Combining HJ CCD, GF-1 WFV and MODIS Data to Generate Daily High Spatial Resolution Synthetic Data for Environmental Process Monitoring
- PMID: 26308017
- PMCID: PMC4555320
- DOI: 10.3390/ijerph120809920
Combining HJ CCD, GF-1 WFV and MODIS Data to Generate Daily High Spatial Resolution Synthetic Data for Environmental Process Monitoring
Abstract
The limitations of satellite data acquisition mean that there is a lack of satellite data with high spatial and temporal resolutions for environmental process monitoring. In this study, we address this problem by applying the Enhanced Spatial and Temporal Adaptive Reflectance Fusion Model (ESTARFM) and the Spatial and Temporal Data Fusion Approach (STDFA) to combine Huanjing satellite charge coupled device (HJ CCD), Gaofen satellite no. 1 wide field of view camera (GF-1 WFV) and Moderate Resolution Imaging Spectroradiometer (MODIS) data to generate daily high spatial resolution synthetic data for land surface process monitoring. Actual HJ CCD and GF-1 WFV data were used to evaluate the precision of the synthetic images using the correlation analysis method. Our method was tested and validated for two study areas in Xinjiang Province, China. The results show that both the ESTARFM and STDFA can be applied to combine HJ CCD and MODIS reflectance data, and GF-1 WFV and MODIS reflectance data, to generate synthetic HJ CCD data and synthetic GF-1 WFV data that closely match actual data with correlation coefficients (r) greater than 0.8989 and 0.8643, respectively. Synthetic red- and near infrared (NIR)-band data generated by ESTARFM are more suitable for the calculation of Normalized Different Vegetation Index (NDVI) than the data generated by STDFA.
Keywords: ESTARFM; GF-1 WFV; HJ CCD; STDFA.
Figures
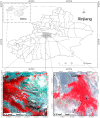
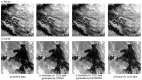
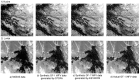
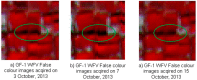
Similar articles
-
Generating Daily Synthetic Landsat Imagery by Combining Landsat and MODIS Data.Sensors (Basel). 2015 Sep 18;15(9):24002-25. doi: 10.3390/s150924002. Sensors (Basel). 2015. PMID: 26393607 Free PMC article.
-
Generating daily high spatial land surface temperatures by combining ASTER and MODIS land surface temperature products for environmental process monitoring.Environ Sci Process Impacts. 2015 Aug;17(8):1396-404. doi: 10.1039/c5em00254k. Epub 2015 Jul 13. Environ Sci Process Impacts. 2015. PMID: 26165141
-
[Identification of forest vegetation types in southern China based on spatio-temporal fusion of GF-1 WFV and MODIS data].Ying Yong Sheng Tai Xue Bao. 2022 Jul;33(7):1948-1956. doi: 10.13287/j.1001-9332.202207.022. Ying Yong Sheng Tai Xue Bao. 2022. PMID: 36052799 Chinese.
-
Estimation of Daily Terrestrial Latent Heat Flux with High Spatial Resolution from MODIS and Chinese GF-1 Data.Sensors (Basel). 2020 May 15;20(10):2811. doi: 10.3390/s20102811. Sensors (Basel). 2020. PMID: 32429110 Free PMC article.
-
A Learning-Enhanced Two-Pair Spatiotemporal Reflectance Fusion Model for GF-2 and GF-1 WFV Satellite Data.Sensors (Basel). 2020 Mar 24;20(6):1789. doi: 10.3390/s20061789. Sensors (Basel). 2020. PMID: 32213863 Free PMC article.
Cited by
-
Generating Daily Synthetic Landsat Imagery by Combining Landsat and MODIS Data.Sensors (Basel). 2015 Sep 18;15(9):24002-25. doi: 10.3390/s150924002. Sensors (Basel). 2015. PMID: 26393607 Free PMC article.
-
Vegetation Dynamic Assessment by NDVI and Field Observations for Sustainability of China's Wulagai River Basin.Int J Environ Res Public Health. 2021 Mar 4;18(5):2528. doi: 10.3390/ijerph18052528. Int J Environ Res Public Health. 2021. PMID: 33806311 Free PMC article.
-
Comparative Analysis of GF-1 and HJ-1 Data to Derive the Optimal Scale for Monitoring Heavy Metal Stress in Rice.Int J Environ Res Public Health. 2018 Mar 6;15(3):461. doi: 10.3390/ijerph15030461. Int J Environ Res Public Health. 2018. PMID: 29509724 Free PMC article.
References
-
- Politi E., Cutler M.E.J., Rowan J.S. Using the NOAA Advanced Very High Resolution Radiometer to characterise temporal and spatial trends in water temperature of large European lakes. Remote Sens. Environ. 2012;126:1–11. doi: 10.1016/j.rse.2012.08.004. - DOI
-
- Maisongrande P., Duchemin B., Dedieu G. VEGETATION/SPOT: An operational mission for the Earth monitoring; presentation of new standard products. Int. J. Remote Sens. 2004;25:9–14. doi: 10.1080/0143116031000115265. - DOI
-
- Salomonson V.V., Barnes W.L., Maymon P.W., Montgomery H.E., Ostrow H. MODIS: Advanced facility instrument for studies of the earth as a system. IEEE Trans. Geosci. Remote Sens. 1992:145–153. doi: 10.1109/36.20292. - DOI
-
- Zhou H., Aizen E., Aizen V. Deriving long term snow cover extent dataset from AVHRR and MODIS data: Central Asia case study. Remote Sens. Environ. 2013;136:146–162. doi: 10.1016/j.rse.2013.04.015. - DOI
-
- Yagoub H., Belbachir A.H., Benabadji N. Detection and mapping vegetation cover based on the Spectral Angle Mapper algorithm using NOAA AVHRR data. Adv. Space Res. 2014;53:1686–1693. doi: 10.1016/j.asr.2014.03.020. - DOI
Publication types
MeSH terms
LinkOut - more resources
Full Text Sources
Other Literature Sources
Miscellaneous