Computational evaluation of exome sequence data using human and model organism phenotypes improves diagnostic efficiency
- PMID: 26562225
- PMCID: PMC4916229
- DOI: 10.1038/gim.2015.137
Computational evaluation of exome sequence data using human and model organism phenotypes improves diagnostic efficiency
Abstract
Purpose: Medical diagnosis and molecular or biochemical confirmation typically rely on the knowledge of the clinician. Although this is very difficult in extremely rare diseases, we hypothesized that the recording of patient phenotypes in Human Phenotype Ontology (HPO) terms and computationally ranking putative disease-associated sequence variants improves diagnosis, particularly for patients with atypical clinical profiles.
Methods: Using simulated exomes and the National Institutes of Health Undiagnosed Diseases Program (UDP) patient cohort and associated exome sequence, we tested our hypothesis using Exomiser. Exomiser ranks candidate variants based on patient phenotype similarity to (i) known disease-gene phenotypes, (ii) model organism phenotypes of candidate orthologs, and (iii) phenotypes of protein-protein association neighbors.
Results: Benchmarking showed Exomiser ranked the causal variant as the top hit in 97% of known disease-gene associations and ranked the correct seeded variant in up to 87% when detectable disease-gene associations were unavailable. Using UDP data, Exomiser ranked the causative variant(s) within the top 10 variants for 11 previously diagnosed variants and achieved a diagnosis for 4 of 23 cases undiagnosed by clinical evaluation.
Conclusion: Structured phenotyping of patients and computational analysis are effective adjuncts for diagnosing patients with genetic disorders.Genet Med 18 6, 608-617.
Figures
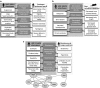
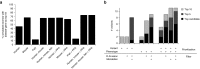
Similar articles
-
An Improved Phenotype-Driven Tool for Rare Mendelian Variant Prioritization: Benchmarking Exomiser on Real Patient Whole-Exome Data.Genes (Basel). 2020 Apr 23;11(4):460. doi: 10.3390/genes11040460. Genes (Basel). 2020. PMID: 32340307 Free PMC article.
-
Increasing phenotypic annotation improves the diagnostic rate of exome sequencing in a rare neuromuscular disorder.Hum Mutat. 2019 Oct;40(10):1797-1812. doi: 10.1002/humu.23792. Epub 2019 Jun 23. Hum Mutat. 2019. PMID: 31231902
-
A pipeline combining multiple strategies for prioritizing heterozygous variants for the identification of candidate genes in exome datasets.Hum Genomics. 2017 May 22;11(1):11. doi: 10.1186/s40246-017-0107-5. Hum Genomics. 2017. PMID: 28532469 Free PMC article.
-
Diagnosing rare diseases after the exome.Cold Spring Harb Mol Case Stud. 2018 Dec 17;4(6):a003392. doi: 10.1101/mcs.a003392. Print 2018 Dec. Cold Spring Harb Mol Case Stud. 2018. PMID: 30559314 Free PMC article. Review.
-
Disease insights through cross-species phenotype comparisons.Mamm Genome. 2015 Oct;26(9-10):548-55. doi: 10.1007/s00335-015-9577-8. Epub 2015 Jun 20. Mamm Genome. 2015. PMID: 26092691 Free PMC article. Review.
Cited by
-
Genomic characterization and computational phenotyping of nitrogen-fixing bacteria isolated from Colombian sugarcane fields.Sci Rep. 2021 Apr 28;11(1):9187. doi: 10.1038/s41598-021-88380-8. Sci Rep. 2021. PMID: 33911103 Free PMC article.
-
The undiagnosed diseases program: Approach to diagnosis.Transl Sci Rare Dis. 2020 Apr 13;4(3-4):179-188. doi: 10.3233/TRD-190045. Transl Sci Rare Dis. 2020. PMID: 32477883 Free PMC article.
-
New insights into the generation and role of de novo mutations in health and disease.Genome Biol. 2016 Nov 28;17(1):241. doi: 10.1186/s13059-016-1110-1. Genome Biol. 2016. PMID: 27894357 Free PMC article. Review.
-
Encoding Clinical Data with the Human Phenotype Ontology for Computational Differential Diagnostics.Curr Protoc Hum Genet. 2019 Sep;103(1):e92. doi: 10.1002/cphg.92. Curr Protoc Hum Genet. 2019. PMID: 31479590 Free PMC article.
-
Applications of molecular networks in biomedicine.Biol Methods Protoc. 2019 Sep 23;4(1):bpz012. doi: 10.1093/biomethods/bpz012. eCollection 2019. Biol Methods Protoc. 2019. PMID: 32395629 Free PMC article. Review.
References
-
- Gahl WA, Tifft CJ. The NIH Undiagnosed Diseases Program: lessons learned. JAMA 2011;305:1904–1905. - PubMed
Publication types
MeSH terms
Grants and funding
LinkOut - more resources
Full Text Sources
Other Literature Sources
Medical