Cited by
-
Fast Prediction of Deterioration and Death Risk in Patients With Acute Exacerbation of Chronic Obstructive Pulmonary Disease Using Vital Signs and Admission History: Retrospective Cohort Study.JMIR Med Inform. 2019 Oct 21;7(4):e13085. doi: 10.2196/13085. JMIR Med Inform. 2019. PMID: 31638595 Free PMC article.
-
Pre-hospital and emergency department pathways of care for exacerbations of chronic obstructive pulmonary disease (COPD).J Thorac Dis. 2019 Oct;11(Suppl 17):S2221-S2229. doi: 10.21037/jtd.2019.10.37. J Thorac Dis. 2019. PMID: 31737349 Free PMC article. Review.
-
How accurate are digital symptom assessment apps for suggesting conditions and urgency advice? A clinical vignettes comparison to GPs.BMJ Open. 2020 Dec 16;10(12):e040269. doi: 10.1136/bmjopen-2020-040269. BMJ Open. 2020. PMID: 33328258 Free PMC article.
-
Development and validation of a nomogram to predict pulmonary function and the presence of chronic obstructive pulmonary disease in a Korean population.BMC Pulm Med. 2021 Jan 19;21(1):32. doi: 10.1186/s12890-021-01391-z. BMC Pulm Med. 2021. PMID: 33468128 Free PMC article.
-
Forecast the Exacerbation in Patients of Chronic Obstructive Pulmonary Disease with Clinical Indicators Using Machine Learning Techniques.Diagnostics (Basel). 2021 May 4;11(5):829. doi: 10.3390/diagnostics11050829. Diagnostics (Basel). 2021. PMID: 34064395 Free PMC article.
References
-
- Dransfield MT, Kunisaki KM, Strand MJ, Anzueto A, Bhatt SP, Bowler RP, et al. Acute Exacerbations and Lung Function Loss in Smokers with and without Chronic Obstructive Pulmonary Disease. Am J Respir Crit Care Med. 2017;195(3):324–330. doi: 10.1164/rccm.201605-1014OC - DOI - PMC - PubMed
-
- Rennard SI, Farmer SG. Exacerbations and Progression of Disease in Asthma and Chronic Obstructive Pulmonary Disease. Proc Am Thorac Soc. 2004;1(2):88–92. doi: 10.1513/pats.2306026 - DOI - PubMed
-
- World Health Organization. Global Surveillance, prevention and control of chronic respiratory diseases: A comprehensive approach; 2007.
-
- Watson PB, Town GI, Holbrook N, Dwan C, Toop LJ, Drennan CJ. Evaluation of a Self-Management Plan for Chronic Obstructive Pulmonary Disease. European Respiratory Review. 1997;10:1267–1271. doi: 10.1183/09031936.97.10061267 - DOI - PubMed
MeSH terms
Grants and funding
LinkOut - more resources
Full Text Sources
Other Literature Sources
Medical