Satellite-Based Spatiotemporal Trends in PM2.5 Concentrations: China, 2004-2013
- PMID: 26220256
- PMCID: PMC4749081
- DOI: 10.1289/ehp.1409481
Satellite-Based Spatiotemporal Trends in PM2.5 Concentrations: China, 2004-2013
Abstract
Background: Three decades of rapid economic development is causing severe and widespread PM2.5 (particulate matter ≤ 2.5 μm) pollution in China. However, research on the health impacts of PM2.5 exposure has been hindered by limited historical PM2.5 concentration data.
Objectives: We estimated ambient PM2.5 concentrations from 2004 to 2013 in China at 0.1° resolution using the most recent satellite data and evaluated model performance with available ground observations.
Methods: We developed a two-stage spatial statistical model using the Moderate Resolution Imaging Spectroradiometer (MODIS) Collection 6 aerosol optical depth (AOD) and assimilated meteorology, land use data, and PM2.5 concentrations from China's recently established ground monitoring network. An inverse variance weighting (IVW) approach was developed to combine MODIS Dark Target and Deep Blue AOD to optimize data coverage. We evaluated model-predicted PM2.5 concentrations from 2004 to early 2014 using ground observations.
Results: The overall model cross-validation R(2) and relative prediction error were 0.79 and 35.6%, respectively. Validation beyond the model year (2013) indicated that it accurately predicted PM2.5 concentrations with little bias at the monthly (R(2) = 0.73, regression slope = 0.91) and seasonal (R(2) = 0.79, regression slope = 0.92) levels. Seasonal variations revealed that winter was the most polluted season and that summer was the cleanest season. Analysis of predicted PM2.5 levels showed a mean annual increase of 1.97 μg/m(3) between 2004 and 2007 and a decrease of 0.46 μg/m(3) between 2008 and 2013.
Conclusions: Our satellite-driven model can provide reliable historical PM2.5 estimates in China at a resolution comparable to those used in epidemiologic studies on the health effects of long-term PM2.5 exposure in North America. This data source can potentially advance research on PM2.5 health effects in China.
Conflict of interest statement
The contents of this publication are solely the responsibility of the grantee and do not necessarily represent the official views of the U.S. EPA. Further, the U.S. EPA does not endorse the purchase of any commercial products or services mentioned in the publication.
The authors declare they have no actual or potential competing financial interests.
Figures
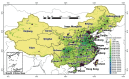
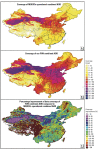
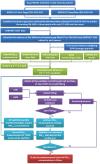
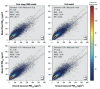
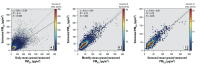
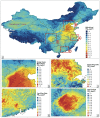
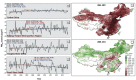
Comment in
-
A Clearer Picture of China's Air: Using Satellite Data and Ground Monitoring to Estimate PM2.5 over Time.Environ Health Perspect. 2016 Feb;124(2):A38. doi: 10.1289/ehp.124-A38. Environ Health Perspect. 2016. PMID: 26829819 Free PMC article. No abstract available.
Similar articles
-
Assessment and statistical modeling of the relationship between remotely sensed aerosol optical depth and PM2.5 in the eastern United States.Res Rep Health Eff Inst. 2012 May;(167):5-83; discussion 85-91. Res Rep Health Eff Inst. 2012. PMID: 22838153
-
Estimating spatiotemporal distribution of PM1 concentrations in China with satellite remote sensing, meteorology, and land use information.Environ Pollut. 2018 Feb;233:1086-1094. doi: 10.1016/j.envpol.2017.10.011. Epub 2017 Oct 13. Environ Pollut. 2018. PMID: 29033176
-
Spatiotemporal continuous estimates of PM2.5 concentrations in China, 2000-2016: A machine learning method with inputs from satellites, chemical transport model, and ground observations.Environ Int. 2019 Feb;123:345-357. doi: 10.1016/j.envint.2018.11.075. Epub 2018 Dec 18. Environ Int. 2019. PMID: 30562706
-
Satellite remote sensing in epidemiological studies.Curr Opin Pediatr. 2016 Apr;28(2):228-34. doi: 10.1097/MOP.0000000000000326. Curr Opin Pediatr. 2016. PMID: 26859287 Free PMC article. Review.
