Evaluation of a Dry EEG System for Application of Passive Brain-Computer Interfaces in Autonomous Driving
- PMID: 28293184
- PMCID: PMC5329046
- DOI: 10.3389/fnhum.2017.00078
Evaluation of a Dry EEG System for Application of Passive Brain-Computer Interfaces in Autonomous Driving
Abstract
We tested the applicability and signal quality of a 16 channel dry electroencephalography (EEG) system in a laboratory environment and in a car under controlled, realistic conditions. The aim of our investigation was an estimation how well a passive Brain-Computer Interface (pBCI) can work in an autonomous driving scenario. The evaluation considered speed and accuracy of self-applicability by an untrained person, quality of recorded EEG data, shifts of electrode positions on the head after driving-related movements, usability, and complexity of the system as such and wearing comfort over time. An experiment was conducted inside and outside of a stationary vehicle with running engine, air-conditioning, and muted radio. Signal quality was sufficient for standard EEG analysis in the time and frequency domain as well as for the use in pBCIs. While the influence of vehicle-induced interferences to data quality was insignificant, driving-related movements led to strong shifts in electrode positions. In general, the EEG system used allowed for a fast self-applicability of cap and electrodes. The assessed usability of the system was still acceptable while the wearing comfort decreased strongly over time due to friction and pressure to the head. From these results we conclude that the evaluated system should provide the essential requirements for an application in an autonomous driving context. Nevertheless, further refinement is suggested to reduce shifts of the system due to body movements and increase the headset's usability and wearing comfort.
Keywords: EEG; ERP; autonomous driving; passive BCI; usability.
Figures
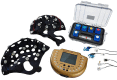
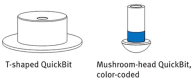
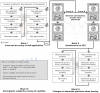
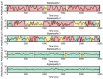
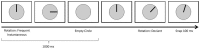
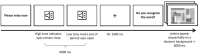
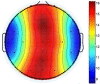
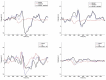
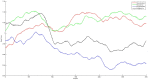
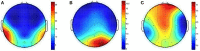
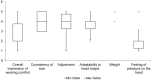
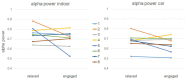
Similar articles
-
Dry EEG in Sports Sciences: A Fast and Reliable Tool to Assess Individual Alpha Peak Frequency Changes Induced by Physical Effort.Front Neurosci. 2019 Sep 20;13:982. doi: 10.3389/fnins.2019.00982. eCollection 2019. Front Neurosci. 2019. PMID: 31619953 Free PMC article.
-
A high-speed brain-computer interface (BCI) using dry EEG electrodes.PLoS One. 2017 Feb 22;12(2):e0172400. doi: 10.1371/journal.pone.0172400. eCollection 2017. PLoS One. 2017. PMID: 28225794 Free PMC article.
-
A Dry EEG-System for Scientific Research and Brain-Computer Interfaces.Front Neurosci. 2011 May 26;5:53. doi: 10.3389/fnins.2011.00053. eCollection 2011. Front Neurosci. 2011. PMID: 21647345 Free PMC article.
-
The Arch Electrode: A Novel Dry Electrode Concept for Improved Wearing Comfort.Front Neurosci. 2021 Oct 18;15:748100. doi: 10.3389/fnins.2021.748100. eCollection 2021. Front Neurosci. 2021. PMID: 34733134 Free PMC article.
-
Passive BCI in Operational Environments: Insights, Recent Advances, and Future Trends.IEEE Trans Biomed Eng. 2017 Jul;64(7):1431-1436. doi: 10.1109/TBME.2017.2694856. Epub 2017 Apr 17. IEEE Trans Biomed Eng. 2017. PMID: 28436837 Review.
Cited by
-
Hybrid Systems to Boost EEG-Based Real-Time Action Decoding in Car Driving Scenarios.Front Neuroergon. 2021 Nov 29;2:784827. doi: 10.3389/fnrgo.2021.784827. eCollection 2021. Front Neuroergon. 2021. PMID: 38235223 Free PMC article.
-
The Sample Size Matters: To What Extent the Participant Reduction Affects the Outcomes of a Neuroscientific Research. A Case-Study in Neuromarketing Field.Sensors (Basel). 2021 Sep 10;21(18):6088. doi: 10.3390/s21186088. Sensors (Basel). 2021. PMID: 34577294 Free PMC article.
-
Categorisation of Mobile EEG: A Researcher's Perspective.Biomed Res Int. 2017;2017:5496196. doi: 10.1155/2017/5496196. Epub 2017 Dec 4. Biomed Res Int. 2017. PMID: 29349078 Free PMC article. Review.
-
Comprehensive evaluation methods for translating BCI into practical applications: usability, user satisfaction and usage of online BCI systems.Front Hum Neurosci. 2024 Jun 5;18:1429130. doi: 10.3389/fnhum.2024.1429130. eCollection 2024. Front Hum Neurosci. 2024. PMID: 38903409 Free PMC article. Review.
-
Estimating Cognitive Workload in an Interactive Virtual Reality Environment Using EEG.Front Hum Neurosci. 2019 Nov 14;13:401. doi: 10.3389/fnhum.2019.00401. eCollection 2019. Front Hum Neurosci. 2019. PMID: 31803035 Free PMC article.
References
-
- Beiker S. A. (2012). Symposium, Legal Aspects of Autonomous Driving, 52. Santa Clara, CA, 1145.
-
- Berka C., Levendowski D. J., Lumicao M. N., Yau A., Davis G., Zivkovic V. T., et al. . (2007). EEG correlates of task engagement and mental workload in vigilance, learning, and memory tasks. Aviat. Space Environ. Med. 78(Suppl. 1), B231–B244. - PubMed
LinkOut - more resources
Full Text Sources
Other Literature Sources
Miscellaneous