Online Learners' Reading Ability Detection Based on Eye-Tracking Sensors
- PMID: 27626418
- PMCID: PMC5038735
- DOI: 10.3390/s16091457
Online Learners' Reading Ability Detection Based on Eye-Tracking Sensors
Abstract
The detection of university online learners' reading ability is generally problematic and time-consuming. Thus the eye-tracking sensors have been employed in this study, to record temporal and spatial human eye movements. Learners' pupils, blinks, fixation, saccade, and regression are recognized as primary indicators for detecting reading abilities. A computational model is established according to the empirical eye-tracking data, and applying the multi-feature regularization machine learning mechanism based on a Low-rank Constraint. The model presents good generalization ability with an error of only 4.9% when randomly running 100 times. It has obvious advantages in saving time and improving precision, with only 20 min of testing required for prediction of an individual learner's reading ability.
Keywords: computational model; eye-tracking sensors; online learner; reading ability detection.
Conflict of interest statement
The authors declare no conflict of interest.
Figures
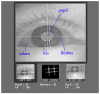
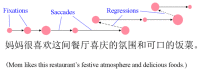
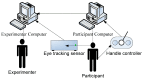
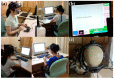
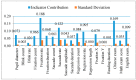
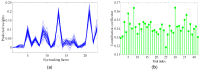
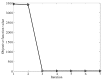
Similar articles
-
Mind Mapping Training's Effects on Reading Ability: Detection Based on Eye Tracking Sensors.Sensors (Basel). 2020 Aug 7;20(16):4422. doi: 10.3390/s20164422. Sensors (Basel). 2020. PMID: 32784791 Free PMC article.
-
Binocularity during reading fixations: Properties of the minimum fixation disparity.Vision Res. 2010 Aug 23;50(18):1775-85. doi: 10.1016/j.visres.2010.05.033. Epub 2010 Jun 1. Vision Res. 2010. PMID: 20573592
-
Effects of voluntary blinks on saccades, vergence eye movements, and saccade-vergence interactions in humans.J Neurophysiol. 2002 Sep;88(3):1220-33. doi: 10.1152/jn.2002.88.3.1220. J Neurophysiol. 2002. PMID: 12205143
-
Eye-movement models for arithmetic and reading performance.Rev Oculomot Res. 1990;4:455-77. Rev Oculomot Res. 1990. PMID: 7492535 Review.
-
Coregistration of eye movements and EEG in natural reading: analyses and review.J Exp Psychol Gen. 2011 Nov;140(4):552-72. doi: 10.1037/a0023885. J Exp Psychol Gen. 2011. PMID: 21744985 Review.
Cited by
-
Impact of Think-Aloud on Eye-Tracking: A Comparison of Concurrent and Retrospective Think-Aloud for Research on Decision-Making in the Game Environment.Sensors (Basel). 2020 May 12;20(10):2750. doi: 10.3390/s20102750. Sensors (Basel). 2020. PMID: 32408507 Free PMC article.
-
State-Aware Deep Item Response Theory using student facial features.Front Artif Intell. 2024 Jan 4;6:1324279. doi: 10.3389/frai.2023.1324279. eCollection 2023. Front Artif Intell. 2024. PMID: 38239499 Free PMC article.
-
Implementation and Evaluation of a Wide-Range Human-Sensing System Based on Cooperating Multiple Range Image Sensors.Sensors (Basel). 2019 Mar 7;19(5):1172. doi: 10.3390/s19051172. Sensors (Basel). 2019. PMID: 30866558 Free PMC article.
-
Don't overthink it: The paradoxical nature of expertise for the detection of errors in conceptual business process models.Front Neurosci. 2022 Nov 24;16:982764. doi: 10.3389/fnins.2022.982764. eCollection 2022. Front Neurosci. 2022. PMID: 36507322 Free PMC article.
-
Evaluating Technicians' Workload and Performance in Diagnosis for Corrective Maintenance.Sensors (Basel). 2024 Mar 18;24(6):1943. doi: 10.3390/s24061943. Sensors (Basel). 2024. PMID: 38544206 Free PMC article.
References
-
- Grubb M. Ph.D. Thesis. University of Houston; Houston, TX, USA: 2011. Predictors of High School Student Success in Online Courses.
-
- Hyönä J. The use of eye movements in the study of multimedia learning. Learn. Instr. 2010;20:172–176. doi: 10.1016/j.learninstruc.2009.02.013. - DOI
MeSH terms
Grants and funding
LinkOut - more resources
Full Text Sources
Other Literature Sources