RACOG and wRACOG: Two Probabilistic Oversampling Techniques
- PMID: 27041974
- PMCID: PMC4814938
- DOI: 10.1109/TKDE.2014.2324567
RACOG and wRACOG: Two Probabilistic Oversampling Techniques
Abstract
As machine learning techniques mature and are used to tackle complex scientific problems, challenges arise such as the imbalanced class distribution problem, where one of the target class labels is under-represented in comparison with other classes. Existing oversampling approaches for addressing this problem typically do not consider the probability distribution of the minority class while synthetically generating new samples. As a result, the minority class is not well represented which leads to high misclassification error. We introduce two Gibbs sampling-based oversampling approaches, namely RACOG and wRACOG, to synthetically generating and strategically selecting new minority class samples. The Gibbs sampler uses the joint probability distribution of attributes of the data to generate new minority class samples in the form of Markov chain. While RACOG selects samples from the Markov chain based on a predefined lag, wRACOG selects those samples that have the highest probability of being misclassified by the existing learning model. We validate our approach using five UCI datasets that were carefully modified to exhibit class imbalance and one new application domain dataset with inherent extreme class imbalance. In addition, we compare the classification performance of the proposed methods with three other existing resampling techniques.
Keywords: Gibbs sampling; Imbalanced class distribution; Markov chain Monte Carlo (MCMC); oversampling.
Figures

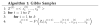
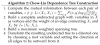
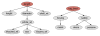
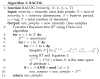
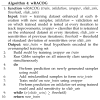
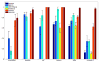
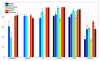
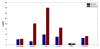
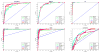
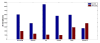
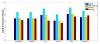
Similar articles
-
RSMOTE: improving classification performance over imbalanced medical datasets.Health Inf Sci Syst. 2020 Jun 12;8(1):22. doi: 10.1007/s13755-020-00112-w. eCollection 2020 Dec. Health Inf Sci Syst. 2020. PMID: 32549976 Free PMC article.
-
Iterative nearest neighborhood oversampling in semisupervised learning from imbalanced data.ScientificWorldJournal. 2013 Jul 10;2013:875450. doi: 10.1155/2013/875450. Print 2013. ScientificWorldJournal. 2013. PMID: 23935439 Free PMC article.
-
Comparison of Resampling Techniques for Imbalanced Datasets in Machine Learning: Application to Epileptogenic Zone Localization From Interictal Intracranial EEG Recordings in Patients With Focal Epilepsy.Front Neuroinform. 2021 Nov 19;15:715421. doi: 10.3389/fninf.2021.715421. eCollection 2021. Front Neuroinform. 2021. PMID: 34867255 Free PMC article.
-
A comprehensive data level analysis for cancer diagnosis on imbalanced data.J Biomed Inform. 2019 Feb;90:103089. doi: 10.1016/j.jbi.2018.12.003. Epub 2019 Jan 3. J Biomed Inform. 2019. PMID: 30611011 Review.
-
A review on over-sampling techniques in classification of multi-class imbalanced datasets: insights for medical problems.Front Digit Health. 2024 Jul 26;6:1430245. doi: 10.3389/fdgth.2024.1430245. eCollection 2024. Front Digit Health. 2024. PMID: 39131184 Free PMC article. Review.
Cited by
-
An Impartial Semi-Supervised Learning Strategy for Imbalanced Classification on VHR Images.Sensors (Basel). 2020 Nov 23;20(22):6699. doi: 10.3390/s20226699. Sensors (Basel). 2020. PMID: 33238513 Free PMC article.
-
Research on Adaptive 1DCNN Network Intrusion Detection Technology Based on BSGM Mixed Sampling.Sensors (Basel). 2023 Jul 6;23(13):6206. doi: 10.3390/s23136206. Sensors (Basel). 2023. PMID: 37448055 Free PMC article.
-
Artificial-Intelligence-Based Prediction of Clinical Events among Hemodialysis Patients Using Non-Contact Sensor Data.Sensors (Basel). 2018 Aug 27;18(9):2833. doi: 10.3390/s18092833. Sensors (Basel). 2018. PMID: 30150592 Free PMC article.
-
Evolutionary Mahalanobis Distance-Based Oversampling for Multi-Class Imbalanced Data Classification.Sensors (Basel). 2021 Oct 4;21(19):6616. doi: 10.3390/s21196616. Sensors (Basel). 2021. PMID: 34640936 Free PMC article.
References
-
- Woods K, Doss C, Bowyer K, Solka J, Priebe C, KW Comparative evaluation of pattern recognition techniques for detection of microcalcifications in mammography. International Journal of Pattern Recognition and Artificial Intelligence. 1993;7(6):1417–1436.
-
- Kubat M, Holte R, Matwin S. Machine learning for the detection of oil spills in satellite radar images. Machine learning. 1998;30(2):195–215.
-
- Phua C, Alahakoon D, Lee V. Minority report in fraud detection: classification of skewed data. ACM SIGKDD Explorations Newsletter. 2004;6(1):50–59.
-
- Turney P, et al. Learning algorithms for keyphrase extraction. Information Retrieval. 2000;2(4):303–336.
-
- Lewis D, Gale W. A sequential algorithm for training text classifiers. Proceedings of the 17th Annual International ACM SIGIR Conference on Research and Development in Information Retrieval; Springer-Verlag New York, Inc; 1994. pp. 3–12.
Grants and funding
LinkOut - more resources
Full Text Sources
Other Literature Sources
Research Materials