-
Use of earth observations for temperature exposure assessment in epidemiological studies.Curr Opin Pediatr. 2019 Apr;31(2):244-250. doi: 10.1097/MOP.0000000000000735. Curr Opin Pediatr. 2019. PMID: 30640891 Review.
Cited by
-
Understanding sources of fine particulate matter in China.Philos Trans A Math Phys Eng Sci. 2020 Oct 30;378(2183):20190325. doi: 10.1098/rsta.2019.0325. Epub 2020 Sep 28. Philos Trans A Math Phys Eng Sci. 2020. PMID: 32981431 Free PMC article.
-
The Short-Term Effects and Burden of Ambient Air Pollution on Hospitalization for Type 2 Diabetes: Time-Stratified Case-Crossover Evidence From Sichuan, China.Geohealth. 2023 Nov 27;7(11):e2023GH000846. doi: 10.1029/2023GH000846. eCollection 2023 Nov. Geohealth. 2023. PMID: 38023385 Free PMC article.
-
Spatial⁻Temporal Variations in NO₂ and PM2.5 over the Chengdu⁻Chongqing Economic Zone in China during 2005⁻2015 Based on Satellite Remote Sensing.Sensors (Basel). 2018 Nov 15;18(11):3950. doi: 10.3390/s18113950. Sensors (Basel). 2018. PMID: 30445681 Free PMC article.
-
Quantitative Assessment of Relationship between Population Exposure to PM2.5 and Socio-Economic Factors at Multiple Spatial Scales over Mainland China.Int J Environ Res Public Health. 2018 Sep 19;15(9):2058. doi: 10.3390/ijerph15092058. Int J Environ Res Public Health. 2018. PMID: 30235898 Free PMC article.
-
Robust prediction of hourly PM2.5 from meteorological data using LightGBM.Natl Sci Rev. 2021 Jan 5;8(10):nwaa307. doi: 10.1093/nsr/nwaa307. eCollection 2021 Oct. Natl Sci Rev. 2021. PMID: 34858602 Free PMC article.
References
-
- Bontemps S, Defourny P, Van Bogaert E, Arino O, Kalogirou V, Perez JR. GlobCover 2009: Products Description and Validation Report. 2011 Available: http://due.esrin.esa.int/files/GLOBCOVER2009_Validation_Report_2.2.pdf [accessed 9 July 2015]
-
- Boys BL, Martin RV, van Donkelaar A, MacDonell RJ, Hsu NC, Cooper MJ, et al. Fifteen-year global time series of satellite-derived fine particulate matter. Environ Sci Technol. 2014;48:11109–11118. - PubMed
-
- Crouse DL, Peters PA, van Donkelaar A, Goldberg MS, Villeneuve PJ, Brion O, et al. 2012Risk of nonaccidental and cardiovascular mortality in relation to long-term exposure to low concentrations of fine particulate matter: a Canadian national-level cohort study. Environ Health Perspect 120708–714.; doi:10.1289/ehp.1104049 - DOI - PMC - PubMed
-
- Duncan BN, Prados AI, Lamsal LN, Liu Y, Streets DG, Gupta P, et al. Satellite data of atmospheric pollution for U.S. air quality applications: examples of applications, summary of data end-user resources, answers to FAQs, and common mistakes to avoid. Atmos Environ. 2014;94:647–662.
Publication types
MeSH terms
Substances
LinkOut - more resources
Full Text Sources
Other Literature Sources