Machine Learning in Medicine
- PMID: 26572668
- PMCID: PMC5831252
- DOI: 10.1161/CIRCULATIONAHA.115.001593
Machine Learning in Medicine
Abstract
Spurred by advances in processing power, memory, storage, and an unprecedented wealth of data, computers are being asked to tackle increasingly complex learning tasks, often with astonishing success. Computers have now mastered a popular variant of poker, learned the laws of physics from experimental data, and become experts in video games - tasks that would have been deemed impossible not too long ago. In parallel, the number of companies centered on applying complex data analysis to varying industries has exploded, and it is thus unsurprising that some analytic companies are turning attention to problems in health care. The purpose of this review is to explore what problems in medicine might benefit from such learning approaches and use examples from the literature to introduce basic concepts in machine learning. It is important to note that seemingly large enough medical data sets and adequate learning algorithms have been available for many decades, and yet, although there are thousands of papers applying machine learning algorithms to medical data, very few have contributed meaningfully to clinical care. This lack of impact stands in stark contrast to the enormous relevance of machine learning to many other industries. Thus, part of my effort will be to identify what obstacles there may be to changing the practice of medicine through statistical learning approaches, and discuss how these might be overcome.
Keywords: artificial intelligence; computers; prognosis; risk factors; statistics.
© 2015 American Heart Association, Inc.
Conflict of interest statement
Figures
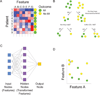
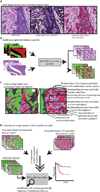
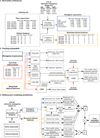
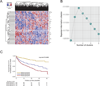
Similar articles
-
Predictive analytics and machine learning in stroke and neurovascular medicine.Neurol Res. 2019 Aug;41(8):681-690. doi: 10.1080/01616412.2019.1609159. Epub 2019 Apr 30. Neurol Res. 2019. PMID: 31038007 Review.
-
eDoctor: machine learning and the future of medicine.J Intern Med. 2018 Dec;284(6):603-619. doi: 10.1111/joim.12822. Epub 2018 Sep 3. J Intern Med. 2018. PMID: 30102808 Review.
-
Artificial intelligence and machine learning in emergency medicine.Emerg Med Australas. 2018 Dec;30(6):870-874. doi: 10.1111/1742-6723.13145. Epub 2018 Jul 16. Emerg Med Australas. 2018. PMID: 30014578
-
Artificial intelligence in reproductive medicine.Reproduction. 2019 Oct;158(4):R139-R154. doi: 10.1530/REP-18-0523. Reproduction. 2019. PMID: 30970326 Free PMC article. Review.
-
Artificial Intelligence and Applications in PM&R.Am J Phys Med Rehabil. 2019 Nov;98(11):e128-e129. doi: 10.1097/PHM.0000000000001171. Am J Phys Med Rehabil. 2019. PMID: 30839314
Cited by
-
Developing clinical prediction models: a step-by-step guide.BMJ. 2024 Sep 3;386:e078276. doi: 10.1136/bmj-2023-078276. BMJ. 2024. PMID: 39227063 Free PMC article.
-
Identification of diagnostic biomarkers of rheumatoid arthritis based on machine learning-assisted comprehensive bioinformatics and its correlation with immune cells.Heliyon. 2024 Aug 5;10(15):e35511. doi: 10.1016/j.heliyon.2024.e35511. eCollection 2024 Aug 15. Heliyon. 2024. PMID: 39170142 Free PMC article.
-
Alternative Splicing, Internal Promoter, Nonsense-Mediated Decay, or All Three: Explaining the Distribution of Truncation Variants in Titin.Circ Cardiovasc Genet. 2016 Oct;9(5):419-425. doi: 10.1161/CIRCGENETICS.116.001513. Epub 2016 Sep 13. Circ Cardiovasc Genet. 2016. PMID: 27625338 Free PMC article.
-
Application of machine learning model to predict osteoporosis based on abdominal computed tomography images of the psoas muscle: a retrospective study.BMC Geriatr. 2022 Oct 13;22(1):796. doi: 10.1186/s12877-022-03502-9. BMC Geriatr. 2022. PMID: 36229793 Free PMC article.
-
Machine learning outperforms traditional logistic regression and offers new possibilities for cardiovascular risk prediction: A study involving 143,043 Chinese patients with hypertension.Front Cardiovasc Med. 2022 Nov 14;9:1025705. doi: 10.3389/fcvm.2022.1025705. eCollection 2022. Front Cardiovasc Med. 2022. PMID: 36451926 Free PMC article.
References
-
- Hastie T, Tibshirani R, Friedman J. The Elements of Statistical Learning. New York, NY: Springer Science & Business Media; 2009.
-
- Abu-Mostafa YS, Magdon-Ismail M, Lin H-T. Learning from Data. 2012 AMLbook.com.
-
- Kannel WB, Doyle JT, McNamara PM, Quickenton P, Gordon T. Precursors of sudden coronary death. Factors related to the incidence of sudden death. Circulation. 1975;51:606–613. - PubMed
-
- Lip GYH, Nieuwlaat R, Pisters R, Lane DA, Crijns HJGM. Refining clinical risk stratification for predicting stroke and thromboembolism in atrial fibrillation using a novel risk factor-based approach: the euro heart survey on atrial fibrillation. Chest. 2010;137:263–272. - PubMed
-
- O'Mahony C, Jichi F, Pavlou M, Monserrat L, Anastasakis A, Rapezzi C, Biagini E, Gimeno JR, Limongelli G, McKenna WJ, Omar RZ, Elliott PM. A novel clinical risk prediction model for sudden cardiac death in hypertrophic cardiomyopathy (HCM risk-SCD) Eur Heart J. 2014;35:2010–2020. - PubMed
Publication types
MeSH terms
Grants and funding
LinkOut - more resources
Full Text Sources
Other Literature Sources