Compressive Sensing of Roller Bearing Faults via Harmonic Detection from Under-Sampled Vibration Signals
- PMID: 26473858
- PMCID: PMC4634422
- DOI: 10.3390/s151025648
Compressive Sensing of Roller Bearing Faults via Harmonic Detection from Under-Sampled Vibration Signals
Abstract
The Shannon sampling principle requires substantial amounts of data to ensure the accuracy of on-line monitoring of roller bearing fault signals. Challenges are often encountered as a result of the cumbersome data monitoring, thus a novel method focused on compressed vibration signals for detecting roller bearing faults is developed in this study. Considering that harmonics often represent the fault characteristic frequencies in vibration signals, a compressive sensing frame of characteristic harmonics is proposed to detect bearing faults. A compressed vibration signal is first acquired from a sensing matrix with information preserved through a well-designed sampling strategy. A reconstruction process of the under-sampled vibration signal is then pursued as attempts are conducted to detect the characteristic harmonics from sparse measurements through a compressive matching pursuit strategy. In the proposed method bearing fault features depend on the existence of characteristic harmonics, as typically detected directly from compressed data far before reconstruction completion. The process of sampling and detection may then be performed simultaneously without complete recovery of the under-sampled signals. The effectiveness of the proposed method is validated by simulations and experiments.
Keywords: compressive sensing; fault detection; harmonic detection; matching pursuit; roller bearing.
Figures
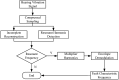
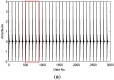
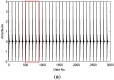
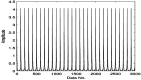
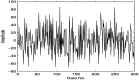
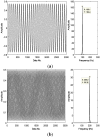
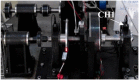
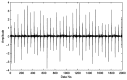
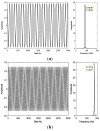
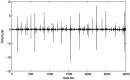
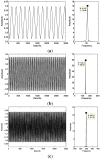
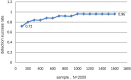
Similar articles
-
A Sparsity-Promoted Decomposition for Compressed Fault Diagnosis of Roller Bearings.Sensors (Basel). 2016 Sep 19;16(9):1524. doi: 10.3390/s16091524. Sensors (Basel). 2016. PMID: 27657063 Free PMC article.
-
Intrinsic Dimension Estimation-Based Feature Selection and Multinomial Logistic Regression for Classification of Bearing Faults Using Compressively Sampled Vibration Signals.Entropy (Basel). 2022 Apr 5;24(4):511. doi: 10.3390/e24040511. Entropy (Basel). 2022. PMID: 35455173 Free PMC article.
-
Diagnosis of Compound Fault Using Sparsity Promoted-Based Sparse Component Analysis.Sensors (Basel). 2017 Jun 6;17(6):1307. doi: 10.3390/s17061307. Sensors (Basel). 2017. PMID: 28587296 Free PMC article.
-
Sparse Recovery Optimization in Wireless Sensor Networks with a Sub-Nyquist Sampling Rate.Sensors (Basel). 2015 Jul 10;15(7):16654-73. doi: 10.3390/s150716654. Sensors (Basel). 2015. PMID: 26184203 Free PMC article. Review.
-
Resonance-Based Sparse Signal Decomposition and its Application in Mechanical Fault Diagnosis: A Review.Sensors (Basel). 2017 Jun 3;17(6):1279. doi: 10.3390/s17061279. Sensors (Basel). 2017. PMID: 28587198 Free PMC article. Review.
Cited by
-
A Machine Condition Monitoring Framework Using Compressed Signal Processing.Sensors (Basel). 2020 Jan 6;20(1):319. doi: 10.3390/s20010319. Sensors (Basel). 2020. PMID: 31935948 Free PMC article.
-
Bearing Fault Diagnosis Using Piecewise Aggregate Approximation and Complete Ensemble Empirical Mode Decomposition with Adaptive Noise.Sensors (Basel). 2022 Sep 1;22(17):6599. doi: 10.3390/s22176599. Sensors (Basel). 2022. PMID: 36081069 Free PMC article.
-
Compressed Sensing Techniques Applied to Ultrasonic Imaging of Cargo Containers.Sensors (Basel). 2017 Jan 15;17(1):162. doi: 10.3390/s17010162. Sensors (Basel). 2017. PMID: 28098841 Free PMC article.
-
Compressed-Sensing Reconstruction Based on Block Sparse Bayesian Learning in Bearing-Condition Monitoring.Sensors (Basel). 2017 Jun 21;17(6):1454. doi: 10.3390/s17061454. Sensors (Basel). 2017. PMID: 28635623 Free PMC article.
-
Compressive Sensing Approach to Harmonics Detection in the Ship Electrical Network.Sensors (Basel). 2020 May 11;20(9):2744. doi: 10.3390/s20092744. Sensors (Basel). 2020. PMID: 32403441 Free PMC article.
References
-
- McFadden P.D., Smith J.D. Vibration monitoring of rolling element bearings by the high-frequency resonance technique—A review. Tribol. Int. 1984;17:3–10. doi: 10.1016/0301-679X(84)90076-8. - DOI
-
- Tandon N., Choudhury A. A Review of Vibration and Acoustic Measurement Methods for the Detection of Defects in Rolling Element Bearings. Tribol. Int. 1999;32:469–480. doi: 10.1016/S0301-679X(99)00077-8. - DOI
-
- Heng R.B., Nor M.J. Statistical analysis of sound and vibration signals for monitoring rolling element bearing condition. Appl. Acoust. 1998;53:211–226. doi: 10.1016/S0003-682X(97)00018-2. - DOI
-
- Junsheng C., Dejie Y., Yu Y. The application of energy operator demodulation approach based on EMD in machinery fault diagnosis. Mech. Syst. Signal Process. 2007;21:668–677. doi: 10.1016/j.ymssp.2005.10.005. - DOI
-
- Ming A.B., Qin Z.Y., Zhang W., Chu F.L. Spectrum auto-correlation analysis and its application to fault diagnosis of rolling element bearings. Mech. Syst. Signal Process. 2013;41:141–154. doi: 10.1016/j.ymssp.2013.08.004. - DOI
LinkOut - more resources
Full Text Sources
Other Literature Sources